
- •Table of Contents
- •What’s New in EViews 5.0
- •What’s New in 5.0
- •Compatibility Notes
- •EViews 5.1 Update Overview
- •Overview of EViews 5.1 New Features
- •Preface
- •Part I. EViews Fundamentals
- •Chapter 1. Introduction
- •What is EViews?
- •Installing and Running EViews
- •Windows Basics
- •The EViews Window
- •Closing EViews
- •Where to Go For Help
- •Chapter 2. A Demonstration
- •Getting Data into EViews
- •Examining the Data
- •Estimating a Regression Model
- •Specification and Hypothesis Tests
- •Modifying the Equation
- •Forecasting from an Estimated Equation
- •Additional Testing
- •Chapter 3. Workfile Basics
- •What is a Workfile?
- •Creating a Workfile
- •The Workfile Window
- •Saving a Workfile
- •Loading a Workfile
- •Multi-page Workfiles
- •Addendum: File Dialog Features
- •Chapter 4. Object Basics
- •What is an Object?
- •Basic Object Operations
- •The Object Window
- •Working with Objects
- •Chapter 5. Basic Data Handling
- •Data Objects
- •Samples
- •Sample Objects
- •Importing Data
- •Exporting Data
- •Frequency Conversion
- •Importing ASCII Text Files
- •Chapter 6. Working with Data
- •Numeric Expressions
- •Series
- •Auto-series
- •Groups
- •Scalars
- •Chapter 7. Working with Data (Advanced)
- •Auto-Updating Series
- •Alpha Series
- •Date Series
- •Value Maps
- •Chapter 8. Series Links
- •Basic Link Concepts
- •Creating a Link
- •Working with Links
- •Chapter 9. Advanced Workfiles
- •Structuring a Workfile
- •Resizing a Workfile
- •Appending to a Workfile
- •Contracting a Workfile
- •Copying from a Workfile
- •Reshaping a Workfile
- •Sorting a Workfile
- •Exporting from a Workfile
- •Chapter 10. EViews Databases
- •Database Overview
- •Database Basics
- •Working with Objects in Databases
- •Database Auto-Series
- •The Database Registry
- •Querying the Database
- •Object Aliases and Illegal Names
- •Maintaining the Database
- •Foreign Format Databases
- •Working with DRIPro Links
- •Part II. Basic Data Analysis
- •Chapter 11. Series
- •Series Views Overview
- •Spreadsheet and Graph Views
- •Descriptive Statistics
- •Tests for Descriptive Stats
- •Distribution Graphs
- •One-Way Tabulation
- •Correlogram
- •Unit Root Test
- •BDS Test
- •Properties
- •Label
- •Series Procs Overview
- •Generate by Equation
- •Resample
- •Seasonal Adjustment
- •Exponential Smoothing
- •Hodrick-Prescott Filter
- •Frequency (Band-Pass) Filter
- •Chapter 12. Groups
- •Group Views Overview
- •Group Members
- •Spreadsheet
- •Dated Data Table
- •Graphs
- •Multiple Graphs
- •Descriptive Statistics
- •Tests of Equality
- •N-Way Tabulation
- •Principal Components
- •Correlations, Covariances, and Correlograms
- •Cross Correlations and Correlograms
- •Cointegration Test
- •Unit Root Test
- •Granger Causality
- •Label
- •Group Procedures Overview
- •Chapter 13. Statistical Graphs from Series and Groups
- •Distribution Graphs of Series
- •Scatter Diagrams with Fit Lines
- •Boxplots
- •Chapter 14. Graphs, Tables, and Text Objects
- •Creating Graphs
- •Modifying Graphs
- •Multiple Graphs
- •Printing Graphs
- •Copying Graphs to the Clipboard
- •Saving Graphs to a File
- •Graph Commands
- •Creating Tables
- •Table Basics
- •Basic Table Customization
- •Customizing Table Cells
- •Copying Tables to the Clipboard
- •Saving Tables to a File
- •Table Commands
- •Text Objects
- •Part III. Basic Single Equation Analysis
- •Chapter 15. Basic Regression
- •Equation Objects
- •Specifying an Equation in EViews
- •Estimating an Equation in EViews
- •Equation Output
- •Working with Equations
- •Estimation Problems
- •Chapter 16. Additional Regression Methods
- •Special Equation Terms
- •Weighted Least Squares
- •Heteroskedasticity and Autocorrelation Consistent Covariances
- •Two-stage Least Squares
- •Nonlinear Least Squares
- •Generalized Method of Moments (GMM)
- •Chapter 17. Time Series Regression
- •Serial Correlation Theory
- •Testing for Serial Correlation
- •Estimating AR Models
- •ARIMA Theory
- •Estimating ARIMA Models
- •ARMA Equation Diagnostics
- •Nonstationary Time Series
- •Unit Root Tests
- •Panel Unit Root Tests
- •Chapter 18. Forecasting from an Equation
- •Forecasting from Equations in EViews
- •An Illustration
- •Forecast Basics
- •Forecasting with ARMA Errors
- •Forecasting from Equations with Expressions
- •Forecasting with Expression and PDL Specifications
- •Chapter 19. Specification and Diagnostic Tests
- •Background
- •Coefficient Tests
- •Residual Tests
- •Specification and Stability Tests
- •Applications
- •Part IV. Advanced Single Equation Analysis
- •Chapter 20. ARCH and GARCH Estimation
- •Basic ARCH Specifications
- •Estimating ARCH Models in EViews
- •Working with ARCH Models
- •Additional ARCH Models
- •Examples
- •Binary Dependent Variable Models
- •Estimating Binary Models in EViews
- •Procedures for Binary Equations
- •Ordered Dependent Variable Models
- •Estimating Ordered Models in EViews
- •Views of Ordered Equations
- •Procedures for Ordered Equations
- •Censored Regression Models
- •Estimating Censored Models in EViews
- •Procedures for Censored Equations
- •Truncated Regression Models
- •Procedures for Truncated Equations
- •Count Models
- •Views of Count Models
- •Procedures for Count Models
- •Demonstrations
- •Technical Notes
- •Chapter 22. The Log Likelihood (LogL) Object
- •Overview
- •Specification
- •Estimation
- •LogL Views
- •LogL Procs
- •Troubleshooting
- •Limitations
- •Examples
- •Part V. Multiple Equation Analysis
- •Chapter 23. System Estimation
- •Background
- •System Estimation Methods
- •How to Create and Specify a System
- •Working With Systems
- •Technical Discussion
- •Vector Autoregressions (VARs)
- •Estimating a VAR in EViews
- •VAR Estimation Output
- •Views and Procs of a VAR
- •Structural (Identified) VARs
- •Cointegration Test
- •Vector Error Correction (VEC) Models
- •A Note on Version Compatibility
- •Chapter 25. State Space Models and the Kalman Filter
- •Background
- •Specifying a State Space Model in EViews
- •Working with the State Space
- •Converting from Version 3 Sspace
- •Technical Discussion
- •Chapter 26. Models
- •Overview
- •An Example Model
- •Building a Model
- •Working with the Model Structure
- •Specifying Scenarios
- •Using Add Factors
- •Solving the Model
- •Working with the Model Data
- •Part VI. Panel and Pooled Data
- •Chapter 27. Pooled Time Series, Cross-Section Data
- •The Pool Workfile
- •The Pool Object
- •Pooled Data
- •Setting up a Pool Workfile
- •Working with Pooled Data
- •Pooled Estimation
- •Chapter 28. Working with Panel Data
- •Structuring a Panel Workfile
- •Panel Workfile Display
- •Panel Workfile Information
- •Working with Panel Data
- •Basic Panel Analysis
- •Chapter 29. Panel Estimation
- •Estimating a Panel Equation
- •Panel Estimation Examples
- •Panel Equation Testing
- •Estimation Background
- •Appendix A. Global Options
- •The Options Menu
- •Print Setup
- •Appendix B. Wildcards
- •Wildcard Expressions
- •Using Wildcard Expressions
- •Source and Destination Patterns
- •Resolving Ambiguities
- •Wildcard versus Pool Identifier
- •Appendix C. Estimation and Solution Options
- •Setting Estimation Options
- •Optimization Algorithms
- •Nonlinear Equation Solution Methods
- •Appendix D. Gradients and Derivatives
- •Gradients
- •Derivatives
- •Appendix E. Information Criteria
- •Definitions
- •Using Information Criteria as a Guide to Model Selection
- •References
- •Index
- •Symbols
- •.DB? files 266
- •.EDB file 262
- •.RTF file 437
- •.WF1 file 62
- •@obsnum
- •Panel
- •@unmaptxt 174
- •~, in backup file name 62, 939
- •Numerics
- •3sls (three-stage least squares) 697, 716
- •Abort key 21
- •ARIMA models 501
- •ASCII
- •file export 115
- •ASCII file
- •See also Unit root tests.
- •Auto-search
- •Auto-series
- •in groups 144
- •Auto-updating series
- •and databases 152
- •Backcast
- •Berndt-Hall-Hall-Hausman (BHHH). See Optimization algorithms.
- •Bias proportion 554
- •fitted index 634
- •Binning option
- •classifications 313, 382
- •Boxplots 409
- •By-group statistics 312, 886, 893
- •coef vector 444
- •Causality
- •Granger's test 389
- •scale factor 649
- •Census X11
- •Census X12 337
- •Chi-square
- •Cholesky factor
- •Classification table
- •Close
- •Coef (coefficient vector)
- •default 444
- •Coefficient
- •Comparison operators
- •Conditional standard deviation
- •graph 610
- •Confidence interval
- •Constant
- •Copy
- •data cut-and-paste 107
- •table to clipboard 437
- •Covariance matrix
- •HAC (Newey-West) 473
- •heteroskedasticity consistent of estimated coefficients 472
- •Create
- •Cross-equation
- •Tukey option 393
- •CUSUM
- •sum of recursive residuals test 589
- •sum of recursive squared residuals test 590
- •Data
- •Database
- •link options 303
- •using auto-updating series with 152
- •Dates
- •Default
- •database 24, 266
- •set directory 71
- •Dependent variable
- •Description
- •Descriptive statistics
- •by group 312
- •group 379
- •individual samples (group) 379
- •Display format
- •Display name
- •Distribution
- •Dummy variables
- •for regression 452
- •lagged dependent variable 495
- •Dynamic forecasting 556
- •Edit
- •See also Unit root tests.
- •Equation
- •create 443
- •store 458
- •Estimation
- •EViews
- •Excel file
- •Excel files
- •Expectation-prediction table
- •Expected dependent variable
- •double 352
- •Export data 114
- •Extreme value
- •binary model 624
- •Fetch
- •File
- •save table to 438
- •Files
- •Fitted index
- •Fitted values
- •Font options
- •Fonts
- •Forecast
- •evaluation 553
- •Foreign data
- •Formula
- •forecast 561
- •Freq
- •DRI database 303
- •F-test
- •for variance equality 321
- •Full information maximum likelihood 698
- •GARCH 601
- •ARCH-M model 603
- •variance factor 668
- •system 716
- •Goodness-of-fit
- •Gradients 963
- •Graph
- •remove elements 423
- •Groups
- •display format 94
- •Groupwise heteroskedasticity 380
- •Help
- •Heteroskedasticity and autocorrelation consistent covariance (HAC) 473
- •History
- •Holt-Winters
- •Hypothesis tests
- •F-test 321
- •Identification
- •Identity
- •Import
- •Import data
- •See also VAR.
- •Index
- •Insert
- •Instruments 474
- •Iteration
- •Iteration option 953
- •in nonlinear least squares 483
- •J-statistic 491
- •J-test 596
- •Kernel
- •bivariate fit 405
- •choice in HAC weighting 704, 718
- •Kernel function
- •Keyboard
- •Kwiatkowski, Phillips, Schmidt, and Shin test 525
- •Label 82
- •Last_update
- •Last_write
- •Latent variable
- •Lead
- •make covariance matrix 643
- •List
- •LM test
- •ARCH 582
- •for binary models 622
- •LOWESS. See also LOESS
- •in ARIMA models 501
- •Mean absolute error 553
- •Metafile
- •Micro TSP
- •recoding 137
- •Models
- •add factors 777, 802
- •solving 804
- •Mouse 18
- •Multicollinearity 460
- •Name
- •Newey-West
- •Nonlinear coefficient restriction
- •Wald test 575
- •weighted two stage 486
- •Normal distribution
- •Numbers
- •chi-square tests 383
- •Object 73
- •Open
- •Option setting
- •Option settings
- •Or operator 98, 133
- •Ordinary residual
- •Panel
- •irregular 214
- •unit root tests 530
- •Paste 83
- •PcGive data 293
- •Polynomial distributed lag
- •Pool
- •Pool (object)
- •PostScript
- •Prediction table
- •Principal components 385
- •Program
- •p-value 569
- •for coefficient t-statistic 450
- •Quiet mode 939
- •RATS data
- •Read 832
- •CUSUM 589
- •Regression
- •Relational operators
- •Remarks
- •database 287
- •Residuals
- •Resize
- •Results
- •RichText Format
- •Robust standard errors
- •Robustness iterations
- •for regression 451
- •with AR specification 500
- •workfile 95
- •Save
- •Seasonal
- •Seasonal graphs 310
- •Select
- •single item 20
- •Serial correlation
- •theory 493
- •Series
- •Smoothing
- •Solve
- •Source
- •Specification test
- •Spreadsheet
- •Standard error
- •Standard error
- •binary models 634
- •Start
- •Starting values
- •Summary statistics
- •for regression variables 451
- •System
- •Table 429
- •font 434
- •Tabulation
- •Template 424
- •Tests. See also Hypothesis tests, Specification test and Goodness of fit.
- •Text file
- •open as workfile 54
- •Type
- •field in database query 282
- •Units
- •Update
- •Valmap
- •find label for value 173
- •find numeric value for label 174
- •Value maps 163
- •estimating 749
- •View
- •Wald test 572
- •nonlinear restriction 575
- •Watson test 323
- •Weighting matrix
- •heteroskedasticity and autocorrelation consistent (HAC) 718
- •kernel options 718
- •White
- •Window
- •Workfile
- •storage defaults 940
- •Write 844
- •XY line
- •Yates' continuity correction 321
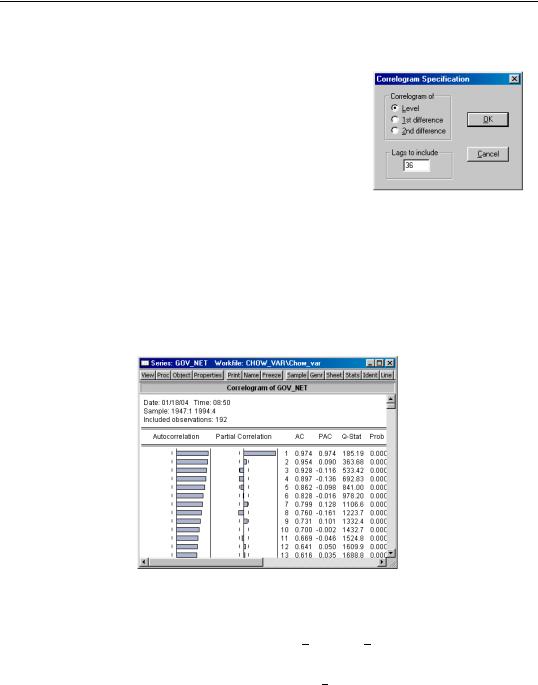
326—Chapter 11. Series
Correlogram
This view displays the autocorrelation and partial autocorrelation functions up to the specified order of lags. These functions characterize the pattern of temporal dependence in the series and typically make sense only for time series data. When you select View/Correlogram… the Correlogram Specification dialog box appears.
You may choose to plot the correlogram of the raw series (level) x, the first difference d(x)=x–x(–1), or the second difference
d(x)-d(x(-1)) = x-2x(-1)+x(-2)
of the series.
You should also specify the highest order of lag to display the correlogram; type in a positive integer in the field box. The series view displays the correlogram and associated statistics:
Autocorrelations (AC)
The autocorrelation of a series Y at lag k is estimated by:
T
Σ ( Yt − Y) ( Yt − k − Y)
τ = t = k + 1
k ---------------------------------------------------------------- (11.15)
T
Σ ( Yt − Y)2
t = 1
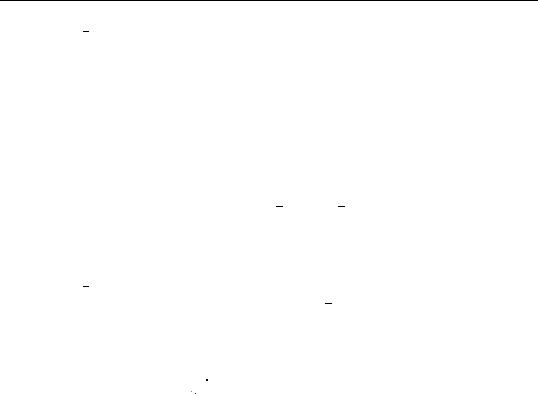
Correlogram—327
where Y is the sample mean of Y . This is the correlation coefficient for values of the series k periods apart. If τ1 is nonzero, it means that the series is first order serially correlated. If τk dies off more or less geometrically with increasing lag k , it is a sign that the series obeys a low-order autoregressive (AR) process. If τk drops to zero after a small number of lags, it is a sign that the series obeys a low-order moving-average (MA) process. See “Serial Correlation Theory” on page 493 for a more complete description of AR and MA processes.
Note that the autocorrelations estimated by EViews differ slightly from theoretical descriptions of the estimator:
T
Σ ( ( Yt − Y )( Yt − k − Y) ) ⁄ ( T − K )
τk = t--------------------------------------------------------------------------------------------= k + 1 |
T |
(11.16) |
||
|
Σ ( Yt − |
|
)2 ⁄ T |
|
|
Y |
|
||
|
t = 1 |
|
where Yt − k = ΣYt − k ⁄ ( T − k) . The difference arises since, for computational simplicity, EViews employs the same overall sample mean Y as the mean of both Yt and Yt − k . While both formulations are consistent estimators, the EViews formulation biases the result toward zero in finite samples.
The dotted lines in the plots of the autocorrelations are the approximate two standard error bounds computed as ±2 ⁄ (T ) . If the autocorrelation is within these bounds, it is not significantly different from zero at (approximately) the 5% significance level.
Partial Autocorrelations (PAC)
The partial autocorrelation at lag k is the regression coefficient on Yt − k when Yt is regressed on a constant, Yt − 1, …, Yt − k . This is a partial correlation since it measures the correlation of Y values that are k periods apart after removing the correlation from the intervening lags. If the pattern of autocorrelation is one that can be captured by an autoregression of order less than k , then the partial autocorrelation at lag k will be close to zero.
The PAC of a pure autoregressive process of order p , AR( p ), cuts off at lag p , while the PAC of a pure moving average (MA) process asymptotes gradually to zero.
EViews estimates the partial autocorrelation at lag k recursively by
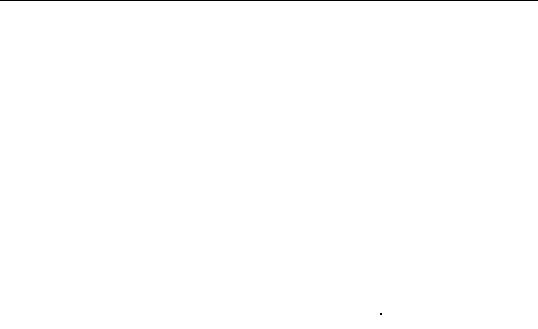
328—Chapter 11. Series
|
|
|
|
τ1 |
|
for k = 1 |
|
|
|
|
|
|
|
||
|
|
|
|
|
k − 1 |
|
|
φ |
|
= |
|
τk − |
Σ φk − 1, j τk − j |
|
(11.17) |
|
k |
|
|
|
j = 1 |
for k > 1 |
|
|
|
|
|
------------------------------------------------- |
|
||
|
|
|
|
|
k − 1 |
|
|
|
|
|
1 − |
Σ φk − 1, j τk − j |
|
|
|
|
|
|
|
|
j = 1 |
|
|
where τk is the estimated autocorrelation at lag k and where, |
|
||||||
|
|
|
φk, j = φk − 1, j − φkφk − 1, k − j. |
(11.18) |
This is a consistent approximation of the partial autocorrelation. The algorithm is described in Box and Jenkins (1976, Part V, Description of computer programs). To obtain a more precise estimate of φ , simply run the regression:
Yt = β0 + β1Yt − 1 + … + βk − 1Yt − (k − 1) + φkYt − k + et |
(11.19) |
where et is a residual. The dotted lines in the plots of the partial autocorrelations are the approximate two standard error bounds computed as ± 2 ⁄ (T ) . If the partial autocorrelation is within these bounds, it is not significantly different from zero at (approximately) the 5% significance level.
Q-Statistics
The last two columns reported in the correlogram are the Ljung-Box Q-statistics and their p-values. The Q-statistic at lag k is a test statistic for the null hypothesis that there is no autocorrelation up to order k and is computed as:
|
k |
2 |
|
|
QLB = T( T + 2 ) |
τj |
|
||
Σ |
(11.20) |
|||
------------ |
||||
|
T − J |
|
||
|
j = 1 |
|
|
where τj is the j-th autocorrelation and T is the number of observations. If the series is not based upon the results of ARIMA estimation, then under the null hypothesis, Q is asymptotically distributed as a χ2 with degrees of freedom equal to the number of autocorrelations. If the series represents the residuals from ARIMA estimation, the appropriate degrees of freedom should be adjusted to represent the number of autocorrelations less the number of AR and MA terms previously estimated. Note also that some care should be taken in interpreting the results of a Ljung-Box test applied to the residuals from an ARMAX specification (see Dezhbaksh, 1990, for simulation evidence on the finite sample performance of the test in this setting).
The Q-statistic is often used as a test of whether the series is white noise. There remains the practical problem of choosing the order of lag to use for the test. If you choose too small a lag, the test may not detect serial correlation at high-order lags. However, if you

Unit Root Test—329
choose too large a lag, the test may have low power since the significant correlation at one lag may be diluted by insignificant correlations at other lags. For further discussion, see Ljung and Box (1979) or Harvey (1990, 1993).
Unit Root Test
This view carries out the Augmented Dickey-Fuller (ADF), GLS transformed Dickey-Fuller (DFGLS), Phillips-Perron (PP), Kwiatkowski, et. al. (KPSS), Elliot, Richardson and Stock (ERS) Point Optimal, and Ng and Perron (NP) unit root tests for whether the series (or it’s first or second difference) is stationary.
See “Nonstationary Time Series” on page 517 for a discussion of stationary and nonstationary time series and additional details on how to carry out the unit roots tests in Eviews.
BDS Test
This view carries out the BDS test for independence, as described in Brock, Dechert, Scheinkman and LeBaron (1996).
The BDS test is a portmanteau test for time based dependence in a series. It can be used for testing against a variety of possible deviations from independence including linear dependence, non-linear dependence, or chaos.
The test can be applied to a series of estimated residuals to check whether the residuals are independent and identically distributed (iid). For example, the residuals from an ARMA model can be tested to see if there is any non-linear dependence in the series after the linear ARMA model has been fitted.
The idea behind the test is fairly simple. To perform the test, we first choose a distance, . We then consider a pair of points. If the observations of the series truly are iid, then for any pair of points, the probability of the distance between these points being less than or equal to epsilon will be constant. We denote this probability by c1( ) .
We can also consider sets consisting of multiple pairs of points. One way we can choose sets of pairs is to move through the consecutive observations of the sample in order. That is, given an observation s , and an observation t of a series X, we can construct a set of pairs of the form:
{{Xs, Xt} , {Xs + 1, Xt + 1} , {Xs + 2, Xt + 2} , …, {Xs + m − 1, Xt + m − 1} } (11.21)
where m is the number of consecutive points used in the set, or embedding dimension. We denote the joint probability of every pair of points in the set satisfying the epsilon condition by the probability cm( ) .
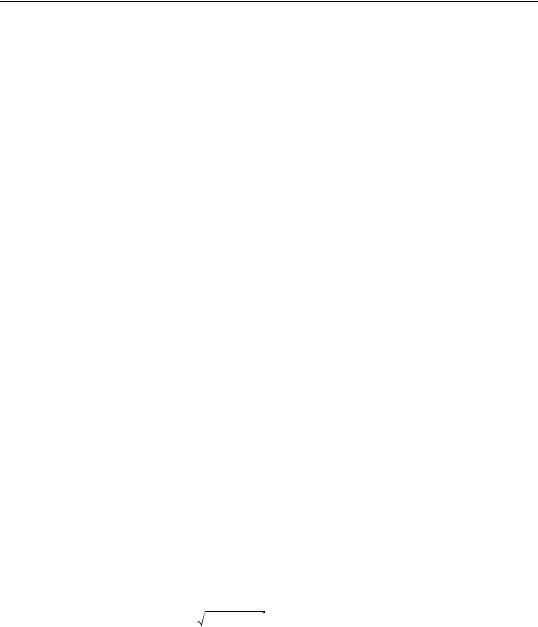
330—Chapter 11. Series
The BDS test proceeds by noting that under the assumption of independence, this probability will simply be the product of the individual probabilities for each pair. That is, if the observations are independent,
cm( ) = c1m( ) . |
(11.22) |
When working with sample data, we do not directly observe c1( ) or cm( ) . We can only estimate them from the sample. As a result, we do not expect this relationship to hold exactly, but only with some error. The larger the error, the less likely it is that the error is caused by random sample variation. The BDS test provides a formal basis for judging the size of this error.
To estimate the probability for a particular dimension, we simply go through all the possible sets of that length that can be drawn from the sample and count the number of sets which satisfy the condition. The ratio of the number of sets satisfying the condition divided by the total number of sets provides the estimate of the probability. Given a sample of n observations of a series X, we can state this condition in mathematical notation,
|
|
2 |
n − m + 1 n − m + 1 m − 1 |
|
|||||
cm, n( ) |
= |
|
Σ |
|
Σ |
|
Π I (Xs + j, Xt + j ) |
(11.23) |
|
(--------------------------------------------------n − m + 1) ( n − m) |
|
|
|||||||
|
|
s = 1 t = s + 1 j = 0 |
|
||||||
|
|
|
|
|
|||||
where I is the indicator function: |
|
|
|
|
|
|
|
||
|
|
|
1 |
if |
|
x − y |
|
≤ |
|
|
|
|
|
|
|||||
|
|
I (x, y ) = |
|
|
(11.24) |
||||
|
|
0 |
otherwise. |
|
Note that the statistics cm, n are often referred to as correlation integrals.
We can then use these sample estimates of the probabilities to construct a test statistic for independence:
b |
( ) = c |
( ) − c |
( )m |
(11.25) |
|
m, n |
m, n |
1, n − m + 1 |
|
where the second term discards the last m − 1 observations from the sample so that it is based on the same number of terms as the first statistic.
Under the assumption of independence, we would expect this statistic to be close to zero. In fact, it is shown in Brock et al. (1996) that
|
|
|
|
( |
|
bm, n( ) |
→ N( 0, 1 ) |
|
|
|
|||||
|
|
|
|
n − m + 1) ------------------ |
|
|
|
||||||||
|
|
|
|
|
|
σm, n( ) |
|
|
|
|
|
|
|||
where |
|
|
|
|
|
|
|
|
|
|
|
|
|
|
|
2 |
|
|
|
m |
m − 1 |
m − j |
|
2j |
|
2 |
|
2m |
2 |
|
2m − 2 |
σm, n( ) |
= |
4 |
k |
|
+ 2 Σ k |
|
c1 |
|
+ ( m − 1) |
c |
1 |
− m |
kc1 |
|
j = 1
(11.26)
(11.27)
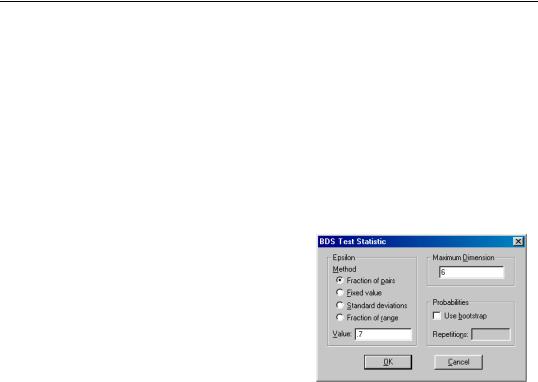
BDS Test—331
and where c1 can be estimated using c1, n . k is the probability of any triplet of points lying within of each other, and is estimated by counting the number of sets satisfying the sample condition:
kn( ) |
|
2 |
n |
n |
n |
|
= |
n--------------------------------------( n − 1 ) -( n − 2) Σ Σ Σ |
(11.28) |
||||
|
|
|
||||
|
|
|
t = 1 s = t + 1 r = s + 1 |
|
( I (Xt, Xs)I (Xs, Xr) + I (Xt, Xr)I (Xr, Xs) + I (Xs, Xt)I (Xt, Xr))
To calculate the BDS test statistic in EViews, simply open the series you would like to test in a window, and choose View/BDS Independence Test.... A dialog will appear prompting you to input options.
To carry out the test, we must choose , the distance used for testing proximity of the data points, and the dimension m , the number of consecutive data points to include in the set.
The dialog provides several choices for how to specify :
•Fraction of pairs: is calculated so as to ensure a certain fraction of the total number of pairs of points in the sample lie within of each other.
•Fixed value: is fixed at a raw value specified in the units as the data series.
•Standard deviations: is calculated as a multiple of the standard deviation of the series.
•Fraction of range: is calculated as a fraction of the range (the difference between the maximum and minimum value) of the series.
The default is to specify as a fraction of pairs, since this method is most invariant to different distributions of the underlying series.
You must also specify the value used in calculating . The meaning of this value varies based on the choice of method. The default value of 0.7 provides a good starting point for the default method when testing shorter dimensions. For testing longer dimensions, you should generally increase the value of to improve the power of the test.
EViews also allows you to specify the maximum correlation dimension for which to calculate the test statistic. EViews will calculate the BDS test statistic for all dimensions from 2 to the specified value, using the same value of or each dimension. Note the same is