
- •Table of Contents
- •What’s New in EViews 5.0
- •What’s New in 5.0
- •Compatibility Notes
- •EViews 5.1 Update Overview
- •Overview of EViews 5.1 New Features
- •Preface
- •Part I. EViews Fundamentals
- •Chapter 1. Introduction
- •What is EViews?
- •Installing and Running EViews
- •Windows Basics
- •The EViews Window
- •Closing EViews
- •Where to Go For Help
- •Chapter 2. A Demonstration
- •Getting Data into EViews
- •Examining the Data
- •Estimating a Regression Model
- •Specification and Hypothesis Tests
- •Modifying the Equation
- •Forecasting from an Estimated Equation
- •Additional Testing
- •Chapter 3. Workfile Basics
- •What is a Workfile?
- •Creating a Workfile
- •The Workfile Window
- •Saving a Workfile
- •Loading a Workfile
- •Multi-page Workfiles
- •Addendum: File Dialog Features
- •Chapter 4. Object Basics
- •What is an Object?
- •Basic Object Operations
- •The Object Window
- •Working with Objects
- •Chapter 5. Basic Data Handling
- •Data Objects
- •Samples
- •Sample Objects
- •Importing Data
- •Exporting Data
- •Frequency Conversion
- •Importing ASCII Text Files
- •Chapter 6. Working with Data
- •Numeric Expressions
- •Series
- •Auto-series
- •Groups
- •Scalars
- •Chapter 7. Working with Data (Advanced)
- •Auto-Updating Series
- •Alpha Series
- •Date Series
- •Value Maps
- •Chapter 8. Series Links
- •Basic Link Concepts
- •Creating a Link
- •Working with Links
- •Chapter 9. Advanced Workfiles
- •Structuring a Workfile
- •Resizing a Workfile
- •Appending to a Workfile
- •Contracting a Workfile
- •Copying from a Workfile
- •Reshaping a Workfile
- •Sorting a Workfile
- •Exporting from a Workfile
- •Chapter 10. EViews Databases
- •Database Overview
- •Database Basics
- •Working with Objects in Databases
- •Database Auto-Series
- •The Database Registry
- •Querying the Database
- •Object Aliases and Illegal Names
- •Maintaining the Database
- •Foreign Format Databases
- •Working with DRIPro Links
- •Part II. Basic Data Analysis
- •Chapter 11. Series
- •Series Views Overview
- •Spreadsheet and Graph Views
- •Descriptive Statistics
- •Tests for Descriptive Stats
- •Distribution Graphs
- •One-Way Tabulation
- •Correlogram
- •Unit Root Test
- •BDS Test
- •Properties
- •Label
- •Series Procs Overview
- •Generate by Equation
- •Resample
- •Seasonal Adjustment
- •Exponential Smoothing
- •Hodrick-Prescott Filter
- •Frequency (Band-Pass) Filter
- •Chapter 12. Groups
- •Group Views Overview
- •Group Members
- •Spreadsheet
- •Dated Data Table
- •Graphs
- •Multiple Graphs
- •Descriptive Statistics
- •Tests of Equality
- •N-Way Tabulation
- •Principal Components
- •Correlations, Covariances, and Correlograms
- •Cross Correlations and Correlograms
- •Cointegration Test
- •Unit Root Test
- •Granger Causality
- •Label
- •Group Procedures Overview
- •Chapter 13. Statistical Graphs from Series and Groups
- •Distribution Graphs of Series
- •Scatter Diagrams with Fit Lines
- •Boxplots
- •Chapter 14. Graphs, Tables, and Text Objects
- •Creating Graphs
- •Modifying Graphs
- •Multiple Graphs
- •Printing Graphs
- •Copying Graphs to the Clipboard
- •Saving Graphs to a File
- •Graph Commands
- •Creating Tables
- •Table Basics
- •Basic Table Customization
- •Customizing Table Cells
- •Copying Tables to the Clipboard
- •Saving Tables to a File
- •Table Commands
- •Text Objects
- •Part III. Basic Single Equation Analysis
- •Chapter 15. Basic Regression
- •Equation Objects
- •Specifying an Equation in EViews
- •Estimating an Equation in EViews
- •Equation Output
- •Working with Equations
- •Estimation Problems
- •Chapter 16. Additional Regression Methods
- •Special Equation Terms
- •Weighted Least Squares
- •Heteroskedasticity and Autocorrelation Consistent Covariances
- •Two-stage Least Squares
- •Nonlinear Least Squares
- •Generalized Method of Moments (GMM)
- •Chapter 17. Time Series Regression
- •Serial Correlation Theory
- •Testing for Serial Correlation
- •Estimating AR Models
- •ARIMA Theory
- •Estimating ARIMA Models
- •ARMA Equation Diagnostics
- •Nonstationary Time Series
- •Unit Root Tests
- •Panel Unit Root Tests
- •Chapter 18. Forecasting from an Equation
- •Forecasting from Equations in EViews
- •An Illustration
- •Forecast Basics
- •Forecasting with ARMA Errors
- •Forecasting from Equations with Expressions
- •Forecasting with Expression and PDL Specifications
- •Chapter 19. Specification and Diagnostic Tests
- •Background
- •Coefficient Tests
- •Residual Tests
- •Specification and Stability Tests
- •Applications
- •Part IV. Advanced Single Equation Analysis
- •Chapter 20. ARCH and GARCH Estimation
- •Basic ARCH Specifications
- •Estimating ARCH Models in EViews
- •Working with ARCH Models
- •Additional ARCH Models
- •Examples
- •Binary Dependent Variable Models
- •Estimating Binary Models in EViews
- •Procedures for Binary Equations
- •Ordered Dependent Variable Models
- •Estimating Ordered Models in EViews
- •Views of Ordered Equations
- •Procedures for Ordered Equations
- •Censored Regression Models
- •Estimating Censored Models in EViews
- •Procedures for Censored Equations
- •Truncated Regression Models
- •Procedures for Truncated Equations
- •Count Models
- •Views of Count Models
- •Procedures for Count Models
- •Demonstrations
- •Technical Notes
- •Chapter 22. The Log Likelihood (LogL) Object
- •Overview
- •Specification
- •Estimation
- •LogL Views
- •LogL Procs
- •Troubleshooting
- •Limitations
- •Examples
- •Part V. Multiple Equation Analysis
- •Chapter 23. System Estimation
- •Background
- •System Estimation Methods
- •How to Create and Specify a System
- •Working With Systems
- •Technical Discussion
- •Vector Autoregressions (VARs)
- •Estimating a VAR in EViews
- •VAR Estimation Output
- •Views and Procs of a VAR
- •Structural (Identified) VARs
- •Cointegration Test
- •Vector Error Correction (VEC) Models
- •A Note on Version Compatibility
- •Chapter 25. State Space Models and the Kalman Filter
- •Background
- •Specifying a State Space Model in EViews
- •Working with the State Space
- •Converting from Version 3 Sspace
- •Technical Discussion
- •Chapter 26. Models
- •Overview
- •An Example Model
- •Building a Model
- •Working with the Model Structure
- •Specifying Scenarios
- •Using Add Factors
- •Solving the Model
- •Working with the Model Data
- •Part VI. Panel and Pooled Data
- •Chapter 27. Pooled Time Series, Cross-Section Data
- •The Pool Workfile
- •The Pool Object
- •Pooled Data
- •Setting up a Pool Workfile
- •Working with Pooled Data
- •Pooled Estimation
- •Chapter 28. Working with Panel Data
- •Structuring a Panel Workfile
- •Panel Workfile Display
- •Panel Workfile Information
- •Working with Panel Data
- •Basic Panel Analysis
- •Chapter 29. Panel Estimation
- •Estimating a Panel Equation
- •Panel Estimation Examples
- •Panel Equation Testing
- •Estimation Background
- •Appendix A. Global Options
- •The Options Menu
- •Print Setup
- •Appendix B. Wildcards
- •Wildcard Expressions
- •Using Wildcard Expressions
- •Source and Destination Patterns
- •Resolving Ambiguities
- •Wildcard versus Pool Identifier
- •Appendix C. Estimation and Solution Options
- •Setting Estimation Options
- •Optimization Algorithms
- •Nonlinear Equation Solution Methods
- •Appendix D. Gradients and Derivatives
- •Gradients
- •Derivatives
- •Appendix E. Information Criteria
- •Definitions
- •Using Information Criteria as a Guide to Model Selection
- •References
- •Index
- •Symbols
- •.DB? files 266
- •.EDB file 262
- •.RTF file 437
- •.WF1 file 62
- •@obsnum
- •Panel
- •@unmaptxt 174
- •~, in backup file name 62, 939
- •Numerics
- •3sls (three-stage least squares) 697, 716
- •Abort key 21
- •ARIMA models 501
- •ASCII
- •file export 115
- •ASCII file
- •See also Unit root tests.
- •Auto-search
- •Auto-series
- •in groups 144
- •Auto-updating series
- •and databases 152
- •Backcast
- •Berndt-Hall-Hall-Hausman (BHHH). See Optimization algorithms.
- •Bias proportion 554
- •fitted index 634
- •Binning option
- •classifications 313, 382
- •Boxplots 409
- •By-group statistics 312, 886, 893
- •coef vector 444
- •Causality
- •Granger's test 389
- •scale factor 649
- •Census X11
- •Census X12 337
- •Chi-square
- •Cholesky factor
- •Classification table
- •Close
- •Coef (coefficient vector)
- •default 444
- •Coefficient
- •Comparison operators
- •Conditional standard deviation
- •graph 610
- •Confidence interval
- •Constant
- •Copy
- •data cut-and-paste 107
- •table to clipboard 437
- •Covariance matrix
- •HAC (Newey-West) 473
- •heteroskedasticity consistent of estimated coefficients 472
- •Create
- •Cross-equation
- •Tukey option 393
- •CUSUM
- •sum of recursive residuals test 589
- •sum of recursive squared residuals test 590
- •Data
- •Database
- •link options 303
- •using auto-updating series with 152
- •Dates
- •Default
- •database 24, 266
- •set directory 71
- •Dependent variable
- •Description
- •Descriptive statistics
- •by group 312
- •group 379
- •individual samples (group) 379
- •Display format
- •Display name
- •Distribution
- •Dummy variables
- •for regression 452
- •lagged dependent variable 495
- •Dynamic forecasting 556
- •Edit
- •See also Unit root tests.
- •Equation
- •create 443
- •store 458
- •Estimation
- •EViews
- •Excel file
- •Excel files
- •Expectation-prediction table
- •Expected dependent variable
- •double 352
- •Export data 114
- •Extreme value
- •binary model 624
- •Fetch
- •File
- •save table to 438
- •Files
- •Fitted index
- •Fitted values
- •Font options
- •Fonts
- •Forecast
- •evaluation 553
- •Foreign data
- •Formula
- •forecast 561
- •Freq
- •DRI database 303
- •F-test
- •for variance equality 321
- •Full information maximum likelihood 698
- •GARCH 601
- •ARCH-M model 603
- •variance factor 668
- •system 716
- •Goodness-of-fit
- •Gradients 963
- •Graph
- •remove elements 423
- •Groups
- •display format 94
- •Groupwise heteroskedasticity 380
- •Help
- •Heteroskedasticity and autocorrelation consistent covariance (HAC) 473
- •History
- •Holt-Winters
- •Hypothesis tests
- •F-test 321
- •Identification
- •Identity
- •Import
- •Import data
- •See also VAR.
- •Index
- •Insert
- •Instruments 474
- •Iteration
- •Iteration option 953
- •in nonlinear least squares 483
- •J-statistic 491
- •J-test 596
- •Kernel
- •bivariate fit 405
- •choice in HAC weighting 704, 718
- •Kernel function
- •Keyboard
- •Kwiatkowski, Phillips, Schmidt, and Shin test 525
- •Label 82
- •Last_update
- •Last_write
- •Latent variable
- •Lead
- •make covariance matrix 643
- •List
- •LM test
- •ARCH 582
- •for binary models 622
- •LOWESS. See also LOESS
- •in ARIMA models 501
- •Mean absolute error 553
- •Metafile
- •Micro TSP
- •recoding 137
- •Models
- •add factors 777, 802
- •solving 804
- •Mouse 18
- •Multicollinearity 460
- •Name
- •Newey-West
- •Nonlinear coefficient restriction
- •Wald test 575
- •weighted two stage 486
- •Normal distribution
- •Numbers
- •chi-square tests 383
- •Object 73
- •Open
- •Option setting
- •Option settings
- •Or operator 98, 133
- •Ordinary residual
- •Panel
- •irregular 214
- •unit root tests 530
- •Paste 83
- •PcGive data 293
- •Polynomial distributed lag
- •Pool
- •Pool (object)
- •PostScript
- •Prediction table
- •Principal components 385
- •Program
- •p-value 569
- •for coefficient t-statistic 450
- •Quiet mode 939
- •RATS data
- •Read 832
- •CUSUM 589
- •Regression
- •Relational operators
- •Remarks
- •database 287
- •Residuals
- •Resize
- •Results
- •RichText Format
- •Robust standard errors
- •Robustness iterations
- •for regression 451
- •with AR specification 500
- •workfile 95
- •Save
- •Seasonal
- •Seasonal graphs 310
- •Select
- •single item 20
- •Serial correlation
- •theory 493
- •Series
- •Smoothing
- •Solve
- •Source
- •Specification test
- •Spreadsheet
- •Standard error
- •Standard error
- •binary models 634
- •Start
- •Starting values
- •Summary statistics
- •for regression variables 451
- •System
- •Table 429
- •font 434
- •Tabulation
- •Template 424
- •Tests. See also Hypothesis tests, Specification test and Goodness of fit.
- •Text file
- •open as workfile 54
- •Type
- •field in database query 282
- •Units
- •Update
- •Valmap
- •find label for value 173
- •find numeric value for label 174
- •Value maps 163
- •estimating 749
- •View
- •Wald test 572
- •nonlinear restriction 575
- •Watson test 323
- •Weighting matrix
- •heteroskedasticity and autocorrelation consistent (HAC) 718
- •kernel options 718
- •White
- •Window
- •Workfile
- •storage defaults 940
- •Write 844
- •XY line
- •Yates' continuity correction 321

662—Chapter 21. Discrete and Limited Dependent Variable Models
Negative Binomial
If we maximize the negative binomial log likelihood, given above, for fixed η2 , we obtain the QMLE of the conditional mean parameters β . This QML estimator is consistent even if the conditional distribution of y is not negative binomial, provided that mi is correctly specified.
EViews sets η2 = 1 by default, which is a special case known as the geometric distribution. You may specify any other (positive) value by changing the number in the Fixed variance parameter field box. For the negative binomial QMLE, EViews by default reports the robust QMLE standard errors.
Views of Count Models
EViews provides a full complement of views of count models. You can examine the estimation output, compute frequencies for the dependent variable, view the covariance matrix, or perform coefficient tests. Additionally, you can select View/Actual, Fitted, Residual…
and pick from a number of views describing the ordinary residuals oi i− ( i ˆ ) , e = y m x , β
or you can examine the correlogram and histogram of these residuals. For the most part, all of these views are self-explanatory.
Note, however, that the LR test statistics presented in the summary statistics at the bottom of the equation output, or as computed under the View/Coefficient Tests/Redundant Variables - Likelihood Ratio… have a known asymptotic distribution only if the conditional distribution is correctly specified. Under the weaker GLM assumption that the true variance is proportional to the nominal variance, we can form a quasi-likelihood ratio,
QLR = |
ˆ 2 |
ˆ |
2 |
is the estimated proportional variance factor. This QLR sta- |
LR ⁄ σ |
, where σ |
|
tistic has an asymptotic χ2 distribution under the assumption that the mean is correctly specified and that the variances follow the GLM structure. EViews does not compute the
ˆ |
2 |
based upon the stan- |
QLR statistic, but it can be estimated by computing an estimate of σ |
|
dardized residuals. We provide an example of the use of the QLR test statistic below.
If the GLM assumption does not hold, then there is no usable QLR test statistic with a known distribution; see Wooldridge (1996).
Procedures for Count Models
Most of the procedures are self-explanatory. Some details are required for the forecasting and residual creation procedures.
• Forecast… provides you the option to forecast the dependent variable |
y |
i |
or the pre- |
||||||
dicted linear index x |
′ ˆ |
|
|
|
|
|
are |
||
β . Note that for all of these models the forecasts of y |
|
||||||||
ˆ |
|
i ˆ |
ˆ |
′ ˆ |
|
|
|
i |
|
given by yi = |
m( xi, β) |
where m(xi, β) = |
exp ( xiβ) . |
|
|
|
|
|

Demonstrations—663
•Make Residual Series… provides the following three types of residuals for count models:
Ordinary |
eoi |
ˆ |
|
= yi−m( xi, β) |
|
Standardized (Pearson) |
|
ˆ |
|
esi |
yi− m( xi, β) |
|
= ------------------------------ |
|
|
|
ˆ ˆ |
|
|
v( xi, β, γ) |
Generalized |
|
eg =(varies) |
|
|
|
where the γ represents any additional parameters in the variance specification. Note that the specification of the variances may vary significantly between specifications.
For example, the Poisson model has v( x |
ˆ |
ˆ |
|||
, β) = |
m( x , β) , while the exponential |
||||
ˆ |
ˆ |
2 |
i |
|
i |
has v( xi, β) = |
m( xi, β) |
|
. |
|
|
The generalized residuals can be used to obtain the score vector by multiplying the generalized residuals by each variable in x . These scores can be used in a variety of LM or conditional moment tests for specification testing; see Wooldridge (1996).
Demonstrations
A Specification Test for Overdispersion
Consider the model:
NUMBi = β1 + β2IPi + β3FEBi + i , |
(21.48) |
where the dependent variable NUMB is the number of strikes, IP is a measure of industrial production, and FEB is a February dummy variable, as reported in Kennan (1985, Table 1).
The results from Poisson estimation of this model are presented below:

664—Chapter 21. Discrete and Limited Dependent Variable Models
Dependent Variable: NUMB
Method: ML/QML - Poisson Count
Date: 09/14/97 Time: 10:58
Sample: 1 103
Included observations: 103
Convergence achieved after 4 iterations
Covariance matrix computed using second derivatives
Variable |
Coefficient |
Std. Error |
z-Statistic |
Prob. |
|
|
|
|
|
C |
1.725630 |
0.043656 |
39.52764 |
0.0000 |
IP |
2.775334 |
0.819104 |
3.388254 |
0.0007 |
FEB |
-0.377407 |
0.174520 |
-2.162539 |
0.0306 |
|
|
|
|
|
R-squared |
0.064502 |
Mean dependent var |
5.495146 |
|
Adjusted R-squared |
0.045792 |
S.D. dependent var |
3.653829 |
|
S.E. of regression |
3.569190 |
Akaike info criterion |
5.583421 |
|
Sum squared resid |
1273.912 |
Schwarz criterion |
5.660160 |
|
Log likelihood |
-284.5462 |
Hannan-Quinn criter. |
5.614503 |
|
Restr. log likelihood |
-292.9694 |
Avg. log likelihood |
-2.762584 |
|
LR statistic (2 df) |
16.84645 |
LR index (Pseudo-R2) |
0.028751 |
|
Probability(LR stat) |
0.000220 |
|
|
|
|
|
|
|
|
Cameron and Trivedi (1990) propose a regression based test of the Poisson restriction
v( xi, β) = m( xi, β) . To carry out the test, first estimate the Poisson model and obtain the fitted values of the dependent variable. Click Forecast and provide a name for the forecasted dependent variable, say NUMB_F. The test is based on an auxiliary regression of
2 |
|
ˆ 2 |
|
|
|
|
|
eoi |
− yi on yi and testing the significance of the regression coefficient. For this example, |
||||||
the test regression can be estimated by the command: |
|
|
|
||||
|
ls (numb-numb_f)^2-numb numb_f^2 |
|
|
|
|||
yielding the following results: |
|
|
|
|
|
||
|
|
Dependent Variable: (NUMB-NUMB_F)^2-NUMB |
|
|
|
||
|
|
Method: Least Squares |
|
|
|
|
|
|
|
Date: 09/14/97 Time: 11:05 |
|
|
|
|
|
|
|
Sample: 1 103 |
|
|
|
|
|
|
|
Included observations: 103 |
|
|
|
|
|
|
|
|
|
|
|
|
|
|
|
Variable |
Coefficient |
Std. Error |
t-Statistic |
Prob. |
|
|
|
|
|
|
|
|
|
|
|
NUMB_F^2 |
0.238874 |
0.052115 |
4.583571 |
0.0000 |
|
|
|
|
|
|
|
|
|
|
|
R-squared |
0.043930 |
Mean dependent var |
6.872929 |
|
|
|
|
Adjusted R-squared |
0.043930 |
S.D. dependent var |
17.65726 |
|
|
|
|
S.E. of regression |
17.26506 |
Akaike info criterion |
8.544908 |
|
|
|
|
Sum squared resid |
30404.41 |
Schwarz criterion |
8.570488 |
|
|
|
|
Log likelihood |
-439.0628 |
Durbin-Watson stat |
1.711805 |
|
|
|
|
|
|
|
|
|
|
The t-statistic of the coefficient is highly significant, leading us to reject the Poisson restriction. Moreover, the estimated coefficient is significantly positive, indicating overdispersion in the residuals.
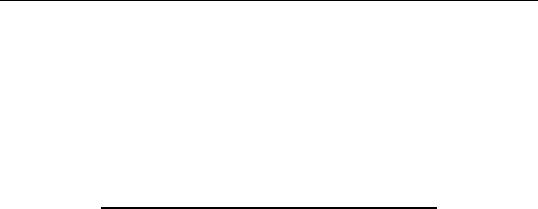
Demonstrations—665
An alternative approach, suggested by Wooldridge (1996), is to regress esi − 1 |
ˆ |
, on yi . To |
perform this test, select Proc/Make Residual Series… and select Standardized. Save the results in a series, say SRESID. Then estimating the regression specification:
sresid^2-1 numbf yields the results:
Dependent Variable: SRESID^2-1
Method: Least Squares
Date: 10/06/97 Time: 16:05
Sample: 1 103
Included observations: 103
Variable |
Coefficient |
Std. Error |
t-Statistic |
Prob. |
|
|
|
|
|
NUMBF |
0.221238 |
0.055002 |
4.022326 |
0.0001 |
|
|
|
|
|
R-squared |
0.017556 |
Mean dependent var |
1.161573 |
|
Adjusted R-squared |
0.017556 |
S.D. dependent var |
3.138974 |
|
S.E. of regression |
3.111299 |
Akaike info criterion |
5.117619 |
|
Sum squared resid |
987.3786 |
Schwarz criterion |
5.143199 |
|
Log likelihood |
-262.5574 |
Durbin-Watson stat |
1.764537 |
|
|
|
|
|
|
Both tests suggest the presence of overdispersion, with the variance approximated by v = m( 1 + 0.23m) .
Given the evidence of overdispersion and the rejection of the Poisson restriction, we will re-estimate the model, allowing for mean-variance inequality. Our approach will be to estimate the two-step negative binomial QMLE specification (termed the quasi-generalized pseudo-maximum likelihood estimator by Gourieroux, Monfort, and Trognon (1984a, b))
using the estimate of ˆ 2 derived above. To compute this estimator, simply select Negative
η
Binomial (QML) and enter 0.22124 in the edit field for Fixed variance parameter.
We will use the GLM variance calculations, so you should click on Option in the Equation Specification dialog and mark the Robust Covariance and GLM options. The estimation results are shown below:

666—Chapter 21. Discrete and Limited Dependent Variable Models
Dependent Variable: NUMB
Method: QML - Negative Binomial Count
Date: 10/11/97 Time: 23:53
Sample: 1 103
Included observations: 103
QML parameter used in estimation: 0.22124
Convergence achieved after 3 iterations
GLM Robust Standard Errors & Covariance
Variance factor estimate = 2.465660162
Covariance matrix computed using second derivatives
Variable |
Coefficient |
Std. Error |
z-Statistic |
Prob. |
|
|
|
|
|
C |
1.724906 |
0.102543 |
16.82135 |
0.0000 |
IP |
2.833103 |
1.919447 |
1.475999 |
0.1399 |
FEB |
-0.369558 |
0.377376 |
-0.979285 |
0.3274 |
|
|
|
|
|
R-squared |
0.064374 |
Mean dependent var |
5.495146 |
|
Adjusted R-squared |
0.045661 |
S.D. dependent var |
3.653829 |
|
S.E. of regression |
3.569435 |
Akaike info criterion |
5.174385 |
|
Sum squared resid |
1274.087 |
Schwarz criterion |
5.251125 |
|
Log likelihood |
-263.4808 |
Hannan-Quinn criter. |
5.205468 |
|
Restr. log likelihood |
-522.9973 |
Avg. log likelihood |
-2.558066 |
|
LR statistic (2 df) |
519.0330 |
LR index (Pseudo-R2) |
0.496210 |
|
Probability(LR stat) |
0.000000 |
|
|
|
|
|
|
|
|
The header indicates that the estimated GLM variance factor is 2.4, suggesting that the negative binomial ML would not have been an appropriate specification. Nevertheless, the negative binomial QML should be consistent, and under the GLM assumption, the standard errors should be consistently estimated. It is worth noting that the coefficients on IP and FEB, which were strongly statistically significant in the Poisson specification, are no longer significantly different from zero at conventional significance levels.
Quasi-likelihood Ratio Statistic
As described by Wooldridge (1996), specification testing using likelihood ratio statistics requires some care when based upon QML models. We illustrate here the differences between a standard LR test for significant coefficients and the corresponding QLR statistic.
From the results above, we know that the overall likelihood ratio statistic for the Poisson model is 16.85, with a corresponding p-value of 0.0002. This statistic is valid under the assumption that m(xi, β) is specified correctly and that the mean-variance equality holds.
We can decisively reject the latter hypothesis, suggesting that we should derive the QML estimator with consistently estimated covariance matrix under the GLM variance assumption. While EViews does not automatically adjust the LR statistic to reflect the QML assumption, it is easy enough to compute the adjustment by hand. Following Wooldridge, we construct the QLR statistic by dividing the original LR statistic by the estimated GLM variance factor.