
- •Table of Contents
- •What’s New in EViews 5.0
- •What’s New in 5.0
- •Compatibility Notes
- •EViews 5.1 Update Overview
- •Overview of EViews 5.1 New Features
- •Preface
- •Part I. EViews Fundamentals
- •Chapter 1. Introduction
- •What is EViews?
- •Installing and Running EViews
- •Windows Basics
- •The EViews Window
- •Closing EViews
- •Where to Go For Help
- •Chapter 2. A Demonstration
- •Getting Data into EViews
- •Examining the Data
- •Estimating a Regression Model
- •Specification and Hypothesis Tests
- •Modifying the Equation
- •Forecasting from an Estimated Equation
- •Additional Testing
- •Chapter 3. Workfile Basics
- •What is a Workfile?
- •Creating a Workfile
- •The Workfile Window
- •Saving a Workfile
- •Loading a Workfile
- •Multi-page Workfiles
- •Addendum: File Dialog Features
- •Chapter 4. Object Basics
- •What is an Object?
- •Basic Object Operations
- •The Object Window
- •Working with Objects
- •Chapter 5. Basic Data Handling
- •Data Objects
- •Samples
- •Sample Objects
- •Importing Data
- •Exporting Data
- •Frequency Conversion
- •Importing ASCII Text Files
- •Chapter 6. Working with Data
- •Numeric Expressions
- •Series
- •Auto-series
- •Groups
- •Scalars
- •Chapter 7. Working with Data (Advanced)
- •Auto-Updating Series
- •Alpha Series
- •Date Series
- •Value Maps
- •Chapter 8. Series Links
- •Basic Link Concepts
- •Creating a Link
- •Working with Links
- •Chapter 9. Advanced Workfiles
- •Structuring a Workfile
- •Resizing a Workfile
- •Appending to a Workfile
- •Contracting a Workfile
- •Copying from a Workfile
- •Reshaping a Workfile
- •Sorting a Workfile
- •Exporting from a Workfile
- •Chapter 10. EViews Databases
- •Database Overview
- •Database Basics
- •Working with Objects in Databases
- •Database Auto-Series
- •The Database Registry
- •Querying the Database
- •Object Aliases and Illegal Names
- •Maintaining the Database
- •Foreign Format Databases
- •Working with DRIPro Links
- •Part II. Basic Data Analysis
- •Chapter 11. Series
- •Series Views Overview
- •Spreadsheet and Graph Views
- •Descriptive Statistics
- •Tests for Descriptive Stats
- •Distribution Graphs
- •One-Way Tabulation
- •Correlogram
- •Unit Root Test
- •BDS Test
- •Properties
- •Label
- •Series Procs Overview
- •Generate by Equation
- •Resample
- •Seasonal Adjustment
- •Exponential Smoothing
- •Hodrick-Prescott Filter
- •Frequency (Band-Pass) Filter
- •Chapter 12. Groups
- •Group Views Overview
- •Group Members
- •Spreadsheet
- •Dated Data Table
- •Graphs
- •Multiple Graphs
- •Descriptive Statistics
- •Tests of Equality
- •N-Way Tabulation
- •Principal Components
- •Correlations, Covariances, and Correlograms
- •Cross Correlations and Correlograms
- •Cointegration Test
- •Unit Root Test
- •Granger Causality
- •Label
- •Group Procedures Overview
- •Chapter 13. Statistical Graphs from Series and Groups
- •Distribution Graphs of Series
- •Scatter Diagrams with Fit Lines
- •Boxplots
- •Chapter 14. Graphs, Tables, and Text Objects
- •Creating Graphs
- •Modifying Graphs
- •Multiple Graphs
- •Printing Graphs
- •Copying Graphs to the Clipboard
- •Saving Graphs to a File
- •Graph Commands
- •Creating Tables
- •Table Basics
- •Basic Table Customization
- •Customizing Table Cells
- •Copying Tables to the Clipboard
- •Saving Tables to a File
- •Table Commands
- •Text Objects
- •Part III. Basic Single Equation Analysis
- •Chapter 15. Basic Regression
- •Equation Objects
- •Specifying an Equation in EViews
- •Estimating an Equation in EViews
- •Equation Output
- •Working with Equations
- •Estimation Problems
- •Chapter 16. Additional Regression Methods
- •Special Equation Terms
- •Weighted Least Squares
- •Heteroskedasticity and Autocorrelation Consistent Covariances
- •Two-stage Least Squares
- •Nonlinear Least Squares
- •Generalized Method of Moments (GMM)
- •Chapter 17. Time Series Regression
- •Serial Correlation Theory
- •Testing for Serial Correlation
- •Estimating AR Models
- •ARIMA Theory
- •Estimating ARIMA Models
- •ARMA Equation Diagnostics
- •Nonstationary Time Series
- •Unit Root Tests
- •Panel Unit Root Tests
- •Chapter 18. Forecasting from an Equation
- •Forecasting from Equations in EViews
- •An Illustration
- •Forecast Basics
- •Forecasting with ARMA Errors
- •Forecasting from Equations with Expressions
- •Forecasting with Expression and PDL Specifications
- •Chapter 19. Specification and Diagnostic Tests
- •Background
- •Coefficient Tests
- •Residual Tests
- •Specification and Stability Tests
- •Applications
- •Part IV. Advanced Single Equation Analysis
- •Chapter 20. ARCH and GARCH Estimation
- •Basic ARCH Specifications
- •Estimating ARCH Models in EViews
- •Working with ARCH Models
- •Additional ARCH Models
- •Examples
- •Binary Dependent Variable Models
- •Estimating Binary Models in EViews
- •Procedures for Binary Equations
- •Ordered Dependent Variable Models
- •Estimating Ordered Models in EViews
- •Views of Ordered Equations
- •Procedures for Ordered Equations
- •Censored Regression Models
- •Estimating Censored Models in EViews
- •Procedures for Censored Equations
- •Truncated Regression Models
- •Procedures for Truncated Equations
- •Count Models
- •Views of Count Models
- •Procedures for Count Models
- •Demonstrations
- •Technical Notes
- •Chapter 22. The Log Likelihood (LogL) Object
- •Overview
- •Specification
- •Estimation
- •LogL Views
- •LogL Procs
- •Troubleshooting
- •Limitations
- •Examples
- •Part V. Multiple Equation Analysis
- •Chapter 23. System Estimation
- •Background
- •System Estimation Methods
- •How to Create and Specify a System
- •Working With Systems
- •Technical Discussion
- •Vector Autoregressions (VARs)
- •Estimating a VAR in EViews
- •VAR Estimation Output
- •Views and Procs of a VAR
- •Structural (Identified) VARs
- •Cointegration Test
- •Vector Error Correction (VEC) Models
- •A Note on Version Compatibility
- •Chapter 25. State Space Models and the Kalman Filter
- •Background
- •Specifying a State Space Model in EViews
- •Working with the State Space
- •Converting from Version 3 Sspace
- •Technical Discussion
- •Chapter 26. Models
- •Overview
- •An Example Model
- •Building a Model
- •Working with the Model Structure
- •Specifying Scenarios
- •Using Add Factors
- •Solving the Model
- •Working with the Model Data
- •Part VI. Panel and Pooled Data
- •Chapter 27. Pooled Time Series, Cross-Section Data
- •The Pool Workfile
- •The Pool Object
- •Pooled Data
- •Setting up a Pool Workfile
- •Working with Pooled Data
- •Pooled Estimation
- •Chapter 28. Working with Panel Data
- •Structuring a Panel Workfile
- •Panel Workfile Display
- •Panel Workfile Information
- •Working with Panel Data
- •Basic Panel Analysis
- •Chapter 29. Panel Estimation
- •Estimating a Panel Equation
- •Panel Estimation Examples
- •Panel Equation Testing
- •Estimation Background
- •Appendix A. Global Options
- •The Options Menu
- •Print Setup
- •Appendix B. Wildcards
- •Wildcard Expressions
- •Using Wildcard Expressions
- •Source and Destination Patterns
- •Resolving Ambiguities
- •Wildcard versus Pool Identifier
- •Appendix C. Estimation and Solution Options
- •Setting Estimation Options
- •Optimization Algorithms
- •Nonlinear Equation Solution Methods
- •Appendix D. Gradients and Derivatives
- •Gradients
- •Derivatives
- •Appendix E. Information Criteria
- •Definitions
- •Using Information Criteria as a Guide to Model Selection
- •References
- •Index
- •Symbols
- •.DB? files 266
- •.EDB file 262
- •.RTF file 437
- •.WF1 file 62
- •@obsnum
- •Panel
- •@unmaptxt 174
- •~, in backup file name 62, 939
- •Numerics
- •3sls (three-stage least squares) 697, 716
- •Abort key 21
- •ARIMA models 501
- •ASCII
- •file export 115
- •ASCII file
- •See also Unit root tests.
- •Auto-search
- •Auto-series
- •in groups 144
- •Auto-updating series
- •and databases 152
- •Backcast
- •Berndt-Hall-Hall-Hausman (BHHH). See Optimization algorithms.
- •Bias proportion 554
- •fitted index 634
- •Binning option
- •classifications 313, 382
- •Boxplots 409
- •By-group statistics 312, 886, 893
- •coef vector 444
- •Causality
- •Granger's test 389
- •scale factor 649
- •Census X11
- •Census X12 337
- •Chi-square
- •Cholesky factor
- •Classification table
- •Close
- •Coef (coefficient vector)
- •default 444
- •Coefficient
- •Comparison operators
- •Conditional standard deviation
- •graph 610
- •Confidence interval
- •Constant
- •Copy
- •data cut-and-paste 107
- •table to clipboard 437
- •Covariance matrix
- •HAC (Newey-West) 473
- •heteroskedasticity consistent of estimated coefficients 472
- •Create
- •Cross-equation
- •Tukey option 393
- •CUSUM
- •sum of recursive residuals test 589
- •sum of recursive squared residuals test 590
- •Data
- •Database
- •link options 303
- •using auto-updating series with 152
- •Dates
- •Default
- •database 24, 266
- •set directory 71
- •Dependent variable
- •Description
- •Descriptive statistics
- •by group 312
- •group 379
- •individual samples (group) 379
- •Display format
- •Display name
- •Distribution
- •Dummy variables
- •for regression 452
- •lagged dependent variable 495
- •Dynamic forecasting 556
- •Edit
- •See also Unit root tests.
- •Equation
- •create 443
- •store 458
- •Estimation
- •EViews
- •Excel file
- •Excel files
- •Expectation-prediction table
- •Expected dependent variable
- •double 352
- •Export data 114
- •Extreme value
- •binary model 624
- •Fetch
- •File
- •save table to 438
- •Files
- •Fitted index
- •Fitted values
- •Font options
- •Fonts
- •Forecast
- •evaluation 553
- •Foreign data
- •Formula
- •forecast 561
- •Freq
- •DRI database 303
- •F-test
- •for variance equality 321
- •Full information maximum likelihood 698
- •GARCH 601
- •ARCH-M model 603
- •variance factor 668
- •system 716
- •Goodness-of-fit
- •Gradients 963
- •Graph
- •remove elements 423
- •Groups
- •display format 94
- •Groupwise heteroskedasticity 380
- •Help
- •Heteroskedasticity and autocorrelation consistent covariance (HAC) 473
- •History
- •Holt-Winters
- •Hypothesis tests
- •F-test 321
- •Identification
- •Identity
- •Import
- •Import data
- •See also VAR.
- •Index
- •Insert
- •Instruments 474
- •Iteration
- •Iteration option 953
- •in nonlinear least squares 483
- •J-statistic 491
- •J-test 596
- •Kernel
- •bivariate fit 405
- •choice in HAC weighting 704, 718
- •Kernel function
- •Keyboard
- •Kwiatkowski, Phillips, Schmidt, and Shin test 525
- •Label 82
- •Last_update
- •Last_write
- •Latent variable
- •Lead
- •make covariance matrix 643
- •List
- •LM test
- •ARCH 582
- •for binary models 622
- •LOWESS. See also LOESS
- •in ARIMA models 501
- •Mean absolute error 553
- •Metafile
- •Micro TSP
- •recoding 137
- •Models
- •add factors 777, 802
- •solving 804
- •Mouse 18
- •Multicollinearity 460
- •Name
- •Newey-West
- •Nonlinear coefficient restriction
- •Wald test 575
- •weighted two stage 486
- •Normal distribution
- •Numbers
- •chi-square tests 383
- •Object 73
- •Open
- •Option setting
- •Option settings
- •Or operator 98, 133
- •Ordinary residual
- •Panel
- •irregular 214
- •unit root tests 530
- •Paste 83
- •PcGive data 293
- •Polynomial distributed lag
- •Pool
- •Pool (object)
- •PostScript
- •Prediction table
- •Principal components 385
- •Program
- •p-value 569
- •for coefficient t-statistic 450
- •Quiet mode 939
- •RATS data
- •Read 832
- •CUSUM 589
- •Regression
- •Relational operators
- •Remarks
- •database 287
- •Residuals
- •Resize
- •Results
- •RichText Format
- •Robust standard errors
- •Robustness iterations
- •for regression 451
- •with AR specification 500
- •workfile 95
- •Save
- •Seasonal
- •Seasonal graphs 310
- •Select
- •single item 20
- •Serial correlation
- •theory 493
- •Series
- •Smoothing
- •Solve
- •Source
- •Specification test
- •Spreadsheet
- •Standard error
- •Standard error
- •binary models 634
- •Start
- •Starting values
- •Summary statistics
- •for regression variables 451
- •System
- •Table 429
- •font 434
- •Tabulation
- •Template 424
- •Tests. See also Hypothesis tests, Specification test and Goodness of fit.
- •Text file
- •open as workfile 54
- •Type
- •field in database query 282
- •Units
- •Update
- •Valmap
- •find label for value 173
- •find numeric value for label 174
- •Value maps 163
- •estimating 749
- •View
- •Wald test 572
- •nonlinear restriction 575
- •Watson test 323
- •Weighting matrix
- •heteroskedasticity and autocorrelation consistent (HAC) 718
- •kernel options 718
- •White
- •Window
- •Workfile
- •storage defaults 940
- •Write 844
- •XY line
- •Yates' continuity correction 321

Two-stage Least Squares—473
HAC Consistent Covariances (Newey-West)
The White covariance matrix described above assumes that the residuals of the estimated equation are serially uncorrelated. Newey and West (1987) have proposed a more general covariance estimator that is consistent in the presence of both heteroskedasticity and autocorrelation of unknown form. The Newey-West estimator is given by,
|
|
|
|
ˆ |
|
|
T |
( X′X) |
−1 ˆ |
−1 |
, |
|
|
|
|
|
ΣNW = ------------ |
Ω( X′X) |
|
|
|||||
|
|
|
|
|
|
T |
− k |
|
|
|
|
|
where: |
|
|
|
|
|
|
|
|
|
|
|
|
ˆ |
|
T |
|
T |
2 |
|
|
|
|
|
|
|
Ω = |
------------ |
Σ ut xtxt′ |
|
|
|
|
|
|
||||
|
T − k t = 1 |
|
|
|
|
|
|
|
|
|||
q |
|
|
− |
v |
|
T |
( xtutut − vxt − v′ + xt − vut − vutxt′) |
|
||||
+ Σ |
1 |
------------ |
Σ |
|
||||||||
v = 1 |
|
|
q + 1 t = v + 1 |
|
|
|
|
|
|
(16.14)
(16.15)
and q , the truncation lag, is a parameter representing the number of autocorrelations used in evaluating the dynamics of the OLS residuals ut . Following the suggestion of Newey and West, EViews sets q using the formula:
q = floor( 4( T ⁄ 100)2 ⁄ 9 ) . |
(16.16) |
To use the Newey-West method, select the Options tab in the Equation Estimation. Check the box labeled Heteroskedasticity Consistent Covariance and press the Newey-West radio button.
Two-stage Least Squares
A fundamental assumption of regression analysis is that the right-hand side variables are uncorrelated with the disturbance term. If this assumption is violated, both OLS and weighted LS are biased and inconsistent.
There are a number of situations where some of the right-hand side variables are correlated with disturbances. Some classic examples occur when:
•There are endogenously determined variables on the right-hand side of the equation.
•Right-hand side variables are measured with error.
For simplicity, we will refer to variables that are correlated with the residuals as endogenous, and variables that are not correlated with the residuals as exogenous or predetermined.
The standard approach in cases where right-hand side variables are correlated with the residuals is to estimate the equation using instrumental variables regression. The idea

474—Chapter 16. Additional Regression Methods
behind instrumental variables is to find a set of variables, termed instruments, that are both (1) correlated with the explanatory variables in the equation, and (2) uncorrelated with the disturbances. These instruments are used to eliminate the correlation between right-hand side variables and the disturbances.
Two-stage least squares (TSLS) is a special case of instrumental variables regression. As the name suggests, there are two distinct stages in two-stage least squares. In the first stage, TSLS finds the portions of the endogenous and exogenous variables that can be attributed to the instruments. This stage involves estimating an OLS regression of each variable in the model on the set of instruments. The second stage is a regression of the original equation, with all of the variables replaced by the fitted values from the first-stage regressions. The coefficients of this regression are the TSLS estimates.
You need not worry about the separate stages of TSLS since EViews will estimate both stages simultaneously using instrumental variables techniques. More formally, let Z be the matrix of instruments, and let y and X be the dependent and explanatory variables. Then the coefficients computed in two-stage least squares are given by,
bTSLS = ( X′Z( Z′Z )−1Z′X)−1X′Z( Z′Z) −1Z′y , |
(16.17) |
and the estimated covariance matrix of these coefficients is given by:
ˆ |
2 |
( X′ Z( Z′Z) |
−1 |
Z′ X) |
−1 |
(16.18) |
ΣTSLS = s |
|
, |
where s2 is the estimated residual variance (square of the standard error of the regression).
Estimating TSLS in EViews
To use two-stage least squares, open the equation specification box by choosing Object/New Object.../Equation… or Quick/Estimate Equation…. Choose TSLS from the Method: combo box and the dialog will change to include an edit window where you will list the instruments.
In the Equation specification edit box, specify your dependent variable and independent variables, and in the Instrument list edit box, provide a list of instruments.

Two-stage Least Squares—475
There are a few things to keep in mind as you enter your instruments:
•In order to calculate TSLS estimates, your specification must satisfy the order condition for identification, which says that there must be at least as many instruments as there are coefficients in your equation. There is an additional rank condition which must also be satisfied. See Davidson and MacKinnon (1994) and Johnston and DiNardo (1997) for additional discussion.
•For econometric reasons that we will not pursue here, any right-hand side variables that are not correlated with the disturbances should be included as instruments.
•The constant, C, is always a suitable instrument, so EViews will add it to the instrument list if you omit it.
For example, suppose you are interested in estimating a consumption equation relating consumption (CONS) to gross domestic product (GDP), lagged consumption (CONS(–1)), a trend variable (TIME) and a constant (C). GDP is endogenous and therefore correlated with the residuals. You may, however, believe that government expenditures (G), the log of the money supply (LM), lagged consumption, TIME, and C, are exogenous and uncorrelated with the disturbances, so that these variables may be used as instruments. Your equation specification is then,
cons c gdp cons(-1) time
and the instrument list is:
c gov cons(-1) time lm
This specification satisfies the order condition for identification, which requires that there are at least as many instruments (five) as there are coefficients (four) in the equation specification.
Furthermore, all of the variables in the consumption equation that are believed to be uncorrelated with the disturbances, (CONS(–1), TIME, and C), appear both in the equation specification and in the instrument list. Note that listing C as an instrument is redundant, since EViews automatically adds it to the instrument list.
Output from TSLS
Below, we present TSLS estimates from a regression of LOG(CS) on a constant and LOG(GDP), with the instrument list “C LOG(CS(-1)) LOG(GDP(-1))”:
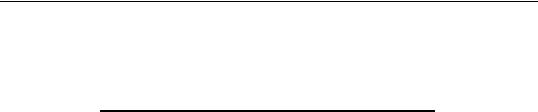
476—Chapter 16. Additional Regression Methods
Dependent Variable: LOG(CS)
Method: Two-Stage Least Squares
Date: 10/15/97 Time: 11:32
Sample(adjusted): 1947:2 1995:1
Included observations: 192 after adjusting endpoints
Instrument list: C LOG(CS(-1)) LOG(GDP(-1))
Variable |
Coefficient |
Std. Error |
t-Statistic |
Prob. |
|
|
|
|
|
C |
-1.209268 |
0.039151 |
-30.88699 |
0.0000 |
LOG(GDP) |
1.094339 |
0.004924 |
222.2597 |
0.0000 |
|
|
|
|
|
R-squared |
0.996168 |
Mean dependent var |
7.480286 |
|
Adjusted R-squared |
0.996148 |
S.D. dependent var |
0.462990 |
|
S.E. of regression |
0.028735 |
Sum squared resid |
0.156888 |
|
F-statistic |
49399.36 |
Durbin-Watson stat |
0.102639 |
|
Prob(F-statistic) |
0.000000 |
|
|
|
|
|
|
|
|
EViews identifies the estimation procedure, as well as the list of instruments in the header. This information is followed by the usual coefficient, t-statistics, and asymptotic p-values.
The summary statistics reported at the bottom of the table are computed using the formulas outlined in “Summary Statistics” on page 451. Bear in mind that all reported statistics are only asymptotically valid. For a discussion of the finite sample properties of TSLS, see Johnston and DiNardo (1997, pp. 355–358) or Davidson and MacKinnon (1984, pp. 221– 224).
EViews uses the structural residuals ut = yt − xt′bTSLS in calculating all of the summary statistics. For example, the standard error of the regression used in the asymptotic
covariance calculation is computed as:
s2 = Σ ut2 ⁄ ( T − k) . |
(16.19) |
t |
|
These structural residuals should be distinguished from the second stage residuals that you would obtain from the second stage regression if you actually computed the two-stage least squares estimates in two separate stages. The second stage residuals are given by
˜ |
ˆ |
ˆ |
ˆ |
ˆ |
are the fitted values from the first-stage regres- |
ut |
= yt − xt′bTSLS , where the |
yt |
and xt |
sions.
We caution you that some of the reported statistics should be interpreted with care. For example, since different equation specifications will have different instrument lists, the reported R2 for TSLS can be negative even when there is a constant in the equation.
Weighted TSLS
You can combine TSLS with weighted regression. Simply enter your TSLS specification as above, then press the Options button, select the Weighted LS/TSLS option, and enter the weighting series.

Two-stage Least Squares—477
Weighted two-stage least squares is performed by multiplying all of the data, including the instruments, by the weight variable, and estimating TSLS on the transformed model. Equivalently, EViews then estimates the coefficients using the formula,
bWTSLS = ( X′W′WZ( Z′W′WZ )−1Z′W′WX)−1 |
|
(16.20) |
||||
|
X′W′ W Z( Z′W′WZ )−1Z′W′Wy |
|
|
|
|
|
The estimated covariance matrix is: |
|
|
|
|
||
ˆ |
2 |
( X′W′WZ( Z′W′ WZ) |
−1 |
Z′W ′WX) |
−1 |
(16.21) |
ΣWTSLS = s |
|
. |
TSLS with AR errors
You can adjust your TSLS estimates to account for serial correlation by adding AR terms to your equation specification. EViews will automatically transform the model to a nonlinear least squares problem, and estimate the model using instrumental variables. Details of this procedure may be found in Fair (1984, pp. 210–214). The output from TSLS with an AR(1) specification looks as follows:
Dependent Variable: LOG(CS)
Method: Two-Stage Least Squares
Date: 10/15/97 Time: 11:42
Sample(adjusted): 1947:2 1995:1
Included observations: 192 after adjusting endpoints
Convergence achieved after 4 iterations
Instrument list: C LOG(CS(-1)) LOG(GDP(-1))
Variable |
Coefficient |
Std. Error |
t-Statistic |
Prob. |
|
|
|
|
|
C |
-1.420705 |
0.203266 |
-6.989390 |
0.0000 |
LOG(GDP) |
1.119858 |
0.025116 |
44.58782 |
0.0000 |
AR(1) |
0.930900 |
0.022267 |
41.80595 |
0.0000 |
|
|
|
|
|
R-squared |
0.999611 |
Mean dependent var |
7.480286 |
|
Adjusted R-squared |
0.999607 |
S.D. dependent var |
0.462990 |
|
S.E. of regression |
0.009175 |
Sum squared resid |
0.015909 |
|
F-statistic |
243139.7 |
Durbin-Watson stat |
1.931027 |
|
Prob(F-statistic) |
0.000000 |
|
|
|
|
|
|
|
|
Inverted AR Roots |
.93 |
|
|
|
|
|
|
|
|
The Options button in the estimation box may be used to change the iteration limit and convergence criterion for the nonlinear instrumental variables procedure.
First-order AR errors
Suppose your specification is:
yt = xt′β + wtγ + ut
(16.22)
ut = ρ1ut − 1 + t

478—Chapter 16. Additional Regression Methods
where xt is a vector of endogenous variables, and wt is a vector of predetermined variables, which, in this context, may include lags of the dependent variable. zt is a vector of instrumental variables not in wt that is large enough to identify the parameters of the model.
In this setting, there are important technical issues to be raised in connection with the choice of instruments. In a widely quoted result, Fair (1970) shows that if the model is estimated using an iterative Cochrane-Orcutt procedure, all of the lagged leftand right-hand side variables (yt − 1, xt − 1, wt − 1) must be included in the instrument list to obtain consistent estimates. In this case, then the instrument list should include:
(wt, zt, yt − 1, xt − 1, wt − 1) . |
(16.23) |
Despite the fact the EViews estimates the model as a nonlinear regression model, the first stage instruments in TSLS are formed as if running Cochrane-Orcutt. Thus, if you choose to omit the lagged leftand right-hand side terms from the instrument list, EViews will automatically add each of the lagged terms as instruments. This fact is noted in your output.
Higher Order AR errors
The AR(1) result extends naturally to specifications involving higher order serial correlation. For example, if you include a single AR(4) term in your model, the natural instrument list will be:
(wt, zt, yt − 4, xt − 4, wt − 4) |
(16.24) |
If you include AR terms from 1 through 4, one possible instrument list is: |
|
(wt, zt, yt − 1, …, yt − 4, xt − 1, …, xt − 4, wt − 1, …, wt − 4) |
(16.25) |
Note that while theoretically valid, this instrument list has a large number of overidentifying instruments, which may lead to computational difficulties and large finite sample biases (Fair (1984, p. 214), Davidson and MacKinnon (1993, pp. 222-224)). In theory, adding instruments should always improve your estimates, but as a practical matter this may not be so in small samples.
Examples
Suppose that you wish to estimate the consumption function by two-stage least squares, allowing for first-order serial correlation. You may then use two-stage least squares with the variable list,
cons c gdp ar(1)
and instrument list:

Two-stage Least Squares—479
c gov log(m1) time cons(-1) gdp(-1)
Notice that the lags of both the dependent and endogenous variables (CONS(–1) and GDP(–1)), are included in the instrument list.
Similarly, consider the consumption function:
cons c cons(-1) gdp ar(1)
A valid instrument list is given by:
c gov log(m1) time cons(-1) cons(-2) gdp(-1)
Here we treat the lagged left and right-hand side variables from the original specification as predetermined and add the lagged values to the instrument list.
Lastly, consider the specification:
cons c gdp ar(1) ar(2) ar(3) ar(4)
Adding all of the relevant instruments in the list, we have:
c gov log(m1) time cons(-1) cons(-2) cons(-3) cons(-4) gdp(-1) gdp(-2) gdp(-3) gdp(-4)
TSLS with MA errors
You can also estimate two-stage least squares variable problems with MA error terms of various orders. To account for the presence of MA errors, simply add the appropriate terms to your specification prior to estimation.
Illustration
Suppose that you wish to estimate the consumption function by two-stage least squares, accounting for first-order moving average errors. You may then use two-stage least squares with the variable list,
cons c gdp ma(1)
and instrument list:
c gov log(m1) time
EViews will add both first and second lags of CONS and GDP to the instrument list.
Technical Details
Most of the technical details are identical to those outlined above for AR errors. EViews transforms the model that is nonlinear in parameters (employing backcasting, if appropriate) and then estimates the model using nonlinear instrumental variables techniques.