
Kluwer - Handbook of Biomedical Image Analysis Vol
.2.pdf522 |
Suri et al. |
[99]Klingensmith, J. D., Shekhar, R., and Vince, D. G., Evaluation of threedimensional segmentation algorithms for the identification of luminal and medial-adventitial borders in intravascular ultrasound images, IEEE Trans. Med. Imaging, Vol. 19, No. 10, pp. 996–1011, 2000.
[100]Ladak, H. M., Thomas, J. B., Mitchell, J. R., Rutt, B. K., and Steinman,
D.A., A semi-automatic technique for measurement of arterial wall from black blood MRI, Med. Phy., Vol. 28, No. 6, p. 1098, 2001.
[101]Lancaster, T. L. and Wilson, D. L., Correcting spatial distortion of histological images, Ann. Biomed. Eng., Vol. XX, No. X, pp. XXX-XXX, 2003.
[102]Lazebnik, R., Lancaster, T. L., Breen, M. S., Lewin J. S., and Wilson,
D.L., Volume registration using needle paths and point landmarks for evaluation of interventional MRI treatments, IEEE Trans. Med. Imaging, Vol. 22, No. 5, pp. 659–660, 2003.
[103]Lorigo, L. M., Faugeras, O., Grimson, W. E. L., Keriven, R., Kikinis, R., Nabavi, A., and Westin, C. F., Codimension-two geodesic active contours for the segmentation of tubular structures, Vol. 1, No. 13–15, pp. 444–451, 2000.
[104]Maes, F., Collignon, A., Vandermeulen, D., Marchal, G., and Suetens, P., Multimodality image registration by maximization of mutual information, IEEE Trans. Med. Imaging, Vol. 16, No. 2, pp. 187–198, 1997.
[105]Merickel, M. B., Carman, C. S., Watterson, W. K., Brookeman, J. R., and Ayers, C. R., Multispectral pattern recognition of MR imagery for the noninvasive analysis of atherosclerosis, In: 9th International Conference on Pattern Recognition, 1988, pp. 1192–1197.
[106]Pallotta, S., Gilardi, M. C., Bettinardi, B., Rizzo, G., Landoni, C., Striano, G., Masi, R., and Fazio, F., Application of a surface matching image registration technique to the correlation of cardiac studies in position emission tomography (PET) by transmission images, Phy. Med. Biol., Vol. 40, No. 10, pp. 1695–1708, 1995.
[107]Pelizzari, C. A., Chen, G. T. Y., Spelbring, D. R., Weichselbaum, R. R., and Chen, C. T., Accurate three-dimensional registration of CT, PET
Lumen Identification, Detection, and Quantification in MR Plaque Volumes |
523 |
and/or MR images of the brain, J. Comput. Assist. Tomography, Vol. 13, No. 1, pp. 20–26, 1989.
[108]Pietrzyk, U., Herholz, K., Fink, G., Jacobs, A., Mielke, R., Slansky, I., Michael, W., and Heiss, W. D., An interactive tecnique for threedimensional image registration: Validation for PET, SPECT, MRI and CT brain studies, J. Nuclear Med., Vol. 35, No. 12, pp. 2011–2018, 1994.
[109]Rioufol, G., Finet, G., Ginon, I., Andre, F., XRossi, R., Vialle, E., Desjoyaux, E., Convert, G., Huret, J. F., and Tabib, A., Multiple atherosclerotic plaque rupture in acute coronary syndrome: A three-vessel intravascular ultrasound study, Circulation, Vol. 106, No. 7, p. 804, 2002.
[110]Saeed, N., Magnetic resonance image segmentation using pattern recognition, and applied to image registration and quantitation, NMR Biomed., Vol. 11, No. 4/5, pp. 157, 1998.
[111]Shattuck, D. W., Sandor-Leahy, S. R., Schaper, K. A., Rottenberg, D. A., and Leahy, R. M., Magnetic resonance image tissue classification using a partial volume model, Neuroimage, Vol. 13, No. 5, p. 856, 2001.
[112]Thieme, T., Wernecke, K. D., Meyer, R., Brandenstein, E., Habedank, D., Hinz, A., Felix, S. B., Baumann, G., and Kleber, F. X., Angioscopic evaluation of atherosclerotic plaques: Validation by histomorphologic analysis and association with stable and unstable coronary syndromes,
J.Am. Coll. Cardiol., Vol. 28, No. 1, pp. 1–6, 1996.
[113]Trouard, T. P., Altbach, M. I., Hunter, G. C., Eskelson, C. D., and Gmitro,
A.F., MRI and NMR spectroscopy of the lipids of atherosclerotic plaque in rabbits and humans, Magn. Reson. Med., Vol. 38, No. 1, pp. 19–26, 1997.
[114]van den Elsen, P. A., Pol, E. J. D., and Viergever, M. A., Medical image matching—A review with classification, IEEE Eng. Med. Biol., Vol. 12, No. 1, pp. 26–39, 1993.
[115]Viola, P. A. and Wells, W. M., III, Alignment by maximization of mutual information, In: IEEE Proceedings of the 5th International Conference on Computer Vision, 1995, pp. 16–23.
524 |
Suri et al. |
[116]Weber, D. A. and Ivanovic, M., Correlative image registration, Semin. Nuclear Med., Vol. 24, No. 4, pp. 311–323, 1994.
[117]West, J., Fitzpatrick, M., Wang, M. Y., Dawant, B. M., Maurer, C. R., Kessler, M. L., Maciunas, R. J., Barillot, C., Lemoine, D., Collignon, A., Maes, F., Suetens, P., Vandermeulen, D., van den Elsen, P. A., Napel, S., Sumanaweera, T. S., Harkness, B. A., Hemler, P. F., Hill, D. L. G., Hawkes, D. J., Studholme, C., Maintz, J. B., Viergever, M. A., Malandain, G., Pennec, X., Noz, M. E., Maguire, G. Q., Pollack, M., Pelizzari, C. A., Robb, R. A., Hanson, D., and Woods, R. P., Comparison and evaluation of retrospective intermodality brain image registration techniques, J. Comput. Assist. Tomography, Vol. 21, No. 4, pp. 554–566, 1997.
[118]Breen, M. S., Lancaster T. L., Lazebnik, R., Nour S. G., Lewin J. S., and Wilson, D. L., Three dimensional method for comparing in vivo interventional MR images of thermally ablated tissue with tissue response,
J.Magn. Reson. Imaging, Vol. 18, No. 1, pp. 90–102, 2003.
[119]Wilson, D. L., Carrillo, A., Zheng, L., Genc, A., Duerk, J. L., and Lewin,
J.S., Evaluation of 3D image registration as applied to MR-guided thermal treatment of liver cancer, J. Magn. Reson. Imaging, Vol. 8, No. 1, pp. 77–84, 1998.
[120]Wink, O., Fast delineation and visualization of vessels in 3-D angiographic images, IEEE Trans. Med. Imaging, Vol. 19, No. 4, pp. 337–346, 2000.
[121]Yu, J. N., Fahey, F. H., Gage, H. D., Eades, C. G., Harkness, B. A., Pelizzari, C. A., and Keyes, J. W., Intermodality, retrospective image registration in the thorax, J. Nuclear Med., Vol. 36, No. 12, pp. 2333– 2338, 1995.
[122]Draney, M. T., Herfkens, R. J., Hughes, T. J. R., Plec, N. J., Wedding, K. L., Zarins, C. K., and Taylor, C. A., Quantification of vessel wall cyclic strain using cine phase contrast magnetic resonance imaging, Ann. Biomed. Eng., Vol. 30, No. 8, pp. 1033–1045, 2002.
[123]MacNeill, B. D., Lowe, H. C., Takano, M., Fuster, V., and Jang, I.-K., Intravascular modalities for detection of vulnerable plaque: current
Lumen Identification, Detection, and Quantification in MR Plaque Volumes |
525 |
status, Arterioscler. Thromb. Vasc. Biol., Vol. 23, No. 8, pp. 1333–1342,
2003.
[124]Ziada, K., Tuzcu, E. M., Nissen, S. E., Ellis, S., Whitlow, P. L., and Franco, I., Prognostic importance of various intravascular ultrasound measurements of lumen size following coronary stenting (submitted).
[125]Ziada, K., Kapadia, S., Tuzcu, E. M., and Nissen, S. E., The current status of intravascular ultrasound imaging, Curr. Prob. Cardiol., Vol. 24, No. 9,
pp.541–616, 1999.
[126]Nair, A., Kuban, B. D., Tuzcu, E. M., Schoenhagen, P., Nissen, S. E., and Vince, D. G., Coronary plaque classification with intravascular ultrasound radiofrequency data analysis, Circulation, Vol. 106, No. 17,
pp.2200–2206, 2002.
[127]Nissen, S. E. and Yock, P., Intravascular ultrasound: Novel pathophysiological insights and current clinical applications, Circulation, Vol. 103, No. 4, pp. 604–616, 2001.
[128]Woods, R. P., Cherry, S. R., and Mazziotta, J. C., Rapid automated algorithm for aligning and reslicing PET images, J. Comput. Assist. Tomography, Vol. 16, No. 4, pp. 620–633, 1992.
[129]Woods, R. P., Mazziotta, J. C., and Cherry, S. R., MRI-PET registration with automated algorithm, J. Comput. Assist. Tomography, Vol. 17, No. 4, pp. 536–546, 1993.
[130]Fei, B. W., Boll, D. T., Duerk, J. L., and Wilson, D. L., Image registration for interventional MRI-guided minimally invasive treatment of prostate cancer, In: The 2nd Joint Meeting of the IEEE Engineering in Medicine and Biology Society and the Biomedical Engineering Society, 2002, Vol. 2, p. 1185.
[131]Fei, B. W., Duerk, J. L., Boll, D. T., Lewin, J. S., and Wilson, D. L., Slice to volume registration and its potential application to interventional MRI guided radiofrequency thermal ablation of prostate cancer, IEEE Trans. Med. Imaging, Vol. 22, No. 4, pp. 515–525, 2003.
526 |
Suri et al. |
[132]Fei, B. W., Duerk, J. L., and Wilson, D. L., Automatic 3D registration for interventional MRI-guided treatment of prostate cancer, Comput. Aided Surg., Vol. 7, No. 5, pp. 257–267, 2002.
[133]Fei, B. W., Frinkley K., and Wilson, D. L., Registration algorithms for interventional MRI-guided treatment of the prostate cancer, Proc. SPIE Med. Imaging, Vol. 5029, pp. 192–201, 2003.
[134]Fei, B. W., Kemper, C., and Wilson, D. L., A comparative study of warping and rigid body registration for the prostate and pelvic MR volumes, Comput. Med. Imaging Graph., Vol. 27, No. 4, pp. 267–281, 2003.
[135]Fei, B. W., Kemper, C., and Wilson, D. L., Three-dimensional warping registration of the pelvis and prostate, In: Proceedings of SPIE Medical Imaging on Image Processing, Sonka, M. and Fitzpatrick, J. M., eds., Vol. 4684, pp. 528–537, 2002.
[136]Fei, B. W., Wheaton, A., Lee, Z., Duerk, J. L., and Wilson, D. L., Automatic MR volume registration and its evaluation for the pelvis and prostate, Phy. Med. Biol., Vol. 47, No. 5, pp. 823–838, 2002.
[137]Fei, B. W., Wheaton, A., Lee, Z., Nagano, K., Duerk, J. L., and Wilson, D. L., Robust registration algorithm for interventional MRI guidance for thermal ablation of prostate cancer, In: Proceedings of SPIE Medical Imaging on Visualization, Display, and Image-Guided Procedures, Ki Mun, S., ed., Vol. 4319, pp. 53–60, 2001.
[138]Wilson, D. L. and Fei, B. W., Three-dimensional semiautomatic warping registration of the prostate and pelvis, Med. Phy. (submitted).
[139]Studholme, C., Hill, D. L. G., and Hawkes, D. J., Automated 3D registration of MR and CT images of the head, Med. Image Anal., Vol. 1, No. 2, pp. 163–175, 1996.
[140]Studholme, C., Hill, D. L. G., and Hawkes, D. J., Automated threedimensional registration of magnetic resonance and positron emission tomography brain images by multiresolution optimization of voxel similarity measures, Med. Phy., Vol. 24, No. 1, pp. 25–35, 1997.
Lumen Identification, Detection, and Quantification in MR Plaque Volumes |
527 |
[141]Song, C. Z. and Yuille, A., Region competition: Unifying snakes, region growing, and Bayes/MDL for multiband image segmentation, IEEE Trans. Patt. Anal. Machine Intell., Vol. 18, No. 9, pp. 884–900, 1996.
[142]Stary, H. C., Chandler, A. B., Glagov, S., Guyton, J. R., Insull, W. J., Rosenfeld, M. E., Schaffer, S. A., Schwartz, C. J., Wagner, W. D., and Wissler, R. W., A definition of initial, fatty streak, and intermediate lesions of atherosclerosis: A report from the Committee on Vascular Lesions of the Council on Arteriosclerosis, American Heart Association, Arterioscler. Thromb., Vol. 14, No. 5, pp. 840–856, 1994.
[143]Hemler, P. F., Napel, S., Sumanaweera, T. S., Pichumani, R., van den Elsen, P. A., Martin, D., Drace, J., Adler, J. R., and Perkash, I., Registration error quantification of a surface-based multimodality image fusion system, Med. Phy., Vol. 22, No. 7, pp. 1049–1056, 1995.
[144]Suri, J. S., White matter/Gray matter boundary segmentation using geometric snakes: A fuzzy deformable model, In: International Conference in Application in Pattern Recognition (ICAPR), Rio de Janeiro, Brazil, March 11–14, 2001.
[145]Zhang, J., The mean field theory in EM procedures for Markov random fields, IEEE Trans. Signal Process., Vol. 40, No. 10, 1992.
[146]Kapur, T., Model Based Three Dimensional Medical Image Segmentation, Ph.D. Thesis, Artificial Intelligence Laboratory, Massachusetts Institute of Technology, Cambridge, MA, May 1999.
[147]Li, S., Markov Random Field Modeling in Computer Vision, Springer Verlag, Berlin, 1995. ISBN 0-387-701-451.
[148]Held, K., Rota Kopps, E., Krause, B., Wells, W., Kikinis, R., and MullerGartner, H., Markov random field segmentation of brain MR images, IEEE Trans. Med. Imaging, Vol. 16, No. 6, pp. 878–887, 1998.
[149]Witkin, A. P., Scale-space filtering, In: Proceedings of 8th International Joint Conference on Artificial Intelligence, Karlsruhe, West Germany, 1983, Vol. 2, pp. 1019–1023.
528 |
Suri et al. |
[150]Koenderink, J. J., The structure of images, Biol. Cyb., Vol. 50, pp. 363– 370, 1984.
[151]Koller, T. M., Gerig, G., Szekely,´ G., and Dettwiler, D., Multiscale detection of curvilinear structures in 2-D and 3-D image data, In: IEEE International Conference on Computer Vision (ICCV), 1995, pp. 864– 869.
[152]Koller, T. M., From Data to Information: Segmentation, Description and Analysis of the Cerebral Vascularity, Ph.D. Thesis, Swiss Federal Institute of Technology, Zurich,¨ 1995.
[153]Gerig, G., Koller, M. Th., Szekely,´ Brechbuhler, C., and Kubler, O., Symbolic description of 3-D structures applied to cerebral vessel tree obtained from MR angiography volume data, In: Proceedings of IPMI, Series Lecture Notes in Computer Science, Vol. 687, Barett, H. H. and Gmitro, A. F., eds., Springer-Verlag, Berlin, pp. 94–111, 1993.
[154]Thirion, J. P. and Gourdon, A., The 3-D marching lines algorithm, Graph. Models Image Process., Vol. 58, No. 6, pp. 503–509, 1996.
[155]Lindeberg, T., Scale-space for discrete signals, IEEE Patt. Anal. Machine Intell., Vol. 12, No. 3, pp. 234–254, 1990.
[156]Lindeberg, T., On scale selection for differential operators, In: Proceedings of the 8th Scandinavian Conference on Image Analysis (SCIA), 1993, pp. 857–866.
[157]Lindeberg, T., Detecting salient blob-like image structures and their scales with a scalespace primal sketch: A method for focus of attention, Int. J. Comput. Vision, Vol. 11, No. 3, pp. 283–318, 1993.
[158]Lindeberg, T., Edge detection and ridge detection with automatic scale selection, In: Proceedings of Computer Vision and Pattern Recognition, 1996, pp. 465–470.
[159]Alyward, S., Bullitte, E., Pizer, S., and Eberly, D., Intensity ridge and widths for tubular object segmentation and description, In: Proceedings of Workshop Mathematical Methods Biomedical Image Analysis (WMMBIA), Amini, A. A. and Bookstein, F. L., eds., pp. 131–138, 1996.
Lumen Identification, Detection, and Quantification in MR Plaque Volumes |
529 |
[160]Lorenz, C., Carlsen, I.-C., Buzug, T. M., Fassnacht, C., and Wesse, J., Multi-scale line segmentation with automatic estimation of width, contrast and tangential direction in 2-D and 3-D medical images, In: Proceedings of Joint Conference on CVRMed and MRCAS, 1997, pp. 233– 242.
[161]Fidrich, M., Following features lines across scale, In: Proceedings of Scale-Space Theory in Computer Vision, Series Lectures Notes in Computer Science, Vol. 1252, ter Haar Romeny, B., Florack, L., Loeenderink, J., and Viergever, M., eds., Springer-Verlag, Berlin, pp. 140–151, 1997.
[162]Lindeberg, T., Feature detection with automatic scale-space selection, Int. J. Comput. Vision, Vol. 30, No. 2, pp. 79–116, 1998.
[163]Prinet, V., Monga, O., and Rocchisani, J. M., Vessels Representation in 2D and 3D Angiograms, International Congress Series (ICS), Vol. 1134, pp. 240–245, 1998. ISSN 0531-5131.
[164]Prinet, V., Monga, O., Ge, C., Loa, X. S., and Ma, S., Thin network extraction in 3-D images: Application of medial angiograms, In: International Conference on Pattern Recognition, Aug. 1996.
[165]Griffin, L., Colchester, A., and Robinson, G., Scale and segmentation of images using maximum gradient paths, Image Vision Comput., Vol. 10, No. 6, pp. 389–402, 1992.
[166]Koenderink, J. and van Doorn, A., Local features of smooth shapes: Ridges and course, In: SPIE Proceedings on Geometric Methods in Computer Vision-II, 1993, Vol. 2031, pp. 2–13.
[167]Koenderink, J. and van Doorn, A., Two-plus-one-dimensional differential geometry, Patt. Recogn. Lett., Vol. 15, No. 5, pp. 439–444, 1994.
[168]Majer, P., A statistical approach to feature detection and scale selection in images, Ph.D. Thesis, University of Gottingen,¨ Gesellschaft fur¨ wissenschaftliche Datenverarbeitung mbH Gottingen,¨ Germany, July 2000.
530 |
Suri et al. |
[169]Wells, W. M., III, Grimson, W. E. L., Kikinis, R., and Jolesz, F. A., Adaptive segmentation of MRI data, IEEE Trans. Med. Imaging, Vol. 15, No. 4,
pp.429–442, 1992.
[170]Gerig, G., Kubler, O., and Jolesz, F. A., Nonlinear anisotropic filtering of MRI data, IEEE Trans. Med. Imaging, Vol. 11, No. 2, pp. 221–232, 1992.
[171]Joshi, M., Cui, J., Doolittle, K., Joshi, S., Van Essen, D., Wang, L., and Miller, M. I., Brain segmentation and the generation of cortical surfaces, Neuroimage, Vol. 9, No. 5, pp. 461–476, 1999.
[172]Dempster, A. D., Laird, N. M., and Rubin, D. B., Maximum likelihood from incomplete data via the EM algorithm, J. R. Stat. Soc., Vol. 39,
pp.1–37, 1977.
[173]Kao, Y.-H., Sorenson, J. A., Bahn, M. M., and Winkler, S. S., DualEcho MRI segmentation using vector decomposition and probability technique: A two tissue model, Magn. Reson. Med., Vol. 32, No. 3,
pp.342–357, 1994.
[174]Geman, S. and Geman, D., Stochastic relaxation, Gibbs distribution and the Bayesian restoration of images, IEEE Trans. Patt. Anal. Machine Intell., Vol. 6, pp. 721–741, 1984.
[175]Bezdek, J. C. and Hall, L. O., Review of MR image segmentation techniques using pattern recognition, Med. Phy., Vol. 20, No. 4, pp. 1033– 1048, 1993.
[176]Hall, L. O. and Bensaid, A. M., A comparison of neural networks and fuzzy clustering techniques in segmenting MRI of the brain, IEEE Trans. Neural Networks, Vol. 3, No. 5, pp. 672–682, 1992.
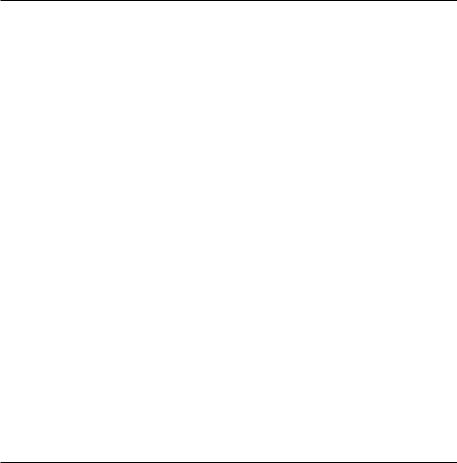