
- •BUSINESSES IN THE BOOK
- •Preface
- •Brief Contents
- •CONTENTS
- •Why Study Strategy?
- •Why Economics?
- •The Need for Principles
- •So What’s the Problem?
- •Firms or Markets?
- •A Framework for Strategy
- •Boundaries of the Firm
- •Market and Competitive Analysis
- •Positioning and Dynamics
- •Internal Organization
- •The Book
- •Endnotes
- •Costs
- •Cost Functions
- •Total Cost Functions
- •Fixed and Variable Costs
- •Average and Marginal Cost Functions
- •The Importance of the Time Period: Long-Run versus Short-Run Cost Functions
- •Sunk versus Avoidable Costs
- •Economic Costs and Profitability
- •Economic versus Accounting Costs
- •Economic Profit versus Accounting Profit
- •Demand and Revenues
- •Demand Curve
- •The Price Elasticity of Demand
- •Brand-Level versus Industry-Level Elasticities
- •Total Revenue and Marginal Revenue Functions
- •Theory of the Firm: Pricing and Output Decisions
- •Perfect Competition
- •Game Theory
- •Games in Matrix Form and the Concept of Nash Equilibrium
- •Game Trees and Subgame Perfection
- •Chapter Summary
- •Questions
- •Endnotes
- •Doing Business in 1840
- •Transportation
- •Communications
- •Finance
- •Production Technology
- •Government
- •Doing Business in 1910
- •Business Conditions in 1910: A “Modern” Infrastructure
- •Production Technology
- •Transportation
- •Communications
- •Finance
- •Government
- •Doing Business Today
- •Modern Infrastructure
- •Transportation
- •Communications
- •Finance
- •Production Technology
- •Government
- •Infrastructure in Emerging Markets
- •Three Different Worlds: Consistent Principles, Changing Conditions, and Adaptive Strategies
- •Chapter Summary
- •Questions
- •Endnotes
- •Definitions
- •Definition of Economies of Scale
- •Definition of Economies of Scope
- •Economies of Scale Due to Spreading of Product-Specific Fixed Costs
- •Economies of Scale Due to Trade-offs among Alternative Technologies
- •“The Division of Labor Is Limited by the Extent of the Market”
- •Special Sources of Economies of Scale and Scope
- •Density
- •Purchasing
- •Advertising
- •Costs of Sending Messages per Potential Consumer
- •Advertising Reach and Umbrella Branding
- •Research and Development
- •Physical Properties of Production
- •Inventories
- •Complementarities and Strategic Fit
- •Sources of Diseconomies of Scale
- •Labor Costs and Firm Size
- •Spreading Specialized Resources Too Thin
- •Bureaucracy
- •Economies of Scale: A Summary
- •The Learning Curve
- •The Concept of the Learning Curve
- •Expanding Output to Obtain a Cost Advantage
- •Learning and Organization
- •The Learning Curve versus Economies of Scale
- •Diversification
- •Why Do Firms Diversify?
- •Efficiency-Based Reasons for Diversification
- •Scope Economies
- •Internal Capital Markets
- •Problematic Justifications for Diversification
- •Diversifying Shareholders’ Portfolios
- •Identifying Undervalued Firms
- •Reasons Not to Diversify
- •Managerial Reasons for Diversification
- •Benefits to Managers from Acquisitions
- •Problems of Corporate Governance
- •The Market for Corporate Control and Recent Changes in Corporate Governance
- •Performance of Diversified Firms
- •Chapter Summary
- •Questions
- •Endnotes
- •Make versus Buy
- •Upstream, Downstream
- •Defining Boundaries
- •Some Make-or-Buy Fallacies
- •Avoiding Peak Prices
- •Tying Up Channels: Vertical Foreclosure
- •Reasons to “Buy”
- •Exploiting Scale and Learning Economies
- •Bureaucracy Effects: Avoiding Agency and Influence Costs
- •Agency Costs
- •Influence Costs
- •Organizational Design
- •Reasons to “Make”
- •The Economic Foundations of Contracts
- •Complete versus Incomplete Contracting
- •Bounded Rationality
- •Difficulties Specifying or Measuring Performance
- •Asymmetric Information
- •The Role of Contract Law
- •Coordination of Production Flows through the Vertical Chain
- •Leakage of Private Information
- •Transactions Costs
- •Relationship-Specific Assets
- •Forms of Asset Specificity
- •The Fundamental Transformation
- •Rents and Quasi-Rents
- •The Holdup Problem
- •Holdup and Ex Post Cooperation
- •The Holdup Problem and Transactions Costs
- •Contract Negotiation and Renegotiation
- •Investments to Improve Ex Post Bargaining Positions
- •Distrust
- •Reduced Investment
- •Recap: From Relationship-Specific Assets to Transactions Costs
- •Chapter Summary
- •Questions
- •Endnotes
- •What Does It Mean to Be “Integrated?”
- •The Property Rights Theory of the Firm
- •Alternative Forms of Organizing Transactions
- •Governance
- •Delegation
- •Recapping PRT
- •Path Dependence
- •Making the Integration Decision
- •Technical Efficiency versus Agency Efficiency
- •The Technical Efficiency/Agency Efficiency Trade-off
- •Real-World Evidence
- •Double Marginalization: A Final Integration Consideration
- •Alternatives to Vertical Integration
- •Tapered Integration: Make and Buy
- •Franchising
- •Strategic Alliances and Joint Ventures
- •Implicit Contracts and Long-Term Relationships
- •Business Groups
- •Keiretsu
- •Chaebol
- •Business Groups in Emerging Markets
- •Chapter Summary
- •Questions
- •Endnotes
- •Competitor Identification and Market Definition
- •The Basics of Competitor Identification
- •Example 5.1 The SSNIP in Action: Defining Hospital Markets
- •Putting Competitor Identification into Practice
- •Empirical Approaches to Competitor Identification
- •Geographic Competitor Identification
- •Measuring Market Structure
- •Market Structure and Competition
- •Perfect Competition
- •Many Sellers
- •Homogeneous Products
- •Excess Capacity
- •Monopoly
- •Monopolistic Competition
- •Demand for Differentiated Goods
- •Entry into Monopolistically Competitive Markets
- •Oligopoly
- •Cournot Quantity Competition
- •The Revenue Destruction Effect
- •Cournot’s Model in Practice
- •Bertrand Price Competition
- •Why Are Cournot and Bertrand Different?
- •Evidence on Market Structure and Performance
- •Price and Concentration
- •Chapter Summary
- •Questions
- •Endnotes
- •6: Entry and Exit
- •Some Facts about Entry and Exit
- •Entry and Exit Decisions: Basic Concepts
- •Barriers to Entry
- •Bain’s Typology of Entry Conditions
- •Analyzing Entry Conditions: The Asymmetry Requirement
- •Structural Entry Barriers
- •Control of Essential Resources
- •Economies of Scale and Scope
- •Marketing Advantages of Incumbency
- •Barriers to Exit
- •Entry-Deterring Strategies
- •Limit Pricing
- •Is Strategic Limit Pricing Rational?
- •Predatory Pricing
- •The Chain-Store Paradox
- •Rescuing Limit Pricing and Predation: The Importance of Uncertainty and Reputation
- •Wars of Attrition
- •Predation and Capacity Expansion
- •Strategic Bundling
- •“Judo Economics”
- •Evidence on Entry-Deterring Behavior
- •Contestable Markets
- •An Entry Deterrence Checklist
- •Entering a New Market
- •Preemptive Entry and Rent Seeking Behavior
- •Chapter Summary
- •Questions
- •Endnotes
- •Microdynamics
- •Strategic Commitment
- •Strategic Substitutes and Strategic Complements
- •The Strategic Effect of Commitments
- •Tough and Soft Commitments
- •A Taxonomy of Commitment Strategies
- •The Informational Benefits of Flexibility
- •Real Options
- •Competitive Discipline
- •Dynamic Pricing Rivalry and Tit-for-Tat Pricing
- •Why Is Tit-for-Tat So Compelling?
- •Coordinating on the Right Price
- •Impediments to Coordination
- •The Misread Problem
- •Lumpiness of Orders
- •Information about the Sales Transaction
- •Volatility of Demand Conditions
- •Facilitating Practices
- •Price Leadership
- •Advance Announcement of Price Changes
- •Most Favored Customer Clauses
- •Uniform Delivered Prices
- •Where Does Market Structure Come From?
- •Sutton’s Endogenous Sunk Costs
- •Innovation and Market Evolution
- •Learning and Industry Dynamics
- •Chapter Summary
- •Questions
- •Endnotes
- •8: Industry Analysis
- •Performing a Five-Forces Analysis
- •Internal Rivalry
- •Entry
- •Substitutes and Complements
- •Supplier Power and Buyer Power
- •Strategies for Coping with the Five Forces
- •Coopetition and the Value Net
- •Applying the Five Forces: Some Industry Analyses
- •Chicago Hospital Markets Then and Now
- •Market Definition
- •Internal Rivalry
- •Entry
- •Substitutes and Complements
- •Supplier Power
- •Buyer Power
- •Commercial Airframe Manufacturing
- •Market Definition
- •Internal Rivalry
- •Barriers to Entry
- •Substitutes and Complements
- •Supplier Power
- •Buyer Power
- •Professional Sports
- •Market Definition
- •Internal Rivalry
- •Entry
- •Substitutes and Complements
- •Supplier Power
- •Buyer Power
- •Conclusion
- •Professional Search Firms
- •Market Definition
- •Internal Rivalry
- •Entry
- •Substitutes and Complements
- •Supplier Power
- •Buyer Power
- •Conclusion
- •Chapter Summary
- •Questions
- •Endnotes
- •Competitive Advantage Defined
- •Maximum Willingness-to-Pay and Consumer Surplus
- •From Maximum Willingness-to-Pay to Consumer Surplus
- •Value-Created
- •Value Creation and “Win–Win” Business Opportunities
- •Value Creation and Competitive Advantage
- •Analyzing Value Creation
- •Value Creation and the Value Chain
- •Value Creation, Resources, and Capabilities
- •Generic Strategies
- •The Strategic Logic of Cost Leadership
- •The Strategic Logic of Benefit Leadership
- •Extracting Profits from Cost and Benefit Advantage
- •Comparing Cost and Benefit Advantages
- •“Stuck in the Middle”
- •Diagnosing Cost and Benefit Drivers
- •Cost Drivers
- •Cost Drivers Related to Firm Size, Scope, and Cumulative Experience
- •Cost Drivers Independent of Firm Size, Scope, or Cumulative Experience
- •Cost Drivers Related to Organization of the Transactions
- •Benefit Drivers
- •Methods for Estimating and Characterizing Costs and Perceived Benefits
- •Estimating Costs
- •Estimating Benefits
- •Strategic Positioning: Broad Coverage versus Focus Strategies
- •Segmenting an Industry
- •Broad Coverage Strategies
- •Focus Strategies
- •Chapter Summary
- •Questions
- •Endnotes
- •The “Shopping Problem”
- •Unraveling
- •Alternatives to Disclosure
- •Nonprofit Firms
- •Report Cards
- •Multitasking: Teaching to the Test
- •What to Measure
- •Risk Adjustment
- •Presenting Report Card Results
- •Gaming Report Cards
- •The Certifier Market
- •Certification Bias
- •Matchmaking
- •When Sellers Search for Buyers
- •Chapter Summary
- •Questions
- •Endnotes
- •Market Structure and Threats to Sustainability
- •Threats to Sustainability in Competitive and Monopolistically Competitive Markets
- •Threats to Sustainability under All Market Structures
- •Evidence: The Persistence of Profitability
- •The Resource-Based Theory of the Firm
- •Imperfect Mobility and Cospecialization
- •Isolating Mechanisms
- •Impediments to Imitation
- •Legal Restrictions
- •Superior Access to Inputs or Customers
- •The Winner’s Curse
- •Market Size and Scale Economies
- •Intangible Barriers to Imitation
- •Causal Ambiguity
- •Dependence on Historical Circumstances
- •Social Complexity
- •Early-Mover Advantages
- •Learning Curve
- •Reputation and Buyer Uncertainty
- •Buyer Switching Costs
- •Network Effects
- •Networks and Standards
- •Competing “For the Market” versus “In the Market”
- •Knocking off a Dominant Standard
- •Early-Mover Disadvantages
- •Imperfect Imitability and Industry Equilibrium
- •Creating Advantage and Creative Destruction
- •Disruptive Technologies
- •The Productivity Effect
- •The Sunk Cost Effect
- •The Replacement Effect
- •The Efficiency Effect
- •Disruption versus the Resource-Based Theory of the Firm
- •Innovation and the Market for Ideas
- •The Environment
- •Factor Conditions
- •Demand Conditions
- •Related Supplier or Support Industries
- •Strategy, Structure, and Rivalry
- •Chapter Summary
- •Questions
- •Endnotes
- •The Principal–Agent Relationship
- •Combating Agency Problems
- •Performance-Based Incentives
- •Problems with Performance-Based Incentives
- •Preferences over Risky Outcomes
- •Risk Sharing
- •Risk and Incentives
- •Selecting Performance Measures: Managing Trade-offs between Costs
- •Do Pay-for-Performance Incentives Work?
- •Implicit Incentive Contracts
- •Subjective Performance Evaluation
- •Promotion Tournaments
- •Efficiency Wages and the Threat of Termination
- •Incentives in Teams
- •Chapter Summary
- •Questions
- •Endnotes
- •13: Strategy and Structure
- •An Introduction to Structure
- •Individuals, Teams, and Hierarchies
- •Complex Hierarchy
- •Departmentalization
- •Coordination and Control
- •Approaches to Coordination
- •Types of Organizational Structures
- •Functional Structure (U-form)
- •Multidivisional Structure (M-form)
- •Matrix Structure
- •Matrix or Division? A Model of Optimal Structure
- •Network Structure
- •Why Are There So Few Structural Types?
- •Structure—Environment Coherence
- •Technology and Task Interdependence
- •Efficient Information Processing
- •Structure Follows Strategy
- •Strategy, Structure, and the Multinational Firm
- •Chapter Summary
- •Questions
- •Endnotes
- •The Social Context of Firm Behavior
- •Internal Context
- •Power
- •The Sources of Power
- •Structural Views of Power
- •Do Successful Organizations Need Powerful Managers?
- •The Decision to Allocate Formal Power to Individuals
- •Culture
- •Culture Complements Formal Controls
- •Culture Facilitates Cooperation and Reduces Bargaining Costs
- •Culture, Inertia, and Performance
- •A Word of Caution about Culture
- •External Context, Institutions, and Strategies
- •Institutions and Regulation
- •Interfirm Resource Dependence Relationships
- •Industry Logics: Beliefs, Values, and Behavioral Norms
- •Chapter Summary
- •Questions
- •Endnotes
- •Glossary
- •Name Index
- •Subject Index

356 • Chapter 10 • Information and Value Creation
total amount invested. When deciding where to place their money, investors naturally prefer analysts who issue accurate forecasts. This gives analysts a strong incentive to do rigorous research. But career concerns—the desire for career advancement—may become paramount when an analyst’s research leads to a contrarian view.
To understand how career concerns can weigh on an analyst’s forecast, consider the dilemma facing a hypothetical analyst named Amit. Suppose that the majority of analysts believe that Google’s stock price is going to increase, but Amit’s diligent research leads to the opposite conclusion. (We will ignore for simplicity the possibility that Google’s share price remains unchanged.) Amit must decide whether to issue a buy or sell order, after which the fate of Google’s stock price will be known to all. Here are the four possible combinations of Amit’s recommendations and stock price movements:
1.Issue a sell recommendation: Google’s price falls.
2.Issue a sell recommendation: Google’s price increases.
3.Issue a buy recommendation: Google’s price falls.
4.Issue a buy recommendation: Google’s price increases.
If Amit issues a sell recommendation and Google’s share price falls, investors will believe that Amit’s forecasts are more accurate than those of other analysts and they will give Amit more money to invest. But if Google’s share increases, investors will question Amit’s accuracy. Suppose instead that Amit ignores his research and follows the herd. Regardless of what happens to Google’s share price, investors will believe that Amit is no more or less accurate than any other. In other words, Amit cannot lose by following the herd. When considering whether to follow convictions and pick against the majority opinion, Amit must assess whether the gains from correctly going against the grain exceed the losses if the majority proves to be correct. Research by Judith Chevalier and Glenn Ellison suggests that young analysts who go out on a limb are punished severely for their mistakes but are only moderately rewarded for their successes.21 More experienced analysts, on the other hand, seem to be forgiven for daring blunders. As a result, young analysts are more conservative and follow the herd, while older analysts are more willing to go where their own research leads them.
Sometimes, certifiers may bias their findings in favor of buyers, particularly when the government requires certification before consumers may purchase a product. Tom Hubbard finds evidence of this in the auto emissions testing market.22 All states require that car owners have their vehicles tested for smog emissions, and vehicle owners in some states have their choice of private testing stations. The testers receive a nice fee to conduct the tests. Hubbard observes that emissions testing is somewhat subjective, providing an opportunity for testers to bias their findings. Although car owners may prefer cleaner air, they also prefer not spending thousands of dollars on repairs to make sure that their cars are in compliance with emissions standards. Hubbard finds evidence that car owners seek out testing stations that issue favorable results, and, as a result, testing stations issue excessively favorable results as a way to lure customers.
MATCHMAKING
The certifier’s role in vertically differentiated markets is fairly simple: establish and quantify the quality differences across products. Certification in horizontally differentiated markets is rather different. In these markets, consumers may disagree about product rankings, and a certifier who declares that a given seller is the best will be giving bad advice to many customers. For example, if you ask a Chicagoan to name the best restaurant, he or she might mention Alinea or L2O, both of which are in
Matchmaking • 357
Chicago and were awarded three Michelin stars. Ask the same question to someone in Copenhagen and he or she will probably brag that Noma was named the best restaurant in the world. As with beauty, the best restaurant is in the eye of the beholder.
There are many sources of horizontal differentiation, such as location. Diners will disagree about their favorite restaurants for many other reasons, based on preferences for different cuisines and ambience and how they feel about service. Such disagreements arise for virtually all horizontally differentiated goods and services, including entertainment, clothing, and automobiles. As long as consumers are not too strongly differentiated, vertical certification (i.e., “this seller is best”) plays an important role because the certifier’s favorite seller is likely to be among everybody’s favorites. But certifiers can do more than offer a vertical ranking. With the right information, certifiers can match consumers to the horizontally differentiated firms that best meet their idiosyncratic needs. By using unprecedented amounts of information about consumer habits to refine this matching process, firms such as Google and Facebook have revolutionized how products are made and marketed.
Recall that when shopping for experience goods, consumers often rely on friends and family. This can be especially helpful for horizontally differentiated goods because consumers usually know something about their friends’ tastes. This may explain why teenage children do not ask their parents for movie recommendations! Third-party certifiers of horizontally differentiated goods can fulfill the same role as trusted friends, by tailoring recommendations to the specific tastes of individual consumers. For example, consumers might read reviews by several movie and restaurant critics and gravitate toward those who share similar tastes.
When newspapers and magazines provided the bulk of movie and restaurant reviews, most individuals necessarily had access to only a few reviewers. This made it hard to find reviewers with the same tastes. This problem was not confined to artistic endeavors. Consumers might find it just as difficult to find someone with similar tastes for clothing or child care. And the shopping problem could be very difficult for medical patients needing specialized care because few if any friends and family have similar medical problems.23
The Internet is changing all this by making it possible for hundreds of millions of individuals to become certifiers, thereby allowing consumers to find a certifier with similar idiosyncratic preferences. For example, someone who enjoyed the Lord of the Rings movies can go to Amazon where he will find lists of movie recommendations by other fans of the fantasy trilogy. By allowing consumers to discuss and debate where to shop for personal services, clothing, food, and nearly everything else, the Internet is like the nineteenth-century town square on steroids. Chicago foodies no longer have to ask their neighbor if they have tried the new fusion restaurant; they can read dozens of reviews at opentable.com and decide which contributors to trust. Even better, their friends can provide instantaneous certification by tweeting reviews at Twitter.
When any Internet user can be a certifier, opportunities for biased certification multiply. Motivation bias and survivor bias remain problems. Market researchers compile lists of influential tweeters and offer them payments to try to influence their tweets. And authors sometimes conspire to increase downloads of their books on eReaders like Amazon’s Kindle through “review swapping”—raving about each other’s works. Netflix has taken the review-matching process one step further, as described in Example 10.6.
When Sellers Search for Buyers
We normally think of certification as a way to help buyers find sellers. But certification can also help sellers find buyers. In vertically differentiated goods markets, sellers include strong certification results in their marketing campaigns. For example, Jaguar
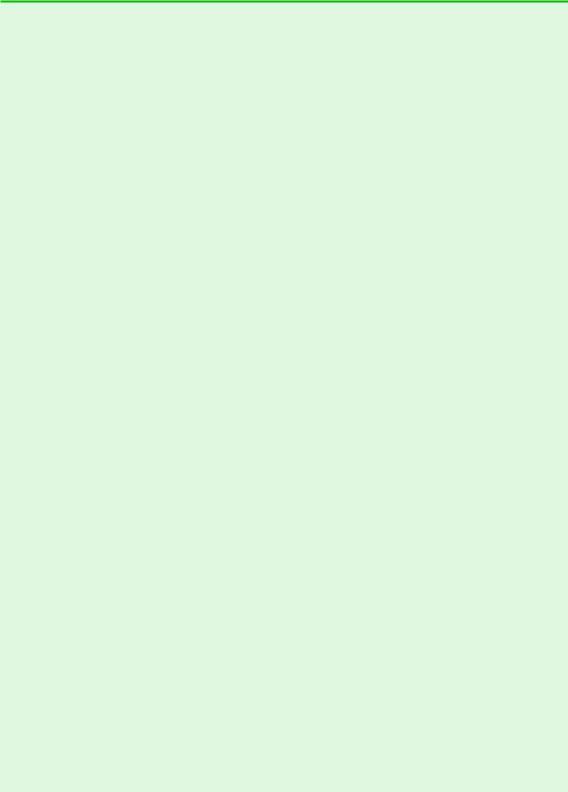
358 • Chapter 10 • Information and Value Creation
EXAMPLE 10.6 THE NETFLIX CHALLENGE
Fearful of a format war like the one between VHS and BetaMax home video systems, leading hardware makers joined Time Warner in 2005 to form the DVD Consortium. In March 1997, the DVD was born, and later that year, Marc Randolph and Reed Hastings founded Netflix, the first DVD-by-mail rental service. In April 1998, the Netflix web site went online, offering DVDs at $4 a rental (plus $2 postage). But the DVD format was still in its infancy, and Netflix was unknown outside of the community of early DVD adopters. At that time, Blockbuster Video was the 800-pound gorilla in movie rentals, and it relied on its vast store network, combined with purchasing and inventory economies, to maintain its dominance. Blockbuster did not view Netflix as a threat and was taking its time mulling over how to respond to the new disc format.
By 1999, the DVD had established itself as the most successful new consumer electronics technology in history, and Netflix’s DVD library was second to none. In September 1999, Netflix launched a subscription plan, whereby renters could specify how many discs they would keep at any given time. When users returned a disc, Netflix would mail out the next one in the queue. By this time Blockbuster was stocking DVDs in most of its stores but was still uninterested in a DVD-by-mail model. This would prove to be a strategic blunder because the economics of DVDs all but wiped out Blockbuster’s scale economies. Movie studios had set prices for DVDs far below those for video tapes, minimizing the importance of purchasing economies. And Netflix’s regional warehousing provided lower inventory costs than could be achieved by Blockbuster’s bricks and mortar stores.
Netflix did more than match Blockbuster’s costs; it offered a service that Blockbuster could not match. Taking a lead from Amazon, Netflix provided users with movie ratings, based on input from other users. But Netflix went one step further. Using proprietary software called Cinematch, Netflix provided individualized ratings. Here is a gross simplification of how
Cinematch worked. Suppose that Mark Shanley wanted to rent The Dark Knight, which he had never seen. Cinematch has already asked Shanley to rate other movies, and he has given five stars (the maximum) to Blade Runner and one star (the minimum) to Transformers. Cinematch scoured the ratings by other users, searching for users who have seen all three films. Cinematch might have found that the typical user who gave five stars to Blade Runner and one star to Transformers gave an average of 4.5 stars to The Dark Knight. Shanley’s predicted rating for The Dark Knight is therefore 4.5 stars.
The Cinematch algorithm is, of course, much more complicated than this, and Netflix recommends that users rate hundreds of movies for its algorithm to give reliable predictions. Even when users have rated over a thousand movies, the Cinematch algorithm is far from perfect. Netflix used the statistic called the root-mean-square error (RMSE) to evaluate Cinematch. The RMSE is computed in steps:
•Compute the difference between the prediction and actual rating for each user and film.
•Square this difference and sum up the squares.
•Take the square root of the sum. Lower scores imply more accurate ratings.
Cinematch produced an RMSE of 0.9514, which implies that the typical prediction was off by a little less than one point. Netflix wanted to do better, and in 2006 it announced that it would award a $1 million prize to anyone who could improve upon the Cinematch RMSE by at least 10 percent. Within a week, a team had produced an algorithm that slightly outperformed Cinematch. But beating Cinematch by 10 percent proved to be a challenge. Finally, in July 2009, BellKor’s Pragmatic Chaos and The Ensemble achieved virtually identical RMSE scores of 0.855 within a few days of each other. By preestablished rules, Netflix provided a second test and BellKor barely won. Netflix awarded BellKor the $1 million prize.
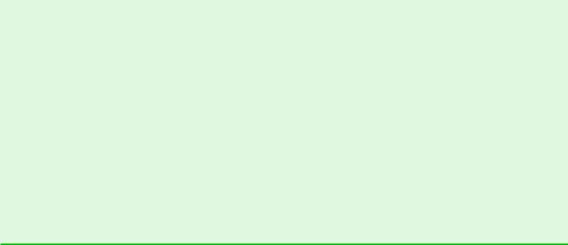
Matchmaking • 359
Netflix got a lot of publicity for this competition, but to Netflix this was a lot more than a game. The recommendation algorithm is serious business. At any time during the past decade, Blockbuster could have entered the DVD-by-mail business. Amazon could also have joined the fray. Netflix’s inventory economies would have provided some protection. But streaming has rapidly overtaken DVD- by-mail. To sustain its advantage, Netflix has to offer something to consumers besides its large inventory of DVD discs. That something is personalized movie ratings. Users know that Netflix’s film recommendations are
very reliable. This reliability depends on three things:
1.Users who have to rate hundreds of films
2.Lots of other users who give similar ratings
3.An algorithm that generates accurate predictions
Netflix has all three; no one else does. Moreover, many Netflix users have been with the company for years, entering film ratings after every rental. These loyal users have high switching costs. Netflix is relying on this loyalty as it fends off video streaming services from Amazon, Apple, and others.
launched an expensive advertising campaign in 2009 after JD Powers ranked it first in reliability, helping it to overcome a bad reputation that had emerged, in part, from poor reliability ratings in Consumer Reports. It can be much more difficult for sellers of horizontally differentiated goods to reach out to buyers because this requires knowledge of individual customer tastes. Television facilitated some matching by allowing firms to place ads that reach a target market. For example, Chevrolet advertises pickup trucks during football games, while Mercedes promotes golf tournaments and Smuckers Jams sponsors figure skating. Cable television further subdivides the market and allows even more targeted marketing.
It goes without saying that horizontally differentiated sellers would like to know as much as possible about the spending habits of potential customers so as to most efficiently place their advertisements. While television and cable may allow for some targeting; the Internet goes one step further. At web sites such as Google and Facebook, every user click is compiled and catalogued. A user who spends time learning about hotels in Florence may soon receive pop-up ads, banner ads, and floating ads from Tuscan goldsmiths. (We know this because one of the textbook authors is still besieged by these ads!) Users who spend time at ikea.com may get ads from other furniture makers, electronics retailers, even real estate brokers. Moreover, advertisers only pay when users click through the message, forcing these web sites to constantly improve their ability to match buyers and sellers.
The revenue model for Google, Facebook, Craig’s List (an online classified ad site), and countless other web sites that do not sell their own goods and services is similar to the revenue model for traditional television and newspapers. These firms make money by allowing sellers to get the attention of potential customers. Thanks to the Internet, sellers can more easily target their messages than ever before. This means not only that consumers are being bombarded with more sales messages than ever before, but also that these messages are more relevant to their idiosyncratic tastes.
As this business model evolves, the shopping problem may return to its roots. Before there was widespread branding and third-party certification, consumers relied on friends and family—the town square—to help evaluate experience goods. Consumers who are steered to sellers by Google or Craig’s List will continue to value information about that seller’s quality. Only now they will obtain that information from virtual town squares. But technology has its limits. Customer satisfaction