
- •brief contents
- •contents
- •preface
- •acknowledgments
- •about this book
- •What’s new in the second edition
- •Who should read this book
- •Roadmap
- •Advice for data miners
- •Code examples
- •Code conventions
- •Author Online
- •About the author
- •about the cover illustration
- •1 Introduction to R
- •1.2 Obtaining and installing R
- •1.3 Working with R
- •1.3.1 Getting started
- •1.3.2 Getting help
- •1.3.3 The workspace
- •1.3.4 Input and output
- •1.4 Packages
- •1.4.1 What are packages?
- •1.4.2 Installing a package
- •1.4.3 Loading a package
- •1.4.4 Learning about a package
- •1.5 Batch processing
- •1.6 Using output as input: reusing results
- •1.7 Working with large datasets
- •1.8 Working through an example
- •1.9 Summary
- •2 Creating a dataset
- •2.1 Understanding datasets
- •2.2 Data structures
- •2.2.1 Vectors
- •2.2.2 Matrices
- •2.2.3 Arrays
- •2.2.4 Data frames
- •2.2.5 Factors
- •2.2.6 Lists
- •2.3 Data input
- •2.3.1 Entering data from the keyboard
- •2.3.2 Importing data from a delimited text file
- •2.3.3 Importing data from Excel
- •2.3.4 Importing data from XML
- •2.3.5 Importing data from the web
- •2.3.6 Importing data from SPSS
- •2.3.7 Importing data from SAS
- •2.3.8 Importing data from Stata
- •2.3.9 Importing data from NetCDF
- •2.3.10 Importing data from HDF5
- •2.3.11 Accessing database management systems (DBMSs)
- •2.3.12 Importing data via Stat/Transfer
- •2.4 Annotating datasets
- •2.4.1 Variable labels
- •2.4.2 Value labels
- •2.5 Useful functions for working with data objects
- •2.6 Summary
- •3 Getting started with graphs
- •3.1 Working with graphs
- •3.2 A simple example
- •3.3 Graphical parameters
- •3.3.1 Symbols and lines
- •3.3.2 Colors
- •3.3.3 Text characteristics
- •3.3.4 Graph and margin dimensions
- •3.4 Adding text, customized axes, and legends
- •3.4.1 Titles
- •3.4.2 Axes
- •3.4.3 Reference lines
- •3.4.4 Legend
- •3.4.5 Text annotations
- •3.4.6 Math annotations
- •3.5 Combining graphs
- •3.5.1 Creating a figure arrangement with fine control
- •3.6 Summary
- •4 Basic data management
- •4.1 A working example
- •4.2 Creating new variables
- •4.3 Recoding variables
- •4.4 Renaming variables
- •4.5 Missing values
- •4.5.1 Recoding values to missing
- •4.5.2 Excluding missing values from analyses
- •4.6 Date values
- •4.6.1 Converting dates to character variables
- •4.6.2 Going further
- •4.7 Type conversions
- •4.8 Sorting data
- •4.9 Merging datasets
- •4.9.1 Adding columns to a data frame
- •4.9.2 Adding rows to a data frame
- •4.10 Subsetting datasets
- •4.10.1 Selecting (keeping) variables
- •4.10.2 Excluding (dropping) variables
- •4.10.3 Selecting observations
- •4.10.4 The subset() function
- •4.10.5 Random samples
- •4.11 Using SQL statements to manipulate data frames
- •4.12 Summary
- •5 Advanced data management
- •5.2 Numerical and character functions
- •5.2.1 Mathematical functions
- •5.2.2 Statistical functions
- •5.2.3 Probability functions
- •5.2.4 Character functions
- •5.2.5 Other useful functions
- •5.2.6 Applying functions to matrices and data frames
- •5.3 A solution for the data-management challenge
- •5.4 Control flow
- •5.4.1 Repetition and looping
- •5.4.2 Conditional execution
- •5.5 User-written functions
- •5.6 Aggregation and reshaping
- •5.6.1 Transpose
- •5.6.2 Aggregating data
- •5.6.3 The reshape2 package
- •5.7 Summary
- •6 Basic graphs
- •6.1 Bar plots
- •6.1.1 Simple bar plots
- •6.1.2 Stacked and grouped bar plots
- •6.1.3 Mean bar plots
- •6.1.4 Tweaking bar plots
- •6.1.5 Spinograms
- •6.2 Pie charts
- •6.3 Histograms
- •6.4 Kernel density plots
- •6.5 Box plots
- •6.5.1 Using parallel box plots to compare groups
- •6.5.2 Violin plots
- •6.6 Dot plots
- •6.7 Summary
- •7 Basic statistics
- •7.1 Descriptive statistics
- •7.1.1 A menagerie of methods
- •7.1.2 Even more methods
- •7.1.3 Descriptive statistics by group
- •7.1.4 Additional methods by group
- •7.1.5 Visualizing results
- •7.2 Frequency and contingency tables
- •7.2.1 Generating frequency tables
- •7.2.2 Tests of independence
- •7.2.3 Measures of association
- •7.2.4 Visualizing results
- •7.3 Correlations
- •7.3.1 Types of correlations
- •7.3.2 Testing correlations for significance
- •7.3.3 Visualizing correlations
- •7.4 T-tests
- •7.4.3 When there are more than two groups
- •7.5 Nonparametric tests of group differences
- •7.5.1 Comparing two groups
- •7.5.2 Comparing more than two groups
- •7.6 Visualizing group differences
- •7.7 Summary
- •8 Regression
- •8.1 The many faces of regression
- •8.1.1 Scenarios for using OLS regression
- •8.1.2 What you need to know
- •8.2 OLS regression
- •8.2.1 Fitting regression models with lm()
- •8.2.2 Simple linear regression
- •8.2.3 Polynomial regression
- •8.2.4 Multiple linear regression
- •8.2.5 Multiple linear regression with interactions
- •8.3 Regression diagnostics
- •8.3.1 A typical approach
- •8.3.2 An enhanced approach
- •8.3.3 Global validation of linear model assumption
- •8.3.4 Multicollinearity
- •8.4 Unusual observations
- •8.4.1 Outliers
- •8.4.3 Influential observations
- •8.5 Corrective measures
- •8.5.1 Deleting observations
- •8.5.2 Transforming variables
- •8.5.3 Adding or deleting variables
- •8.5.4 Trying a different approach
- •8.6 Selecting the “best” regression model
- •8.6.1 Comparing models
- •8.6.2 Variable selection
- •8.7 Taking the analysis further
- •8.7.1 Cross-validation
- •8.7.2 Relative importance
- •8.8 Summary
- •9 Analysis of variance
- •9.1 A crash course on terminology
- •9.2 Fitting ANOVA models
- •9.2.1 The aov() function
- •9.2.2 The order of formula terms
- •9.3.1 Multiple comparisons
- •9.3.2 Assessing test assumptions
- •9.4 One-way ANCOVA
- •9.4.1 Assessing test assumptions
- •9.4.2 Visualizing the results
- •9.6 Repeated measures ANOVA
- •9.7 Multivariate analysis of variance (MANOVA)
- •9.7.1 Assessing test assumptions
- •9.7.2 Robust MANOVA
- •9.8 ANOVA as regression
- •9.9 Summary
- •10 Power analysis
- •10.1 A quick review of hypothesis testing
- •10.2 Implementing power analysis with the pwr package
- •10.2.1 t-tests
- •10.2.2 ANOVA
- •10.2.3 Correlations
- •10.2.4 Linear models
- •10.2.5 Tests of proportions
- •10.2.7 Choosing an appropriate effect size in novel situations
- •10.3 Creating power analysis plots
- •10.4 Other packages
- •10.5 Summary
- •11 Intermediate graphs
- •11.1 Scatter plots
- •11.1.3 3D scatter plots
- •11.1.4 Spinning 3D scatter plots
- •11.1.5 Bubble plots
- •11.2 Line charts
- •11.3 Corrgrams
- •11.4 Mosaic plots
- •11.5 Summary
- •12 Resampling statistics and bootstrapping
- •12.1 Permutation tests
- •12.2 Permutation tests with the coin package
- •12.2.2 Independence in contingency tables
- •12.2.3 Independence between numeric variables
- •12.2.5 Going further
- •12.3 Permutation tests with the lmPerm package
- •12.3.1 Simple and polynomial regression
- •12.3.2 Multiple regression
- •12.4 Additional comments on permutation tests
- •12.5 Bootstrapping
- •12.6 Bootstrapping with the boot package
- •12.6.1 Bootstrapping a single statistic
- •12.6.2 Bootstrapping several statistics
- •12.7 Summary
- •13 Generalized linear models
- •13.1 Generalized linear models and the glm() function
- •13.1.1 The glm() function
- •13.1.2 Supporting functions
- •13.1.3 Model fit and regression diagnostics
- •13.2 Logistic regression
- •13.2.1 Interpreting the model parameters
- •13.2.2 Assessing the impact of predictors on the probability of an outcome
- •13.2.3 Overdispersion
- •13.2.4 Extensions
- •13.3 Poisson regression
- •13.3.1 Interpreting the model parameters
- •13.3.2 Overdispersion
- •13.3.3 Extensions
- •13.4 Summary
- •14 Principal components and factor analysis
- •14.1 Principal components and factor analysis in R
- •14.2 Principal components
- •14.2.1 Selecting the number of components to extract
- •14.2.2 Extracting principal components
- •14.2.3 Rotating principal components
- •14.2.4 Obtaining principal components scores
- •14.3 Exploratory factor analysis
- •14.3.1 Deciding how many common factors to extract
- •14.3.2 Extracting common factors
- •14.3.3 Rotating factors
- •14.3.4 Factor scores
- •14.4 Other latent variable models
- •14.5 Summary
- •15 Time series
- •15.1 Creating a time-series object in R
- •15.2 Smoothing and seasonal decomposition
- •15.2.1 Smoothing with simple moving averages
- •15.2.2 Seasonal decomposition
- •15.3 Exponential forecasting models
- •15.3.1 Simple exponential smoothing
- •15.3.3 The ets() function and automated forecasting
- •15.4 ARIMA forecasting models
- •15.4.1 Prerequisite concepts
- •15.4.2 ARMA and ARIMA models
- •15.4.3 Automated ARIMA forecasting
- •15.5 Going further
- •15.6 Summary
- •16 Cluster analysis
- •16.1 Common steps in cluster analysis
- •16.2 Calculating distances
- •16.3 Hierarchical cluster analysis
- •16.4 Partitioning cluster analysis
- •16.4.2 Partitioning around medoids
- •16.5 Avoiding nonexistent clusters
- •16.6 Summary
- •17 Classification
- •17.1 Preparing the data
- •17.2 Logistic regression
- •17.3 Decision trees
- •17.3.1 Classical decision trees
- •17.3.2 Conditional inference trees
- •17.4 Random forests
- •17.5 Support vector machines
- •17.5.1 Tuning an SVM
- •17.6 Choosing a best predictive solution
- •17.7 Using the rattle package for data mining
- •17.8 Summary
- •18 Advanced methods for missing data
- •18.1 Steps in dealing with missing data
- •18.2 Identifying missing values
- •18.3 Exploring missing-values patterns
- •18.3.1 Tabulating missing values
- •18.3.2 Exploring missing data visually
- •18.3.3 Using correlations to explore missing values
- •18.4 Understanding the sources and impact of missing data
- •18.5 Rational approaches for dealing with incomplete data
- •18.6 Complete-case analysis (listwise deletion)
- •18.7 Multiple imputation
- •18.8 Other approaches to missing data
- •18.8.1 Pairwise deletion
- •18.8.2 Simple (nonstochastic) imputation
- •18.9 Summary
- •19 Advanced graphics with ggplot2
- •19.1 The four graphics systems in R
- •19.2 An introduction to the ggplot2 package
- •19.3 Specifying the plot type with geoms
- •19.4 Grouping
- •19.5 Faceting
- •19.6 Adding smoothed lines
- •19.7 Modifying the appearance of ggplot2 graphs
- •19.7.1 Axes
- •19.7.2 Legends
- •19.7.3 Scales
- •19.7.4 Themes
- •19.7.5 Multiple graphs per page
- •19.8 Saving graphs
- •19.9 Summary
- •20 Advanced programming
- •20.1 A review of the language
- •20.1.1 Data types
- •20.1.2 Control structures
- •20.1.3 Creating functions
- •20.2 Working with environments
- •20.3 Object-oriented programming
- •20.3.1 Generic functions
- •20.3.2 Limitations of the S3 model
- •20.4 Writing efficient code
- •20.5 Debugging
- •20.5.1 Common sources of errors
- •20.5.2 Debugging tools
- •20.5.3 Session options that support debugging
- •20.6 Going further
- •20.7 Summary
- •21 Creating a package
- •21.1 Nonparametric analysis and the npar package
- •21.1.1 Comparing groups with the npar package
- •21.2 Developing the package
- •21.2.1 Computing the statistics
- •21.2.2 Printing the results
- •21.2.3 Summarizing the results
- •21.2.4 Plotting the results
- •21.2.5 Adding sample data to the package
- •21.3 Creating the package documentation
- •21.4 Building the package
- •21.5 Going further
- •21.6 Summary
- •22 Creating dynamic reports
- •22.1 A template approach to reports
- •22.2 Creating dynamic reports with R and Markdown
- •22.3 Creating dynamic reports with R and LaTeX
- •22.4 Creating dynamic reports with R and Open Document
- •22.5 Creating dynamic reports with R and Microsoft Word
- •22.6 Summary
- •afterword Into the rabbit hole
- •appendix A Graphical user interfaces
- •appendix B Customizing the startup environment
- •appendix C Exporting data from R
- •Delimited text file
- •Excel spreadsheet
- •Statistical applications
- •appendix D Matrix algebra in R
- •appendix E Packages used in this book
- •appendix F Working with large datasets
- •F.1 Efficient programming
- •F.2 Storing data outside of RAM
- •F.3 Analytic packages for out-of-memory data
- •F.4 Comprehensive solutions for working with enormous datasets
- •appendix G Updating an R installation
- •G.1 Automated installation (Windows only)
- •G.2 Manual installation (Windows and Mac OS X)
- •G.3 Updating an R installation (Linux)
- •references
- •index
- •Symbols
- •Numerics
- •23.1 The lattice package
- •23.2 Conditioning variables
- •23.3 Panel functions
- •23.4 Grouping variables
- •23.5 Graphic parameters
- •23.6 Customizing plot strips
- •23.7 Page arrangement
- •23.8 Going further
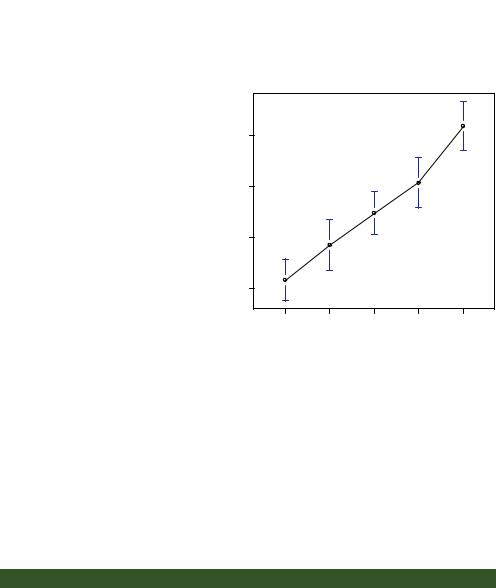
One-way ANOVA |
219 |
|
Signif. codes: 0 '***' 0.001 '**' 0.01 '*' 0.05 '.' 0.1 ' ' 1 |
|
|
> library(gplots) |
|
f Plots group means |
|
||
> plotmeans(response ~ trt, xlab="Treatment", ylab="Response", |
|
and confidence |
main="Mean Plot\nwith 95% CI") |
|
intervals |
> detach(cholesterol) |
|
|
Looking at the output, you can |
|
|
Mean Plot with 95% CI |
|
||||||
see that 10 patients received each |
|
|
|
|||||||
|
|
|
|
|
|
|||||
of the drug regimens b. From |
|
|
|
|
|
|
||||
the means, it appears that drugE |
|
20 |
|
|
|
|
||||
produced the greatest cholesterol |
|
|
|
|
|
|||||
|
|
|
|
|
|
|||||
reduction, whereas 1time pro- |
|
|
|
|
|
|
||||
duced the least c. Standard devi- |
Response |
15 |
|
|
|
|
||||
ations |
were |
relatively |
constant |
|
|
|
|
|||
across the five groups, ranging |
|
|
|
|
||||||
from 2.88 to 3.48 d. The ANOVA |
|
10 |
|
|
|
|
||||
F test for treatment (trt) is signifi- |
|
|
|
|
|
|||||
|
|
|
|
|
|
|||||
cant (p < .0001), providing evi- |
|
|
|
|
|
|
||||
dence that the five treatments |
|
5 |
|
|
|
|
||||
aren’t all equally effective e. |
|
n=10 |
n=10 |
n=10 |
n=10 |
n=10 |
||||
The plotmeans() function in |
|
1time |
2times |
4times |
drugD |
drugE |
||||
the gplots package can be used |
|
|
|
Treatment |
|
|
||||
to produce a graph of group |
Figure 9.1 Treatment group means with 95% confidence |
|||||||||
means |
and |
their |
confidence |
intervals for five cholesterol-reducing drug regimens |
||||||
intervals f. A plot of the treat- |
|
|
|
|
|
|
||||
ment means, with 95% confidence limits, is provided in figure 9.1 and allows you to |
||||||||||
clearly see these treatment differences. |
|
|
|
|
|
9.3.1Multiple comparisons
The ANOVA F test for treatment tells you that the five drug regimens aren’t equally effective, but it doesn’t tell you which treatments differ from one another. You can use a multiple comparison procedure to answer this question. For example, the TukeyHSD() function provides a test of all pairwise differences between group means, as shown next.
Listing 9.2 Tukey HSD pairwise group comparisons
>TukeyHSD(fit)
Tukey multiple comparisons of means 95% family-wise confidence level
Fit: aov(formula = response ~ trt)
$trt
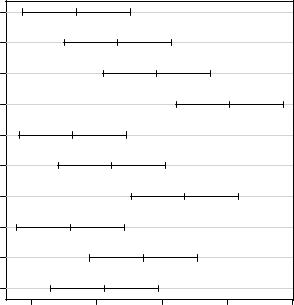
220 |
|
CHAPTER 9 |
Analysis of variance |
|
|
diff |
lwr |
upr |
p adj |
2times-1time |
3.44 |
-0.658 |
7.54 |
0.138 |
4times-1time |
6.59 |
2.492 |
10.69 |
0.000 |
drugD-1time |
9.58 |
5.478 |
13.68 |
0.000 |
drugE-1time |
15.17 |
11.064 |
19.27 |
0.000 |
4times-2times |
3.15 |
-0.951 |
7.25 |
0.205 |
drugD-2times |
6.14 |
2.035 |
10.24 |
0.001 |
drugE-2times |
11.72 |
7.621 |
15.82 |
0.000 |
drugD-4times |
2.99 |
-1.115 |
7.09 |
0.251 |
drugE-4times |
8.57 |
4.471 |
12.67 |
0.000 |
drugE-drugD |
5.59 |
1.485 |
9.69 |
0.003 |
>par(las=2)
>par(mar=c(5,8,4,2))
>plot(TukeyHSD(fit))
For example, the mean cholesterol reductions for 1time and 2times aren’t significantly different from each other (p = 0.138), whereas the difference between 1time and 4times is significantly different (p < .001).
The pairwise comparisons are plotted in figure 9.2. The first par statement rotates the axis labels, and the second one increases the left margin area so that the labels fit (par options are covered in chapter 3). In this graph, confidence intervals that include 0 indicate treatments that aren’t significantly different (p > 0.5).
95% family−wise confidence level
2times−1tim e
4times−1tim e
drugD−1time
drugE−1time
4times−2times
drugD−2times
drugE−2times
drugD−4times
drugE−4times
drugE−drugD
0 |
5 |
10 |
15 |
20 |
|
Differences in mean levels of trt |
|
|
Figure 9.2 Plot of Tukey HSD pairwise mean comparisons
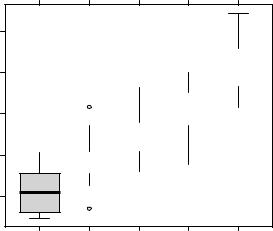
|
|
|
One-way ANOVA |
221 |
a |
a |
b |
|
|
|
b |
c |
|
|
|
|
c |
d |
|
|
|
|
|
|
25 |
|
|
|
|
|
|
|
|
|
|
|
|
|
|
|
|
|
response |
20 |
|
|
|
|
|
|
|
|
|
|
|
|
|
|
|
|
|
|
|
|
|
|
|
|
|
|
|
|
|
|
|
|
|
|
||
|
|
|
|
|
|
|
|
|
|
|
|
|
|
|
|
|
||
|
|
|
|
|
|
|
|
|
|
|
|
|
|
|
||||
15 |
|
|
|
|
|
|
|
|
|
|
|
|
|
|
|
|
|
|
|
|
|
|
|
|
|
|
|
|
|
|
|
|
|
|
|
||
|
|
|
|
|
|
|
|
|
|
|
|
|
|
|
|
|
||
|
|
|
|
|
|
|
|
|
|
|
|
|
|
|
|
|
||
|
|
|
|
|
|
|
|
|
|
|
|
|
|
|
|
|
||
10 |
|
|
|
|
|
|
|
|
|
|
|
|
|
|
|
|
|
|
|
|
|
|
|
|
|
|
|
|
|
|
|
|
|
|
|
|
|
|
|
|
|
|
|
|
|
|
|
|
|
|
|
|
|
|
|
|
|
5 |
|
|
|
|
|
|
|
|
|
|
|
|
|
|
|
|
|
|
|
|
|
|
|
|
|
|
|
|
|
|
|
|
|
|
|
|
|
|
|
|
|
|
|
|
|
|
|
|
|
|
|
|
|
|
|
|
|
|
|
|
|
|
|
|
|
|
|
|
|
|
|
|
|
Figure 9.3 Tukey HSD tests provided by the multcomp package
1time |
2times |
4times |
drugD |
drugE |
trt
The glht() function in the multcomp package provides a much more comprehensive set of methods for multiple mean comparisons that you can use for both linear models (such as those described in this chapter) and generalized linear models (covered in chapter 13). The following code reproduces the Tukey HSD test, along with a different graphical representation of the results (figure 9.3):
>library(multcomp)
>par(mar=c(5,4,6,2))
>tuk <- glht(fit, linfct=mcp(trt="Tukey"))
>plot(cld(tuk, level=.05),col="lightgrey")
In this code, the par statement increases the top margin to fit the letter array. The level option in the cld() function provides the significance level to use (0.05, or 95% confidence in this case).
Groups (represented by box plots) that have the same letter don’t have significantly different means. You can see that 1time and 2times aren’t significantly different (they both have the letter a) and that 2times and 4times aren’t significantly different (they both have the letter b); but that 1time and 4times are different (they don’t share a letter). Personally, I find figure 9.3 easier to read than figure 9.2. It also has the advantage of providing information on the distribution of scores within each group.
From these results, you can see that taking the cholesterol-lowering drug in 5 mg doses four times a day was better than taking a 20 mg dose once per day. The competitor drugD wasn’t superior to this four-times-per-day regimen. But competitor drugE was superior to both drugD and all three dosage strategies for the focus drug.
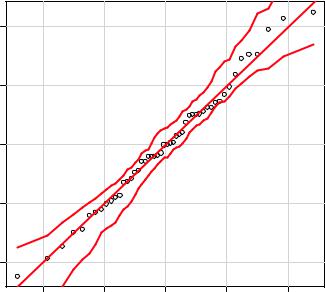
222 |
CHAPTER 9 Analysis of variance |
Multiple comparisons methodology is a complex and rapidly changing area of study. To learn more, see Bretz, Hothorn, and Westfall (2010).
9.3.2Assessing test assumptions
As you saw in the previous chapter, confidence in results depends on the degree to which your data satisfies the assumptions underlying the statistical tests. In a one-way ANOVA, the dependent variable is assumed to be normally distributed and have equal variance in each group. You can use a Q-Q plot to assess the normality assumption:
>library(car)
>qqPlot(lm(response ~ trt, data=cholesterol),
simulate=TRUE, main="Q-Q Plot", labels=FALSE)
Note the qqPlot() requires an lm() fit. The graph is provided in figure 9.4. The data falls within the 95% confidence envelope, suggesting that the normality assumption has been met fairly well.
R provides several tests for the equality (homogeneity) of variances. For example, you can perform Bartlett’s test with this code:
> bartlett.test(response ~ trt, data=cholesterol)
Bartlett test of homogeneity of variances
data: response by trt
Bartlett's K-squared = 0.5797, df = 4, p-value = 0.9653
Q−Q Plot
cholesterol)) |
2 |
|
|
|
|
data = |
1 |
|
|
|
|
Residuals(lm(response ~ trt, |
−1 0 |
|
|
|
|
Studentized |
−2 |
|
|
|
|
|
−2 |
−1 |
0 |
1 |
2 |
Figure 9.4
Test of normality
t Quantiles