
- •Table of Contents
- •What’s New in EViews 5.0
- •What’s New in 5.0
- •Compatibility Notes
- •EViews 5.1 Update Overview
- •Overview of EViews 5.1 New Features
- •Preface
- •Part I. EViews Fundamentals
- •Chapter 1. Introduction
- •What is EViews?
- •Installing and Running EViews
- •Windows Basics
- •The EViews Window
- •Closing EViews
- •Where to Go For Help
- •Chapter 2. A Demonstration
- •Getting Data into EViews
- •Examining the Data
- •Estimating a Regression Model
- •Specification and Hypothesis Tests
- •Modifying the Equation
- •Forecasting from an Estimated Equation
- •Additional Testing
- •Chapter 3. Workfile Basics
- •What is a Workfile?
- •Creating a Workfile
- •The Workfile Window
- •Saving a Workfile
- •Loading a Workfile
- •Multi-page Workfiles
- •Addendum: File Dialog Features
- •Chapter 4. Object Basics
- •What is an Object?
- •Basic Object Operations
- •The Object Window
- •Working with Objects
- •Chapter 5. Basic Data Handling
- •Data Objects
- •Samples
- •Sample Objects
- •Importing Data
- •Exporting Data
- •Frequency Conversion
- •Importing ASCII Text Files
- •Chapter 6. Working with Data
- •Numeric Expressions
- •Series
- •Auto-series
- •Groups
- •Scalars
- •Chapter 7. Working with Data (Advanced)
- •Auto-Updating Series
- •Alpha Series
- •Date Series
- •Value Maps
- •Chapter 8. Series Links
- •Basic Link Concepts
- •Creating a Link
- •Working with Links
- •Chapter 9. Advanced Workfiles
- •Structuring a Workfile
- •Resizing a Workfile
- •Appending to a Workfile
- •Contracting a Workfile
- •Copying from a Workfile
- •Reshaping a Workfile
- •Sorting a Workfile
- •Exporting from a Workfile
- •Chapter 10. EViews Databases
- •Database Overview
- •Database Basics
- •Working with Objects in Databases
- •Database Auto-Series
- •The Database Registry
- •Querying the Database
- •Object Aliases and Illegal Names
- •Maintaining the Database
- •Foreign Format Databases
- •Working with DRIPro Links
- •Part II. Basic Data Analysis
- •Chapter 11. Series
- •Series Views Overview
- •Spreadsheet and Graph Views
- •Descriptive Statistics
- •Tests for Descriptive Stats
- •Distribution Graphs
- •One-Way Tabulation
- •Correlogram
- •Unit Root Test
- •BDS Test
- •Properties
- •Label
- •Series Procs Overview
- •Generate by Equation
- •Resample
- •Seasonal Adjustment
- •Exponential Smoothing
- •Hodrick-Prescott Filter
- •Frequency (Band-Pass) Filter
- •Chapter 12. Groups
- •Group Views Overview
- •Group Members
- •Spreadsheet
- •Dated Data Table
- •Graphs
- •Multiple Graphs
- •Descriptive Statistics
- •Tests of Equality
- •N-Way Tabulation
- •Principal Components
- •Correlations, Covariances, and Correlograms
- •Cross Correlations and Correlograms
- •Cointegration Test
- •Unit Root Test
- •Granger Causality
- •Label
- •Group Procedures Overview
- •Chapter 13. Statistical Graphs from Series and Groups
- •Distribution Graphs of Series
- •Scatter Diagrams with Fit Lines
- •Boxplots
- •Chapter 14. Graphs, Tables, and Text Objects
- •Creating Graphs
- •Modifying Graphs
- •Multiple Graphs
- •Printing Graphs
- •Copying Graphs to the Clipboard
- •Saving Graphs to a File
- •Graph Commands
- •Creating Tables
- •Table Basics
- •Basic Table Customization
- •Customizing Table Cells
- •Copying Tables to the Clipboard
- •Saving Tables to a File
- •Table Commands
- •Text Objects
- •Part III. Basic Single Equation Analysis
- •Chapter 15. Basic Regression
- •Equation Objects
- •Specifying an Equation in EViews
- •Estimating an Equation in EViews
- •Equation Output
- •Working with Equations
- •Estimation Problems
- •Chapter 16. Additional Regression Methods
- •Special Equation Terms
- •Weighted Least Squares
- •Heteroskedasticity and Autocorrelation Consistent Covariances
- •Two-stage Least Squares
- •Nonlinear Least Squares
- •Generalized Method of Moments (GMM)
- •Chapter 17. Time Series Regression
- •Serial Correlation Theory
- •Testing for Serial Correlation
- •Estimating AR Models
- •ARIMA Theory
- •Estimating ARIMA Models
- •ARMA Equation Diagnostics
- •Nonstationary Time Series
- •Unit Root Tests
- •Panel Unit Root Tests
- •Chapter 18. Forecasting from an Equation
- •Forecasting from Equations in EViews
- •An Illustration
- •Forecast Basics
- •Forecasting with ARMA Errors
- •Forecasting from Equations with Expressions
- •Forecasting with Expression and PDL Specifications
- •Chapter 19. Specification and Diagnostic Tests
- •Background
- •Coefficient Tests
- •Residual Tests
- •Specification and Stability Tests
- •Applications
- •Part IV. Advanced Single Equation Analysis
- •Chapter 20. ARCH and GARCH Estimation
- •Basic ARCH Specifications
- •Estimating ARCH Models in EViews
- •Working with ARCH Models
- •Additional ARCH Models
- •Examples
- •Binary Dependent Variable Models
- •Estimating Binary Models in EViews
- •Procedures for Binary Equations
- •Ordered Dependent Variable Models
- •Estimating Ordered Models in EViews
- •Views of Ordered Equations
- •Procedures for Ordered Equations
- •Censored Regression Models
- •Estimating Censored Models in EViews
- •Procedures for Censored Equations
- •Truncated Regression Models
- •Procedures for Truncated Equations
- •Count Models
- •Views of Count Models
- •Procedures for Count Models
- •Demonstrations
- •Technical Notes
- •Chapter 22. The Log Likelihood (LogL) Object
- •Overview
- •Specification
- •Estimation
- •LogL Views
- •LogL Procs
- •Troubleshooting
- •Limitations
- •Examples
- •Part V. Multiple Equation Analysis
- •Chapter 23. System Estimation
- •Background
- •System Estimation Methods
- •How to Create and Specify a System
- •Working With Systems
- •Technical Discussion
- •Vector Autoregressions (VARs)
- •Estimating a VAR in EViews
- •VAR Estimation Output
- •Views and Procs of a VAR
- •Structural (Identified) VARs
- •Cointegration Test
- •Vector Error Correction (VEC) Models
- •A Note on Version Compatibility
- •Chapter 25. State Space Models and the Kalman Filter
- •Background
- •Specifying a State Space Model in EViews
- •Working with the State Space
- •Converting from Version 3 Sspace
- •Technical Discussion
- •Chapter 26. Models
- •Overview
- •An Example Model
- •Building a Model
- •Working with the Model Structure
- •Specifying Scenarios
- •Using Add Factors
- •Solving the Model
- •Working with the Model Data
- •Part VI. Panel and Pooled Data
- •Chapter 27. Pooled Time Series, Cross-Section Data
- •The Pool Workfile
- •The Pool Object
- •Pooled Data
- •Setting up a Pool Workfile
- •Working with Pooled Data
- •Pooled Estimation
- •Chapter 28. Working with Panel Data
- •Structuring a Panel Workfile
- •Panel Workfile Display
- •Panel Workfile Information
- •Working with Panel Data
- •Basic Panel Analysis
- •Chapter 29. Panel Estimation
- •Estimating a Panel Equation
- •Panel Estimation Examples
- •Panel Equation Testing
- •Estimation Background
- •Appendix A. Global Options
- •The Options Menu
- •Print Setup
- •Appendix B. Wildcards
- •Wildcard Expressions
- •Using Wildcard Expressions
- •Source and Destination Patterns
- •Resolving Ambiguities
- •Wildcard versus Pool Identifier
- •Appendix C. Estimation and Solution Options
- •Setting Estimation Options
- •Optimization Algorithms
- •Nonlinear Equation Solution Methods
- •Appendix D. Gradients and Derivatives
- •Gradients
- •Derivatives
- •Appendix E. Information Criteria
- •Definitions
- •Using Information Criteria as a Guide to Model Selection
- •References
- •Index
- •Symbols
- •.DB? files 266
- •.EDB file 262
- •.RTF file 437
- •.WF1 file 62
- •@obsnum
- •Panel
- •@unmaptxt 174
- •~, in backup file name 62, 939
- •Numerics
- •3sls (three-stage least squares) 697, 716
- •Abort key 21
- •ARIMA models 501
- •ASCII
- •file export 115
- •ASCII file
- •See also Unit root tests.
- •Auto-search
- •Auto-series
- •in groups 144
- •Auto-updating series
- •and databases 152
- •Backcast
- •Berndt-Hall-Hall-Hausman (BHHH). See Optimization algorithms.
- •Bias proportion 554
- •fitted index 634
- •Binning option
- •classifications 313, 382
- •Boxplots 409
- •By-group statistics 312, 886, 893
- •coef vector 444
- •Causality
- •Granger's test 389
- •scale factor 649
- •Census X11
- •Census X12 337
- •Chi-square
- •Cholesky factor
- •Classification table
- •Close
- •Coef (coefficient vector)
- •default 444
- •Coefficient
- •Comparison operators
- •Conditional standard deviation
- •graph 610
- •Confidence interval
- •Constant
- •Copy
- •data cut-and-paste 107
- •table to clipboard 437
- •Covariance matrix
- •HAC (Newey-West) 473
- •heteroskedasticity consistent of estimated coefficients 472
- •Create
- •Cross-equation
- •Tukey option 393
- •CUSUM
- •sum of recursive residuals test 589
- •sum of recursive squared residuals test 590
- •Data
- •Database
- •link options 303
- •using auto-updating series with 152
- •Dates
- •Default
- •database 24, 266
- •set directory 71
- •Dependent variable
- •Description
- •Descriptive statistics
- •by group 312
- •group 379
- •individual samples (group) 379
- •Display format
- •Display name
- •Distribution
- •Dummy variables
- •for regression 452
- •lagged dependent variable 495
- •Dynamic forecasting 556
- •Edit
- •See also Unit root tests.
- •Equation
- •create 443
- •store 458
- •Estimation
- •EViews
- •Excel file
- •Excel files
- •Expectation-prediction table
- •Expected dependent variable
- •double 352
- •Export data 114
- •Extreme value
- •binary model 624
- •Fetch
- •File
- •save table to 438
- •Files
- •Fitted index
- •Fitted values
- •Font options
- •Fonts
- •Forecast
- •evaluation 553
- •Foreign data
- •Formula
- •forecast 561
- •Freq
- •DRI database 303
- •F-test
- •for variance equality 321
- •Full information maximum likelihood 698
- •GARCH 601
- •ARCH-M model 603
- •variance factor 668
- •system 716
- •Goodness-of-fit
- •Gradients 963
- •Graph
- •remove elements 423
- •Groups
- •display format 94
- •Groupwise heteroskedasticity 380
- •Help
- •Heteroskedasticity and autocorrelation consistent covariance (HAC) 473
- •History
- •Holt-Winters
- •Hypothesis tests
- •F-test 321
- •Identification
- •Identity
- •Import
- •Import data
- •See also VAR.
- •Index
- •Insert
- •Instruments 474
- •Iteration
- •Iteration option 953
- •in nonlinear least squares 483
- •J-statistic 491
- •J-test 596
- •Kernel
- •bivariate fit 405
- •choice in HAC weighting 704, 718
- •Kernel function
- •Keyboard
- •Kwiatkowski, Phillips, Schmidt, and Shin test 525
- •Label 82
- •Last_update
- •Last_write
- •Latent variable
- •Lead
- •make covariance matrix 643
- •List
- •LM test
- •ARCH 582
- •for binary models 622
- •LOWESS. See also LOESS
- •in ARIMA models 501
- •Mean absolute error 553
- •Metafile
- •Micro TSP
- •recoding 137
- •Models
- •add factors 777, 802
- •solving 804
- •Mouse 18
- •Multicollinearity 460
- •Name
- •Newey-West
- •Nonlinear coefficient restriction
- •Wald test 575
- •weighted two stage 486
- •Normal distribution
- •Numbers
- •chi-square tests 383
- •Object 73
- •Open
- •Option setting
- •Option settings
- •Or operator 98, 133
- •Ordinary residual
- •Panel
- •irregular 214
- •unit root tests 530
- •Paste 83
- •PcGive data 293
- •Polynomial distributed lag
- •Pool
- •Pool (object)
- •PostScript
- •Prediction table
- •Principal components 385
- •Program
- •p-value 569
- •for coefficient t-statistic 450
- •Quiet mode 939
- •RATS data
- •Read 832
- •CUSUM 589
- •Regression
- •Relational operators
- •Remarks
- •database 287
- •Residuals
- •Resize
- •Results
- •RichText Format
- •Robust standard errors
- •Robustness iterations
- •for regression 451
- •with AR specification 500
- •workfile 95
- •Save
- •Seasonal
- •Seasonal graphs 310
- •Select
- •single item 20
- •Serial correlation
- •theory 493
- •Series
- •Smoothing
- •Solve
- •Source
- •Specification test
- •Spreadsheet
- •Standard error
- •Standard error
- •binary models 634
- •Start
- •Starting values
- •Summary statistics
- •for regression variables 451
- •System
- •Table 429
- •font 434
- •Tabulation
- •Template 424
- •Tests. See also Hypothesis tests, Specification test and Goodness of fit.
- •Text file
- •open as workfile 54
- •Type
- •field in database query 282
- •Units
- •Update
- •Valmap
- •find label for value 173
- •find numeric value for label 174
- •Value maps 163
- •estimating 749
- •View
- •Wald test 572
- •nonlinear restriction 575
- •Watson test 323
- •Weighting matrix
- •heteroskedasticity and autocorrelation consistent (HAC) 718
- •kernel options 718
- •White
- •Window
- •Workfile
- •storage defaults 940
- •Write 844
- •XY line
- •Yates' continuity correction 321

Procedures for Binary Equations—633
Dependent Variable: GRADE |
|
|
|
|
|
|||
Method: ML - Binary Probit |
|
|
|
|
|
|||
Date: 07/31/00 |
Time: 15:57 |
|
|
|
|
|
||
Sample: 1 32 |
|
|
|
|
|
|
|
|
Included observations: 32 |
|
|
|
|
|
|
||
Andrews and Hosmer-Lemeshow Goodness-of-Fit Tests |
|
|
|
|||||
Grouping based upon predicted risk (randomize ties) |
|
|
|
|||||
|
|
|
|
|
|
|
|
|
|
Quantile of Risk |
|
Dep=0 |
|
Dep=1 |
Total |
H-L |
|
|
Low |
High |
Actual |
Expect |
Actual |
Expect |
Obs |
Value |
|
|
|
|
|
|
|
|
|
1 |
0.0161 |
0.0185 |
3 |
2.94722 |
0 |
0.05278 |
3 |
0.05372 |
2 |
0.0186 |
0.0272 |
3 |
2.93223 |
0 |
0.06777 |
3 |
0.06934 |
3 |
0.0309 |
0.0457 |
3 |
2.87888 |
0 |
0.12112 |
3 |
0.12621 |
4 |
0.0531 |
0.1088 |
3 |
2.77618 |
0 |
0.22382 |
3 |
0.24186 |
5 |
0.1235 |
0.1952 |
2 |
3.29779 |
2 |
0.70221 |
4 |
2.90924 |
6 |
0.2732 |
0.3287 |
3 |
2.07481 |
0 |
0.92519 |
3 |
1.33775 |
7 |
0.3563 |
0.5400 |
2 |
1.61497 |
1 |
1.38503 |
3 |
0.19883 |
8 |
0.5546 |
0.6424 |
1 |
1.20962 |
2 |
1.79038 |
3 |
0.06087 |
9 |
0.6572 |
0.8342 |
0 |
0.84550 |
3 |
2.15450 |
3 |
1.17730 |
10 |
0.8400 |
0.9522 |
1 |
0.45575 |
3 |
3.54425 |
4 |
0.73351 |
|
|
|
|
|
|
|
|
|
|
|
Total |
21 |
21.0330 |
11 |
10.9670 |
32 |
6.90863 |
|
|
|
|
|
|
|
|
|
H-L Statistic: |
|
6.9086 |
|
|
Prob. Chi-Sq(8) |
|
0.5465 |
|
Andrews Statistic: |
20.6045 |
|
|
Prob. Chi-Sq(10) |
0.0240 |
|||
|
|
|
|
|
|
|
|
|
The columns labeled “Quantiles of Risk” depict the high and low value of the predicted probability for each decile. Also depicted are the actual and expected number of observations in each group, as well as the contribution of each group to the overall Hosmer-Leme- show (H-L) statistic—large values indicate large differences between the actual and predicted values for that decile.
The χ2 statistics are reported at the bottom of the table. Since grouping on the basis of the fitted values falls within the structure of an Andrews test, we report results for both the H- L and the Andrews test statistic. The p-value for the HL test is large while the value for the Andrews test statistic is small, providing mixed evidence of problems. Furthermore, the relatively small sample sizes suggest that caution is in order in interpreting the results.
Procedures for Binary Equations
In addition to the usual procedures for equations, EViews allows you to forecast the dependent variable and linear index, or to compute a variety of residuals associated with the binary model.
Forecast
EViews allows you to compute either the fitted probability, ˆ i p
ted values of the index xi′ β . From the equation toolbar select ability/Index)…, and then click on the desired entry.
= |
ˆ |
1 − F( −xi′β) , or the fit- |
Proc/Forecast (Fitted Prob-
As with other estimators, you can select a forecast sample, and display a graph of the forecast. If your explanatory variables, xt , include lagged values of the binary dependent vari-

634—Chapter 21. Discrete and Limited Dependent Variable Models
able yt , forecasting with the Dynamic option instructs EViews to use the fitted values , to derive the forecasts, in contrast with the Static option, which uses the actual
(lagged) yt − 1 .
Neither forecast evaluations nor automatic calculation of standard errors of the forecast are currently available for this estimation method. The latter can be computed using the variance matrix of the coefficients displayed by View/Covariance Matrix, or using the
@covariance function.
You can use the fitted index in a variety of ways, for example, to compute the marginal effects of the explanatory variables. Simply forecast the fitted index and save the results in a series, say XB. Then the auto-series @dnorm(-xb), @dlogistic(-xb), or @dex- treme(-xb) may be multiplied by the coefficients of interest to provide an estimate of the derivatives of the expected value of yi with respect to the j-th variable in xi :
∂E( yi |
xi, β) |
|
------------------------------ = f( −xi′β) βj . |
(21.14) |
|
∂xij |
|
Make Residual Series
Proc/Make Residual Series gives you the option of generating one of the following three types of residuals:
|
|
|
Ordinary |
eoi |
|
ˆ |
|
|
|
|
|
|
|
= yi − pi |
|
|
|
||
|
|
|
Standardized |
|
|
ˆ |
|
|
|
|
|
|
|
esi |
= |
y i − pi |
|
|
|
|
|
|
|
-- -------------------------ˆ |
ˆ |
|
|
||
|
|
|
|
|
|
p i ( 1 − pi) |
|
|
|
|
|
|
Generalized |
|
|
ˆ |
ˆ |
|
|
|
|
|
|
egi |
= |
( y i − p i ) f( −xi′β) |
φ |
|
|
|
|
|
|
------------------------------------------ˆ |
ˆ |
|
|||
|
|
|
|
|
|
p i ( 1 |
− pi) |
|
|
ˆ |
= |
|
ˆ |
|
|
|
|
|
|
where pi |
1 − F( − xi′β) is the fitted probability, and the distribution and density func- |
tions F and f , depend on the specified distribution.
The ordinary residuals have been described above. The standardized residuals are simply the ordinary residuals divided by an estimate of the theoretical standard deviation. The generalized residuals are derived from the first order conditions that define the ML estimates. The first order conditions may be regarded as an orthogonality condition between the generalized residuals and the regressors x .
∂l( β) |
= |
N ( yi − ( 1 − F( −xi′β) ) ) f( − xi′β) |
N |
|
-------------∂β |
Σ |
---------------------------------------------------------------------------F ( − x i ′ β ) ( 1 − F ( − x i ′ β ) ) |
xi = Σ eg, i xi . (21.15) |
|
|
i = 1 |
i = 1 |

Procedures for Binary Equations—635
This property is analogous to the orthogonality condition between the (ordinary) residuals and the regressors in linear regression models.
The usefulness of the generalized residuals derives from the fact that you can easily obtain the score vectors by multiplying the generalized residuals by each of the regressors in x . These scores can be used in a variety of LM specification tests (see Chesher, Lancaster and Irish (1985), and Gourieroux, Monfort, Renault, and Trognon (1987)). We provide an example below.
Demonstrations
You can easily use the results of a binary model in additional analysis. Here, we provide demonstrations of using EViews to plot a probability response curve and to test for heteroskedasticity in the residuals.
Plotting Probability Response Curves
You can use the estimated coefficients from a binary model to examine how the predicted probabilities vary with an independent variable. To do so, we will use the EViews built-in modeling features.
For the probit example above, suppose we are interested in the effect of teaching method (PSI) on educational improvement (GRADE). We wish to plot the fitted probabilities of GRADE improvement as a function of GPA for the two values of PSI, fixing the values of other variables at their sample means.
We will perform the analysis using a grid of values for GPA from 2 to 4. First, we will create a series containing the values of GPA for which we wish to examine the fitted probabilities for GRADE. The easiest way to do this is to use the @trend function to generate a new series:
series gpa_plot=2+(4-2)*@trend/(@obs(@trend)-1)
@trend creates a series that begins at 0 in the first observation of the sample, and increases by 1 for each subsequent observation, up through @obs-1.
Next, we will use a model object to define and perform the desired computations. The following discussion skims over many of the useful features of EViews models. Those wishing greater detail should consult Chapter 26, “Models”, beginning on page 777.
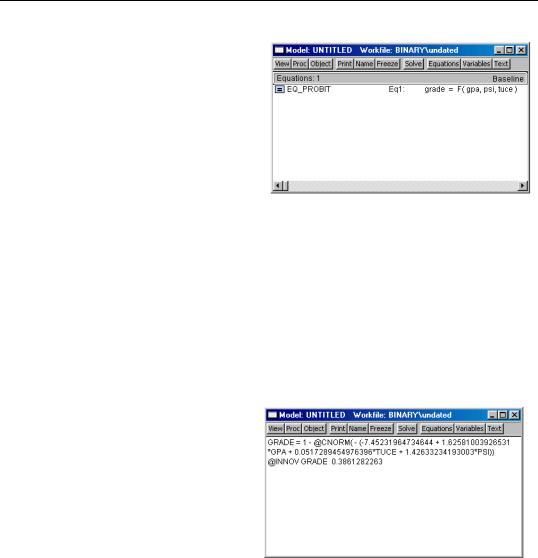
636—Chapter 21. Discrete and Limited Dependent Variable Models
First, we create a model out of the estimated equation by selecting Proc/ Make Model from the equation toolbar. EViews will create an untitled model object containing a link to the estimated equation and will open the model window.
Next we want to edit this model specification so that calculations are performed using our simulation values. To
do so we must first break the link between the original equation and the model specification by selecting Proc/Links/Break All Links. Next, click on the Text button or select View/Source Text to display the text editing screen.
We wish to create two separate equations: one with the value of PSI set to 0 and one with the value of PSI set to 1 (you can, of course, use copy-and-paste to aid in creating the additional equation). We will also edit the specification so that references to GPA are replaced with the series of simulation values GPA_PLOT, and references to TUCE are replaced with the calculated mean, “@MEAN(TUCE)”. The GRADE_0 equation sets PSI to 0, while the GRADE_1 contains an additional expression, 1.426332342, which is the coefficient on the PSI variable.
Once you have edited your model, click on Solve and set the “Active” solution scenario to “Actuals”. This tells EViews that you wish to place the solutions in the series “GRADE_0” and “GRADE_1” as specified in the equation definitions. You can safely ignore the remaining solution settings and simply click on OK. EViews will report that your model has solved successfully.
You are now ready to plot results. Select Object/New Object.../Group, and enter:
gpa_plot grade_0 grade_1
EViews will open an untitled group window containing these two series. Select View/ Graph/XY line to display the probability of GRADE improvement plotted against GPA for those with and without PSI (and with the TUCE evaluated at means).

Procedures for Binary Equations—637
EViews will open a group window containing these series. Select View/Graph/XY line from the group toolbar to display a graph of your results.
We have annotated the graph slightly so that you can better judge the effect of the new teaching methods (PSI) on the GPA—Grade Improvement relationship.
Testing for Heteroskedasticity
As an example of specification tests |
|
for binary dependent variable mod- |
|
els, we carry out the LM test for het- |
|
eroskedasticity using the artificial |
|
regression method described by |
|
Davidson and MacKinnon (1993, |
|
section 15.4). We test the null |
|
hypothesis of homoskedasticity |
|
against the alternative of heteroskedasticity of the form: |
|
var( ui) = exp ( 2zi′γ) , |
(21.16) |
where γ is an unknown parameter. In this example, we take PSI as the only variable in z . The test statistic is the explained sum of squares from the regression:
|
|
ˆ |
|
|
ˆ |
′ |
|
ˆ |
|
ˆ |
|
|
|
( y i − p i ) |
= |
f ( − xi′ β) |
+ |
f( −xi′β) ( −xi |
′β) |
′b |
2 + vi , |
(21.17) |
|||||
-- ------------------------- ˆ |
( 1 |
ˆ |
-- -------------------------ˆ |
ˆ |
xib1 |
------------------------------------ˆ |
ˆ |
----- zi |
|||||
p i |
− pi) |
|
p i ( 1 |
− pi) |
|
|
pi( 1 |
− pi) |
|
|
|
which is asymptotically distributed as a χ2 with degrees of freedom equal to the number of variables in z (in this case 1).
ˆ |
and fitted index xi′β . |
To carry out the test, we first retrieve the fitted probabilities pi |
Click on the Forecast button and first save the fitted probabilities as P_HAT and then the index as XB (you will have to click Forecast twice to save the two series).
Next, the dependent variable in the test regression may be obtained as the standardized residual. Select Proc/Make Residual Series… and select Standardized Residual. We will save the series as BRMR_Y.
Lastly, we will use the built-in EViews functions for evaluating the normal density and cumulative distribution function to create a group object containing the independent variables:
series fac=@dnorm(-xb)/@sqrt(p_hat*(1-p_hat))
group brmr_x fac (gpa*fac) (tuce*fac) (psi*fac)