
- •Brief Contents
- •Contents
- •Preface
- •Who Should Use this Book
- •Philosophy
- •A Short Word on Experiments
- •Acknowledgments
- •Rational Choice Theory and Rational Modeling
- •Rationality and Demand Curves
- •Bounded Rationality and Model Types
- •References
- •Rational Choice with Fixed and Marginal Costs
- •Fixed versus Sunk Costs
- •The Sunk Cost Fallacy
- •Theory and Reactions to Sunk Cost
- •History and Notes
- •Rational Explanations for the Sunk Cost Fallacy
- •Transaction Utility and Flat-Rate Bias
- •Procedural Explanations for Flat-Rate Bias
- •Rational Explanations for Flat-Rate Bias
- •History and Notes
- •Theory and Reference-Dependent Preferences
- •Rational Choice with Income from Varying Sources
- •The Theory of Mental Accounting
- •Budgeting and Consumption Bundles
- •Accounts, Integrating, or Segregating
- •Payment Decoupling, Prepurchase, and Credit Card Purchases
- •Investments and Opening and Closing Accounts
- •Reference Points and Indifference Curves
- •Rational Choice, Temptation and Gifts versus Cash
- •Budgets, Accounts, Temptation, and Gifts
- •Rational Choice over Time
- •References
- •Rational Choice and Default Options
- •Rational Explanations of the Status Quo Bias
- •History and Notes
- •Reference Points, Indifference Curves, and the Consumer Problem
- •An Evolutionary Explanation for Loss Aversion
- •Rational Choice and Getting and Giving Up Goods
- •Loss Aversion and the Endowment Effect
- •Rational Explanations for the Endowment Effect
- •History and Notes
- •Thought Questions
- •Rational Bidding in Auctions
- •Procedural Explanations for Overbidding
- •Levels of Rationality
- •Bidding Heuristics and Transparency
- •Rational Bidding under Dutch and First-Price Auctions
- •History and Notes
- •Rational Prices in English, Dutch, and First-Price Auctions
- •Auction with Uncertainty
- •Rational Bidding under Uncertainty
- •History and Notes
- •References
- •Multiple Rational Choice with Certainty and Uncertainty
- •The Portfolio Problem
- •Narrow versus Broad Bracketing
- •Bracketing the Portfolio Problem
- •More than the Sum of Its Parts
- •The Utility Function and Risk Aversion
- •Bracketing and Variety
- •Rational Bracketing for Variety
- •Changing Preferences, Adding Up, and Choice Bracketing
- •Addiction and Melioration
- •Narrow Bracketing and Motivation
- •Behavioral Bracketing
- •History and Notes
- •Rational Explanations for Bracketing Behavior
- •Statistical Inference and Information
- •Calibration Exercises
- •Representativeness
- •Conjunction Bias
- •The Law of Small Numbers
- •Conservatism versus Representativeness
- •Availability Heuristic
- •Bias, Bigotry, and Availability
- •History and Notes
- •References
- •Rational Information Search
- •Risk Aversion and Production
- •Self-Serving Bias
- •Is Bad Information Bad?
- •History and Notes
- •Thought Questions
- •Rational Decision under Risk
- •Independence and Rational Decision under Risk
- •Allowing Violations of Independence
- •The Shape of Indifference Curves
- •Evidence on the Shape of Probability Weights
- •Probability Weights without Preferences for the Inferior
- •History and Notes
- •Thought Questions
- •Risk Aversion, Risk Loving, and Loss Aversion
- •Prospect Theory
- •Prospect Theory and Indifference Curves
- •Does Prospect Theory Solve the Whole Problem?
- •Prospect Theory and Risk Aversion in Small Gambles
- •History and Notes
- •References
- •The Standard Models of Intertemporal Choice
- •Making Decisions for Our Future Self
- •Projection Bias and Addiction
- •The Role of Emotions and Visceral Factors in Choice
- •Modeling the Hot–Cold Empathy Gap
- •Hindsight Bias and the Curse of Knowledge
- •History and Notes
- •Thought Questions
- •The Fully Additive Model
- •Discounting in Continuous Time
- •Why Would Discounting Be Stable?
- •Naïve Hyperbolic Discounting
- •Naïve Quasi-Hyperbolic Discounting
- •The Common Difference Effect
- •The Absolute Magnitude Effect
- •History and Notes
- •References
- •Rationality and the Possibility of Committing
- •Commitment under Time Inconsistency
- •Choosing When to Do It
- •Of Sophisticates and Naïfs
- •Uncommitting
- •History and Notes
- •Thought Questions
- •Rationality and Altruism
- •Public Goods Provision and Altruistic Behavior
- •History and Notes
- •Thought Questions
- •Inequity Aversion
- •Holding Firms Accountable in a Competitive Marketplace
- •Fairness
- •Kindness Functions
- •Psychological Games
- •History and Notes
- •References
- •Of Trust and Trustworthiness
- •Trust in the Marketplace
- •Trust and Distrust
- •Reciprocity
- •History and Notes
- •References
- •Glossary
- •Index

|
|
|
|
Behavioral Bracketing |
|
149 |
|
furniture. For example, auto dealers often emphasize the amount of the monthly payment rather than the total cost. It sounds much cheaper to buy a car for “only $450 a month” than for “only $37,000.” Old commercials for charitable organizations often suggested that for just the cost of a cup of coffee each day, one could help others in need. Read, Loewenstein, and Rabin mused, “Imagine the response to the plea that your donation will cost ‘only $350 per year, no more than the cost of a small refrigerator.’”
Narrow Bracketing and Motivation
There is evidence of yet another way narrow bracketing can lead to higher overall utility. In some cases, narrow bracketing may be used as a self-control device. On the surface, this seems counterintuitive after a lengthy discussion on how narrow bracketing can lead to addiction and poor behavior. However, we often observe people intentionally using narrow bracketing to achieve very large goals. For example, many addicts try to avoid the object of their addiction “one day at a time.” Similarly the common adage states that great journeys begin with a single step. In both of these examples, the object is to break down a difficult and apparently insurmountable task into very small and doable tasks. It might sound very difficult for addicts to cease their addictive behavior for the next 40 years. Stopping for a day is much more achievable. As well, bracketing too broadly can make it difficult to verify progress on a goal. If one’s goal is to save $5,000 in a year, it may be easier to splurge in the interim because there is so much time left until the goal must be reached. Alternatively, if the goal is to save $210 from every paycheck, one quickly knows if one is on track and whether there is room to splurge and still meet the goal.
Behavioral Bracketing
Read, Loewenstein, and Rabin suggest several potential causes of bracketing and factors that can affect bracketing behavior. Among these are social heuristics, the timing of decisions, limits on cognitive capacity, and motivation. It is easy to see how joint decisions can quickly become so complex as to require someone to narrowly bracket decisions to create any reasoned response. Food consumption is a convenient example. Optimizing would require that a diner take into account all the food decisions she will make together. But, in a day one person makes an average of 200 to 300 food decisions (what to eat, when, where, etc.). It would take too much time and energy to consider these problems together. Thus, it is not particularly clear that one would be better off for having done it.
The rational model does not consider the cognitive costs of such an optimization problem. The timing of decisions makes sequential bracketing natural. We are somewhat forced to think through a decision when it is placed in front of us. Thus, we often make decisions as they arise, bracketing decisions by sequence. Returning to our food example, we would decide on breakfast in the morning, not considering our later meals. Around noon we would be forced to decide what to eat for lunch. Here our decision on breakfast might influence our decision because we are more or less hungry or feel more or less guilty about our level of consumption that morning. But we do not consider dinner at the time we decide on lunch. If the sequence of decisions is one of the primary drivers of bracketing, then our decisions are much more backward looking than forward looking.
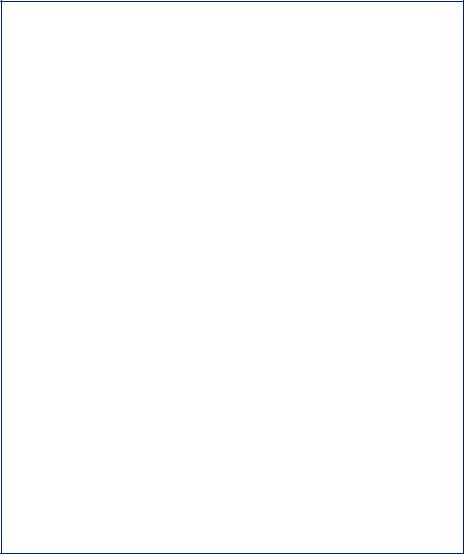
|
|
|
|
|
150 |
|
BRACKETING DECISIONS |
Social heuristics clearly play a role as well. Social heuristics include cases in which society has, by convention, bracketed certain decisions or items together. Thus, we have conventions on the number and timing of meals throughout the day. Such conventions can influence the types of choices we make. For example, Read, Loewenstein, and Rabin find that people indicate a desire to consume more bread pudding when asked separately how much they would eat each weekday and each weekend day than if they were simply asked how much they would eat on each day in a week. Bracketing the weekend separately might induce people to think of this as an opportunity to make some exceptions to their normal routine and consume more of those things they normally try to limit.
History and Notes
The issue of bracketing has lurked in the economics and marketing literatures for quite some time, but the question of bracketing was first raised in a serious fashion by Itamar Simonson regarding the sequencing of choices. Issues such as the peanuts effect and segmenting of decisions were raised in passing by Richard Thaler, Daniel Kahneman, and Dan Lovallo, among others, in proposing mental accounting and other behavioral economic phenomena. Later these concepts were formalized by Daniel Read, George Loewenstein, and Matthew Rabin, whose work much of this chapter is based upon. These models have been very valuable in solving a puzzle in the addiction literature. Addicts display compulsive and binging behavior, but there is also evidence that they respond to monetary incentives to stop the addictive behavior. This is inconsistent with most other psychology-based models of addiction because these models suppose that the behavior ignores the monetary consequences of their actions. Alternatively, financial incentives might allow the narrowly bracketed decision to lead away from addictive substances, thus bringing the melioration optimum in line with the overall optimum.
The general rule would guide the consumer to use broader brackets when it is feasible. This leads to better decisions in nearly all cases. However, there are some clear exceptions, such as when using narrow bracketing to motivate behavior (like quitting an addiction) or if the cognitive decision costs of broad bracketing are overwhelming. The types of bracketing you as a marketer might want to induce depends substantially on the types of products you are selling. Goods that cost substantial amounts up front, but that provide delayed benefits, are more likely to be purchased using broad bracketing. Thus, universities often tout the longterm benefits of being an alumnus and the strength of their alumni organizations. Alternatively, goods that provide substantial benefits now (potentially with greater costs in the future) are better sold using narrow bracketing. Thus, automobiles are sold for their visual appeal, the feel of driving, and other things that can be experienced now.
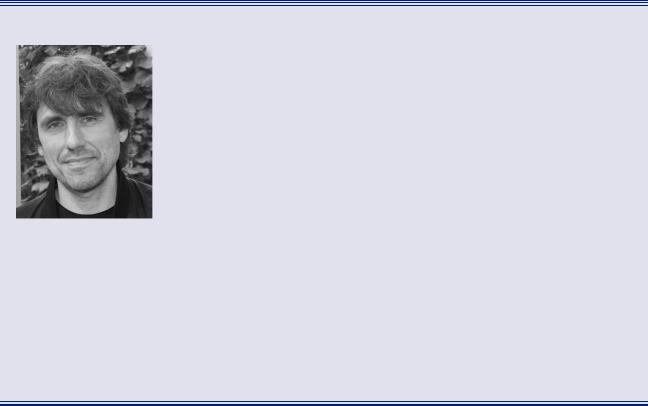
|
|
|
|
Rational Explanations for Bracketing Behavior |
|
151 |
|
Rational Explanations for Bracketing Behavior
A quick note is necessary regarding addictive behavior and rational models. Gary Becker and Kevin Murphy proposed a rational model of addiction in the late 1980s that is often used to inform policy regarding addictive and compulsive behaviors. Their model supposes that addictive goods provide greater and greater utility as one consumes them. Thus, the person who becomes strongly addicted may be optimizing over time by developing their addiction. Such a model, however, requires that addicted people on average would not regret their addiction or change their consumption pattern. This does not appear to be the case. Rather, many addicts wish to end the addiction and lament their having started in the first place. Such regret is consistent with irrational addiction. We have presented one model of addiction in this chapter whereby the addictive substance reduces the value of all activities while increasing the relative utility of the addictive activity. In Part III of this book, another model of irrational addiction is discussed.
Biographical Note
Courtesy of Drazen Prelec
Drazen Prelec (1955–)
B.A., Harvard College, 1978; Ph.D. Harvard
University, 1983; held faculty positions at
Massachusetts Institute of Technology and Harvard
University
Drazen Prelec was trained as an experimental psychologist and an applied mathematician. Combining these skills, his work encompasses mathematical models of human irrationality and the neuroscience of decision. He has made substantial contributions in the behavioral economics of consumer decision making,
risky choice, self-control, and time discounting. His work on neuroscience uses magnetic resonance imaging to map the location of brain activity as people are faced with various types of choices. Experimental work has examined questions such as whether the payment mechanism (cash or credit) influences how much one is willing to spend. His work on self-control has contributed to the science of bracketing and to time discounting. More recent work examines how people may be motivated to obtain indicators of success even if the indicators are not causally related to success. Prelec has published in many of the top economic, business, and psychology journals, holding appointments in all three disciplines. He is the recipient of a prestigious John Simon Guggenheim Fellowship.
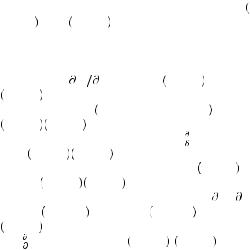
|
|
|
|
|
|
|
|
|
|
|
|
|
|
|
|
|
|
|
|
|
|
|
|
|
|
|
|
|
|
|
|
|
|
152 |
|
|
|
|
|
BRACKETING DECISIONS |
|
|
|
|
|
|
|
|
|
|
|
|
|
|
|
|
|
|
|
|
|||||
|
T H O U G H T Q U E S T I O N S |
|
|
|
|
|
|
|
|
|
|
|
|
|
|
|
|
|
|
|
|
|
|
|||||||||
|
1. |
Financial |
planners and investment advisors often |
|
probability 0.50, and − $100 with 0.50 probability. |
|||||||||||||||||||||||||||
|
|
instruct their clients to hold a broad portfolio of |
|
Suppose that Schuyler’s preferences can be repre- |
||||||||||||||||||||||||||||
|
|
investments to reduce the overall risk. Having a large |
|
sented by the value function in (6.15). Would she |
||||||||||||||||||||||||||||
|
|
number of uncorrelated or negatively correlated |
|
take the gamble? Would she be willing to take four |
||||||||||||||||||||||||||||
|
|
investments in one’s portfolio reduces the variance of |
|
of these gambles? |
|
|
|
|
|
|
|
|||||||||||||||||||||
|
|
the return on investment. At first blush, it might appear |
(b) |
Suppose that Sydney would turn down a single |
||||||||||||||||||||||||||||
|
|
that the advisor is suggesting that investments are more |
||||||||||||||||||||||||||||||
|
|
|
case of this gamble. Consider a gamble that results |
|||||||||||||||||||||||||||||
|
|
attractive |
when |
grouped |
together. Contrast |
this |
with |
|
||||||||||||||||||||||||
|
|
|
in a loss of $600 with probability 0.5 and a gain of |
|||||||||||||||||||||||||||||
|
|
the risk aggregation bias discussed in this chapter. Is |
|
|||||||||||||||||||||||||||||
|
|
|
x with |
|
the |
remaining probability. If |
we |
knew |
||||||||||||||||||||||||
|
|
this diversification a good idea? If the investor were |
|
|
||||||||||||||||||||||||||||
|
|
|
Sydney |
behaved according to |
expected utility, |
|||||||||||||||||||||||||||
|
|
not allowed to diversify, should she still be willing to |
|
|||||||||||||||||||||||||||||
|
|
|
how much would x need to be before he could |
|||||||||||||||||||||||||||||
|
|
buy any single investment in the portfolio? |
|
|
|
|||||||||||||||||||||||||||
|
|
|
|
|
possibly be willing to take the new gamble? Use |
|||||||||||||||||||||||||||
|
|
|
|
|
|
|
|
|
|
|
|
|
|
|
||||||||||||||||||
|
2. |
Expected |
utility theory suggests that all |
risk |
pre- |
|
equation 6.23 to produce your answer. |
|
|
|
||||||||||||||||||||||
|
|
ferences |
are |
due to diminishing marginal |
utility of |
5. Suppose Rosario |
faces |
a two-period time-allocation |
||||||||||||||||||||||||
|
|
wealth. We have briefly discussed some reasons for |
||||||||||||||||||||||||||||||
|
|
problem. Rosario can allocate 10 hours of time in each |
||||||||||||||||||||||||||||||
|
|
doubting |
this hypothesis. |
Why |
might |
diminishing |
||||||||||||||||||||||||||
|
|
period between two activities: work and family. The |
||||||||||||||||||||||||||||||
|
|
marginal |
utility |
of wealth |
be |
related |
to |
risk |
pre- |
|||||||||||||||||||||||
|
|
utility function |
for |
|
the first time |
period |
is u1 x1, |
|||||||||||||||||||||||||
|
|
ferences? |
What |
other explanations for risk |
behavior |
|
||||||||||||||||||||||||||
|
|
10 − x1 |
= x10.5 |
10 − x1 0.5, where x is the amount of |
||||||||||||||||||||||||||||
|
|
can you think of? How would these alternative motives |
||||||||||||||||||||||||||||||
|
|
time spent at work, 10 − x is the amount of time spent |
||||||||||||||||||||||||||||||
|
|
suggest |
behavior that is |
different from |
diminishing |
|||||||||||||||||||||||||||
|
|
with family, |
and the subscript refers to time period. |
|||||||||||||||||||||||||||||
|
|
marginal utility of wealth? |
|
|
|
|
|
|||||||||||||||||||||||||
|
|
|
|
|
|
|
Thus the marginal utility of time at work in the first |
|||||||||||||||||||||||||
|
|
|
|
|
|
|
|
|
|
|
|
|
|
|||||||||||||||||||
|
3. Many small farms sell their vegetable crops through |
period |
is |
|
|
|
u1 |
|
|
x1 = 0.5x1− 0.5 10 − x1 0.5 − 0.5x10.5 |
||||||||||||||||||||||
|
|
cooperative arrangements. A subscriber pays in |
10 − x1 |
− 0.5. In the second period, the utility function |
||||||||||||||||||||||||||||
|
|
advance for a certain portion of the crop. When the |
is |
given |
by |
|
u2 |
x2, 10 − x2, x1, 10 − x1 |
= x1x20.5 + |
|||||||||||||||||||||||
|
|
crop is harvested, the subscriber receives (usually |
10 − x1 |
10 − x2 |
0.5. Marginal utility of time at work |
|||||||||||||||||||||||||||
|
|
weekly) deliveries of produce. The produce is com- |
in the |
second |
|
period |
is given by |
|
u2 |
= 0.5x1x − 0.5 |
||||||||||||||||||||||
|
|
|
|
|
||||||||||||||||||||||||||||
|
|
posed of the particular crops the farmer has decided to |
− 0.5 10 − x1 |
10 − x2 |
|
|
x2 |
|
|
|
2 |
|||||||||||||||||||||
|
|
− 0.5. Thus, total utility for both |
||||||||||||||||||||||||||||||
|
|
grow that year. Consider yourself as a potential sub- |
periods |
is |
|
given |
|
by |
u1 + u2 = |
x10.5 |
10 − x1 |
|
0.5 + |
|||||||||||||||||||
|
|
scriber to this system. You can subscribe for a fixed fee |
x1x20.5 + 10 − x1 |
10 − x2 0.5, resulting |
in |
total |
|
mar- |
||||||||||||||||||||||||
|
|
or you can purchase your vegetables as needed |
ginal utility |
of |
work |
in period |
1 of |
u1 |
|
x1 = |
||||||||||||||||||||||
|
|
throughout the year. Suppose someone considering |
0.5x1− 0.5 10 − x1 |
0.5 − 0.5x10.5 10 − x1 |
− 0.5 + x20.5 − |
|||||||||||||||||||||||||||
|
|
subscribing before the season starts brackets broadly, |
10 − x2 |
0.5, and total marginal utility of work in period |
||||||||||||||||||||||||||||
|
|
|
|
|
|
|
|
|
|
|
|
|
|
|
|
u2 |
|
|
|
|
− 0.5 |
− 0.5 10 − x1 |
10 − x2 |
− 0.5 |
|
|||||||
|
|
and someone purchasing vegetables throughout the |
2 of |
|
|
|
= 0.5x1x2 |
|
|
|
|
. The |
||||||||||||||||||||
|
|
|
x2 |
|
|
|
|
|||||||||||||||||||||||||
|
|
season brackets narrowly. How will bracketing affect |
optimal allocation can be found by setting marginal |
|||||||||||||||||||||||||||||
|
|
the number and types of vegetables purchased? If you |
utility of time at work in each period to zero. If Rosario |
|||||||||||||||||||||||||||||
|
|
were marketing such a subscription, how could you use |
brackets broadly, what will be the optimal allocation of |
|||||||||||||||||||||||||||||
|
|
bracketing to encourage purchases? |
|
|
|
time between work and family in both time periods? |
||||||||||||||||||||||||||
|
4. |
(a) Suppose that Schuyler faces the choice of whether |
How will |
Rosario |
allocate her time if |
she displays |
||||||||||||||||||||||||||
|
melioration? What is the level of utility in each solution? |
|||||||||||||||||||||||||||||||
|
|
to |
take |
a |
gamble |
that results in |
$120 |
with |
||||||||||||||||||||||||
|
|
|
|
|
|
|
|
|
|
|
|
|
|
|
|
|
|
|
|
|
||||||||||||
|
R E F E R E N C E S |
|
|
|
|
|
|
|
|
|
|
|
|
|
|
|
|
|
|
|
|
|
|
|
|
|
||||||
|
Barberis, N., and M. Huang. “Mental Accounting, Loss Aversion, and |
Benartzi, S., and R.H. Thaler. “Myopic Loss Aversion and the Equity |
||||||||||||||||||||||||||||||
|
Individual Stock Returns.” Journal of Finance 56(2001): |
Premium Puzzle.” Quarterly Journal of Economics 110(1995): |
||||||||||||||||||||||||||||||
|
1247–1292. |
|
|
|
|
|
|
|
|
|
|
|
73–92. |
|
|
|
|
|
|
|
|
|
|
|
|
|
|
|
|
|
|
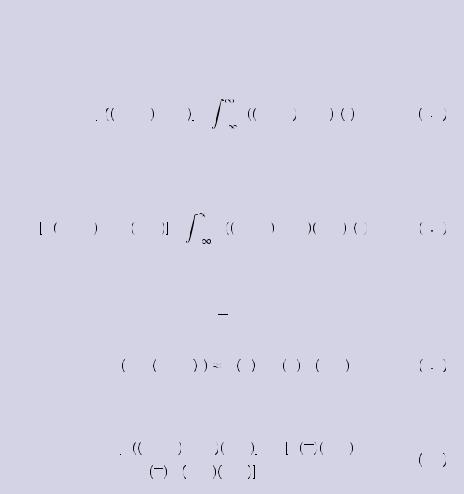
|
|
|
|
References |
|
153 |
|
Camerer, C., L. Babcock, G. Loewenstein, and R. Thaler. “Labor Supply of New York City Cabdrivers: One Day at a Time.”
Quarterly Journal of Economics 112(1997): 407–441.
Hernstein, R.J., G.F. Loewenstein, D. Prelec, and W. Vaughan, Jr.
“Utility Maximization and Melioration: Internalities in Individual Choice.” Journal of Behavioral Decision Making 6(1993): 149–185.
Rabin, M. “Risk Aversion and Expected-Utility Theory: A Calibration Theorem.” Econometrica 68(2000): 1281–1292.
Read, D., and G. Loewenstein. “Diversification Bias: Explaining the Discrepancy in Variety Seeking Between Combined and Separated Choices.” Journal of Experimental Psychology: Applied 1 (1995): 34–49.
Read, D., G. Loewenstein, and M. Rabin. “Choice Bracketing.”
Journal of Risk and Uncertainty 19(1999): 171–197.
Samuelson, P.A. “Risk and Uncertainty: A Fallacy of Large Numbers.” Scientia 98(1963): 108–113.
Simonson, I. “The Effect of Purchase Quantity and Timing on Variety Seeking Behavior.” Journal of Marketing Research 32(1990): 150–162.
Thaler, R.H., A. Tversky, D. Kahneman, and A. Schwartz. “The Effect of Myopia and Loss Aversion on Risk: An Experimental Test.” Quarterly Journal of Economics 112(1997): 647–661.
Tversky, A., and D. Kahneman. “Rational Choice and the Framing of Decisions.” Journal of Business 59(1986): S251–S278.
Advanced Concept
The Portfolio Problem
The portfolio problem can be written as in equation 6.12:
max E u w − wz + wzz = |
u w − wz + wzz f z dz, |
6 A |
wx |
− |
|
|
|
where wz is the amount to be invested in the risky asset, w is the total amount of wealth to be allocated between the two assets, and f z
is the probability density function. The first-order condition can be written as
E u′ w − wz + wzz z − 1 = |
u′ w − wz + wzz z − 1 f z dz = 0. 6 B |
|
− |
It is often useful to use Taylor series approximations to derive results when using expected utility theory. In this case, we can generate a Taylor series approximation around the expected value of the investment portfolio, Ew − wz
+ wzz
=
w − wz
+ wzμ = w. A first-order Taylor series expansion of the marginal utility function results in
u′ wz + w − wz z u′ |
|
+ u″ |
|
wz z − μ . |
6 C |
w |
w |
Using the utility approximation from (6.C), the first-order condition from (6.B) can be written as
E u′ w − wz + wzz z − 1 E u′ w z − 1
6D
+ u″ w wz z − μ z − 1 = 0,
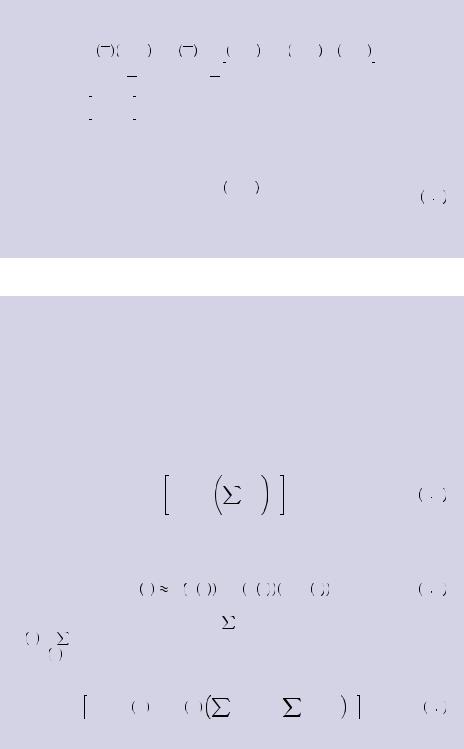
|
|
|
|
|
154 |
|
BRACKETING DECISIONS |
or, completing the square in the second term,
u′ w μ − 1 + u″ w wzE z − μ 2 + μ z − μ − z − μ
6
E
= u′w
μ − 1
+ u″
w
wzσ2 = 0
where σ2 = E z − μ
2 . The value σ2 is commonly called the variance of z, and it measures how dispersed the values of z are. Thus the higher the value of σ2, the more risky is the investment. The result in (6.E) follows because E
z − μ
= μ − μ = 0. Thus, the first-order condition results in
wz = |
μ − 1 |
, |
6 F |
|
|||
|
σ2RA |
|
where RA = − u″u′, is the coefficient of absolute risk aversion.
Advanced Concept
Bracketing the Portfolio Problem
For now, let us suppose that v in equations 6.16 and 6.18 is continuously differentiable. This will allow us to again use a Taylor series expansion to approximate the decision rule and compare behavior with broad and narrow bracketing. Thus, we are omitting the possibility of loss aversion, which involves a function that is kinked at the reference point. equation 6.19 yields first-order conditions given by
|
n |
|
|
E − 1 + v′ |
|
xizi zi = 0. |
6 G |
|
i = |
1 |
|
As before, we will approximate the marginal value function using a first-order
Taylor series expansion around the mean. This yields
|
|
v′ y |
v′ E y + v″ E y y − E y . |
6 H |
||||||
In the case of the portfolio value y = |
n |
|
|
|||||||
i = 1 xizi, the expectation of the return is |
||||||||||
E y = |
n |
is the mean return of |
investment i. We |
will use |
||||||
i = 1 xiμi, where μi |
||||||||||
|
= E y |
in the argument of the value function to signify that this value is held |
||||||||
w |
||||||||||
constant. Thus, we can rewrite the first-order condition in equation (6.G) as |
||||||||||
|
|
E − 1 + v′ |
|
|
|
|
|
n |
n |
|
|
|
|
zi + v″ |
|
|
j = 1 xjzj − |
j = 1 xjμj zi = 0, |
6 I |
||
|
|
w |
w |

|
|
|
|
References |
|
155 |
|
which can be rewritten as
− 1 + v′ |
|
|
|
|
|
|
n |
|
n |
|
|
μi + v″ |
|
E |
j = 1 xj zj − μj |
zi − μi + |
|
zj − μj μi |
|||
w |
w |
j = 1 |
||||||||
|
|
|
|
|
|
|
n |
|
|
6 J |
= − 1 + v′ w μi + v″ |
|
|
= 0. |
|
|
|||||
w |
|
j = 1 xjσij |
|
|
Here, σij = E zi − μi
zj − μj
is the variance of investment i, when i = j, and the covariance of investment i and j when they are unequal. Thus, solving (6.I) yields
|
μi |
|
1 |
− |
j i xjσij |
|
|
|
|
″ |
|
||||
xi = |
+ |
v |
|
. |
6 K |
||
|
|
|
|
||||
|
RAσii |
σii |
|
If instead, we suppose that decisions are narrowly bracketed, equation 6.21 has first-order conditions
E − 1 + v′ xizi zi = 0. |
6 L |
Again we can use the Taylor series approximation from equation 6.H, only now y = xizi, and Ey
= xiμi. Thus, we can rewrite equation 6.K as
E − 1 + v′ |
|
|
zi + v″ |
|
|
xi zi − μi zi = 0. |
6 M |
||||||||
w |
w |
||||||||||||||
Completing the square, we obtain |
|
|
|
|
|
|
|
|
|
||||||
− 1 + v′ |
|
|
μi + v″ |
|
|
|
xiσii = 0, |
6 N |
|||||||
w |
w |
||||||||||||||
or, |
|
|
|
|
|
|
|
|
|
||||||
|
|
|
|
|
μi |
|
1 |
|
|
|
|||||
xi = |
|
+ |
|
v″ |
|
. |
6 O |
||||||||
|
|
|
|
||||||||||||
|
|
|
|
RAσii |
|
|
|
σii |
|
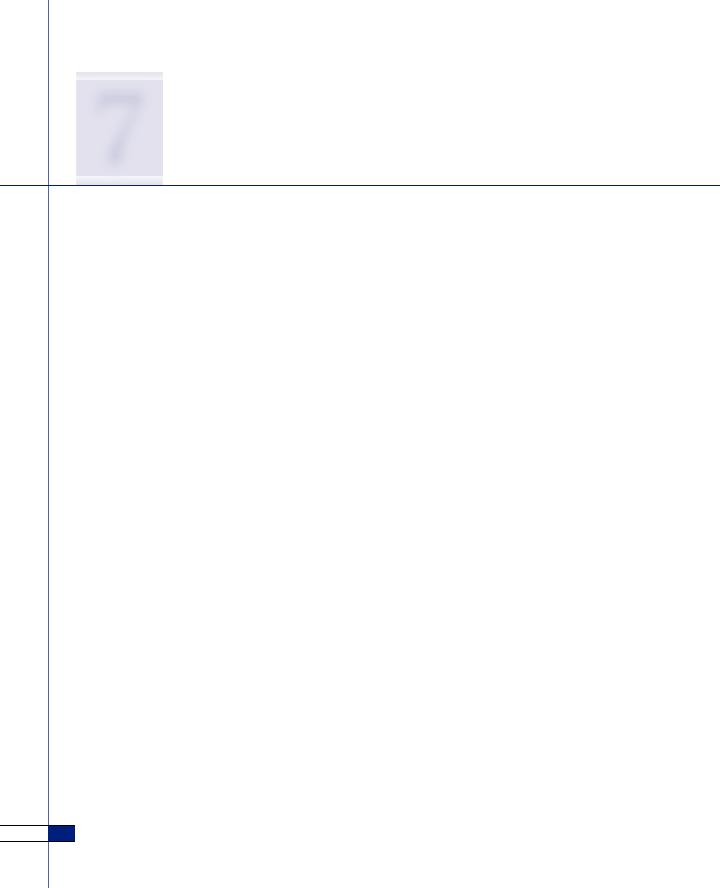
|
|
|
|
|
Representativeness |
|
7 |
|
|
and Availability |
|
|
|
|
|
|
|
Cornell University’s basketball team won the Ivy League championship in 2008, 2009, and 2010. Along the way the team set several team records for three-point shooting. In conversation, the basketball coach was asked about how he decides which players he will use in any particular game. He listed several key factors such as the particular matchups with the opposing team’s players, but he also mentioned the importance of putting in the guy who has been on a shooting streak in the last several games. When pressed, he admitted that there were several factors that could contribute to such a streak but felt that if in a single game a regularly good shooter was cold, he would sit him on the bench in favor of a player with lower average percentage shooting. In fact, many coaches, players, and fans feel they can quickly discern when a player is either on a streak or in a slump in terms of shooting.
In dealing with risky decisions, one must first come to grips with the underlying uncertainty. In other words, before one can make a decision between two choices that involve uncertain outcomes, one must first develop a perception of how uncertain each of the choices is. Thus, a coach deciding which basketball players to put on the court wants to determine how reliable his shooters are and how likely each of his players are to stop key players on the other team from scoring. The coach cannot know before placing a player in a game how that player will perform. But the coach can use previous performance to form beliefs about how the players will perform, thus helping to decide which will play and for how long.
Scientists often find themselves in a similar situation in trying to decide which of several hypotheses may be true. Before conducting experiments, they cannot know with certainty which hypothesis performs best. Often, scientific data are relatively weak, providing little information about the true underlying relationship. In this case it can be difficult to determine the causal effects that might have generated the data. Nonetheless, in order to publish the results, it is necessary to draw some conclusions even from weak data. This chapter deals with how people use available information to form beliefs when facing uncertainty. These beliefs are the basis for decisions regarding investment, hiring, strategy, and virtually every aspect of business management. In reality, we face uncertainty on a constant basis, and given our cognitive limits, we have developed heuristics and other tools to simplify the process of learning and forming perceptions when facing uncertain outcomes. To appreciate the impact of these heuristics on decision outcomes, it is first important to review some basic statistical theory.
156