
Kluwer - Handbook of Biomedical Image Analysis Vol
.2.pdfA Knowledge-Based Scheme for Digital Mammography |
653 |
the inclusion of the 200 normal mammograms compared with the results presented in Table 11.16. The aim of the false-positive reduction knowledge-based component described is to reduce the false-positive count, while maintaining sensitivity in the detection of lesions. The next section describes how this is achieved in this configuration of the adaptive knowledge-based model.
11.6.2.3 Reduction of False-Positives
False positives are initially reduced by removing regions with an area less than a predefined threshold Tarea. We choose Tarea = 122 pixels, thus any region less than 5 mm in diameter is removed. This approach is used here. From the remaining suspicious regions, features are extracted, and using a trained ANN classifier, a region is labelled as abnormal or normal.
Feature extraction: For those regions that remain following the application of the area test, the 316-dimensional feature vector described in [42] is extracted using the pixels comprising the region. To improve classifier generalization [32] unbiased PCA is used to map the 316-dimensional feature vector into a lower dimensional feature space. PCA is used on a per breast type basis, so that the number of principal components is selected independently for each breast type. Using this approach, for each predicted breast type, the number of principal components selected are as follows: (type 1, 37 components; type 2, 33 components; type 3, 35 components; type 4, 41 components). From this table it can be seen that the highest dimensional feature space results from the densest breast types (type 4), which are the generally the hardest to interpret by an expert radiologist [37].
Model order selection: In order to maximize the performance of each ANN for each predicted breast type, model order selection of the ANN classifier is performed. By varying the number of hidden nodes and performing a classification on all suspicious region, ROC can be performed and the area under the ROC curve ( AZ ) computed. The optimal number of hidden nodes is determined as that maximising the AZ value.
11.6.2.4 Results
This section presents the results from applying the false-positive reduction methodology on the suspicious regions resulting from the knowledge-based

654 |
Singh and Bovis |
Table 11.19: Sensitivity and average number of false-positives per image for
200 abnormal and 200 normal images
|
|
|
|
|
After segmentation |
After falsepositive |
|||||
|
|
After segmentation |
|
prefiltering |
|
reduction (OP) |
|||||
|
|
|
|
|
|
|
|
|
|
|
|
Breast type |
Sensitivity |
FP/i |
|
Sensitivity |
FP/i |
Sensitivity |
FP/i |
||||
|
|
|
|
|
|
|
|
||||
1 |
0.79 |
207.26 |
0.77 |
8.98 |
|
0.64 |
3.41 |
||||
2 |
0.80 |
162.48 |
0.80 |
10.95 |
0.70 |
7.05 |
|||||
3 |
0.96 |
161.86 |
0.89 |
7.96 |
|
0.89 |
7.76 |
||||
4 |
0.80 |
136.45 |
0.76 |
6.71 |
|
0.76 |
7.63 |
||||
Mean |
0.84 |
167.01 |
0.81 |
8.65 |
|
0.75 |
6.46 |
||||
|
|
|
|
|
|
|
|
|
|
|
|
Values after segmentation, after region prefiltering, and after false-positive reduction using optimized classifier at ROC operating point, each by breast type (FP/ i = average number of false-positive per image,
OP = operating point).
contrast enhancement and segmentation components. Table 11.19 shows the sensitivity in the detection of breast lesions and average number of false-positive regions over all mammograms of each predicted breast type. The first column of shows performance after segmentation, the second column shows the results after region prefiltering, and the final column shows the results following classification using the optimized ANN.
The aim of false-positive reduction is to reduce the average number of falsepositive regions per image while maintaining sensitivity levels. The prefiltering stage can be seen from the results as being very effective in reducing the falsepositive count. After region prefiltering sensitivity has dropped by just over 3.5% and the average number of false-positive regions to 8.65 for all 400 images. The mean sensitivity drops more sharply when reducing false-positive regions with the trained ANNs. The performance of each ANN is reported at the operating point on the ROC curve where the cost of a false positive and false negative are set equal (CFP = CFN = 1) and the a priori probability of a positive case is set,
P(D+) = 0.5. These costs and priors can be adjusted by the expert radiologist to reflect the required level of sensitivity. Optimization of their values is outside the scope of this study. The largest reduction in false-positive regions using the ANN is seen for the fatty, type 1 breasts. For the denser breasts, types 3 and 4, the operating point selected maintains the level of sensitivity but does not significantly reduce the false-positive count. In fact for the densest breasts, the false-positive count rises indicating the nontrivial nature of this classification problem.
A Knowledge-Based Scheme for Digital Mammography |
655 |
11.6.3 Key Observations
This section has presented a configuration of the adaptive knowledge-based model. The performance of the model has been evaluated on a dataset of 200 abnormal mammograms from four different breast types. The aim of the evaluation has been to demonstrate the utility of the model compared with that of individual experts. Following this, the performance of a specific configuration of the adaptive knowledge-based model has been evaluated on a dataset of 400 mammograms, comprising 200 abnormal and 200 normal images. From these evaluations, the following key observations can be made:
1.Utility of knowledge-based contrast enhancement component: Using a dataset of 200 abnormal mammograms, the utility of the knowledge-based contrast enhancement expert has been demonstrated to be greater than that of the best performing expert contrast enhancement method. Using the predicted optimal contrast enhancement method in image segmentation results in a 60% improvement in the detection of abnormal regions over the original segmentation. This is compared to a 54% improvement from the single best performing expert, the FUZZY contrast enhancement method.
2.Utility of knowledge-based segmentation component: By optimally combining the segmentation outcomes of 10 different segmentation experts, each operating on a unique feature space partition, the knowledge based segmentation component resulted in a mean ROC AZ value of 0.72 for 200 mammograms from four breast types. This is compared to the best performing gray-scale segmentation expert reporting a mean AZ value of 0.65.
3.Utility of adaptive knowledge-based model in presence of normal mammograms: Evaluation of the performance of this configuration of the adaptive knowledge-based model on a dataset of 400 mammograms comprising 200 abnormal and 200 normal images results in a segmentation sensitivity of 0.84 for the detection of breast lesion with 167.01 false-positive regions per image. This demonstrates a high level of sensitivity in the presence of a complete spectrum of mammogram types.
4.False-positive reduction: The results following region prefiltering in the false-positive reduction methodology demonstrate the utility of the region
656 |
Singh and Bovis |
size thresholding strategy. Following classification by each trained optimized ANN results in a sensitivity of 0.75 with 6.46 false-positive regions per image.
11.7 Conclusions
In this chapter we have presented a framework for adaptive selection of image processing components based on image properties. Throughout the study, we have evaluated the different components and the overall model on the same dataset in order to produce a consistent and comparable set of results. The framework presented here has generic applicability to medical imaging applications and we are confident that further research will involve such knowledgebased approaches.
Questions
1.What is the essence of the chapter?
2.What are the two main areas used in this chapter when it comes to X-ray breast imaging?
3.What are the different measures used for X-ray breast “contrast enhancement”? Discuss each of them.
4.Show how the knowledge-based system works for the contrast enhancement.
5.What is the role of image segmentation here and how it is done?
6.What is double network mapping (DNM)?
7.What is breast profile mapping (BPM)? Discuss in detail.
8.Discuss the weighted GMM/MRF model of segmentation of breast masses in X-ray images. State mathematically and then discuss the pseudo algo-
rithm.
9. Compare the four models W G M MS, W G M MU , W G M MSM RF , W G M MUM RF .
10. List some key observations in adaptive knowledge-based model.

A Knowledge-Based Scheme for Digital Mammography |
657 |
Bibliography
[1]Rangayyan, R. M. et al., Improvement of sensitivity of breast cancer diagnosis with adaptive neighbourhood contrast enhancement of mammograms, IEEE Trans. Inf. Tech. Biomed., Vol. 1, No. 3, pp. 161–169, 1997.
[2]Petrick, N. et al., Automated detection of breast masses on mammograms using adaptive contrast enhancement and texture classification, Med. Phys., Vol. 23, pp. 1685–1696, 1996.
[3]Li, L., Qian, W., and Clarke, L. P., Digital mammography: Computer assisted diagnosis method for mass detection with multiorientation and multiresolution wavelet transforms, Acad. Radiol., Vol. 4, No. 11, pp. 724–731, 1997.
[4]Sahiner, B. et al., Image feature selection by a genetic algorithm: Application to classification of mass and normal breast tissue, Med. Phys., Vol. 23, No. 10, pp. 1671–1683, 1996.
[5]Sahiner, B. et al., Computer-aided characterisation of mammographic masses: Accuracy of mass segmentation and its effects on characterisation, IEEE Trans. Med. Imaging, Vol. 20, No. 12, pp. 1275–1284, 2001.
[6]Polakowski, W. E. et al., Computer aided breast cancer detection and diagnosis of masses using difference of Gaussians and derivativebased feature saliency, IEEE Trans. Med. Imaging, Vol. 16, pp. 811–819, 1997.
[7]Yin, F. F. et al., Comparison of bilateral subtraction and single image processing techniques in the computerised detection of mammographic masses, Invest. Radiol., Vol. 28, No. 6, pp. 473–481, 1993.
[8]Singh, S. and Al-Mansoori, R., Identification of region of interest in digital mammograms, J. Intell. Syst., Vol. 10, No. 2, pp. 183–210, 2000.
[9]Zheng, B., Chang, Y., and Gur, D., Adaptive computer-aided diagnosis scheme of digitised mammograms, Acad. Radiol., vol. 3, pp. 806–814, 1996.
658 |
Singh and Bovis |
[10]Matsubara, T. et al., Development of new schemes for detection and analysis of mammographic masses, In: Proceedings of the International Conference on Intelligent Information Systems, 1997.
[11]Lai, S. and Fang, M., Adaptive medical image visualisation based on hierarchical neural networks and intelligent decision fusion, In: Proceedings of IEEE Signal Processing Society Workshop, pp. 438–447, 1998.
[12]Lai, S. and Fang, M., A hierarchical neural network algorithm for robust and automatic windowing of MR images, Artif. Intell. Med., Vol. 19,
pp.97–119, 2000.
[13]Pitiot, A., Toga, A. W., Ayache, N., and Thompson, P., Texture based MRI segmentation with a two stage hybrid neural classifier, In: Proceedings of IEEE IJCNN Conference, 2002, Vol. 3, pp. 2053–2058.
[14]Sha, D. D. and Sutton, J. P., Towards automated enhancement, segmentation and classification of digital brain images using networks of networks, Inf. Sci., Vol. 138, pp. 45–77, 2001.
[15]Fenster, S. D. and Kender, J. R., Sectored snakes: Evaluating learnedenergy segmentations, IEEE Trans. Med. Imaging, Vol. 23, No. 9,
pp.1028–2034, 2001.
[16]Perner, P., An architecture for a CBR image segmentation system, Eng. Appl. Artif. Intell., Vol. 12, No. 6, pp. 749–759, 1999.
[17]Guan, L., Anderson, J. A., and Sutton, J. P., A network of networks processing model for image regularisation, IEEE Trans. Neural Networks, Vol. 8, No. 1, pp. 169–174, 1997.
[18]Bovis, K. J., An Adaptive Knowledge-Based Model for Detecting Masses in Screening Mammograms, Ph.D. Thesis, Department of Computer Science, University of Exeter, 2003.
[19]Singh, S. and Bovis, K. J., Digital mammography segmentation, In: Advanced Algorithmic Approach to Medical Image Segmentation: State- of-the-Art Application in Cardiology, Neurology, Mammography and Pathology, Suri, J., Setarehdan, S. K., and Singh, S., eds., Springer-Verlag, Berlin, pp. 440–540, 2001.
A Knowledge-Based Scheme for Digital Mammography |
659 |
[20]Bovis, K. J. and Singh, S., Enhancement technique evaluation using quantitative measures on digital mammograms, In: Proceedings of the 5th International Workshop on Digital Mammography, Toronto, Canada, Yaffe, M. J., ed., Medical Physics Publishing, pp. 547–553, 2000.
[21]Weszka, J. S. and Rosenfeld, A., A comparison study of texture measures for terrain classification, In: Proceedings of Conference in Computer Graphics, 1975, pp. 62–64.
[22]Hair, J., Anderson, R., and Tatham, R., Multivariate data analysis, 1998.
[23]Webb, A., Statistical Pattern Recognition, Arnold, 1999.
[24]Zhang, Y., Brady, M., and Smith, S., Segmentation of brain MR images through a hidden Markov random field model and the expectation minimisation algorithm, IEEE Trans. Med. Imaging, Vol. 20, No. 1, pp. 45–57, 2001.
[25]Kallergi, M., Carney, G. M., and Gaviria, J., Evaluating the performance of detection algorithms in digital mammography, Med. Phys., Vol. 26, No. 2, pp. 267–275, 1999.
[26]Bovis, K. J. and Singh, S., Classification of mammographic breast density using a combined classifier paradigm, In: Medical Image Understanding and Analysis (MIUA) Conference, Portsmouth, July 22–23, 2002.
[27]Duda, R. O., Hart, P. E., and Stork, D. G., Pattern Classification, Wiley, Neq York, 2001.
[28]Sonka, M., Hlavac, V., and Boyle, R., Image Processing, Analysis and Machine Vision, PSW Publishing, 1999.
[29]Besag, J., On the statistical analysis of dirty pictures, J. Roy. Soc. B, Vol. 48, No. 3, pp. 259–302, 1986.
[30]Dubes, R. C. and Jain, A. K., Random field models in image analysis, J. Appl. Stat., Vol. 16, No. 2, pp. 131–163, 1989.
[31]Geman, S. and Geman, D., Stochastic relaxation Gibbs distributions and Bayesian restoration of image, IEEE Trans. PAMI, Vol. 6, No.6, pp. 721–741, 1984.
660 |
Singh and Bovis |
[32]Bishop, C. M., Neural Networks for Pattern Recognition, Oxford University Press, 1995.
[33]Kittler, J., Combining classifiers: A theoretical framework, Patt. Anal. Appl., Vol. 1, No. 1, pp. 18–27, 1998.
[34]Jacobs, R. A. et. al., Adaptive mixtures of local experts, Neural Comput., Vol. 3, pp. 79–87, 1991
[35]Dempster, A. P., Laird, N. M., and Rubin, D. B., Maximum likelihood from incomplete data via the EM algorithm, J. Roy. Stat. Soc. B, Vol. 39, pp. 1–38, 1977.
[36]Yin, F. F. et al. Computerised detection of masses in digital mammograms: Analysis of bilateral subtraction images, Med. Phys., Vol. 18, No. 5, pp. 955–963, 1991.
[37]Kopans, D., Breast Imaging, Lippincott-Raven, 1998.
[38]Haralick et al., 1973.
[39]Wei et al., 1997.
[40]Metz, C. E., Basic principles of ROC analysis, Sem. Nuclear Med., Vol. 8, No. 4, pp. 283–298, 1978.
[41]Kupinski, M. A. and Anastasio, M. A., Multi-objective genetic optimisation of diagnostic classifiers with implications for generating receiver operating characteristic curves, IEEE Trans. Med. Imaging, Vol. 18, No. 8, pp. 675–685, 1999.
[42]Bovis, K. J. and Singh, S., Learning the optimal contrast enhancement of mammographic breast masses, In: Proceedings of the 6th International Workshop on Digital Mammography, Bremen, Germany, June, 22–25, 2002, Springer, Berlin, pp. 179–181, 2002.
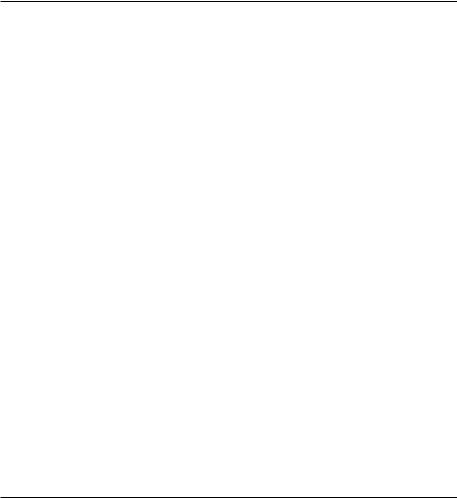
662 |
Herman and Carvalho |
algorithms perform the whole segmentation process without any user intervention, usually by obtaining all information necessary to perform the segmentation from prior knowledge about the class of problems to which the segmentation at hand belongs.
Algorithms can be also be classified according to how they solve the segmentation problem. Point-based algorithms make a local decision about a point’s membership to an object. This decision can be based solely on the point’s brightness value or on the brightness values of a small neighborhood surrounding the point. A widely used and very simple point-based segmentation algorithm is thresholding, where a user selects one or two brightness values that are interpreted as lower and/or upper values of the brightness of the object to be segmented. Then, all pixels whose values are in the specified brightness range are considered to be part of the object. It is easy to see that algorithms of this type are very sensitive to noise, to inhomogeneous illumination, and are not appropriate for segmenting textured objects.
Edge-based segmentation algorithms usually work in two steps by first detecting edges in the image and then grouping or linking them into boundaries of objects based on the orientation of the edges and on prior knowledge regarding the expected shape of objects. Common edge detection procedures include the use of gradient operators, Laplacians or the Canny edge detector [1], while edge linking can be performed locally by searching small local pixel neighborhoods or globally by making use of the Hough Transform [2], for example. Other edgebased segmentation algorithms use active contour models, such as snakes [3] or balloons [4]. Snakes are energy-minimizing splines guided by external constraint forces and pushed by image forces (edges) toward image features, while balloons use image forces to stop their inflated curve models on image features. There are also global optimization algorithms [5–7] that segment images by minimizing various energy functions defined in terms of pixel labels and prior knowledge.
Region-based algorithms are subdivided into region growing and split-and- merge algorithms. Region growing algorithms, as the name suggests, start with preselected seed points forming the initial regions that grow according to some predefined rules until the whole image is labeled. Split-and-merge algorithms begin by subdividing an image into arbitrary disjoint regions, and then split and/or merge them repeatedly until some preset conditions are satisfied. The methods of balloons [4, 8] and level sets [9, 10] can also be considered region