
- •Table of Contents
- •What’s New in EViews 5.0
- •What’s New in 5.0
- •Compatibility Notes
- •EViews 5.1 Update Overview
- •Overview of EViews 5.1 New Features
- •Preface
- •Part I. EViews Fundamentals
- •Chapter 1. Introduction
- •What is EViews?
- •Installing and Running EViews
- •Windows Basics
- •The EViews Window
- •Closing EViews
- •Where to Go For Help
- •Chapter 2. A Demonstration
- •Getting Data into EViews
- •Examining the Data
- •Estimating a Regression Model
- •Specification and Hypothesis Tests
- •Modifying the Equation
- •Forecasting from an Estimated Equation
- •Additional Testing
- •Chapter 3. Workfile Basics
- •What is a Workfile?
- •Creating a Workfile
- •The Workfile Window
- •Saving a Workfile
- •Loading a Workfile
- •Multi-page Workfiles
- •Addendum: File Dialog Features
- •Chapter 4. Object Basics
- •What is an Object?
- •Basic Object Operations
- •The Object Window
- •Working with Objects
- •Chapter 5. Basic Data Handling
- •Data Objects
- •Samples
- •Sample Objects
- •Importing Data
- •Exporting Data
- •Frequency Conversion
- •Importing ASCII Text Files
- •Chapter 6. Working with Data
- •Numeric Expressions
- •Series
- •Auto-series
- •Groups
- •Scalars
- •Chapter 7. Working with Data (Advanced)
- •Auto-Updating Series
- •Alpha Series
- •Date Series
- •Value Maps
- •Chapter 8. Series Links
- •Basic Link Concepts
- •Creating a Link
- •Working with Links
- •Chapter 9. Advanced Workfiles
- •Structuring a Workfile
- •Resizing a Workfile
- •Appending to a Workfile
- •Contracting a Workfile
- •Copying from a Workfile
- •Reshaping a Workfile
- •Sorting a Workfile
- •Exporting from a Workfile
- •Chapter 10. EViews Databases
- •Database Overview
- •Database Basics
- •Working with Objects in Databases
- •Database Auto-Series
- •The Database Registry
- •Querying the Database
- •Object Aliases and Illegal Names
- •Maintaining the Database
- •Foreign Format Databases
- •Working with DRIPro Links
- •Part II. Basic Data Analysis
- •Chapter 11. Series
- •Series Views Overview
- •Spreadsheet and Graph Views
- •Descriptive Statistics
- •Tests for Descriptive Stats
- •Distribution Graphs
- •One-Way Tabulation
- •Correlogram
- •Unit Root Test
- •BDS Test
- •Properties
- •Label
- •Series Procs Overview
- •Generate by Equation
- •Resample
- •Seasonal Adjustment
- •Exponential Smoothing
- •Hodrick-Prescott Filter
- •Frequency (Band-Pass) Filter
- •Chapter 12. Groups
- •Group Views Overview
- •Group Members
- •Spreadsheet
- •Dated Data Table
- •Graphs
- •Multiple Graphs
- •Descriptive Statistics
- •Tests of Equality
- •N-Way Tabulation
- •Principal Components
- •Correlations, Covariances, and Correlograms
- •Cross Correlations and Correlograms
- •Cointegration Test
- •Unit Root Test
- •Granger Causality
- •Label
- •Group Procedures Overview
- •Chapter 13. Statistical Graphs from Series and Groups
- •Distribution Graphs of Series
- •Scatter Diagrams with Fit Lines
- •Boxplots
- •Chapter 14. Graphs, Tables, and Text Objects
- •Creating Graphs
- •Modifying Graphs
- •Multiple Graphs
- •Printing Graphs
- •Copying Graphs to the Clipboard
- •Saving Graphs to a File
- •Graph Commands
- •Creating Tables
- •Table Basics
- •Basic Table Customization
- •Customizing Table Cells
- •Copying Tables to the Clipboard
- •Saving Tables to a File
- •Table Commands
- •Text Objects
- •Part III. Basic Single Equation Analysis
- •Chapter 15. Basic Regression
- •Equation Objects
- •Specifying an Equation in EViews
- •Estimating an Equation in EViews
- •Equation Output
- •Working with Equations
- •Estimation Problems
- •Chapter 16. Additional Regression Methods
- •Special Equation Terms
- •Weighted Least Squares
- •Heteroskedasticity and Autocorrelation Consistent Covariances
- •Two-stage Least Squares
- •Nonlinear Least Squares
- •Generalized Method of Moments (GMM)
- •Chapter 17. Time Series Regression
- •Serial Correlation Theory
- •Testing for Serial Correlation
- •Estimating AR Models
- •ARIMA Theory
- •Estimating ARIMA Models
- •ARMA Equation Diagnostics
- •Nonstationary Time Series
- •Unit Root Tests
- •Panel Unit Root Tests
- •Chapter 18. Forecasting from an Equation
- •Forecasting from Equations in EViews
- •An Illustration
- •Forecast Basics
- •Forecasting with ARMA Errors
- •Forecasting from Equations with Expressions
- •Forecasting with Expression and PDL Specifications
- •Chapter 19. Specification and Diagnostic Tests
- •Background
- •Coefficient Tests
- •Residual Tests
- •Specification and Stability Tests
- •Applications
- •Part IV. Advanced Single Equation Analysis
- •Chapter 20. ARCH and GARCH Estimation
- •Basic ARCH Specifications
- •Estimating ARCH Models in EViews
- •Working with ARCH Models
- •Additional ARCH Models
- •Examples
- •Binary Dependent Variable Models
- •Estimating Binary Models in EViews
- •Procedures for Binary Equations
- •Ordered Dependent Variable Models
- •Estimating Ordered Models in EViews
- •Views of Ordered Equations
- •Procedures for Ordered Equations
- •Censored Regression Models
- •Estimating Censored Models in EViews
- •Procedures for Censored Equations
- •Truncated Regression Models
- •Procedures for Truncated Equations
- •Count Models
- •Views of Count Models
- •Procedures for Count Models
- •Demonstrations
- •Technical Notes
- •Chapter 22. The Log Likelihood (LogL) Object
- •Overview
- •Specification
- •Estimation
- •LogL Views
- •LogL Procs
- •Troubleshooting
- •Limitations
- •Examples
- •Part V. Multiple Equation Analysis
- •Chapter 23. System Estimation
- •Background
- •System Estimation Methods
- •How to Create and Specify a System
- •Working With Systems
- •Technical Discussion
- •Vector Autoregressions (VARs)
- •Estimating a VAR in EViews
- •VAR Estimation Output
- •Views and Procs of a VAR
- •Structural (Identified) VARs
- •Cointegration Test
- •Vector Error Correction (VEC) Models
- •A Note on Version Compatibility
- •Chapter 25. State Space Models and the Kalman Filter
- •Background
- •Specifying a State Space Model in EViews
- •Working with the State Space
- •Converting from Version 3 Sspace
- •Technical Discussion
- •Chapter 26. Models
- •Overview
- •An Example Model
- •Building a Model
- •Working with the Model Structure
- •Specifying Scenarios
- •Using Add Factors
- •Solving the Model
- •Working with the Model Data
- •Part VI. Panel and Pooled Data
- •Chapter 27. Pooled Time Series, Cross-Section Data
- •The Pool Workfile
- •The Pool Object
- •Pooled Data
- •Setting up a Pool Workfile
- •Working with Pooled Data
- •Pooled Estimation
- •Chapter 28. Working with Panel Data
- •Structuring a Panel Workfile
- •Panel Workfile Display
- •Panel Workfile Information
- •Working with Panel Data
- •Basic Panel Analysis
- •Chapter 29. Panel Estimation
- •Estimating a Panel Equation
- •Panel Estimation Examples
- •Panel Equation Testing
- •Estimation Background
- •Appendix A. Global Options
- •The Options Menu
- •Print Setup
- •Appendix B. Wildcards
- •Wildcard Expressions
- •Using Wildcard Expressions
- •Source and Destination Patterns
- •Resolving Ambiguities
- •Wildcard versus Pool Identifier
- •Appendix C. Estimation and Solution Options
- •Setting Estimation Options
- •Optimization Algorithms
- •Nonlinear Equation Solution Methods
- •Appendix D. Gradients and Derivatives
- •Gradients
- •Derivatives
- •Appendix E. Information Criteria
- •Definitions
- •Using Information Criteria as a Guide to Model Selection
- •References
- •Index
- •Symbols
- •.DB? files 266
- •.EDB file 262
- •.RTF file 437
- •.WF1 file 62
- •@obsnum
- •Panel
- •@unmaptxt 174
- •~, in backup file name 62, 939
- •Numerics
- •3sls (three-stage least squares) 697, 716
- •Abort key 21
- •ARIMA models 501
- •ASCII
- •file export 115
- •ASCII file
- •See also Unit root tests.
- •Auto-search
- •Auto-series
- •in groups 144
- •Auto-updating series
- •and databases 152
- •Backcast
- •Berndt-Hall-Hall-Hausman (BHHH). See Optimization algorithms.
- •Bias proportion 554
- •fitted index 634
- •Binning option
- •classifications 313, 382
- •Boxplots 409
- •By-group statistics 312, 886, 893
- •coef vector 444
- •Causality
- •Granger's test 389
- •scale factor 649
- •Census X11
- •Census X12 337
- •Chi-square
- •Cholesky factor
- •Classification table
- •Close
- •Coef (coefficient vector)
- •default 444
- •Coefficient
- •Comparison operators
- •Conditional standard deviation
- •graph 610
- •Confidence interval
- •Constant
- •Copy
- •data cut-and-paste 107
- •table to clipboard 437
- •Covariance matrix
- •HAC (Newey-West) 473
- •heteroskedasticity consistent of estimated coefficients 472
- •Create
- •Cross-equation
- •Tukey option 393
- •CUSUM
- •sum of recursive residuals test 589
- •sum of recursive squared residuals test 590
- •Data
- •Database
- •link options 303
- •using auto-updating series with 152
- •Dates
- •Default
- •database 24, 266
- •set directory 71
- •Dependent variable
- •Description
- •Descriptive statistics
- •by group 312
- •group 379
- •individual samples (group) 379
- •Display format
- •Display name
- •Distribution
- •Dummy variables
- •for regression 452
- •lagged dependent variable 495
- •Dynamic forecasting 556
- •Edit
- •See also Unit root tests.
- •Equation
- •create 443
- •store 458
- •Estimation
- •EViews
- •Excel file
- •Excel files
- •Expectation-prediction table
- •Expected dependent variable
- •double 352
- •Export data 114
- •Extreme value
- •binary model 624
- •Fetch
- •File
- •save table to 438
- •Files
- •Fitted index
- •Fitted values
- •Font options
- •Fonts
- •Forecast
- •evaluation 553
- •Foreign data
- •Formula
- •forecast 561
- •Freq
- •DRI database 303
- •F-test
- •for variance equality 321
- •Full information maximum likelihood 698
- •GARCH 601
- •ARCH-M model 603
- •variance factor 668
- •system 716
- •Goodness-of-fit
- •Gradients 963
- •Graph
- •remove elements 423
- •Groups
- •display format 94
- •Groupwise heteroskedasticity 380
- •Help
- •Heteroskedasticity and autocorrelation consistent covariance (HAC) 473
- •History
- •Holt-Winters
- •Hypothesis tests
- •F-test 321
- •Identification
- •Identity
- •Import
- •Import data
- •See also VAR.
- •Index
- •Insert
- •Instruments 474
- •Iteration
- •Iteration option 953
- •in nonlinear least squares 483
- •J-statistic 491
- •J-test 596
- •Kernel
- •bivariate fit 405
- •choice in HAC weighting 704, 718
- •Kernel function
- •Keyboard
- •Kwiatkowski, Phillips, Schmidt, and Shin test 525
- •Label 82
- •Last_update
- •Last_write
- •Latent variable
- •Lead
- •make covariance matrix 643
- •List
- •LM test
- •ARCH 582
- •for binary models 622
- •LOWESS. See also LOESS
- •in ARIMA models 501
- •Mean absolute error 553
- •Metafile
- •Micro TSP
- •recoding 137
- •Models
- •add factors 777, 802
- •solving 804
- •Mouse 18
- •Multicollinearity 460
- •Name
- •Newey-West
- •Nonlinear coefficient restriction
- •Wald test 575
- •weighted two stage 486
- •Normal distribution
- •Numbers
- •chi-square tests 383
- •Object 73
- •Open
- •Option setting
- •Option settings
- •Or operator 98, 133
- •Ordinary residual
- •Panel
- •irregular 214
- •unit root tests 530
- •Paste 83
- •PcGive data 293
- •Polynomial distributed lag
- •Pool
- •Pool (object)
- •PostScript
- •Prediction table
- •Principal components 385
- •Program
- •p-value 569
- •for coefficient t-statistic 450
- •Quiet mode 939
- •RATS data
- •Read 832
- •CUSUM 589
- •Regression
- •Relational operators
- •Remarks
- •database 287
- •Residuals
- •Resize
- •Results
- •RichText Format
- •Robust standard errors
- •Robustness iterations
- •for regression 451
- •with AR specification 500
- •workfile 95
- •Save
- •Seasonal
- •Seasonal graphs 310
- •Select
- •single item 20
- •Serial correlation
- •theory 493
- •Series
- •Smoothing
- •Solve
- •Source
- •Specification test
- •Spreadsheet
- •Standard error
- •Standard error
- •binary models 634
- •Start
- •Starting values
- •Summary statistics
- •for regression variables 451
- •System
- •Table 429
- •font 434
- •Tabulation
- •Template 424
- •Tests. See also Hypothesis tests, Specification test and Goodness of fit.
- •Text file
- •open as workfile 54
- •Type
- •field in database query 282
- •Units
- •Update
- •Valmap
- •find label for value 173
- •find numeric value for label 174
- •Value maps 163
- •estimating 749
- •View
- •Wald test 572
- •nonlinear restriction 575
- •Watson test 323
- •Weighting matrix
- •heteroskedasticity and autocorrelation consistent (HAC) 718
- •kernel options 718
- •White
- •Window
- •Workfile
- •storage defaults 940
- •Write 844
- •XY line
- •Yates' continuity correction 321
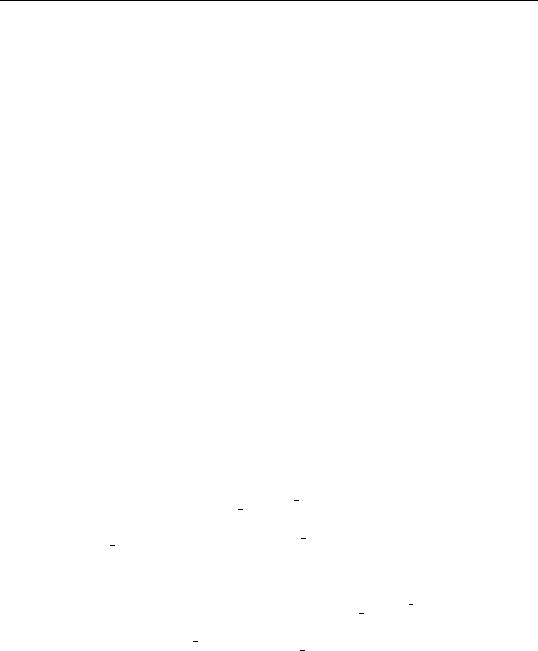
654—Chapter 21. Discrete and Limited Dependent Variable Models
To estimate the truncated model, press Object/Copy Object… from the tobit equation toolbar again. From the new untitled equation window that appears, press Estimate, mark the Truncated sample option, and click OK. Save the truncated regression by pressing the Name button, say as EQ_TR.
Then the LR test statistic and its p-value can be saved as a scalar by the commands:
scalar lr_test=2*(eq_bin.@logl+eq_tr.@logl-eq_tobit.@logl)
scalar lr_pval=1-@cchisq(lr_test,eq_tobit.@ncoef)
Double click on the scalar name to display the value in the status line at the bottom of the EViews window. For the example data set, the p-value is 0.066, which rejects the tobit model at the 10% level, but not at the 5% level.
For other specification tests for the tobit, see Greene (1997, 20.3.4) or Pagan and Vella (1989).
Truncated Regression Models
A close relative of the censored regression model is the truncated regression model. Suppose that an observation is not observed whenever the dependent variable falls below one threshold, or exceeds a second threshold. This sampling rule occurs, for example, in earnings function studies for low-income families that exclude observations with incomes above a threshold, and in studies of durables demand among individuals who purchase durables.
The general two-limit truncated regression model may be written as:
|
|
|
yi = xi′β + i |
(21.32) |
||||
where y |
i |
= y |
is only observed if: |
|
|
|
|
|
|
i |
|
|
< y < c |
|
|
|
|
|
|
|
c |
i |
i |
. |
(21.33) |
|
|
|
|
|
i |
|
|
If there is no lower truncation, then we can set ci = −∞ . If there is no upper truncation, then we set ci = ∞ .
The log likelihood function associated with these data is given by:
N |
|
l( β, σ) = Σ log f( ( yi − xi′β) ⁄ σ) 1( ci < yi < ci) |
(21.34) |
i = 1
− log ( F( ( ci − xi′β) ⁄ σ) − F( ( ci − xi′β) ⁄ σ) ).
The likelihood function is maximized with respect to β and σ , using standard iterative methods.

Procedures for Truncated Equations—655
Estimating a Truncated Model in EViews
Estimation of a truncated regression model follows the same steps as estimating a censored regression:
•Select Quick/Estimate Equation… from the main menu, and in the Equation Specification dialog, select the CENSORED estimation method. The censored and truncated regression dialog will appear.
•Enter the name of the truncated dependent variable and the list of the regressors in the Equation Specification field, and select one of the three distributions for the error term. You must enter your specification by list.
•Indicate that you wish to estimate the truncated model by checking the Truncated sample option.
•Specify the truncation points of the dependent variable by entering the appropriate expressions in the two edit fields. If you leave an edit field blank, EViews will assume that there is no truncation along that dimension.
You should keep a few points in mind. First, truncated estimation is only available for models where the truncation points are known, since the likelihood function is not otherwise defined. If you attempt to specify your truncation points by index, EViews will issue an error message indicating that this selection is not available.
Second, EViews will issue an error message if any values of the dependent variable are outside the truncation points. Furthermore, EViews will automatically exclude any observations that are exactly equal to a truncation point. Thus, if you specify zero as the lower truncation limit, EViews will issue an error message if any observations are less than zero, and will exclude any observations where the dependent variable exactly equals zero.
The cumulative distribution function and density of the assumed distribution will be used to form the likelihood function, as described above.
Procedures for Truncated Equations
EViews provides the same procedures for truncated equations as for censored equations. The residual and forecast calculations differ to reflect the truncated dependent variable and the different likelihood function.
Make Residual Series
Select Proc/Make Residual Series, and select from among the three types of residuals. The three types of residuals for censored models are defined as:

656—Chapter 21. Discrete and Limited Dependent Variable Models
Ordinary |
|
eoi |
= yi − E( yi |
|
|
ci |
|
ˆ |
ˆ |
|
|
|
|||||||||
|
|
|
|
< yi |
< ci;xi, β, σ) |
|||||
|
|
|
|
|
|
|
|
|
|
|
Standardized |
|
|
|
yi − E( yi |
|
|
ci |
|
ˆ |
ˆ |
|
|
|
|
|||||||
|
|
esi |
= |
|
|
< yi |
< ci;xi, β, σ) |
|||
|
|
----------------------------------------------------------------------------- |
|
ci |
|
ˆ |
ˆ - |
|||
|
|
|
|
|
||||||
|
|
|
|
var( yi |
|
|
< yi |
< ci;xi, β, σ) |
||
|
|
|
|
|
|
|
|
|
|
|
Generalized |
|
|
|
|
|
|
ˆ |
ˆ |
|
|
|
egi = − |
f′( ( yi − xi′β) ⁄ σ) |
|
|
||||||
|
------------------------------------------- |
|
′ ˆ |
ˆ |
|
|
||||
|
|
|
|
σf( ( yi − xiβ) ⁄ σ) |
|
|
||||
|
|
f |
|
ˆ |
ˆ |
|
|
|
ˆ |
ˆ |
|
− |
( ( ci − xi′β) ⁄ |
σ) − f( ( ci − xi′β) ⁄ |
σ) |
||||||
|
------------------------------------------------------------------------------------------------ |
|
ˆ |
⁄ |
|
ˆ |
|
ˆ |
ˆ - |
|
|
|
|
|
|
|
|||||
|
|
σ( F( ( ci − xi′ β) |
|
σ)−F( ( ci − xi′β) |
⁄ σ)) |
where f , F , are the density and distribution functions. Details on the computation of
E( i i < i < i ˆ ˆ ) are provided below. i β, σ
y c y c ;x ,
The generalized residuals may be used as the basis of a number of LM tests, including LM tests of normality (see Chesher and Irish (1984, 1987), and Gourieroux, Monfort and Trognon (1987); Greene (1997) provides a brief discussion and additional references).
Forecasting
EViews provides you with the option of forecasting the expected observed dependent vari-
able, E( yi |
|
ˆ ˆ |
|
|
ˆ ˆ |
|
|
||||
|
|||||
|
xi, β, σ) , or the expected latent variable, E( yi |
|
|
xi, β, σ) . |
To forecast the expected latent variable, select Forecast from the equation toolbar to open the forecast dialog, click on Index - Expected latent variable, and enter a name for the
|
|
|
|
|
|
|
ˆ |
ˆ |
|
|
|
|
|
|
|||
series to hold the output. The forecasts of the expected latent variable E( yi |
|
xi, β, σ) are |
||||||
computed using: |
|
|
ˆ |
|
ˆ |
|
|
|
ˆ |
|
|
ˆ |
|
|
(21.35) |
||
|
|
|
||||||
yi |
= E( yi |
|
xi, β, σ) = |
xi′β . |
|
|
To forecast the expected observed dependent variable for the truncated model, you should select Expected dependent variable, and enter a series name. These forecasts are computed using:
ˆ |
= |
E( yi |
|
|
ci |
|
ˆ ˆ |
(21.36) |
|
||||||||
yi |
|
|
< yi |
< ci;xi, β, σ) |
so that the expectations for the latent variable are taken with respect to the conditional (on being observed) distribution of the yi . Note that these forecasts always satisfy the inequality ci < yˆ i < ci .
It is instructive to compare this latter expected value with the expected value derived for the censored model in Equation (21.30) above (repeated here for convenience):
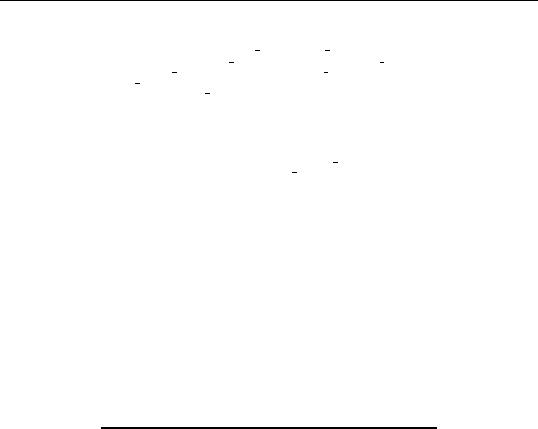
Procedures for Truncated Equations—657
ˆ |
(yi |
|
ˆ |
|
ˆ |
ci Pr(yi = ci |
|
ˆ |
ˆ |
|
|
(21.37) |
||
|
|
|
|
|||||||||||
yi = E |
|
xi, β, |
σ) = |
|
xi, β, σ) |
|
|
|||||||
|
|
ci |
|
< ci; |
ˆ ˆ |
|
|
< ci |
|
ˆ |
ˆ |
|||
|
|
|
||||||||||||
+ E(yi |
|
|
< yi |
xi, β, σ) Pr(ci |
< yi |
|
xi, β, σ) |
|||||||
+ ci Pr(yi = ci |
|
ˆ |
ˆ |
|
|
|
|
|
|
|||||
|
|
|
|
|
|
|
||||||||
|
xi, β, σ). |
|
|
|
|
|
|
The expected value of the dependent variable for the truncated model is the first part of the middle term of the censored expected value. The differences between the two expected values (the probability weight and the first and third terms) reflect the different treatment of latent observations that do not lie between ci and ci . In the censored case, those observations are included in the sample and are accounted for in the expected value. In the truncated case, data outside the interval are not observed and are not used in the expected value computation.
Illustration
As an example, we reestimate the Fair tobit model from above, truncating the data so that observations at or below zero are removed from the sample. The output from truncated estimation of the Fair model is presented below:
Dependent Variable: Y_PT
Method: ML - Censored Normal (TOBIT)
Date: 10/13/97 Time: 22:45
Sample(adjusted): 452 601
Included observations: 150 after adjusting endpoints
Truncated sample
Left censoring (value) at zero
Convergence achieved after 8 iterations
Covariance matrix computed using second derivatives
|
Coefficient |
Std. Error |
z-Statistic |
Prob. |
|
|
|
|
|
C |
12.37288 |
5.178306 |
2.389368 |
0.0169 |
Z1 |
-1.336872 |
1.453133 |
-0.919993 |
0.3576 |
Z2 |
-0.044792 |
0.116141 |
-0.385670 |
0.6997 |
Z3 |
0.544182 |
0.220119 |
2.472218 |
0.0134 |
Z4 |
-2.142896 |
1.787720 |
-1.198675 |
0.2307 |
Z5 |
-1.423128 |
0.600472 |
-2.370014 |
0.0178 |
Z6 |
-0.316721 |
0.322327 |
-0.982609 |
0.3258 |
Z7 |
0.621428 |
0.478827 |
1.297813 |
0.1944 |
Z8 |
-1.210037 |
0.552131 |
-2.191578 |
0.0284 |
|
|
|
|
|
|
Error Distribution |
|
|
|
|
|
|
|
|
SCALE:C(10) |
5.379557 |
0.688875 |
7.809196 |
0.0000 |
|
|
|
|
|
R-squared |
0.654664 |
Mean dependent var |
1.455907 |
|
Adjusted R-squared |
0.649405 |
S.D. dependent var |
3.298758 |
|
S.E. of regression |
1.953229 |
Akaike info criterion |
1.333891 |
|
Sum squared resid |
2254.726 |
Schwarz criterion |
1.407079 |
|
Log likelihood |
-390.8342 |
Hannan-Quinn criter. |
1.362379 |
|
Avg. log likelihood |
-0.650306 |
|
|
|
|
|
|
|
|
Left censored obs |
0 |
Right censored obs |
0 |
|
Uncensored obs |
150 |
Total obs |
|
150 |
|
|
|
|
|