
- •Table of Contents
- •What’s New in EViews 5.0
- •What’s New in 5.0
- •Compatibility Notes
- •EViews 5.1 Update Overview
- •Overview of EViews 5.1 New Features
- •Preface
- •Part I. EViews Fundamentals
- •Chapter 1. Introduction
- •What is EViews?
- •Installing and Running EViews
- •Windows Basics
- •The EViews Window
- •Closing EViews
- •Where to Go For Help
- •Chapter 2. A Demonstration
- •Getting Data into EViews
- •Examining the Data
- •Estimating a Regression Model
- •Specification and Hypothesis Tests
- •Modifying the Equation
- •Forecasting from an Estimated Equation
- •Additional Testing
- •Chapter 3. Workfile Basics
- •What is a Workfile?
- •Creating a Workfile
- •The Workfile Window
- •Saving a Workfile
- •Loading a Workfile
- •Multi-page Workfiles
- •Addendum: File Dialog Features
- •Chapter 4. Object Basics
- •What is an Object?
- •Basic Object Operations
- •The Object Window
- •Working with Objects
- •Chapter 5. Basic Data Handling
- •Data Objects
- •Samples
- •Sample Objects
- •Importing Data
- •Exporting Data
- •Frequency Conversion
- •Importing ASCII Text Files
- •Chapter 6. Working with Data
- •Numeric Expressions
- •Series
- •Auto-series
- •Groups
- •Scalars
- •Chapter 7. Working with Data (Advanced)
- •Auto-Updating Series
- •Alpha Series
- •Date Series
- •Value Maps
- •Chapter 8. Series Links
- •Basic Link Concepts
- •Creating a Link
- •Working with Links
- •Chapter 9. Advanced Workfiles
- •Structuring a Workfile
- •Resizing a Workfile
- •Appending to a Workfile
- •Contracting a Workfile
- •Copying from a Workfile
- •Reshaping a Workfile
- •Sorting a Workfile
- •Exporting from a Workfile
- •Chapter 10. EViews Databases
- •Database Overview
- •Database Basics
- •Working with Objects in Databases
- •Database Auto-Series
- •The Database Registry
- •Querying the Database
- •Object Aliases and Illegal Names
- •Maintaining the Database
- •Foreign Format Databases
- •Working with DRIPro Links
- •Part II. Basic Data Analysis
- •Chapter 11. Series
- •Series Views Overview
- •Spreadsheet and Graph Views
- •Descriptive Statistics
- •Tests for Descriptive Stats
- •Distribution Graphs
- •One-Way Tabulation
- •Correlogram
- •Unit Root Test
- •BDS Test
- •Properties
- •Label
- •Series Procs Overview
- •Generate by Equation
- •Resample
- •Seasonal Adjustment
- •Exponential Smoothing
- •Hodrick-Prescott Filter
- •Frequency (Band-Pass) Filter
- •Chapter 12. Groups
- •Group Views Overview
- •Group Members
- •Spreadsheet
- •Dated Data Table
- •Graphs
- •Multiple Graphs
- •Descriptive Statistics
- •Tests of Equality
- •N-Way Tabulation
- •Principal Components
- •Correlations, Covariances, and Correlograms
- •Cross Correlations and Correlograms
- •Cointegration Test
- •Unit Root Test
- •Granger Causality
- •Label
- •Group Procedures Overview
- •Chapter 13. Statistical Graphs from Series and Groups
- •Distribution Graphs of Series
- •Scatter Diagrams with Fit Lines
- •Boxplots
- •Chapter 14. Graphs, Tables, and Text Objects
- •Creating Graphs
- •Modifying Graphs
- •Multiple Graphs
- •Printing Graphs
- •Copying Graphs to the Clipboard
- •Saving Graphs to a File
- •Graph Commands
- •Creating Tables
- •Table Basics
- •Basic Table Customization
- •Customizing Table Cells
- •Copying Tables to the Clipboard
- •Saving Tables to a File
- •Table Commands
- •Text Objects
- •Part III. Basic Single Equation Analysis
- •Chapter 15. Basic Regression
- •Equation Objects
- •Specifying an Equation in EViews
- •Estimating an Equation in EViews
- •Equation Output
- •Working with Equations
- •Estimation Problems
- •Chapter 16. Additional Regression Methods
- •Special Equation Terms
- •Weighted Least Squares
- •Heteroskedasticity and Autocorrelation Consistent Covariances
- •Two-stage Least Squares
- •Nonlinear Least Squares
- •Generalized Method of Moments (GMM)
- •Chapter 17. Time Series Regression
- •Serial Correlation Theory
- •Testing for Serial Correlation
- •Estimating AR Models
- •ARIMA Theory
- •Estimating ARIMA Models
- •ARMA Equation Diagnostics
- •Nonstationary Time Series
- •Unit Root Tests
- •Panel Unit Root Tests
- •Chapter 18. Forecasting from an Equation
- •Forecasting from Equations in EViews
- •An Illustration
- •Forecast Basics
- •Forecasting with ARMA Errors
- •Forecasting from Equations with Expressions
- •Forecasting with Expression and PDL Specifications
- •Chapter 19. Specification and Diagnostic Tests
- •Background
- •Coefficient Tests
- •Residual Tests
- •Specification and Stability Tests
- •Applications
- •Part IV. Advanced Single Equation Analysis
- •Chapter 20. ARCH and GARCH Estimation
- •Basic ARCH Specifications
- •Estimating ARCH Models in EViews
- •Working with ARCH Models
- •Additional ARCH Models
- •Examples
- •Binary Dependent Variable Models
- •Estimating Binary Models in EViews
- •Procedures for Binary Equations
- •Ordered Dependent Variable Models
- •Estimating Ordered Models in EViews
- •Views of Ordered Equations
- •Procedures for Ordered Equations
- •Censored Regression Models
- •Estimating Censored Models in EViews
- •Procedures for Censored Equations
- •Truncated Regression Models
- •Procedures for Truncated Equations
- •Count Models
- •Views of Count Models
- •Procedures for Count Models
- •Demonstrations
- •Technical Notes
- •Chapter 22. The Log Likelihood (LogL) Object
- •Overview
- •Specification
- •Estimation
- •LogL Views
- •LogL Procs
- •Troubleshooting
- •Limitations
- •Examples
- •Part V. Multiple Equation Analysis
- •Chapter 23. System Estimation
- •Background
- •System Estimation Methods
- •How to Create and Specify a System
- •Working With Systems
- •Technical Discussion
- •Vector Autoregressions (VARs)
- •Estimating a VAR in EViews
- •VAR Estimation Output
- •Views and Procs of a VAR
- •Structural (Identified) VARs
- •Cointegration Test
- •Vector Error Correction (VEC) Models
- •A Note on Version Compatibility
- •Chapter 25. State Space Models and the Kalman Filter
- •Background
- •Specifying a State Space Model in EViews
- •Working with the State Space
- •Converting from Version 3 Sspace
- •Technical Discussion
- •Chapter 26. Models
- •Overview
- •An Example Model
- •Building a Model
- •Working with the Model Structure
- •Specifying Scenarios
- •Using Add Factors
- •Solving the Model
- •Working with the Model Data
- •Part VI. Panel and Pooled Data
- •Chapter 27. Pooled Time Series, Cross-Section Data
- •The Pool Workfile
- •The Pool Object
- •Pooled Data
- •Setting up a Pool Workfile
- •Working with Pooled Data
- •Pooled Estimation
- •Chapter 28. Working with Panel Data
- •Structuring a Panel Workfile
- •Panel Workfile Display
- •Panel Workfile Information
- •Working with Panel Data
- •Basic Panel Analysis
- •Chapter 29. Panel Estimation
- •Estimating a Panel Equation
- •Panel Estimation Examples
- •Panel Equation Testing
- •Estimation Background
- •Appendix A. Global Options
- •The Options Menu
- •Print Setup
- •Appendix B. Wildcards
- •Wildcard Expressions
- •Using Wildcard Expressions
- •Source and Destination Patterns
- •Resolving Ambiguities
- •Wildcard versus Pool Identifier
- •Appendix C. Estimation and Solution Options
- •Setting Estimation Options
- •Optimization Algorithms
- •Nonlinear Equation Solution Methods
- •Appendix D. Gradients and Derivatives
- •Gradients
- •Derivatives
- •Appendix E. Information Criteria
- •Definitions
- •Using Information Criteria as a Guide to Model Selection
- •References
- •Index
- •Symbols
- •.DB? files 266
- •.EDB file 262
- •.RTF file 437
- •.WF1 file 62
- •@obsnum
- •Panel
- •@unmaptxt 174
- •~, in backup file name 62, 939
- •Numerics
- •3sls (three-stage least squares) 697, 716
- •Abort key 21
- •ARIMA models 501
- •ASCII
- •file export 115
- •ASCII file
- •See also Unit root tests.
- •Auto-search
- •Auto-series
- •in groups 144
- •Auto-updating series
- •and databases 152
- •Backcast
- •Berndt-Hall-Hall-Hausman (BHHH). See Optimization algorithms.
- •Bias proportion 554
- •fitted index 634
- •Binning option
- •classifications 313, 382
- •Boxplots 409
- •By-group statistics 312, 886, 893
- •coef vector 444
- •Causality
- •Granger's test 389
- •scale factor 649
- •Census X11
- •Census X12 337
- •Chi-square
- •Cholesky factor
- •Classification table
- •Close
- •Coef (coefficient vector)
- •default 444
- •Coefficient
- •Comparison operators
- •Conditional standard deviation
- •graph 610
- •Confidence interval
- •Constant
- •Copy
- •data cut-and-paste 107
- •table to clipboard 437
- •Covariance matrix
- •HAC (Newey-West) 473
- •heteroskedasticity consistent of estimated coefficients 472
- •Create
- •Cross-equation
- •Tukey option 393
- •CUSUM
- •sum of recursive residuals test 589
- •sum of recursive squared residuals test 590
- •Data
- •Database
- •link options 303
- •using auto-updating series with 152
- •Dates
- •Default
- •database 24, 266
- •set directory 71
- •Dependent variable
- •Description
- •Descriptive statistics
- •by group 312
- •group 379
- •individual samples (group) 379
- •Display format
- •Display name
- •Distribution
- •Dummy variables
- •for regression 452
- •lagged dependent variable 495
- •Dynamic forecasting 556
- •Edit
- •See also Unit root tests.
- •Equation
- •create 443
- •store 458
- •Estimation
- •EViews
- •Excel file
- •Excel files
- •Expectation-prediction table
- •Expected dependent variable
- •double 352
- •Export data 114
- •Extreme value
- •binary model 624
- •Fetch
- •File
- •save table to 438
- •Files
- •Fitted index
- •Fitted values
- •Font options
- •Fonts
- •Forecast
- •evaluation 553
- •Foreign data
- •Formula
- •forecast 561
- •Freq
- •DRI database 303
- •F-test
- •for variance equality 321
- •Full information maximum likelihood 698
- •GARCH 601
- •ARCH-M model 603
- •variance factor 668
- •system 716
- •Goodness-of-fit
- •Gradients 963
- •Graph
- •remove elements 423
- •Groups
- •display format 94
- •Groupwise heteroskedasticity 380
- •Help
- •Heteroskedasticity and autocorrelation consistent covariance (HAC) 473
- •History
- •Holt-Winters
- •Hypothesis tests
- •F-test 321
- •Identification
- •Identity
- •Import
- •Import data
- •See also VAR.
- •Index
- •Insert
- •Instruments 474
- •Iteration
- •Iteration option 953
- •in nonlinear least squares 483
- •J-statistic 491
- •J-test 596
- •Kernel
- •bivariate fit 405
- •choice in HAC weighting 704, 718
- •Kernel function
- •Keyboard
- •Kwiatkowski, Phillips, Schmidt, and Shin test 525
- •Label 82
- •Last_update
- •Last_write
- •Latent variable
- •Lead
- •make covariance matrix 643
- •List
- •LM test
- •ARCH 582
- •for binary models 622
- •LOWESS. See also LOESS
- •in ARIMA models 501
- •Mean absolute error 553
- •Metafile
- •Micro TSP
- •recoding 137
- •Models
- •add factors 777, 802
- •solving 804
- •Mouse 18
- •Multicollinearity 460
- •Name
- •Newey-West
- •Nonlinear coefficient restriction
- •Wald test 575
- •weighted two stage 486
- •Normal distribution
- •Numbers
- •chi-square tests 383
- •Object 73
- •Open
- •Option setting
- •Option settings
- •Or operator 98, 133
- •Ordinary residual
- •Panel
- •irregular 214
- •unit root tests 530
- •Paste 83
- •PcGive data 293
- •Polynomial distributed lag
- •Pool
- •Pool (object)
- •PostScript
- •Prediction table
- •Principal components 385
- •Program
- •p-value 569
- •for coefficient t-statistic 450
- •Quiet mode 939
- •RATS data
- •Read 832
- •CUSUM 589
- •Regression
- •Relational operators
- •Remarks
- •database 287
- •Residuals
- •Resize
- •Results
- •RichText Format
- •Robust standard errors
- •Robustness iterations
- •for regression 451
- •with AR specification 500
- •workfile 95
- •Save
- •Seasonal
- •Seasonal graphs 310
- •Select
- •single item 20
- •Serial correlation
- •theory 493
- •Series
- •Smoothing
- •Solve
- •Source
- •Specification test
- •Spreadsheet
- •Standard error
- •Standard error
- •binary models 634
- •Start
- •Starting values
- •Summary statistics
- •for regression variables 451
- •System
- •Table 429
- •font 434
- •Tabulation
- •Template 424
- •Tests. See also Hypothesis tests, Specification test and Goodness of fit.
- •Text file
- •open as workfile 54
- •Type
- •field in database query 282
- •Units
- •Update
- •Valmap
- •find label for value 173
- •find numeric value for label 174
- •Value maps 163
- •estimating 749
- •View
- •Wald test 572
- •nonlinear restriction 575
- •Watson test 323
- •Weighting matrix
- •heteroskedasticity and autocorrelation consistent (HAC) 718
- •kernel options 718
- •White
- •Window
- •Workfile
- •storage defaults 940
- •Write 844
- •XY line
- •Yates' continuity correction 321

Chapter 25. State Space Models and the Kalman Filter
The EViews sspace (state space) object provides a straightforward, easy-to-use interface for specifying, estimating, and working with the results of your single or multiple equation dynamic system. EViews provides a wide range of specification, filtering, smoothing, and other forecasting tools which aid you in working with dynamic systems specified in state space form.
A wide range of time series models, including the classical linear regression model and ARIMA models, can be written and estimated as special cases of a state space specification. State space models have been applied in the econometrics literature to model unobserved variables: (rational) expectations, measurement errors, missing observations, permanent income, unobserved components (cycles and trends), and the non-accelerating rate of unemployment. Extensive surveys of applications of state space models in econometrics can be found in Hamilton (1994a, Chapter 13; 1994b) and Harvey (1989, Chapters 3, 4).
There are two main benefits to representing a dynamic system in state space form. First, the state space allows unobserved variables (known as the state variables) to be incorporated into, and estimated along with, the observable model. Second, state space models can be analyzed using a powerful recursive algorithm known as the Kalman (Bucy) filter. The Kalman filter algorithm has been used, among other things, to compute exact, finite sample forecasts for Gaussian ARMA models, multivariate (vector) ARMA models, MIMIC (multiple indicators and multiple causes), Markov switching models, and time varying (random) coefficient models.
Those of you who have used early versions of the sspace object will note that much was changed with the EViews 4 release. We strongly recommend that you read “Converting from Version 3 Sspace” on page 775 before loading existing workfiles and before beginning to work with the new state space routines.
Background
We present here a very brief discussion of the specification and estimation of a linear state space model. Those desiring greater detail are directed to Harvey (1989), Hamilton (1994a, Chapter 13, 1994b), and especially the excellent treatment of Koopman, Shephard and Doornik (1999).
Specification
A linear state space representation of the dynamics of the n × 1 vector yt is given by the system of equations:
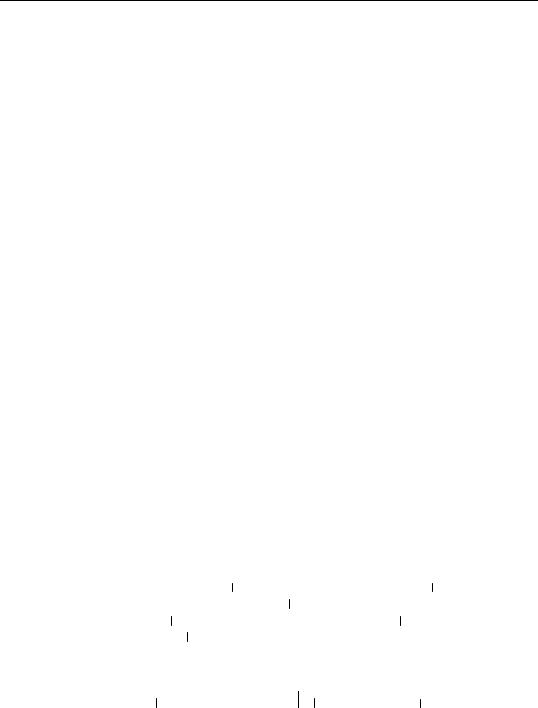
754—Chapter 25. State Space Models and the Kalman Filter
yt = ct + Ztαt + t |
(25.1) |
αt + 1 = dt + Ttαt + vt |
(25.2) |
where αt is an m × 1 vector of possibly unobserved state variables, where ct , Zt , dt and Tt are conformable vectors and matrices, and where t and vt are vectors of mean zero, Gaussian disturbances. Note that the unobserved state vector is assumed to move over time as a first-order vector autoregression.
We will refer to the first set of equations as the “signal” or “observation” equations and the second set as the “state” or “transition” equations. The disturbance vectors t and vt are assumed to be serially independent, with contemporaneous variance structure:
|
|
|
|
|
|
|
|
|
|
Ωt = var |
t |
= |
|
Ht |
Gt |
(25.3) |
|||
|
|
|
Gt′ Qt |
||||||
|
|
vt |
|
|
|||||
|
|
|
|
|
|
|
|
|
|
where Ht is an n × n symmetric variance matrix, Qt |
is an m × m symmetric variance |
||||||||
matrix, and Gt is an n × m matrix of covariances. |
|
|
|
In the discussion that follows, we will generalize the specification given in (25.1)—(25.3) by allowing the system matrices and vectors Ξt ≡ { ct, dt, Zt, Tt, Ht, Qt, Gt} to depend upon observable explanatory variables Xt and unobservable parameters θ . Estimation of the parameters θ is discussed in “Estimation” beginning on page 757.
Filtering
Consider the conditional distribution of the state vector αt given information available at time s . We can define the mean and variance matrix of the conditional distribution as:
|
|
at |
|
s ≡ Es( αt) |
(25.4) |
||||
|
|||||||||
Pt |
|
s ≡ Es[ ( αt − at |
|
s) ( αt − at |
|
s) ′ ] |
(25.5) |
||
|
|
|
where the subscript below the expectation operator indicates that expectations are taken using the conditional distribution for that period.
One important conditional distribution is obtained by setting s = t − 1 , so that we obtain the one-step ahead mean at t − 1 and one-step ahead variance Pt t − 1 of the states
αt . Under the Gaussian error assumption, at t − 1 is also the minimum mean square error estimator of αt and Pt t − 1 is the mean square error (MSE) of at t − 1 . If the normality assumption is dropped, at t − 1 is still the minimum mean square linear estimator of αt .
Given the one-step ahead state conditional mean, we can also form the (linear) minimum MSE one-step ahead estimate of yt :
˜ |
≡ Et − 1( yt) = E( yt at t − 1) = ct + Ztat t − 1 |
(25.6) |
yt = yt t − 1 |
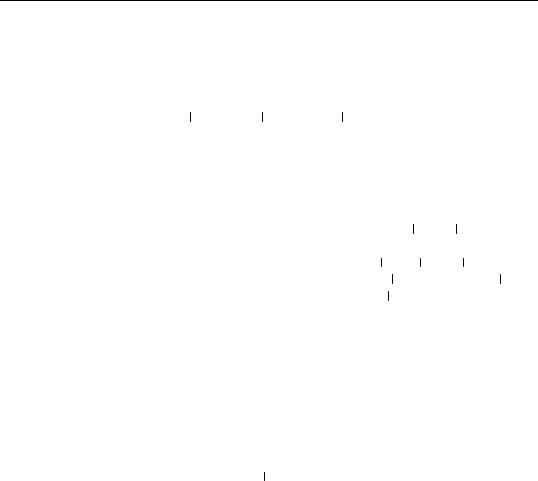
Background—755
The one-step ahead prediction error is given by,
˜ |
= |
t |
˜ |
t − 1 |
(25.7) |
t |
t − 1 ≡ yt − yt |
and the prediction error variance is defined as:
˜ |
≡ var( t t − 1) = ZtPt t − 1Zt′ + Ht |
(25.8) |
Ft = Ft t − 1 |
The Kalman (Bucy) filter is a recursive algorithm for sequentially updating the one-step ahead estimate of the state mean and variance given new information. Details on the recursion are provided in the references above. For our purposes, it is sufficient to note that given initial values for the state mean and covariance, values for the system matrices Ξt , and observations on yt , the Kalman filter may be used to compute one-step ahead estimates of the state and the associated mean square error matrix, {at t − 1, Pt t − 1} , the contemporaneous or filtered state mean and variance, { at, Pt} , and the one-step ahead prediction, prediction error, and prediction error variance, { yt t − 1, t t − 1, Ft t − 1} . Note that we may also obtain the standardized prediction residual, et t − 1 , by dividing t t − 1 by the square-root of the corresponding diagonal element of Ft t − 1 .
Fixed-Interval Smoothing
Suppose that we observe the sequence of data up to time period T . The process of using this information to form expectations at any time period up to T is known as fixed-interval smoothing. Despite the fact that there are a variety of other distinct forms of smoothing (e.g., fixed-point, fixed-lag), we will use the term smoothing to refer to fixed-interval smoothing.
Additional details on the smoothing procedure are provided in the references given above. For now, note that smoothing uses all of the information in the sample to provide
ˆ ≡ ≡ E ( )
smoothed estimates of the states, αt at T T αt , and smoothed estimates of the state
variances, Vt ≡ varT( αt) . The matrix Vt may also be interpreted as the MSE of the
smoothed state estimate ˆ t .
α
As with the one-step ahead states and variances above, we may use the smoothed values to form smoothed estimates of the signal variables,
ˆ |
|
ˆ |
ˆ |
(25.9) |
||
|
||||||
yt ≡ E( yt |
|
αt) |
= ct + Ztαt |
|||
and to compute the variance of the smoothed signal estimates: |
|
|||||
|
ˆ |
|
|
|||
St ≡ var( yt |
|
T) |
= ZtVtZt′ . |
(25.10) |
||
|
Lastly, the smoothing procedure allows us to compute smoothed disturbance estimates,
ˆ |
≡ t |
|
T ≡ ET( t) |
ˆ |
≡ vt |
|
T ≡ ET( vt) , and a corresponding smoothed disturbance |
t |
|
and vt |
|
||||
|
|
variance matrix:

756—Chapter 25. State Space Models and the Kalman Filter
|
|
|
|
|
|
|
ˆ |
= var |
t |
(25.11) |
|||
Ωt |
|
|
|
|
||
|
|
T |
vt |
|
|
|
|
|
|
|
|
|
|
Dividing the smoothed disturbance estimates by the square roots of the corresponding diagonal elements of the smoothed variance matrix yields the standardized smoothed disturbance estimates eˆt and νˆ t .
Forecasting
There are a variety of types of forecasting which may be performed with state space models. These methods differ primarily in what and how information is used. We will focus on the three methods that are supported by EViews built-in forecasting routines.
n-Step Ahead Forecasting
Earlier, we examined the notion of one-step ahead prediction. Consider now the notion of multi-step ahead prediction of observations, in which we take a fixed set of information available at a given period, and forecast several periods ahead. Modifying slightly the expressions in (25.4)—(25.8) yields the n-step ahead state conditional mean and variance:
|
|
|
|
|
at + n |
|
t ≡ Et( αt + n) , |
(25.12) |
|||||||||
|
|||||||||||||||||
Pt + n |
|
|
t ≡ Et[ ( αt + n − at + n |
|
t)( αt + n − at + n |
|
t) ′ ] |
(25.13) |
|||||||||
|
|
|
|||||||||||||||
the n-step ahead forecast, |
|
||||||||||||||||
|
|
|
yt + n |
|
t ≡ Et( yt + n) = ct + Ztat + n |
|
t |
(25.14) |
|||||||||
|
|
|
|
||||||||||||||
and the corresponding n-step ahead forecast MSE matrix: |
|
||||||||||||||||
Ft + n |
|
|
˜ |
t) = Zt + nPt + n |
|
tZt + n′ + Ht |
(25.15) |
||||||||||
t ≡ MSE( yt + n |
|
for n = 1, 2, … . As before, at + n t may also be interpreted as the minimum MSE estimate of αt + n based on the information set available at time t , and Pt + n t is the MSE of the estimate.
It is worth emphasizing that the definitions given above for the forecast MSE matrices do not account for extra variability introduced in the estimation of any unknown
parameters θ . In this setting, the Ft + n t will understate the true variability of the forecast, and should be viewed as being computed conditional on the specific value of the estimated parameters.
It is also worth noting that the n-step ahead forecasts may be computed using a slightly modified version of the basic Kalman recursion (Harvey 1989). To forecast at period
s = t + n , simply initialize a Kalman filter at time t + 1 with the values of the predicted states and state covariances using information at time t , and run the filter forward

Background—757
n − 1 additional periods using no additional signal information. This procedure is repeated for each observation in the forecast sample, s = t + 1, …, t + n .
Dynamic Forecasting
The concept of dynamic forecasting should be familiar to you from other EViews estimation objects. In dynamic forecasting, we start at the beginning of the forecast sample t , and compute a complete set of n-period ahead forecasts for each period n = 1, …, n in the forecast interval. Thus, if we wish to start at period t and forecast dynamically to
t+ n , we would compute a one-step ahead forecast for t + 1 , a two-step ahead forecast for t + 2 , and so forth, up to an n -step ahead forecast for t + n . It may be useful to
note that as with n-step ahead forecasting, we simply initialize a Kalman filter at time
t+ 1 and run the filter forward additional periods using no additional signal information. For dynamic forecasting, however, only one n-step ahead forecast is required to compute all of the forecast values since the information set is not updated from the beginning of the forecast period.
Smoothed Forecasting
Alternatively, we can compute smoothed forecasts which use all available signal data over the forecast sample (for example, at + n t + n ). These forward looking forecasts may be computed by initializing the states at the start of the forecast period, and performing a Kalman smooth over the entire forecast period using all relevant signal data. This technique is useful in settings where information on the entire path of the signals is used to interpolate values throughout the forecast sample.
We make one final comment about the forecasting methods described above. For traditional n-step ahead and dynamic forecasting, the states are typically initialized using the one-step ahead forecasts of the states and variances at the start of the forecast window. For smoothed forecasts, one would generally initialize the forecasts using the corresponding smoothed values of states and variances. There may, however, be situations where you wish to choose a different set of initial values for the forecast filter or smoother. The EViews forecasting routines (described in “State Space Procedures” beginning on
page 771) provide you with considerable control over these initial settings. Be aware, however, that the interpretation of the forecasts in terms of the available information will change if you choose alternative settings.
Estimation
To implement the Kalman filter and the fixed-interval smoother, we must first replace any unknown elements of the system matrices by their estimates. Under the assumption that the t and vt are Gaussian, the sample log likelihood:
|
nT |
1 |
|
|
˜ |
|
1 |
|
˜ |
˜ |
−1˜ |
(25.16) |
|
|
|
|
|
||||||||
log L( θ) |
= − ------log 2π− -- |
Σ |
log |
Ft( θ) |
|
− -- |
Σ |
t′ ( θ)Ft( θ) |
t( θ) |
|||
|
2 |
2 |
|
|
2 |
|
|
|
|
|||
|
|
|
t |
|
|
|
|
t |
|
|
|
|