
- •List of Tables
- •List of Figures
- •Table of Notation
- •Preface
- •Boolean retrieval
- •An example information retrieval problem
- •Processing Boolean queries
- •The extended Boolean model versus ranked retrieval
- •References and further reading
- •The term vocabulary and postings lists
- •Document delineation and character sequence decoding
- •Obtaining the character sequence in a document
- •Choosing a document unit
- •Determining the vocabulary of terms
- •Tokenization
- •Dropping common terms: stop words
- •Normalization (equivalence classing of terms)
- •Stemming and lemmatization
- •Faster postings list intersection via skip pointers
- •Positional postings and phrase queries
- •Biword indexes
- •Positional indexes
- •Combination schemes
- •References and further reading
- •Dictionaries and tolerant retrieval
- •Search structures for dictionaries
- •Wildcard queries
- •General wildcard queries
- •Spelling correction
- •Implementing spelling correction
- •Forms of spelling correction
- •Edit distance
- •Context sensitive spelling correction
- •Phonetic correction
- •References and further reading
- •Index construction
- •Hardware basics
- •Blocked sort-based indexing
- •Single-pass in-memory indexing
- •Distributed indexing
- •Dynamic indexing
- •Other types of indexes
- •References and further reading
- •Index compression
- •Statistical properties of terms in information retrieval
- •Dictionary compression
- •Dictionary as a string
- •Blocked storage
- •Variable byte codes
- •References and further reading
- •Scoring, term weighting and the vector space model
- •Parametric and zone indexes
- •Weighted zone scoring
- •Learning weights
- •The optimal weight g
- •Term frequency and weighting
- •Inverse document frequency
- •The vector space model for scoring
- •Dot products
- •Queries as vectors
- •Computing vector scores
- •Sublinear tf scaling
- •Maximum tf normalization
- •Document and query weighting schemes
- •Pivoted normalized document length
- •References and further reading
- •Computing scores in a complete search system
- •Index elimination
- •Champion lists
- •Static quality scores and ordering
- •Impact ordering
- •Cluster pruning
- •Components of an information retrieval system
- •Tiered indexes
- •Designing parsing and scoring functions
- •Putting it all together
- •Vector space scoring and query operator interaction
- •References and further reading
- •Evaluation in information retrieval
- •Information retrieval system evaluation
- •Standard test collections
- •Evaluation of unranked retrieval sets
- •Evaluation of ranked retrieval results
- •Assessing relevance
- •A broader perspective: System quality and user utility
- •System issues
- •User utility
- •Results snippets
- •References and further reading
- •Relevance feedback and query expansion
- •Relevance feedback and pseudo relevance feedback
- •The Rocchio algorithm for relevance feedback
- •Probabilistic relevance feedback
- •When does relevance feedback work?
- •Relevance feedback on the web
- •Evaluation of relevance feedback strategies
- •Pseudo relevance feedback
- •Indirect relevance feedback
- •Summary
- •Global methods for query reformulation
- •Vocabulary tools for query reformulation
- •Query expansion
- •Automatic thesaurus generation
- •References and further reading
- •XML retrieval
- •Basic XML concepts
- •Challenges in XML retrieval
- •A vector space model for XML retrieval
- •Evaluation of XML retrieval
- •References and further reading
- •Exercises
- •Probabilistic information retrieval
- •Review of basic probability theory
- •The Probability Ranking Principle
- •The 1/0 loss case
- •The PRP with retrieval costs
- •The Binary Independence Model
- •Deriving a ranking function for query terms
- •Probability estimates in theory
- •Probability estimates in practice
- •Probabilistic approaches to relevance feedback
- •An appraisal and some extensions
- •An appraisal of probabilistic models
- •Bayesian network approaches to IR
- •References and further reading
- •Language models for information retrieval
- •Language models
- •Finite automata and language models
- •Types of language models
- •Multinomial distributions over words
- •The query likelihood model
- •Using query likelihood language models in IR
- •Estimating the query generation probability
- •Language modeling versus other approaches in IR
- •Extended language modeling approaches
- •References and further reading
- •Relation to multinomial unigram language model
- •The Bernoulli model
- •Properties of Naive Bayes
- •A variant of the multinomial model
- •Feature selection
- •Mutual information
- •Comparison of feature selection methods
- •References and further reading
- •Document representations and measures of relatedness in vector spaces
- •k nearest neighbor
- •Time complexity and optimality of kNN
- •The bias-variance tradeoff
- •References and further reading
- •Exercises
- •Support vector machines and machine learning on documents
- •Support vector machines: The linearly separable case
- •Extensions to the SVM model
- •Multiclass SVMs
- •Nonlinear SVMs
- •Experimental results
- •Machine learning methods in ad hoc information retrieval
- •Result ranking by machine learning
- •References and further reading
- •Flat clustering
- •Clustering in information retrieval
- •Problem statement
- •Evaluation of clustering
- •Cluster cardinality in K-means
- •Model-based clustering
- •References and further reading
- •Exercises
- •Hierarchical clustering
- •Hierarchical agglomerative clustering
- •Time complexity of HAC
- •Group-average agglomerative clustering
- •Centroid clustering
- •Optimality of HAC
- •Divisive clustering
- •Cluster labeling
- •Implementation notes
- •References and further reading
- •Exercises
- •Matrix decompositions and latent semantic indexing
- •Linear algebra review
- •Matrix decompositions
- •Term-document matrices and singular value decompositions
- •Low-rank approximations
- •Latent semantic indexing
- •References and further reading
- •Web search basics
- •Background and history
- •Web characteristics
- •The web graph
- •Spam
- •Advertising as the economic model
- •The search user experience
- •User query needs
- •Index size and estimation
- •Near-duplicates and shingling
- •References and further reading
- •Web crawling and indexes
- •Overview
- •Crawling
- •Crawler architecture
- •DNS resolution
- •The URL frontier
- •Distributing indexes
- •Connectivity servers
- •References and further reading
- •Link analysis
- •The Web as a graph
- •Anchor text and the web graph
- •PageRank
- •Markov chains
- •The PageRank computation
- •Hubs and Authorities
- •Choosing the subset of the Web
- •References and further reading
- •Bibliography
- •Author Index
140 |
7 Computing scores in a complete search system |
with the highest values for g(d) + tf-idft,d. The list itself is, like all the postings lists considered so far, sorted by a common order (either by document IDs or by static quality). Then at query time, we only compute the net scores (7.2) for documents in the union of these global champion lists. Intuitively, this has the effect of focusing on documents likely to have large net scores.
We conclude the discussion of global champion lists with one further idea. We maintain for each term t two postings lists consisting of disjoint sets of documents, each sorted by g(d) values. The first list, which we call high, contains the m documents with the highest tf values for t. The second list, which we call low, contains all other documents containing t. When processing a query, we first scan only the high lists of the query terms, computing net scores for any document on the high lists of all (or more than a certain number of) query terms. If we obtain scores for K documents in the process, we terminate. If not, we continue the scanning into the low lists, scoring documents in these postings lists. This idea is developed further in Section 7.2.1.
7.1.5Impact ordering
In all the postings lists described thus far, we order the documents consistently by some common ordering: typically by document ID but in Section 7.1.4 by static quality scores. As noted at the end of Section 6.3.3, such a common ordering supports the concurrent traversal of all of the query terms’ postings lists, computing the score for each document as we encounter it. Computing scores in this manner is sometimes referred to as document-at-a- time scoring. We will now introduce a technique for inexact top-K retrieval in which the postings are not all ordered by a common ordering, thereby precluding such a concurrent traversal. We will therefore require scores to be “accumulated” one term at a time as in the scheme of Figure 6.14, so that we have term-at-a-time scoring.
The idea is to order the documents d in the postings list of term t by
decreasing order of tft,d. Thus, the ordering of documents will vary from one postings list to another, and we cannot compute scores by a concurrent traversal of the postings lists of all query terms. Given postings lists ordered
by decreasing order of tft,d, two ideas have been found to significantly lower the number of documents for which we accumulate scores: (1) when traversing the postings list for a query term t, we stop after considering a prefix of the postings list – either after a fixed number of documents r have been
seen, or after the value of tft,d has dropped below a threshold; (2) when accumulating scores in the outer loop of Figure 6.14, we consider the query terms in decreasing order of idf, so that the query terms likely to contribute the most to the final scores are considered first. This latter idea too can be adaptive at the time of processing a query: as we get to query terms with lower idf, we can determine whether to proceed based on the changes in
Online edition (c) 2009 Cambridge UP
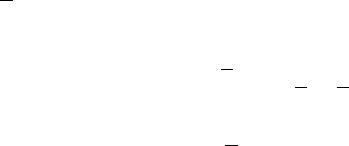
7.1 Efficient scoring and ranking |
141 |
document scores from processing the previous query term. If these changes are minimal, we may omit accumulation from the remaining query terms, or alternatively process shorter prefixes of their postings lists.
These ideas form a common generalization of the methods introduced in Sections 7.1.2–7.1.4. We may also implement a version of static ordering in which each postings list is ordered by an additive combination of static and query-dependent scores. We would again lose the consistency of ordering across postings, thereby having to process query terms one at time accumulating scores for all documents as we go along. Depending on the particular scoring function, the postings list for a document may be ordered by other quantities than term frequency; under this more general setting, this idea is known as impact ordering.
7.1.6Cluster pruning
In cluster pruning we have a preprocessing step during which we cluster the document vectors. Then at query time, we consider only documents in a small number of clusters as candidates for which we compute cosine scores. Specifically, the preprocessing step is as follows:
1.Pick √N documents at random from the collection. Call these leaders.
2.For each document that is not a leader, we compute its nearest leader.
We refer to documents that are not leaders as followers. Intuitively, in the partition of the followers induced by the use of √N randomly chosen leaders, the expected number of followers for each leader is ≈ N/√N = √N. Next, query processing proceeds as follows:
1.Given a query q, find the leader L that is closest to q. This entails computing cosine similarities from q to each of the √N leaders.
2.The candidate set A consists of L together with its followers. We compute the cosine scores for all documents in this candidate set.
The use of randomly chosen leaders for clustering is fast and likely to reflect the distribution of the document vectors in the vector space: a region of the vector space that is dense in documents is likely to produce multiple leaders and thus a finer partition into sub-regions. This illustrated in Figure 7.3.
Variations of cluster pruning introduce additional parameters b1 and b2, both of which are positive integers. In the pre-processing step we attach each follower to its b1 closest leaders, rather than a single closest leader. At query time we consider the b2 leaders closest to the query q. Clearly, the basic scheme above corresponds to the case b1 = b2 = 1. Further, increasing b1 or
Online edition (c) 2009 Cambridge UP

142 |
7 Computing scores in a complete search system |
Figure 7.3 Cluster pruning.
b2 increases the likelihood of finding K documents that are more likely to be in the set of true top-scoring K documents, at the expense of more computation. We reiterate this approach when describing clustering in Chapter 16 (page 354).
?Exercise 7.1
We suggested above (Figure 7.2) that the postings for static quality ordering be in
decreasing order of g(d). Why do we use the decreasing rather than the increasing order?
Exercise 7.2
When discussing champion lists, we simply used the r documents with the largest tf values to create the champion list for t. But when considering global champion lists,
we used idf as well, identifying documents with the largest values of g(d) + tf-idft,d. Why do we differentiate between these two cases?
Exercise 7.3
If we were to only have one-term queries, explain why the use of global champion lists with r = K suffices for identifying the K highest scoring documents. What is a simple modification to this idea if we were to only have s-term queries for any fixed integer s > 1?
Exercise 7.4
Explain how the common global ordering by g(d) values in all high and low lists helps make the score computation efficient.
Online edition (c) 2009 Cambridge UP