
Kluwer - Handbook of Biomedical Image Analysis Vol
.2.pdf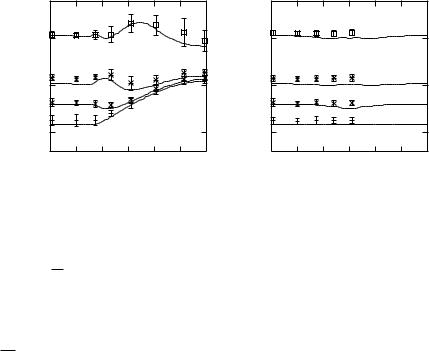

Hessian-Based Multiscale Enhancement and Segmentation |
583 |
θ and φ and the plots of the simulated thickness representing the dependences
on sheet normal orientation θ and φ. Figures 10.22(a) and 10.22(b) show the
√
plots of the dependences of θ and φ with σ = 22 xy, respectively. Good agreement between the simulated and the in vitro thicknesses was observed in both cases although the in vitro thicknesses was slightly greater than the simulated thickness. The biases, i.e., the difference between the simulated thickness and the average of in vitro thickness, were predominantly around 0.1 mm or less (except for θ = 75◦ of τ = 3 mm), and the SDs of the in vitro thickness were mostly within 0.1 mm (except for θ = 45◦ of τ = 2 mm and θ ≥ 35◦ of τ = 3 mm). It should be noted that the dependence on φ is theoretically equivalent to
the dependence on θ when the anisotropy is z = 1.
xy
10.6 Concluding Remarks
We have presented a framework for multiscale analysis of the second-order local structures in medical volume data based on the analysis of the Hessian matrix. Multiscale filtering methods for enhancement of sheet, line, and blob structures were formulated. The guidelines for the filter design were clarified based on detailed analyses of singleand multiscale filter responses using mathematical local structure models. Further, formal approaches to description and quantification of sheet and line structures were presented. The accuracy of width quantification of sheet structures were theoretically analyzed and its inherent limits due to imaging resolution and postprocessing parameters were derived. In this chapter, we purely focus on local structures. Future work will include grouping these local structures to obtain higher-level descriptions incorporating global structures.
10.7 Acknowledgments
The author thanks Dr. Ron Kikinis and Dr. Shin Nakajima at Harvard Medical School and Brigham and Women’s Hospital for providing MR data of a brain, Dr. Hironobu Ohmatsu of the National Cancer Center, Japan, for providing CT data of a chest, Dr. Nobuyuki Shiraga at Keio University for providing abdominal CT data, Dr. Shigeyuki Yoshida at Osaka University for providing a CT data of a
584 |
Sato |
chest, and Dr. Katsuyuki Nakanishi, Dr. Hisashi Tanaka, Dr. Nobuhiko Sugano, and Dr. Takashi Nishii at Osaka University for providing hip joint MR data and phantom MR data. The author also thanks all the above researchers and Prof. Shinicni Tamura at Osaka University for fruitful discussion.
Questions
1.Summarize a series of procedures for multiscale enhancement filtering described in this chapter from input original images to output final filterenhanced images.
2.Explain the parameters involved in the procedures and discuss how to select these parameters.
3.Derive the mathematical formula of the width response curves shown in Fig. 10.3.
4.Discuss the effect of the anisotropic resolution (voxel shape) of input volume data on multiscale enhancement filtering.

Hessian-Based Multiscale Enhancement and Segmentation |
585 |
Bibliography
[1]Knutsson, H., Representing local structure using tensors, In: Proceedings of 6th Scandinavian Conference on Image Analysis, 1989, pp. 244– 251.
[2]Westin, C.-F., A Tensor Framework for Multidimensional Signal Processings, Ph.D. Thesis No. 348, Linkoping¨ University, Sweden, 1994.
[3]Koller, T . M., Gerig, G., Szekely, G., and Dettwiler, D., Multiscale detection of curvilinear structures in 2-D and 3-D image data, In: Proceedings of Fifth International Conference on Computer Vision, 1995, pp. 864– 869.
[4]Aylward, S., Bullitt, E., Pizer, S., and Eberly, D., Intensity ridge and widths for tubular object segmentation and description, In: Proceedings of IEEE Workshop on Mathematical Methods in Biomedical Image Analysis, 1996, pp. 131–138.
[5]Sato, Y., Nakajima, S., Atsumi, H., Koller, T., Gerig, G., Yoshida, S., and Kikinis, K., 3D multi-scale line filter for segmentation and visualization of curvilinear structures in medical images, In: Lecture Notes in Computer Science, Vol. 1205, pp. 213–222, 1997. Proceedings of CVRMedMRCAS’97, Glenoble, France.
[6]Lorenz, C., Carlsen, I.-C., Buzug, T. M., Fassnacht, C., and Wesse, J., Multi-scale line segmentation with automatic estimation of width, contrast and tangential direction in 2D and 3D medical images, In: Lecture Notes in Computer Science, Vol. 1205, pp. 233–242, 1997. Proceedings of CVRMed-MRCAS’97, Glenoble, France.
[7]Sato, Y., Nakajima, S., Shiraga, N., Atsumi, H., Yoshida, S., Koller, T., Guido, G., and Kikinis, R., Three-dimensional multi-scale line filter for segmentation and visualization of curvilinear structures in medical images, Med. Image Anal., Vol. 2, No. 2, pp. 143–168, 1998.
[8]Frangi, A., Niessen, W., Vincken, K., and Viergever, M., Multiscale vessel enhancement filtering, Vol. 1426 In: Proceedings of MICCAI’98, Boston, Massachusetts, 1998, pp. 130–137.
586 |
Sato |
[9]Westin, C.-F., Warfield, S., Bhalerao, A., Mui, L., Richolt, J., and Kikinis, R., Tensor controlled local structure enhancement of CT images for bone segmentation, In: Lecture Notes in Computer Science, Vol. 1426, pp. 1205–1212, 1998. Proceedings of MICCAI’98, Boston, Massachusetts.
[10]Sato, Y., Kubota T., Nakanishi K., Sugano N., Nishii T., Ohzono K., Nakamura H., Ochi O., and Tamura S., Three-dimensional reconstruction and quantification of hip joint cartilages from magnetic resonance images, In: Lecture Notes in Computer Science, Vol. 1679, pp. 338–347, 1999. Proceedings of MICCAI’99, Cambridge, UK.
[11]Sato, Y., Westin, C.-F., Bhalerao, A., Nakajima, S., Shiraga, N., Tamura, S., and Kikinis, R., Tissue classification based on 3D local intensity structures for volume rendering, IEEE Trans. Visual. Comput. Graphics, Vol. 6, No. 2, pp. 160–180, 2000.
[12]Sato, Y. and Tamura S., Detection and quantification of line and sheet structures in 3-D images, In: Lecture Notes in Computer Sceinece, Vol. 1935, pp. 164–165, 2000. Proceedings of MICCAI 2000, Pittsburgh, Pennsylvania.
[13]Aylward, S. R. and Bullitt, E., Initialization, noise, singularities, and scale in height ridge traversal for tubular object centerline extraction, IEEE Trans. Med. Imaging, Vol. 21, No. 2, pp. 61–75, 2002.
[14]Suri, J. S., Liu, K., Reden L., and Laxminarayan, S. N., White and black blood volumetric angiographic filtering: Ellipsoidal scale-space approach, IEEE Trans. Inform. Tech. Biomed., Vol. 6, No. 2, pp. 142–158, 2002.
[15]Suri, J. S., Liu, K., Reden L., and Laxminarayan, S. N., A review on MR vascular image processing algorithms: Acquisition and prefiltering, Part I, IEEE Trans. Inform. Tech. Biomed., Vol. 6, No. 4, pp. 324–337, 2002.
[16]Suri, J. S., Liu, K., Reden L., and Laxminarayan, S. N., A review on MR vascular image processing algorithms: Skeleton versus nonskeleton approaches, Part I, IEEE Trans. Inform. Tech. Biomed., Vol. 6, No. 4, pp. 338–350, 2002.
Hessian-Based Multiscale Enhancement and Segmentation |
587 |
[17]Sato, Y., Nakanishi, K., Tanaka, H., Nishii, T., Sugano, N., Nakamura, H., Ochi, T., and Tamura, S., Limits to the accuracy of 3D thickness measurement in magnetic resonance images, In: Lecture Notes in Computer Science, Vol. 2208, pp. 803–810, 2001. Proceedings of MICCAI2001, Utrecht, The Netherlands.
[18]Sato, Y., Tanaka, H., Nishii, T., Nakanishi, K., Sugano, N., Kubota, T., Nakamura, H., Yoshikawa, H., Ochi, T., and Tamura, S., Limits on the accuracy of 3D thickness measurement in magnetic resonance images— Effects of voxel anisotropy, IEEE Trans. Med. Imaging, Vol. 22, No. 9, pp. 1076–1088, 2003.
[19]Haralick, R. M., Watson, L. T., and Laffey, T. J., The topographic primal sketch, Int. J. Robotic Res., Vol. 2, No. 1, pp. 50–72, 1983.
[20]Marr, D., Vision—A Computational Investigation into the Human Representation and Processing of Visual Information, W. H. Freeman, New York, 1982.
[21]Lindeberg, T., On scale selection for differential operators, Proc. 8th Scandinavian Conference on Image Analysis, pp. 857–866, 1993.
[22]Lindeberg, T., Feature detection with automatic scale selection, Int. J. Comput. Vision, Vol. 30, No. 2, pp. 77–116, 1998.
[23]Lindeberg, T., Edge Detection and ridge detection with Automatic Scale Selection, Int. J. Comput. Vision, Vol. 30, No. 2, pp. 117–154, 1998.
[24]Hylton, N. M., Simovsky, I., Li, A . J., and Hale, J . D., Impact of section doubling on MR angiography, Radiology, Vol. 185, No. 3, pp. 899–902, 1992.
[25]Du, Y. P., Parker, D. L., Davis, W. L., and Cao, G., Reduction of partialvolume artifacts with zero-filled interpolation in three-dimensional MR angiography, J. Magn. Reson. Imaging, Vol. 4, No. 5, pp. 733–741, 1995.
[26]Kikinis, R., Gleason, P. L., Moriarty, T. M., Moore, M. R., Alexander, E., III, Stieg, P. E., Matsumae, M., Lorensen, W. E., Cline, H. E., Black, P. M., Jolesz, F. A., Computer-assisted interactive three-dimensional planning
588 |
Sato |
for neurosurgical procedures, Neurosurgery, Vol. 38, No. 4, pp. 640–651,
1996.
[27]Nakajima, S., Atsumi, H., Kikinis, R., Moriarty, T. M., Metcalf, D. C., Jolesz, F. A., and Black, P. M., Use of cortical surface vessel registration for image-guided neurosurgery, Neurosurgery, Vol. 40, No. 6, pp. 1201– 1210, 1997.
[28]Levoy, M., Display of surfaces from volume data, IEEE Comput. Graphics Appl., Vol. 8, No. 3, pp. 29–37, 1988.
[29]Lacroute, P. and Levoy M., Fast volume rendering using a shearwarp factorization of the viewing transform, In: Proceedings of SIGGRAPH’94, 1994, pp. 451–458.
[30]Vandarbrug, S. J., Semilinear line detectors, Comput. Graphics Image Process., Vol. 4, pp. 287–293, 1975.
[31]Burt, P. J. and Adelson E. H., The Laplacian pyramid as a compact image code, IEEE Trans. Commun., Vol. 31, No. 4, pp. 532–540, 1983.
[32]Shiraga, N., Sato, Y., Kohda, E., Okada, Y., Sato, K., Hasebe, T., Hiramatsu, K., Kikinis, R., and Jolesz, F. A., Three dimensional display of the osteosclerotic lesion by volume rendering method, Nippon Acta Radiol. Vol. 58, No. 2, p. S84, 1998.
[33]Shimizu, A., Hasegawa, J., and Toriwaki J., Minimum directional difference filter for extraction of circumscribed shadows in chest X-ray images and its characteristics, IEICE Trans., Vol. J-76D-II, No. 2, pp. 241– 249, 1993.
[34]Giger, M. L., Bae, K. T., and MacMahon, H., Computerized detection of pulmonary nodules in computed tomography images, Invest. Radiol., Vol. 24, No. 4, pp. 459–465, 1994.
[35]Kanazawa, K., Kubo, M., Niki, N., Satoh, H., Ohmatsu, H., Eguchi, K., and Moriyama, N., Computer aided screening system for lung cancer based on helical CT images, In: Lecture Notes in Computer Science, Vol. 1131, pp. 223–228, 1996. Proceedings of Visualization in Biomedical Computing, Hamburg, Germany.
Hessian-Based Multiscale Enhancement and Segmentation |
589 |
[36]Steger, C., An unbiased detector of curvilinear structures, IEEE Trans. Patt. Anal. Machine Intell., Vol. 20, No. 2, pp. 113–125, 1998.
[37] Nakanishi, N., Tanaka, H., Nishii, T., Masuhara, K., Narumi, Y., and Nakamura, H., MR evaluation of the articular cartilage of the femoral head during traction, Acta Radiol., Vol. 40, No. 1, pp. 60–63, 1999.
[38]Nakanishi, K., Tanaka, H., Sugano, N., Sato, Y., Ueguchi, T., Kubota, T., Tamura, S., and Nakamura, H., MR-based three-dimensional presentation of cartilage thickness in the femoral head, Euro. Radiol., Vol. 11, No. 11, pp. 2178–2183, 2001.
[39]Parker, D. L., Du, Y. P., and Davis, W. L., The voxel sensitivity function in Fourier transform imaging: applications to magnetic resonance angiography, Magn. Reson. Med., Vol. 33, No. 2, pp. 156–162, 1995.
[40]Hoogeveen, R. M., Bakker, C. J. G., and Viergever, M. A., Limits to the accuracy of vessel diameter measurement in MR angiography, J. Magn. Reson. Imaging, Vol. 8, No. 6, pp. 1228–1235, 1998.
[41]Steckner, M. C., Drost, D. J., and Prato, F. S., Computing the modulation transfer function of a magnetic resonance imager, Med. Phy., Vol. 21, No. 3, pp. 483–489, 1994.
[42]Canny, J., A computational approach to edge detection, IEEE Trans. Patt. Anal. Machine Intell., Vol. 8, No. 6, pp. 679–698, 1986.
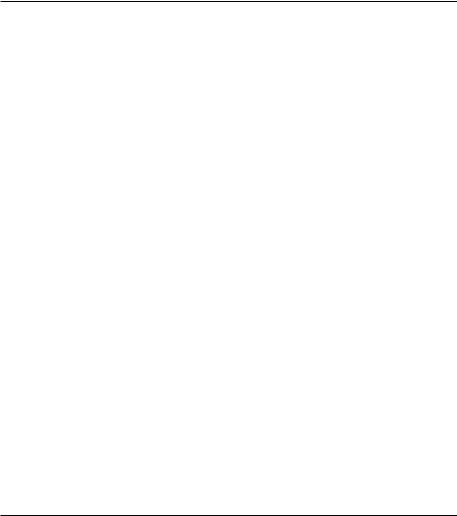
592 |
Singh and Bovis |
based on the properties of the image under consideration, predict the single best algorithm to be applied at each layer from this set. We demonstrate that this scheme of work has significant advantages over a nonadaptive structure (where only one algorithm is available per layer and it is fixed for all images in the dataset).
We aim to answer the following questions: (a) What is a knowledge-based framework? We discuss the components of this framework in section 11.2 putting it in the context of previous research. (b) How does the image enhancement layer work in this framework? This is detailed in section 11.3 where we discuss measures of image viewability based on enhancement, and demonstrate the role of good enhancement in image segmentation. We also propose two new mapping schemes that can map the image features to chosen enhancement methods. (c) How does the image segmentation layer work within the knowledgebased framework? In section 11.4 we detail the implementation of sophisticated Gaussian mixture models in both supervised and unsupervised modes, with an expert combination framework and compare them on overlap measures.
(d) What are the different strategies for reducing false positives? In section 11.5 we discuss several postprocessing steps that are aimed at reducing the number of false positives per image. (e) Is the adaptive knowledgebased framework superior to a nonadaptive scheme that uses the same algorithms across all images uniformly? We discuss our results on this issue in section 11.6 where we show the relative superiority of the adaptive framework.
11.2 Knowledge-Based Framework
The CAD scheme detailed in this chapter is based on an adaptive framework. An adaptive framework is capable of modifying itself such that it is more suitable to the environment within which it operates. Within the context of CAD, an adaptable component or a framework, attempts to automatically optimize the lesion detection process for a given mammogram. Broadly speaking, an adaptive characteristic can be built into CAD in three different ways: (1) Using a deterministic component; (2) knowledge-based component; (3) with a knowledge-based framework. Each approach may be used in combination with the others. These approaches are summarized below.