
- •brief contents
- •contents
- •preface
- •acknowledgments
- •about this book
- •What’s new in the second edition
- •Who should read this book
- •Roadmap
- •Advice for data miners
- •Code examples
- •Code conventions
- •Author Online
- •About the author
- •about the cover illustration
- •1 Introduction to R
- •1.2 Obtaining and installing R
- •1.3 Working with R
- •1.3.1 Getting started
- •1.3.2 Getting help
- •1.3.3 The workspace
- •1.3.4 Input and output
- •1.4 Packages
- •1.4.1 What are packages?
- •1.4.2 Installing a package
- •1.4.3 Loading a package
- •1.4.4 Learning about a package
- •1.5 Batch processing
- •1.6 Using output as input: reusing results
- •1.7 Working with large datasets
- •1.8 Working through an example
- •1.9 Summary
- •2 Creating a dataset
- •2.1 Understanding datasets
- •2.2 Data structures
- •2.2.1 Vectors
- •2.2.2 Matrices
- •2.2.3 Arrays
- •2.2.4 Data frames
- •2.2.5 Factors
- •2.2.6 Lists
- •2.3 Data input
- •2.3.1 Entering data from the keyboard
- •2.3.2 Importing data from a delimited text file
- •2.3.3 Importing data from Excel
- •2.3.4 Importing data from XML
- •2.3.5 Importing data from the web
- •2.3.6 Importing data from SPSS
- •2.3.7 Importing data from SAS
- •2.3.8 Importing data from Stata
- •2.3.9 Importing data from NetCDF
- •2.3.10 Importing data from HDF5
- •2.3.11 Accessing database management systems (DBMSs)
- •2.3.12 Importing data via Stat/Transfer
- •2.4 Annotating datasets
- •2.4.1 Variable labels
- •2.4.2 Value labels
- •2.5 Useful functions for working with data objects
- •2.6 Summary
- •3 Getting started with graphs
- •3.1 Working with graphs
- •3.2 A simple example
- •3.3 Graphical parameters
- •3.3.1 Symbols and lines
- •3.3.2 Colors
- •3.3.3 Text characteristics
- •3.3.4 Graph and margin dimensions
- •3.4 Adding text, customized axes, and legends
- •3.4.1 Titles
- •3.4.2 Axes
- •3.4.3 Reference lines
- •3.4.4 Legend
- •3.4.5 Text annotations
- •3.4.6 Math annotations
- •3.5 Combining graphs
- •3.5.1 Creating a figure arrangement with fine control
- •3.6 Summary
- •4 Basic data management
- •4.1 A working example
- •4.2 Creating new variables
- •4.3 Recoding variables
- •4.4 Renaming variables
- •4.5 Missing values
- •4.5.1 Recoding values to missing
- •4.5.2 Excluding missing values from analyses
- •4.6 Date values
- •4.6.1 Converting dates to character variables
- •4.6.2 Going further
- •4.7 Type conversions
- •4.8 Sorting data
- •4.9 Merging datasets
- •4.9.1 Adding columns to a data frame
- •4.9.2 Adding rows to a data frame
- •4.10 Subsetting datasets
- •4.10.1 Selecting (keeping) variables
- •4.10.2 Excluding (dropping) variables
- •4.10.3 Selecting observations
- •4.10.4 The subset() function
- •4.10.5 Random samples
- •4.11 Using SQL statements to manipulate data frames
- •4.12 Summary
- •5 Advanced data management
- •5.2 Numerical and character functions
- •5.2.1 Mathematical functions
- •5.2.2 Statistical functions
- •5.2.3 Probability functions
- •5.2.4 Character functions
- •5.2.5 Other useful functions
- •5.2.6 Applying functions to matrices and data frames
- •5.3 A solution for the data-management challenge
- •5.4 Control flow
- •5.4.1 Repetition and looping
- •5.4.2 Conditional execution
- •5.5 User-written functions
- •5.6 Aggregation and reshaping
- •5.6.1 Transpose
- •5.6.2 Aggregating data
- •5.6.3 The reshape2 package
- •5.7 Summary
- •6 Basic graphs
- •6.1 Bar plots
- •6.1.1 Simple bar plots
- •6.1.2 Stacked and grouped bar plots
- •6.1.3 Mean bar plots
- •6.1.4 Tweaking bar plots
- •6.1.5 Spinograms
- •6.2 Pie charts
- •6.3 Histograms
- •6.4 Kernel density plots
- •6.5 Box plots
- •6.5.1 Using parallel box plots to compare groups
- •6.5.2 Violin plots
- •6.6 Dot plots
- •6.7 Summary
- •7 Basic statistics
- •7.1 Descriptive statistics
- •7.1.1 A menagerie of methods
- •7.1.2 Even more methods
- •7.1.3 Descriptive statistics by group
- •7.1.4 Additional methods by group
- •7.1.5 Visualizing results
- •7.2 Frequency and contingency tables
- •7.2.1 Generating frequency tables
- •7.2.2 Tests of independence
- •7.2.3 Measures of association
- •7.2.4 Visualizing results
- •7.3 Correlations
- •7.3.1 Types of correlations
- •7.3.2 Testing correlations for significance
- •7.3.3 Visualizing correlations
- •7.4 T-tests
- •7.4.3 When there are more than two groups
- •7.5 Nonparametric tests of group differences
- •7.5.1 Comparing two groups
- •7.5.2 Comparing more than two groups
- •7.6 Visualizing group differences
- •7.7 Summary
- •8 Regression
- •8.1 The many faces of regression
- •8.1.1 Scenarios for using OLS regression
- •8.1.2 What you need to know
- •8.2 OLS regression
- •8.2.1 Fitting regression models with lm()
- •8.2.2 Simple linear regression
- •8.2.3 Polynomial regression
- •8.2.4 Multiple linear regression
- •8.2.5 Multiple linear regression with interactions
- •8.3 Regression diagnostics
- •8.3.1 A typical approach
- •8.3.2 An enhanced approach
- •8.3.3 Global validation of linear model assumption
- •8.3.4 Multicollinearity
- •8.4 Unusual observations
- •8.4.1 Outliers
- •8.4.3 Influential observations
- •8.5 Corrective measures
- •8.5.1 Deleting observations
- •8.5.2 Transforming variables
- •8.5.3 Adding or deleting variables
- •8.5.4 Trying a different approach
- •8.6 Selecting the “best” regression model
- •8.6.1 Comparing models
- •8.6.2 Variable selection
- •8.7 Taking the analysis further
- •8.7.1 Cross-validation
- •8.7.2 Relative importance
- •8.8 Summary
- •9 Analysis of variance
- •9.1 A crash course on terminology
- •9.2 Fitting ANOVA models
- •9.2.1 The aov() function
- •9.2.2 The order of formula terms
- •9.3.1 Multiple comparisons
- •9.3.2 Assessing test assumptions
- •9.4 One-way ANCOVA
- •9.4.1 Assessing test assumptions
- •9.4.2 Visualizing the results
- •9.6 Repeated measures ANOVA
- •9.7 Multivariate analysis of variance (MANOVA)
- •9.7.1 Assessing test assumptions
- •9.7.2 Robust MANOVA
- •9.8 ANOVA as regression
- •9.9 Summary
- •10 Power analysis
- •10.1 A quick review of hypothesis testing
- •10.2 Implementing power analysis with the pwr package
- •10.2.1 t-tests
- •10.2.2 ANOVA
- •10.2.3 Correlations
- •10.2.4 Linear models
- •10.2.5 Tests of proportions
- •10.2.7 Choosing an appropriate effect size in novel situations
- •10.3 Creating power analysis plots
- •10.4 Other packages
- •10.5 Summary
- •11 Intermediate graphs
- •11.1 Scatter plots
- •11.1.3 3D scatter plots
- •11.1.4 Spinning 3D scatter plots
- •11.1.5 Bubble plots
- •11.2 Line charts
- •11.3 Corrgrams
- •11.4 Mosaic plots
- •11.5 Summary
- •12 Resampling statistics and bootstrapping
- •12.1 Permutation tests
- •12.2 Permutation tests with the coin package
- •12.2.2 Independence in contingency tables
- •12.2.3 Independence between numeric variables
- •12.2.5 Going further
- •12.3 Permutation tests with the lmPerm package
- •12.3.1 Simple and polynomial regression
- •12.3.2 Multiple regression
- •12.4 Additional comments on permutation tests
- •12.5 Bootstrapping
- •12.6 Bootstrapping with the boot package
- •12.6.1 Bootstrapping a single statistic
- •12.6.2 Bootstrapping several statistics
- •12.7 Summary
- •13 Generalized linear models
- •13.1 Generalized linear models and the glm() function
- •13.1.1 The glm() function
- •13.1.2 Supporting functions
- •13.1.3 Model fit and regression diagnostics
- •13.2 Logistic regression
- •13.2.1 Interpreting the model parameters
- •13.2.2 Assessing the impact of predictors on the probability of an outcome
- •13.2.3 Overdispersion
- •13.2.4 Extensions
- •13.3 Poisson regression
- •13.3.1 Interpreting the model parameters
- •13.3.2 Overdispersion
- •13.3.3 Extensions
- •13.4 Summary
- •14 Principal components and factor analysis
- •14.1 Principal components and factor analysis in R
- •14.2 Principal components
- •14.2.1 Selecting the number of components to extract
- •14.2.2 Extracting principal components
- •14.2.3 Rotating principal components
- •14.2.4 Obtaining principal components scores
- •14.3 Exploratory factor analysis
- •14.3.1 Deciding how many common factors to extract
- •14.3.2 Extracting common factors
- •14.3.3 Rotating factors
- •14.3.4 Factor scores
- •14.4 Other latent variable models
- •14.5 Summary
- •15 Time series
- •15.1 Creating a time-series object in R
- •15.2 Smoothing and seasonal decomposition
- •15.2.1 Smoothing with simple moving averages
- •15.2.2 Seasonal decomposition
- •15.3 Exponential forecasting models
- •15.3.1 Simple exponential smoothing
- •15.3.3 The ets() function and automated forecasting
- •15.4 ARIMA forecasting models
- •15.4.1 Prerequisite concepts
- •15.4.2 ARMA and ARIMA models
- •15.4.3 Automated ARIMA forecasting
- •15.5 Going further
- •15.6 Summary
- •16 Cluster analysis
- •16.1 Common steps in cluster analysis
- •16.2 Calculating distances
- •16.3 Hierarchical cluster analysis
- •16.4 Partitioning cluster analysis
- •16.4.2 Partitioning around medoids
- •16.5 Avoiding nonexistent clusters
- •16.6 Summary
- •17 Classification
- •17.1 Preparing the data
- •17.2 Logistic regression
- •17.3 Decision trees
- •17.3.1 Classical decision trees
- •17.3.2 Conditional inference trees
- •17.4 Random forests
- •17.5 Support vector machines
- •17.5.1 Tuning an SVM
- •17.6 Choosing a best predictive solution
- •17.7 Using the rattle package for data mining
- •17.8 Summary
- •18 Advanced methods for missing data
- •18.1 Steps in dealing with missing data
- •18.2 Identifying missing values
- •18.3 Exploring missing-values patterns
- •18.3.1 Tabulating missing values
- •18.3.2 Exploring missing data visually
- •18.3.3 Using correlations to explore missing values
- •18.4 Understanding the sources and impact of missing data
- •18.5 Rational approaches for dealing with incomplete data
- •18.6 Complete-case analysis (listwise deletion)
- •18.7 Multiple imputation
- •18.8 Other approaches to missing data
- •18.8.1 Pairwise deletion
- •18.8.2 Simple (nonstochastic) imputation
- •18.9 Summary
- •19 Advanced graphics with ggplot2
- •19.1 The four graphics systems in R
- •19.2 An introduction to the ggplot2 package
- •19.3 Specifying the plot type with geoms
- •19.4 Grouping
- •19.5 Faceting
- •19.6 Adding smoothed lines
- •19.7 Modifying the appearance of ggplot2 graphs
- •19.7.1 Axes
- •19.7.2 Legends
- •19.7.3 Scales
- •19.7.4 Themes
- •19.7.5 Multiple graphs per page
- •19.8 Saving graphs
- •19.9 Summary
- •20 Advanced programming
- •20.1 A review of the language
- •20.1.1 Data types
- •20.1.2 Control structures
- •20.1.3 Creating functions
- •20.2 Working with environments
- •20.3 Object-oriented programming
- •20.3.1 Generic functions
- •20.3.2 Limitations of the S3 model
- •20.4 Writing efficient code
- •20.5 Debugging
- •20.5.1 Common sources of errors
- •20.5.2 Debugging tools
- •20.5.3 Session options that support debugging
- •20.6 Going further
- •20.7 Summary
- •21 Creating a package
- •21.1 Nonparametric analysis and the npar package
- •21.1.1 Comparing groups with the npar package
- •21.2 Developing the package
- •21.2.1 Computing the statistics
- •21.2.2 Printing the results
- •21.2.3 Summarizing the results
- •21.2.4 Plotting the results
- •21.2.5 Adding sample data to the package
- •21.3 Creating the package documentation
- •21.4 Building the package
- •21.5 Going further
- •21.6 Summary
- •22 Creating dynamic reports
- •22.1 A template approach to reports
- •22.2 Creating dynamic reports with R and Markdown
- •22.3 Creating dynamic reports with R and LaTeX
- •22.4 Creating dynamic reports with R and Open Document
- •22.5 Creating dynamic reports with R and Microsoft Word
- •22.6 Summary
- •afterword Into the rabbit hole
- •appendix A Graphical user interfaces
- •appendix B Customizing the startup environment
- •appendix C Exporting data from R
- •Delimited text file
- •Excel spreadsheet
- •Statistical applications
- •appendix D Matrix algebra in R
- •appendix E Packages used in this book
- •appendix F Working with large datasets
- •F.1 Efficient programming
- •F.2 Storing data outside of RAM
- •F.3 Analytic packages for out-of-memory data
- •F.4 Comprehensive solutions for working with enormous datasets
- •appendix G Updating an R installation
- •G.1 Automated installation (Windows only)
- •G.2 Manual installation (Windows and Mac OS X)
- •G.3 Updating an R installation (Linux)
- •references
- •index
- •Symbols
- •Numerics
- •23.1 The lattice package
- •23.2 Conditioning variables
- •23.3 Panel functions
- •23.4 Grouping variables
- •23.5 Graphic parameters
- •23.6 Customizing plot strips
- •23.7 Page arrangement
- •23.8 Going further
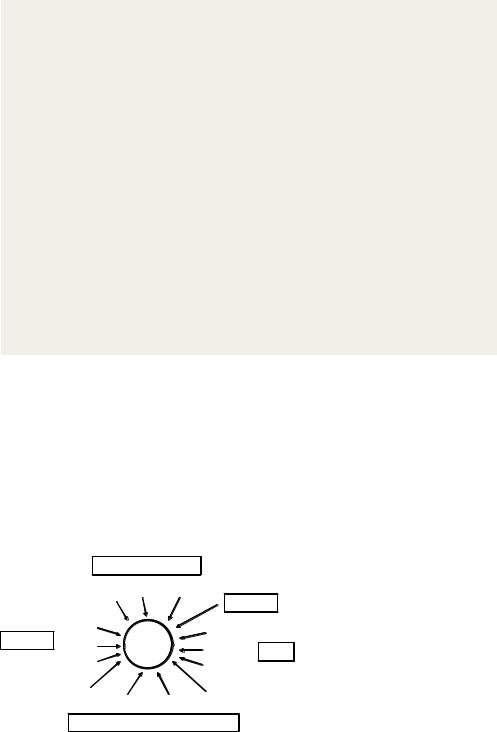
32 |
CHAPTER 2 Creating a dataset |
(continued)
■R doesn’t provide multiline or block comments. You must start each line of a multiline comment with #. For debugging purposes, you can also surround code that you want the interpreter to ignore with the statement if(FALSE){...}. Changing the FALSE to TRUE allows the code to be executed.
■Assigning a value to a nonexistent element of a vector, matrix, array, or list expands that structure to accommodate the new value. For example, consider the following:
>x <- c(8, 6, 4)
>x[7] <- 10
>x
[1]8 6 4 NA NA NA 10
The vector x has expanded from three to seven elements through the assignment. x <- x[1:3] would shrink it back to three elements.
■R doesn’t have scalar values. Scalars are represented as one-element vectors.
■Indices in R start at 1, not at 0. In the vector earlier, x[1] is 8.
■Variables can’t be declared. They come into existence on first assignment.
To learn more, see John Cook’s excellent blog post, “R Language for Programmers” (http://mng.bz/6NwQ). Programmers looking for stylistic guidance may also want to check out “Google’s R Style Guide” (http://mng.bz/i775).
2.3Data input
Now that you have data structures, you need to put some data in them! As a data analyst, you’re typically faced with data that comes from a variety of sources and in a variety of formats. Your task is to import the data into your tools, analyze the data, and report on the results. R provides a wide range of tools for importing data. The definitive guide for importing data in R is the R Data Import/Export manual available at http://mng.bz/urwn.
As you can see in figure 2.2, R can import data from the keyboard, from text files, from Microsoft Excel and Access, from popular statistical packages, from a variety of
|
Statistical packages |
|||
|
SAS |
SPSS |
Stata |
|
|
|
|
Keyboard |
|
|
ASCII |
R |
Excel |
|
Text files |
|
|||
XML |
netCFD Other |
|||
|
||||
Webscraping |
|
HDF5 |
SQL MySQL Oracle Access
Figure 2.2 Sources of data that
Database management systems |
can be imported into R |
|
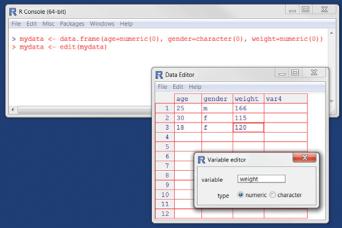
Data input |
33 |
relational database management systems, from specialty databases, and from web sites and online services. Because you never know where your data will come from, we’ll cover each of them here. You only need to read about the ones you’re going to be using.
2.3.1Entering data from the keyboard
Perhaps the simplest way to enter data is from the keyboard. There are two common methods: entering data through R’s built-in text editor and embedding data directly into your code. We’ll consider the editor first.
The edit() function in R invokes a text editor that lets you enter data manually. Here are the steps:
1Create an empty data frame (or matrix) with the variable names and modes you want to have in the final dataset.
2Invoke the text editor on this data object, enter your data, and save the results to the data object.
The following example creates a data frame named mydata with three variables: age (numeric), gender (character), and weight (numeric). You then invoke the text editor, add your data, and save the results:
mydata <- data.frame(age=numeric(0), gender=character(0), weight=numeric(0))
mydata <- edit(mydata)
Assignments like age=numeric(0) create a variable of a specific mode, but without actual data. Note that the result of the editing is assigned back to the object itself. The edit() function operates on a copy of the object. If you don’t assign it a destination, all of your edits will be lost!
The results of invoking the edit() function on a Windows platform are shown in figure 2.3. In this figure, I’ve added some data. If you click a column title, the editor
Figure 2.3 Entering data via the built-in editor on a Windows platform
34 |
CHAPTER 2 Creating a dataset |
gives you the option of changing the variable name and type (numeric or character). You can add variables by clicking the titles of unused columns. When the text editor is closed, the results are saved to the object assigned (mydata, in this case). Invoking mydata <- edit(mydata) again allows you to edit the data you’ve entered and to add new data. A shortcut for mydata <- edit(mydata) is fix(mydata).
Alternatively, you can embed the data directly in your program. For example, the code
mydatatxt <- " age gender weight 25 m 166
30 f 115
18 f 120
"
mydata <- read.table(header=TRUE, text=mydatatxt)
creates the same data frame as that created with the edit() function. A character string is created containing the raw data, and the read.table() function is used to process the string and return a data frame. The read.table() function is described more fully in the next section.
Keyboard data entry can be convenient when you’re working with small datasets. For larger datasets, you’ll want to use the methods described next: importing data from existing text files, Excel spreadsheets, statistical packages, or databasemanagement systems.
2.3.2Importing data from a delimited text file
You can import data from delimited text files using read.table(), a function that reads a file in table format and saves it as a data frame. Each row of the table appears as one line in the file. The syntax is
mydataframe <- read.table(file, options)
where file is a delimited ASCII file and the options are parameters controlling how data is processed. The most common options are listed in table 2.2.
Table 2.2 read.table() options
Option |
Description |
|
|
header |
A logical value indicating whether the file contains the variable names in the first line. |
sep |
The delimiter separating data values. The default is sep="", which denotes one or |
|
more spaces, tabs, new lines, or carriage returns. Use sep="," to read comma- |
|
delimited files, and sep="\t" to read tab-delimited files. |
row.names |
An optional parameter specifying one or more variables to represent row identifiers. |
col.names |
If the first row of the data file doesn’t contain variable names (header=FALSE), you |
|
can use col.names to specify a character vector containing the variable names. If |
|
header=FALSE and the col.names option is omitted, variables will be named V1, |
|
V2, and so on. |
|
|
|
Data input |
35 |
Table 2.2 read.table() options |
|
|
|
|
|
Option |
Description |
|
|
|
|
na.strings |
Optional character vector indicating missing-values codes. For example, na.strings |
|
|
=c("-9", "?") converts each -9 and ? value to NA as the data is read. |
|
colClasses |
Optional vector of classes to be assign to the columns. For example, colClasses |
|
|
=c("numeric", "numeric", "character", "NULL", "numeric") reads the |
|
|
first two columns as numeric, reads the third column as character, skips the fourth col- |
|
|
umn, and reads the fifth column as numeric. If there are more than five columns in the |
|
|
data, the values in colClasses are recycled. When you’re reading large text files, |
|
|
including the colClasses option can speed up processing considerably. |
|
quote |
Character(s) used to delimit strings that contain special characters. By default this is |
|
|
either double (") or single (') quotes. |
|
skip |
The number of lines in the data file to skip before beginning to read the data. This |
|
|
option is useful for skipping header comments in the file. |
|
strings- |
A logical value indicating whether character variables should be converted to factors. |
|
AsFactors |
The default is TRUE unless this is overridden by colClasses. When you’re processing |
|
|
large text files, setting stringsAsFactors=FALSE can speed up processing. |
|
text |
A character string specifying a text string to process. If text is specified, leave file |
|
|
blank. An example is given in section 2.3.1. |
|
|
|
|
Consider a text file named studentgrades.csv containing students’ grades in math, science, and social studies. Each line of the file represents a student. The first line contains the variable names, separated with commas. Each subsequent line contains a student’s information, also separated with commas. The first few lines of the file are as follows:
StudentID,First,Last,Math,Science,Social Studies
011,Bob,Smith,90,80,67
012,Jane,Weary,75,,80
010,Dan,"Thornton, III",65,75,70
040,Mary,"O'Leary",90,95,92
The file can be imported into a data frame using the following code:
grades <- read.table("studentgrades.csv", header=TRUE, row.names="StudentID", sep=",")
The results are as follows:
> grades
|
First |
Last Math Science Social.Studies |
|||
11 |
Bob |
Smith |
90 |
80 |
67 |
12 |
Jane |
Weary |
75 |
NA |
80 |
10 |
Dan |
Thornton, III |
65 |
75 |
70 |
40 |
Mary |
O'Leary |
90 |
95 |
92 |
> str(grades)
36 |
|
|
CHAPTER 2 Creating a dataset |
|||
'data.frame': |
4 obs. of |
5 |
variables: |
|||
$ |
First |
: Factor w/ |
4 levels "Bob","Dan","Jane",..: 1 3 2 4 |
|||
$ |
Last |
: Factor w/ |
4 levels "O'Leary","Smith",..: 2 4 3 1 |
|||
$ |
Math |
: int |
90 |
75 65 |
90 |
|
$ |
Science |
: int |
80 |
NA 75 |
95 |
|
$ |
Social.Studies: int |
67 |
80 70 |
92 |
There are several interesting things to note about how the data is imported. The variable name Social Studies is automatically renamed to follow R conventions. The StudentID column is now the row name, no longer has a label, and has lost its leading zero. The missing science grade for Jane is correctly read as missing. I had to put quotation marks around Dan's last name in order to escape the comma between Thornton and III. Otherwise, R would have seen seven values on that line, rather than six. I also had to put quotation marks around O'Leary. Otherwise, R would have read the single quote as a string delimiter (which isn’t what I want). Finally, the first and last names are converted to factors.
By default, read.table() converts character variables to factors, which may not always be desirable. For example, there would be little reason to convert a character variable containing a respondent’s comments into a factor. You can suppress this behavior in a number of ways. Including the option stringsAsFactors=FALSE turns off this behavior for all character variables. Alternatively, you can use the colClasses option to specify a class (for example, logical, numeric, character, or factor) for each column.
Importing the same data with
grades <- read.table("studentgrades.csv", header=TRUE, row.names="StudentID", sep=",", colClasses=c("character", "character", "character",
"numeric", "numeric", "numeric"))
produces the following data frame:
> grades |
|
|
|
|
|
|
|
First |
|
Last |
Math Science |
Social.Studies |
|
011 |
Bob |
Smith |
90 |
80 |
67 |
|
012 |
Jane |
Weary |
75 |
NA |
80 |
|
010 |
Dan |
Thornton, |
III |
65 |
75 |
70 |
040 |
Mary |
O'Leary |
90 |
95 |
92 |
|
> str(grades) |
|
|
|
|
|
|
'data.frame': |
4 obs. of |
5 variables: |
|
|||
$ |
First |
: chr |
"Bob" "Jane" |
"Dan" "Mary" |
||
$ |
Last |
: chr |
"Smith" "Weary" |
"Thornton, III" "O'Leary" |
||
$ |
Math |
: num |
90 |
75 65 90 |
|
|
$ |
Science |
: num |
80 |
NA 75 95 |
|
|
$ |
Social.Studies: num |
67 |
80 70 92 |
|
|
Note that the row names retain their leading zero and First and Last are no longer factors. Additionally, the grades are stored as real values rather than integers.