
- •brief contents
- •contents
- •preface
- •acknowledgments
- •about this book
- •What’s new in the second edition
- •Who should read this book
- •Roadmap
- •Advice for data miners
- •Code examples
- •Code conventions
- •Author Online
- •About the author
- •about the cover illustration
- •1 Introduction to R
- •1.2 Obtaining and installing R
- •1.3 Working with R
- •1.3.1 Getting started
- •1.3.2 Getting help
- •1.3.3 The workspace
- •1.3.4 Input and output
- •1.4 Packages
- •1.4.1 What are packages?
- •1.4.2 Installing a package
- •1.4.3 Loading a package
- •1.4.4 Learning about a package
- •1.5 Batch processing
- •1.6 Using output as input: reusing results
- •1.7 Working with large datasets
- •1.8 Working through an example
- •1.9 Summary
- •2 Creating a dataset
- •2.1 Understanding datasets
- •2.2 Data structures
- •2.2.1 Vectors
- •2.2.2 Matrices
- •2.2.3 Arrays
- •2.2.4 Data frames
- •2.2.5 Factors
- •2.2.6 Lists
- •2.3 Data input
- •2.3.1 Entering data from the keyboard
- •2.3.2 Importing data from a delimited text file
- •2.3.3 Importing data from Excel
- •2.3.4 Importing data from XML
- •2.3.5 Importing data from the web
- •2.3.6 Importing data from SPSS
- •2.3.7 Importing data from SAS
- •2.3.8 Importing data from Stata
- •2.3.9 Importing data from NetCDF
- •2.3.10 Importing data from HDF5
- •2.3.11 Accessing database management systems (DBMSs)
- •2.3.12 Importing data via Stat/Transfer
- •2.4 Annotating datasets
- •2.4.1 Variable labels
- •2.4.2 Value labels
- •2.5 Useful functions for working with data objects
- •2.6 Summary
- •3 Getting started with graphs
- •3.1 Working with graphs
- •3.2 A simple example
- •3.3 Graphical parameters
- •3.3.1 Symbols and lines
- •3.3.2 Colors
- •3.3.3 Text characteristics
- •3.3.4 Graph and margin dimensions
- •3.4 Adding text, customized axes, and legends
- •3.4.1 Titles
- •3.4.2 Axes
- •3.4.3 Reference lines
- •3.4.4 Legend
- •3.4.5 Text annotations
- •3.4.6 Math annotations
- •3.5 Combining graphs
- •3.5.1 Creating a figure arrangement with fine control
- •3.6 Summary
- •4 Basic data management
- •4.1 A working example
- •4.2 Creating new variables
- •4.3 Recoding variables
- •4.4 Renaming variables
- •4.5 Missing values
- •4.5.1 Recoding values to missing
- •4.5.2 Excluding missing values from analyses
- •4.6 Date values
- •4.6.1 Converting dates to character variables
- •4.6.2 Going further
- •4.7 Type conversions
- •4.8 Sorting data
- •4.9 Merging datasets
- •4.9.1 Adding columns to a data frame
- •4.9.2 Adding rows to a data frame
- •4.10 Subsetting datasets
- •4.10.1 Selecting (keeping) variables
- •4.10.2 Excluding (dropping) variables
- •4.10.3 Selecting observations
- •4.10.4 The subset() function
- •4.10.5 Random samples
- •4.11 Using SQL statements to manipulate data frames
- •4.12 Summary
- •5 Advanced data management
- •5.2 Numerical and character functions
- •5.2.1 Mathematical functions
- •5.2.2 Statistical functions
- •5.2.3 Probability functions
- •5.2.4 Character functions
- •5.2.5 Other useful functions
- •5.2.6 Applying functions to matrices and data frames
- •5.3 A solution for the data-management challenge
- •5.4 Control flow
- •5.4.1 Repetition and looping
- •5.4.2 Conditional execution
- •5.5 User-written functions
- •5.6 Aggregation and reshaping
- •5.6.1 Transpose
- •5.6.2 Aggregating data
- •5.6.3 The reshape2 package
- •5.7 Summary
- •6 Basic graphs
- •6.1 Bar plots
- •6.1.1 Simple bar plots
- •6.1.2 Stacked and grouped bar plots
- •6.1.3 Mean bar plots
- •6.1.4 Tweaking bar plots
- •6.1.5 Spinograms
- •6.2 Pie charts
- •6.3 Histograms
- •6.4 Kernel density plots
- •6.5 Box plots
- •6.5.1 Using parallel box plots to compare groups
- •6.5.2 Violin plots
- •6.6 Dot plots
- •6.7 Summary
- •7 Basic statistics
- •7.1 Descriptive statistics
- •7.1.1 A menagerie of methods
- •7.1.2 Even more methods
- •7.1.3 Descriptive statistics by group
- •7.1.4 Additional methods by group
- •7.1.5 Visualizing results
- •7.2 Frequency and contingency tables
- •7.2.1 Generating frequency tables
- •7.2.2 Tests of independence
- •7.2.3 Measures of association
- •7.2.4 Visualizing results
- •7.3 Correlations
- •7.3.1 Types of correlations
- •7.3.2 Testing correlations for significance
- •7.3.3 Visualizing correlations
- •7.4 T-tests
- •7.4.3 When there are more than two groups
- •7.5 Nonparametric tests of group differences
- •7.5.1 Comparing two groups
- •7.5.2 Comparing more than two groups
- •7.6 Visualizing group differences
- •7.7 Summary
- •8 Regression
- •8.1 The many faces of regression
- •8.1.1 Scenarios for using OLS regression
- •8.1.2 What you need to know
- •8.2 OLS regression
- •8.2.1 Fitting regression models with lm()
- •8.2.2 Simple linear regression
- •8.2.3 Polynomial regression
- •8.2.4 Multiple linear regression
- •8.2.5 Multiple linear regression with interactions
- •8.3 Regression diagnostics
- •8.3.1 A typical approach
- •8.3.2 An enhanced approach
- •8.3.3 Global validation of linear model assumption
- •8.3.4 Multicollinearity
- •8.4 Unusual observations
- •8.4.1 Outliers
- •8.4.3 Influential observations
- •8.5 Corrective measures
- •8.5.1 Deleting observations
- •8.5.2 Transforming variables
- •8.5.3 Adding or deleting variables
- •8.5.4 Trying a different approach
- •8.6 Selecting the “best” regression model
- •8.6.1 Comparing models
- •8.6.2 Variable selection
- •8.7 Taking the analysis further
- •8.7.1 Cross-validation
- •8.7.2 Relative importance
- •8.8 Summary
- •9 Analysis of variance
- •9.1 A crash course on terminology
- •9.2 Fitting ANOVA models
- •9.2.1 The aov() function
- •9.2.2 The order of formula terms
- •9.3.1 Multiple comparisons
- •9.3.2 Assessing test assumptions
- •9.4 One-way ANCOVA
- •9.4.1 Assessing test assumptions
- •9.4.2 Visualizing the results
- •9.6 Repeated measures ANOVA
- •9.7 Multivariate analysis of variance (MANOVA)
- •9.7.1 Assessing test assumptions
- •9.7.2 Robust MANOVA
- •9.8 ANOVA as regression
- •9.9 Summary
- •10 Power analysis
- •10.1 A quick review of hypothesis testing
- •10.2 Implementing power analysis with the pwr package
- •10.2.1 t-tests
- •10.2.2 ANOVA
- •10.2.3 Correlations
- •10.2.4 Linear models
- •10.2.5 Tests of proportions
- •10.2.7 Choosing an appropriate effect size in novel situations
- •10.3 Creating power analysis plots
- •10.4 Other packages
- •10.5 Summary
- •11 Intermediate graphs
- •11.1 Scatter plots
- •11.1.3 3D scatter plots
- •11.1.4 Spinning 3D scatter plots
- •11.1.5 Bubble plots
- •11.2 Line charts
- •11.3 Corrgrams
- •11.4 Mosaic plots
- •11.5 Summary
- •12 Resampling statistics and bootstrapping
- •12.1 Permutation tests
- •12.2 Permutation tests with the coin package
- •12.2.2 Independence in contingency tables
- •12.2.3 Independence between numeric variables
- •12.2.5 Going further
- •12.3 Permutation tests with the lmPerm package
- •12.3.1 Simple and polynomial regression
- •12.3.2 Multiple regression
- •12.4 Additional comments on permutation tests
- •12.5 Bootstrapping
- •12.6 Bootstrapping with the boot package
- •12.6.1 Bootstrapping a single statistic
- •12.6.2 Bootstrapping several statistics
- •12.7 Summary
- •13 Generalized linear models
- •13.1 Generalized linear models and the glm() function
- •13.1.1 The glm() function
- •13.1.2 Supporting functions
- •13.1.3 Model fit and regression diagnostics
- •13.2 Logistic regression
- •13.2.1 Interpreting the model parameters
- •13.2.2 Assessing the impact of predictors on the probability of an outcome
- •13.2.3 Overdispersion
- •13.2.4 Extensions
- •13.3 Poisson regression
- •13.3.1 Interpreting the model parameters
- •13.3.2 Overdispersion
- •13.3.3 Extensions
- •13.4 Summary
- •14 Principal components and factor analysis
- •14.1 Principal components and factor analysis in R
- •14.2 Principal components
- •14.2.1 Selecting the number of components to extract
- •14.2.2 Extracting principal components
- •14.2.3 Rotating principal components
- •14.2.4 Obtaining principal components scores
- •14.3 Exploratory factor analysis
- •14.3.1 Deciding how many common factors to extract
- •14.3.2 Extracting common factors
- •14.3.3 Rotating factors
- •14.3.4 Factor scores
- •14.4 Other latent variable models
- •14.5 Summary
- •15 Time series
- •15.1 Creating a time-series object in R
- •15.2 Smoothing and seasonal decomposition
- •15.2.1 Smoothing with simple moving averages
- •15.2.2 Seasonal decomposition
- •15.3 Exponential forecasting models
- •15.3.1 Simple exponential smoothing
- •15.3.3 The ets() function and automated forecasting
- •15.4 ARIMA forecasting models
- •15.4.1 Prerequisite concepts
- •15.4.2 ARMA and ARIMA models
- •15.4.3 Automated ARIMA forecasting
- •15.5 Going further
- •15.6 Summary
- •16 Cluster analysis
- •16.1 Common steps in cluster analysis
- •16.2 Calculating distances
- •16.3 Hierarchical cluster analysis
- •16.4 Partitioning cluster analysis
- •16.4.2 Partitioning around medoids
- •16.5 Avoiding nonexistent clusters
- •16.6 Summary
- •17 Classification
- •17.1 Preparing the data
- •17.2 Logistic regression
- •17.3 Decision trees
- •17.3.1 Classical decision trees
- •17.3.2 Conditional inference trees
- •17.4 Random forests
- •17.5 Support vector machines
- •17.5.1 Tuning an SVM
- •17.6 Choosing a best predictive solution
- •17.7 Using the rattle package for data mining
- •17.8 Summary
- •18 Advanced methods for missing data
- •18.1 Steps in dealing with missing data
- •18.2 Identifying missing values
- •18.3 Exploring missing-values patterns
- •18.3.1 Tabulating missing values
- •18.3.2 Exploring missing data visually
- •18.3.3 Using correlations to explore missing values
- •18.4 Understanding the sources and impact of missing data
- •18.5 Rational approaches for dealing with incomplete data
- •18.6 Complete-case analysis (listwise deletion)
- •18.7 Multiple imputation
- •18.8 Other approaches to missing data
- •18.8.1 Pairwise deletion
- •18.8.2 Simple (nonstochastic) imputation
- •18.9 Summary
- •19 Advanced graphics with ggplot2
- •19.1 The four graphics systems in R
- •19.2 An introduction to the ggplot2 package
- •19.3 Specifying the plot type with geoms
- •19.4 Grouping
- •19.5 Faceting
- •19.6 Adding smoothed lines
- •19.7 Modifying the appearance of ggplot2 graphs
- •19.7.1 Axes
- •19.7.2 Legends
- •19.7.3 Scales
- •19.7.4 Themes
- •19.7.5 Multiple graphs per page
- •19.8 Saving graphs
- •19.9 Summary
- •20 Advanced programming
- •20.1 A review of the language
- •20.1.1 Data types
- •20.1.2 Control structures
- •20.1.3 Creating functions
- •20.2 Working with environments
- •20.3 Object-oriented programming
- •20.3.1 Generic functions
- •20.3.2 Limitations of the S3 model
- •20.4 Writing efficient code
- •20.5 Debugging
- •20.5.1 Common sources of errors
- •20.5.2 Debugging tools
- •20.5.3 Session options that support debugging
- •20.6 Going further
- •20.7 Summary
- •21 Creating a package
- •21.1 Nonparametric analysis and the npar package
- •21.1.1 Comparing groups with the npar package
- •21.2 Developing the package
- •21.2.1 Computing the statistics
- •21.2.2 Printing the results
- •21.2.3 Summarizing the results
- •21.2.4 Plotting the results
- •21.2.5 Adding sample data to the package
- •21.3 Creating the package documentation
- •21.4 Building the package
- •21.5 Going further
- •21.6 Summary
- •22 Creating dynamic reports
- •22.1 A template approach to reports
- •22.2 Creating dynamic reports with R and Markdown
- •22.3 Creating dynamic reports with R and LaTeX
- •22.4 Creating dynamic reports with R and Open Document
- •22.5 Creating dynamic reports with R and Microsoft Word
- •22.6 Summary
- •afterword Into the rabbit hole
- •appendix A Graphical user interfaces
- •appendix B Customizing the startup environment
- •appendix C Exporting data from R
- •Delimited text file
- •Excel spreadsheet
- •Statistical applications
- •appendix D Matrix algebra in R
- •appendix E Packages used in this book
- •appendix F Working with large datasets
- •F.1 Efficient programming
- •F.2 Storing data outside of RAM
- •F.3 Analytic packages for out-of-memory data
- •F.4 Comprehensive solutions for working with enormous datasets
- •appendix G Updating an R installation
- •G.1 Automated installation (Windows only)
- •G.2 Manual installation (Windows and Mac OS X)
- •G.3 Updating an R installation (Linux)
- •references
- •index
- •Symbols
- •Numerics
- •23.1 The lattice package
- •23.2 Conditioning variables
- •23.3 Panel functions
- •23.4 Grouping variables
- •23.5 Graphic parameters
- •23.6 Customizing plot strips
- •23.7 Page arrangement
- •23.8 Going further
312 |
CHAPTER 13 Generalized linear models |
The ability to model a response variable with multiple categories (both ordered and unordered) is an important extension, but it comes at the expense of greater interpretive complexity. Assessing model fit and regression diagnostics in these cases will also be more complex.
In the Affairs example, the number of extramarital contacts was dichotomized into a yes/no response variable because our interest centered on whether respondents had an affair in the past year. If our interest had been centered on magnitude— the number of encounters in the past year—we would have analyzed the count data directly. One popular approach to analyzing count data is Poisson regression, the next topic we’ll address.
13.3 Poisson regression
Poisson regression is useful when you’re predicting an outcome variable representing counts from a set of continuous and/or categorical predictor variables. A comprehensive yet accessible introduction to Poisson regression is provided by Coxe, West, and Aiken (2009).
To illustrate the fitting of a Poisson regression model, along with some issues that can come up in the analysis, we’ll use the Breslow seizure data (Breslow, 1993) provided in the robust package. Specifically, we’ll consider the impact of an antiepileptic drug treatment on the number of seizures occurring over an eight-week period following the initiation of therapy. Be sure to install the robust package before continuing.
Data were collected on the age and number of seizures reported by patients suffering from simple or complex partial seizures during an eight-week period before, and eight-week period after, randomization into a drug or placebo condition. SumY (the number of seizures in the eight-week period post-randomization) is the response variable. Treatment condition (Trt), age in years (Age), and number of seizures reported in the baseline eight-week period (Base) are the predictor variables. The baseline number of seizures and age are included because of their potential effect on the response variable. We're interested in whether or not evidence exists that the drug treatment decreases the number of seizures after accounting for these covariates.
First, let’s look at summary statistics for the dataset:
>data(breslow.dat, package="robust")
>names(breslow.dat)
[1] |
"ID" |
"Y1" |
|
"Y2" |
"Y3" |
"Y4" |
"Base" |
"Age" "Trt" "Ysum" |
[10] |
"sumY" |
"Age10" |
"Base4" |
|
|
|
|
|
> summary(breslow.dat[c(6,7,8,10)]) |
|
|
|
|||||
|
Base |
|
|
Age |
|
Trt |
sumY |
|
Min. |
: |
6.0 |
Min. |
:18.0 |
placebo :28 |
Min. |
: 0.0 |
|
1st |
Qu.: 12.0 |
1st |
Qu.:23.0 |
progabide:31 |
1st Qu.: 11.5 |
|||
Median : 22.0 |
Median :28.0 |
|
|
Median |
: 16.0 |
|||
Mean |
: 31.2 |
Mean |
:28.3 |
|
|
Mean |
: 33.1 |
|
3rd |
Qu.: 41.0 |
3rd |
Qu.:32.0 |
|
|
3rd Qu.: 36.0 |
||
Max. |
:151.0 |
Max. |
:42.0 |
|
|
Max. |
:302.0 |
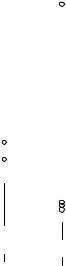
|
|
|
|
|
|
|
|
|
|
|
|
|
|
|
|
|
|
|
|
|
|
|
Poisson regression |
313 |
||||||||||||
|
|
|
Distribution of Seizures |
|
|
|
Group Comparisons |
|||||||||||||||||||||||||||||
|
30 |
|
|
|
|
|
|
|
|
|
|
|
|
|
|
|
|
|
|
|
|
|
300 |
|
|
|
|
|
|
|
|
|
|
|
|
|
|
|
|
|
|
|
|
|
|
|
|
|
|
|
|
|
|
|
|
|
|
|
|
|
|
|
|
|
|
|
|
|
|
|
|
||
|
|
|
|
|
|
|
|
|
|
|
|
|
|
|
|
|
|
|
|
|
|
|
|
|
|
|
|
|
|
|
|
|
|
|
||
|
|
|
|
|
|
|
|
|
|
|
|
|
|
|
|
|
|
|
|
|
|
250 |
|
|
|
|
|
|
|
|
|
|
|
|
|
|
|
|
|
|
|
|
|
|
|
|
|
|
|
|
|
|
|
|
|
|
|
|
|
|
|
|
|
|
|
|
|
|
|
|
|
||
|
25 |
|
|
|
|
|
|
|
|
|
|
|
|
|
|
|
|
|
|
|
|
|
|
|
|
|
|
|
|
|
|
|
|
|
|
|
|
|
|
|
|
|
|
|
|
|
|
|
|
|
|
|
|
|
|
|
|
|
|
|
|
|
|
|
|
|
|
|
|
|
|
||
|
|
|
|
|
|
|
|
|
|
|
|
|
|
|
|
|
|
|
|
|
|
200 |
|
|
|
|
|
|
|
|
|
|
|
|
|
|
|
|
|
|
|
|
|
|
|
|
|
|
|
|
|
|
|
|
|
|
|
|
|
|
|
|
|
|
|
|
|
|
|
|
|
||
Frequency |
20 |
|
|
|
|
|
|
|
|
|
|
|
|
|
|
|
|
|
|
|
|
|
|
|
|
|
|
|
|
|
|
|
|
|
|
|
|
|
|
|
|
|
|
|
|
|
|
|
|
|
|
|
|
|
|
|
|
|
|
|
|
|
|
|
|
|
|
|
|
|
|||
|
|
|
|
|
|
|
|
|
|
|
|
|
|
|
|
|
|
|
|
|
150 |
|
|
|
|
|
|
|
|
|
|
|
|
|
||
|
|
|
|
|
|
|
|
|
|
|
|
|
|
|
|
|
|
|
|
|
|
|
|
|
|
|
|
|
|
|
|
|
|
|||
15 |
|
|
|
|
|
|
|
|
|
|
|
|
|
|
|
|
|
|
|
|
|
|
|
|
|
|
|
|
|
|
|
|
|
|
||
|
|
|
|
|
|
|
|
|
|
|
|
|
|
|
|
|
|
|
|
|
|
|
|
|
|
|
|
|
|
|
|
|
|
|||
|
|
|
|
|
|
|
|
|
|
|
|
|
|
|
|
|
|
|
|
|
100 |
|
|
|
|
|
|
|
|
|
|
|
|
|
||
|
10 |
|
|
|
|
|
|
|
|
|
|
|
|
|
|
|
|
|
|
|
|
|
|
|
|
|
|
|
|
|
|
|
|
|
|
|
|
|
|
|
|
|
|
|
|
|
|
|
|
|
|
|
|
|
|
|
|
|
|
|
|
|
|
|
|
|
|
|
|
|
|
||
|
|
|
|
|
|
|
|
|
|
|
|
|
|
|
|
|
|
|
|
|
|
|
|
|
|
|
|
|
|
|
|
|
|
|
||
|
|
|
|
|
|
|
|
|
|
|
|
|
|
|
|
|
|
|
|
|
|
|
|
|
|
|
|
|
|
|
|
|
||||
|
|
|
|
|
|
|
|
|
|
|
|
|
|
|
|
|
|
|
|
|
|
50 |
|
|
|
|
|
|
|
|
|
|
|
|
|
|
|
5 |
|
|
|
|
|
|
|
|
|
|
|
|
|
|
|
|
|
|
|
|
|
|
|
|
|
|
|
|
|
|
|
|
|
|
|
|
0 |
|
|
|
|
|
|
|
|
|
|
|
|
|
|
|
|
|
|
|
|
|
0 |
|
|
|
|
|
|
|
|
|
|
|
|
|
|
|
|
|
|
|
|
|
|
|
|
|
|
|
|
|
|
|
|
|
|
|
|
|
|
|
|
|
|
|
|
|
|
|
|
||
|
|
|
|
|
|
|
|
|
|
|
|
|
|
|
|
|
|
|
|
|
|
|
|
|
|
|
|
|
|
|
|
|
|
|
||
|
|
|
|
|
|
|
|
|
|
|
|
|
|
|
|
|
|
|
|
|
|
|
|
|
|
|
|
|
|
|
|
|
|
|
||
|
|
|
|
|
|
|
|
|
|
|
|
|
|
|
|
|
|
|
|
|
|
|
|
|
|
|
|
|
|
|
|
|
|
|
|
|
|
|
|
|
|
|
|
|
|
|
|
|
|
|
|
|
|
|
|
|
|
|
|
|
|
|
|
|
|
|
|
|
|
|
|
|
|
|
0 50 |
150 |
250 |
|
|
|
|
|
|
placebo |
|
progabide |
||||||||||||||||||||||||
|
|
|
|
|
|
|
|
|
Seizure Count |
|
|
|
|
|
|
Treatment |
Figure 13.1 Distribution of post-treatment seizure counts (source: Breslow seizure data)
Note that although there are 12 variables in the dataset, we’re limiting our attention to the 4 described earlier. Both the baseline and post-randomization number of seizures are highly skewed. Let’s look at the response variable in more detail. The following code produces the graphs in figure 13.1:
opar <- par(no.readonly=TRUE) par(mfrow=c(1,2)) attach(breslow.dat)
hist(sumY, breaks=20, xlab="Seizure Count", main="Distribution of Seizures")
boxplot(sumY ~ Trt, xlab="Treatment", main="Group Comparisons") par(opar)
You can clearly see the skewed nature of the dependent variable and the possible presence of outliers. At first glance, the number of seizures in the drug condition appears to be smaller and has a smaller variance. (You’d expect a smaller variance to accompany a smaller mean with Poisson distributed data.) Unlike standard OLS regression, this heterogeneity of variance isn’t a problem in Poisson regression.
The next step is to fit the Poisson regression:
>fit <- glm(sumY ~ Base + Age + Trt, data=breslow.dat, family=poisson())
>summary(fit)
314 |
CHAPTER 13 Generalized linear models |
Call:
glm(formula = sumY ~ Base + Age + Trt, family = poisson(), data = breslow.dat)
Deviance Residuals: |
|
|
|
|
||
Min |
1Q |
Median |
3Q |
Max |
|
|
-6.057 -2.043 -0.940 |
0.793 11.006 |
|
|
|||
Coefficients: |
|
|
|
|
|
|
|
|
Estimate Std. Error z value Pr(>|z|) |
|
|||
(Intercept) |
|
1.948826 |
0.135619 |
14.37 |
< 2e-16 *** |
|
Base |
|
0.022652 |
0.000509 |
44.48 |
< 2e-16 |
*** |
Age |
|
0.022740 |
0.004024 |
5.65 |
1.6e-08 |
*** |
Trtprogabide -0.152701 |
0.047805 |
-3.19 |
0.0014 |
** |
||
--- |
|
|
|
|
|
|
Signif. codes: |
0 '***' 0.001 '**' 0.01 '*' 0.05 '.' 0.1 ' ' 1 |
(Dispersion parameter for poisson family taken to be 1)
Null deviance: 2122.73 on 58 degrees of freedom
Residual deviance: 559.44 on 55 degrees of freedom
AIC: 850.7
Number of Fisher Scoring iterations: 5
The output provides the deviances, regression parameters, and standard errors, and tests that these parameters are 0. Note that each of the predictor variables is significant at the p < 0.05 level.
13.3.1Interpreting the model parameters
The model coefficients are obtained using the coef() function or by examining the Coefficients table in the summary() function output:
> coef(fit) |
|
|
|
(Intercept) |
Base |
Age |
Trtprogabide |
1.9488 |
0.0227 |
0.0227 |
-0.1527 |
In a Poisson regression, the dependent variable being modeled is the log of the conditional mean loge(λ). The regression parameter 0.0227 for Age indicates that a oneyear increase in age is associated with a 0.03 increase in the log mean number of seizures, holding baseline seizures and treatment condition constant. The intercept is the log mean number of seizures when each of the predictors equals 0. Because you can’t have a zero age and none of the participants had a zero number of baseline seizures, the intercept isn’t meaningful in this case.
It’s usually much easier to interpret the regression coefficients in the original scale of the dependent variable (number of seizures, rather than log number of seizures). To accomplish this, exponentiate the coefficients:
> exp(coef(fit)) |
|
|
|
(Intercept) |
Base |
Age |
Trtprogabide |
7.020 |
1.023 |
1.023 |
0.858 |
Now you see that a one-year increase in age multiplies the expected number of seizures by 1.023, holding the other variables constant. This means that increased age is
Poisson regression |
315 |
associated with higher numbers of seizures. More important, a one-unit change in Trt (that is, moving from placebo to progabide) multiplies the expected number of seizures by 0.86. You’d expect a 20% decrease in the number of seizures for the drug group compared with the placebo group, holding baseline number of seizures and age constant.
It’s important to remember that, like the exponentiated parameters in logistic regression, the exponentiated parameters in the Poisson model have a multiplicative rather than an additive effect on the response variable. Also, as with logistic regression, you must evaluate your model for overdispersion.
13.3.2Overdispersion
In a Poisson distribution, the variance and mean are equal. Overdispersion occurs in Poisson regression when the observed variance of the response variable is larger than would be predicted by the Poisson distribution. Because overdispersion is often encountered when dealing with count data and can have a negative impact on the interpretation of the results, we’ll spend some time discussing it.
There are several reasons why overdispersion may occur (Coxe et al., 2009):
■The omission of an important predictor variable can lead to overdispersion.
■Overdispersion can also be caused by a phenomenon known as state dependence. Within observations, each event in a count is assumed to be independent. For the seizure data, this would imply that for any patient, the probability of a seizure is independent of each other seizure. But this assumption is often untenable. For a given individual, the probability of having a first seizure is unlikely to be the same as the probability of having a 40th seizure, given that they’ve already had 39.
■In longitudinal studies, overdispersion can be caused by the clustering inherent in repeated measures data. We won’t discuss longitudinal Poisson models here.
If overdispersion is present and you don’t account for it in your model, you’ll get standard errors and confidence intervals that are too small, and significance tests that are too liberal (that is, you’ll find effects that aren’t really there).
As with logistic regression, overdispersion is suggested if the ratio of the residual deviance to the residual degrees of freedom is much larger than 1. For the seizure data, the ratio is
> deviance(fit)/df.residual(fit) [1] 10.17
which is clearly much larger than 1.
The qcc package provides a test for overdispersion in the Poisson case. (Be sure to download and install this package before first use.) You can test for overdispersion in the seizure data using the following code:
>library(qcc)
>qcc.overdispersion.test(breslow.dat$sumY, type="poisson")
316 |
CHAPTER 13 Generalized linear models |
||
Overdispersion test Obs.Var/Theor.Var |
Statistic |
p-value |
|
poisson data |
62.9 |
3646 |
0 |
Not surprisingly, the significance test has a p-value less than 0.05, strongly suggesting the presence of overdispersion.
You can still fit a model to your data using the glm() function, by replacing family="poisson" with family="quasipoisson". Doing so is analogous to the approach to logistic regression when overdispersion is present:
> fit.od <- glm(sumY ~ Base + Age + Trt, data=breslow.dat, family=quasipoisson())
> summary(fit.od)
Call:
glm(formula = sumY ~ Base + Age + Trt, family = quasipoisson(), data = breslow.dat)
Deviance Residuals: |
|
|
|
|
||
Min |
1Q |
Median |
3Q |
Max |
|
|
-6.057 -2.043 -0.940 |
0.793 11.006 |
|
|
|||
Coefficients: |
|
|
|
|
|
|
|
Estimate Std. Error t |
value Pr(>|t|) |
|
|||
(Intercept) |
|
1.94883 |
0.46509 |
4.19 |
0.00010 |
*** |
Base |
|
0.02265 |
0.00175 |
12.97 |
< 2e-16 *** |
|
Age |
|
0.02274 |
0.01380 |
1.65 |
0.10509 |
|
Trtprogabide -0.15270 |
0.16394 |
-0.93 |
0.35570 |
|
||
--- |
|
|
|
|
|
|
Signif. codes: |
0 '***' 0.001 '**' |
0.01 '*' 0.05 '.' 0.1 ' ' 1 |
(Dispersion parameter for quasipoisson family taken to be 11.8)
Null deviance: 2122.73 on 58 degrees of freedom
Residual deviance: 559.44 on 55 degrees of freedom
AIC: NA
Number of Fisher Scoring iterations: 5
Notice that the parameter estimates in the quasi-Poisson approach are identical to those produced by the Poisson approach. The standard errors are much larger, though. In this case, the larger standard errors have led to p-values for Trt (and Age) that are greater than 0.05. When you take overdispersion into account, there’s insufficient evidence to declare that the drug regimen reduces seizure counts more than receiving a placebo, after controlling for baseline seizure rate and age.
Please remember that this example is used for demonstration purposes only. The results shouldn’t be taken to imply anything about the efficacy of progabide in the real world. I’m not a doctor—at least not a medical doctor—and I don’t even play one on TV.
We’ll finish this exploration of Poisson regression with a discussion of some important variants and extensions.