
- •List of Tables
- •List of Figures
- •Table of Notation
- •Preface
- •Boolean retrieval
- •An example information retrieval problem
- •Processing Boolean queries
- •The extended Boolean model versus ranked retrieval
- •References and further reading
- •The term vocabulary and postings lists
- •Document delineation and character sequence decoding
- •Obtaining the character sequence in a document
- •Choosing a document unit
- •Determining the vocabulary of terms
- •Tokenization
- •Dropping common terms: stop words
- •Normalization (equivalence classing of terms)
- •Stemming and lemmatization
- •Faster postings list intersection via skip pointers
- •Positional postings and phrase queries
- •Biword indexes
- •Positional indexes
- •Combination schemes
- •References and further reading
- •Dictionaries and tolerant retrieval
- •Search structures for dictionaries
- •Wildcard queries
- •General wildcard queries
- •Spelling correction
- •Implementing spelling correction
- •Forms of spelling correction
- •Edit distance
- •Context sensitive spelling correction
- •Phonetic correction
- •References and further reading
- •Index construction
- •Hardware basics
- •Blocked sort-based indexing
- •Single-pass in-memory indexing
- •Distributed indexing
- •Dynamic indexing
- •Other types of indexes
- •References and further reading
- •Index compression
- •Statistical properties of terms in information retrieval
- •Dictionary compression
- •Dictionary as a string
- •Blocked storage
- •Variable byte codes
- •References and further reading
- •Scoring, term weighting and the vector space model
- •Parametric and zone indexes
- •Weighted zone scoring
- •Learning weights
- •The optimal weight g
- •Term frequency and weighting
- •Inverse document frequency
- •The vector space model for scoring
- •Dot products
- •Queries as vectors
- •Computing vector scores
- •Sublinear tf scaling
- •Maximum tf normalization
- •Document and query weighting schemes
- •Pivoted normalized document length
- •References and further reading
- •Computing scores in a complete search system
- •Index elimination
- •Champion lists
- •Static quality scores and ordering
- •Impact ordering
- •Cluster pruning
- •Components of an information retrieval system
- •Tiered indexes
- •Designing parsing and scoring functions
- •Putting it all together
- •Vector space scoring and query operator interaction
- •References and further reading
- •Evaluation in information retrieval
- •Information retrieval system evaluation
- •Standard test collections
- •Evaluation of unranked retrieval sets
- •Evaluation of ranked retrieval results
- •Assessing relevance
- •A broader perspective: System quality and user utility
- •System issues
- •User utility
- •Results snippets
- •References and further reading
- •Relevance feedback and query expansion
- •Relevance feedback and pseudo relevance feedback
- •The Rocchio algorithm for relevance feedback
- •Probabilistic relevance feedback
- •When does relevance feedback work?
- •Relevance feedback on the web
- •Evaluation of relevance feedback strategies
- •Pseudo relevance feedback
- •Indirect relevance feedback
- •Summary
- •Global methods for query reformulation
- •Vocabulary tools for query reformulation
- •Query expansion
- •Automatic thesaurus generation
- •References and further reading
- •XML retrieval
- •Basic XML concepts
- •Challenges in XML retrieval
- •A vector space model for XML retrieval
- •Evaluation of XML retrieval
- •References and further reading
- •Exercises
- •Probabilistic information retrieval
- •Review of basic probability theory
- •The Probability Ranking Principle
- •The 1/0 loss case
- •The PRP with retrieval costs
- •The Binary Independence Model
- •Deriving a ranking function for query terms
- •Probability estimates in theory
- •Probability estimates in practice
- •Probabilistic approaches to relevance feedback
- •An appraisal and some extensions
- •An appraisal of probabilistic models
- •Bayesian network approaches to IR
- •References and further reading
- •Language models for information retrieval
- •Language models
- •Finite automata and language models
- •Types of language models
- •Multinomial distributions over words
- •The query likelihood model
- •Using query likelihood language models in IR
- •Estimating the query generation probability
- •Language modeling versus other approaches in IR
- •Extended language modeling approaches
- •References and further reading
- •Relation to multinomial unigram language model
- •The Bernoulli model
- •Properties of Naive Bayes
- •A variant of the multinomial model
- •Feature selection
- •Mutual information
- •Comparison of feature selection methods
- •References and further reading
- •Document representations and measures of relatedness in vector spaces
- •k nearest neighbor
- •Time complexity and optimality of kNN
- •The bias-variance tradeoff
- •References and further reading
- •Exercises
- •Support vector machines and machine learning on documents
- •Support vector machines: The linearly separable case
- •Extensions to the SVM model
- •Multiclass SVMs
- •Nonlinear SVMs
- •Experimental results
- •Machine learning methods in ad hoc information retrieval
- •Result ranking by machine learning
- •References and further reading
- •Flat clustering
- •Clustering in information retrieval
- •Problem statement
- •Evaluation of clustering
- •Cluster cardinality in K-means
- •Model-based clustering
- •References and further reading
- •Exercises
- •Hierarchical clustering
- •Hierarchical agglomerative clustering
- •Time complexity of HAC
- •Group-average agglomerative clustering
- •Centroid clustering
- •Optimality of HAC
- •Divisive clustering
- •Cluster labeling
- •Implementation notes
- •References and further reading
- •Exercises
- •Matrix decompositions and latent semantic indexing
- •Linear algebra review
- •Matrix decompositions
- •Term-document matrices and singular value decompositions
- •Low-rank approximations
- •Latent semantic indexing
- •References and further reading
- •Web search basics
- •Background and history
- •Web characteristics
- •The web graph
- •Spam
- •Advertising as the economic model
- •The search user experience
- •User query needs
- •Index size and estimation
- •Near-duplicates and shingling
- •References and further reading
- •Web crawling and indexes
- •Overview
- •Crawling
- •Crawler architecture
- •DNS resolution
- •The URL frontier
- •Distributing indexes
- •Connectivity servers
- •References and further reading
- •Link analysis
- •The Web as a graph
- •Anchor text and the web graph
- •PageRank
- •Markov chains
- •The PageRank computation
- •Hubs and Authorities
- •Choosing the subset of the Web
- •References and further reading
- •Bibliography
- •Author Index
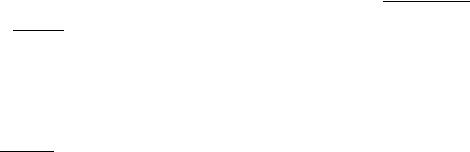
128 |
|
|
|
|
|
|
6 Scoring, term weighting and the vector space model |
|
|
|
|
||||||||||
|
|
|
|
|
|
|
|
|
|
|
|
|
|
|
|
||||||
|
Term frequency |
Document frequency |
|
Normalization |
|
|
|
|
|||||||||||||
|
n (natural) |
tft,d |
|
|
|
n (no) |
1 |
|
|
|
|
|
n (none) |
1 |
|
|
|
|
|
||
|
l (logarithm) |
1 + log(tft,d) |
t (idf) |
log |
|
N |
|
c (cosine) |
1 |
|
|
|
|
||||||||
|
|
|
|
|
√ |
|
|
|
|||||||||||||
|
dft |
|
|
||||||||||||||||||
|
|
|
|
w12+w22+...+w2M |
|
|
|||||||||||||||
|
a (augmented) |
0.5 + |
|
0.5×tft,d |
|
p (prob idf) |
max |
{ |
0, log N−dft |
} |
u (pivoted |
1/u (Section 6.4.4) |
|||||||||
|
|
maxt(tft,d) |
|||||||||||||||||||
|
|
|
|
|
|
|
|
|
dft |
unique) |
|
|
|
|
|
|
|
||||
|
|
1 |
if tft d > 0 |
|
|
|
|
|
|
|
|
|
|
|
|
|
|
||||
|
|
|
|
|
|
|
|
|
|
|
|
|
α |
|
|
|
|||||
|
b (boolean) |
0 |
otherwise, |
|
|
|
|
|
|
|
b (byte size) |
1/CharLength |
|
, α |
< 1 |
||||||
|
L (log ave) |
|
1+log(tft,d) |
|
|
|
|
|
|
|
|
|
|
|
|
|
|
|
|
||
|
|
1+log(avet d(tft,d)) |
|
|
|
|
|
|
|
|
|
|
|
|
|
|
|
||||
|
|
|
|
|
|
|
|
|
|
|
|
|
|
|
|
|
|
Figure 6.15 SMART notation for tf-idf variants. Here CharLength is the number of characters in the document.
3. More generally, a document in which the most frequent term appears roughly as often as many other terms should be treated differently from one with a more skewed distribution.
6.4.3 Document and query weighting schemes
Equation (6.12) is fundamental to information retrieval systems that use any form of vector space scoring. Variations from one vector space scoring method
to another hinge on the specific choices of weights in the vectors ~ ( ) and
V d
~ ( ). Figure 6.15 lists some of the principal weighting schemes in use for
V q
each of ~ ( ) and ~ ( ), together with a mnemonic for representing a spe-
V d V q
cific combination of weights; this system of mnemonics is sometimes called SMART notation, following the authors of an early text retrieval system. The mnemonic for representing a combination of weights takes the form ddd.qqq where the first triplet gives the term weighting of the document vector, while the second triplet gives the weighting in the query vector. The first letter in each triplet specifies the term frequency component of the weighting, the second the document frequency component, and the third the form of normalization used. It is quite common to apply different normalization func-
tions to ~ ( ) and ~ ( ). For example, a very standard weighting scheme
V d V q
is lnc.ltc, where the document vector has log-weighted term frequency, no idf (for both effectiveness and efficiency reasons), and cosine normalization, while the query vector uses log-weighted term frequency, idf weighting, and cosine normalization.
Online edition (c) 2009 Cambridge UP
6.4.4
PIVOTED DOCUMENT LENGTH NORMALIZATION
6.4 Variant tf-idf functions |
129 |
Pivoted normalized document length
In Section 6.3.1 we normalized each document vector by the Euclidean length of the vector, so that all document vectors turned into unit vectors. In doing so, we eliminated all information on the length of the original document; this masks some subtleties about longer documents. First, longer documents will – as a result of containing more terms – have higher tf values. Second, longer documents contain more distinct terms. These factors can conspire to raise the scores of longer documents, which (at least for some information needs) is unnatural. Longer documents can broadly be lumped into two categories: (1) verbose documents that essentially repeat the same content – in these, the length of the document does not alter the relative weights of different terms; (2) documents covering multiple different topics, in which the search terms probably match small segments of the document but not all of it – in this case, the relative weights of terms are quite different from a single short document that matches the query terms. Compensating for this phenomenon is a form of document length normalization that is independent of term and document frequencies. To this end, we introduce a form of normalizing the vector representations of documents in the collection, so that the resulting “normalized” documents are not necessarily of unit length. Then, when we compute the dot product score between a (unit) query vector and such a normalized document, the score is skewed to account for the effect of document length on relevance. This form of compensation for document
length is known as pivoted document length normalization.
Consider a document collection together with an ensemble of queries for that collection. Suppose that we were given, for each query q and for each document d, a Boolean judgment of whether or not d is relevant to the query q; in Chapter 8 we will see how to procure such a set of relevance judgments for a query ensemble and a document collection. Given this set of relevance judgments, we may compute a probability of relevance as a function of document length, averaged over all queries in the ensemble. The resulting plot may look like the curve drawn in thick lines in Figure 6.16. To compute this curve, we bucket documents by length and compute the fraction of relevant documents in each bucket, then plot this fraction against the median document length of each bucket. (Thus even though the “curve” in Figure 6.16 appears to be continuous, it is in fact a histogram of discrete buckets of document length.)
On the other hand, the curve in thin lines shows what might happen with the same documents and query ensemble if we were to use relevance as prescribed by cosine normalization Equation (6.12) – thus, cosine normalization has a tendency to distort the computed relevance vis-à-vis the true relevance, at the expense of longer documents. The thin and thick curves crossover at a point p corresponding to document length ℓp, which we refer to as the pivot
Online edition (c) 2009 Cambridge UP

130 |
|
|
|
|
|
|
6 Scoring, term weighting and the vector space model |
|
|
Relevance |
|
|
|||||
|
|
|
6 |
|
|
|
|
|
|
|
|
|
|
||||
|
|
|
|
|
|
|
|
|
|
|
|
|
|
|
|
|
|
|
|
|
|
|
|
p |
|
|
|
|
|
|
|
|
|
|
|
|
|
|
|
|
|
|
||
|
|
|
|
|
|
|
|
|
|
|
|
|
|
|
|
|
|
|
|
|
|
|
|
|
|
|
|
|
|
|
|
|
|
||
|
|
|
|
|
|
|
|
|
|
|
|
|
|
|
|
||
|
|
|
|
|
|
Document length |
||
|
|
|
|
|
|
|
||
|
|
|
|
|
|
|
- |
|
|
|
|
|
|
|
ℓp |
|
|
|
Figure 6.16 Pivoted document length normalization. |
|||||||
|
|
|
|
|
|
|
|
|
length; dashed lines mark this point on the x− and y− axes. The idea of pivoted document length normalization would then be to “rotate” the cosine normalization curve counter-clockwise about p so that it more closely matches thick line representing the relevance vs. document length curve. As mentioned at the beginning of this section, we do so by using in Equa-
|
~ |
|
tion (6.12) a normalization factor for each document vector V(d) that is not |
|
the Euclidean length of that vector, but instead one that is larger than the Eu- |
|
clidean length for documents of length less than ℓp, and smaller for longer |
|
documents. |
|
~ |
|
To this end, we first note that the normalizing term for V(d) in the de- |
|
~ |
|
nominator of Equation (6.12) is its Euclidean length, denoted |V(d)|. In the |
|
simplest implementation of pivoted document length normalization, we use |
|
~ |
|
a normalization factor in the denominator that is linear in |V(d)|, but one |
|
~ |
|
of slope < 1 as in Figure 6.17. In this figure, the x− axis represents |V(d)|, |
|
while the y−axis represents possible normalization factors we can use. The |
|
thin line y = x depicts the use of cosine normalization. Notice the following |
|
aspects of the thick line representing pivoted length normalization: |
|
1. It is linear in the document length and has the form |
(6.16) |
~ |
a|V(d)| + (1 − a)piv, |
Online edition (c) 2009 Cambridge UP
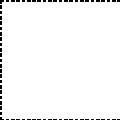
6.4 Variant tf-idf functions |
131 |
|
Pivoted normalization |
|
|
|
|
|
|
|
|
|
|
||||
|
|
|
|
6 |
y = x; Cosine |
|
|
|
|
||||||
|
|
|
|
|
|
|
|||||||||
|
|
|
|
|
|
|
|
|
|
|
|
|
|||
|
|
|
|
|
|
Pivoted |
|
|
|
|
|||||
|
|
|
|
|
|
|
|
|
|
|
|
|
|
||
|
|
|
|
|
|
|
|
|
|
|
|
|
|
|
|
|
|
|
|
|
|
|
|
|
|
|
|
|
|
|
|
|
|
|
|
|
|
|
|
|
|
|
|
|
|
|
|
|
|
|
|
|
|
|
|
|
|
|
|
|
|
|
|
|
|
|
|
|
|
|
|
|
|
|
|
|
|
|
|
|
|
|
|
|
|
|
|
|
|
|
|
|
|
|
|
|
|
|
|
|
|
|
|
|
|
|
|
|
~ |
||
|
|
|
|
|
|
|
|
|
|
|
|
- |
|V(d)| |
||
|
|
|
|
|
piv |
|
|
|
|
|
|
|
|
|
|
|
|
Figure 6.17 Implementing pivoted document length normalization by linear scal- |
|||||||||||||
|
|
ing. |
|
|
|
|
|
|
|
|
|
|
|||
|
|
|
|
|
|||||||||||
|
|
where piv is the cosine normalization value at which the two curves in- |
|||||||||||||
|
|
tersect. |
|
|
|
|
|
|
|
|
|
|
|||
|
|
2. Its slope is a < 1 and (3) it crosses the y = x line at piv. |
|||||||||||||
|
|
It has been argued that in practice, Equation (6.16) is well approximated by |
|||||||||||||
|
|
|
|
|
|
aud + (1 − a)piv, |
|||||||||
|
|
where ud is the number of unique terms in document d. |
|||||||||||||
|
|
Of course, pivoted document length normalization is not appropriate for |
|||||||||||||
|
|
all applications. For instance, in a collection of answers to frequently asked |
|||||||||||||
|
|
questions (say, at a customer service website), relevance may have little to |
|||||||||||||
|
|
do with document length. In other cases the dependency may be more com- |
|||||||||||||
|
|
plex than can be accounted for by a simple linear pivoted normalization. In |
|||||||||||||
|
|
such cases, document length can be used as a feature in the machine learning |
|||||||||||||
|
|
based scoring approach of Section 6.1.2. |
|
|
|
|
|||||||||
|
? |
Exercise 6.18 |
|
|
|
|
|
|
|
|
|
|
|||
EUCLIDEAN DISTANCE |
One measure of the similarity of two vectors is the Euclidean distance (or L2 distance) |
||||||||||||||
|
between them: |
|
|
|
= v |
|
|
|
|
|
|||||
|
|
|
|
|
~x |
− |
~y |
| |
∑ (xi |
− |
yi)2 |
||||
|
|
| |
|
|
ui=1 |
|
|
|
|||||||
|
|
|
|
|
|
|
|
|
|
u |
M |
|
|
|
|
|
|
|
|
|
|
|
|
|
|
t |
|
|
|
|
|
Online edition (c) 2009 Cambridge UP

132 |
|
|
|
6 Scoring, term weighting and the vector space model |
||||
|
|
query |
|
|
document |
|
qi · di |
|
|
|
|
|
|||||
|
word |
tf wf df |
idf |
qi = wf-idf |
|
tf wf di = normalized wf |
|
|
|
digital |
10,000 |
|
|
|
|
|
|
|
video |
100,000 |
|
|
|
|
|
|
|
cameras |
50,000 |
|
|
|
|
|
|
|
|
|
|
|
|
|
|
|
Table 6.1 Cosine computation for Exercise 6.19.
Given a query q and documents d1, d2, . . ., we may rank the documents di in order of increasing Euclidean distance from q. Show that if q and the di are all normalized to unit vectors, then the rank ordering produced by Euclidean distance is identical to that produced by cosine similarities.
Exercise 6.19
Compute the vector space similarity between the query “digital cameras” and the document “digital cameras and video cameras” by filling out the empty columns in Table 6.1. Assume N = 10,000,000, logarithmic term weighting (wf columns) for query and document, idf weighting for the query only and cosine normalization for the document only. Treat and as a stop word. Enter term counts in the tf columns. What is the final similarity score?
Exercise 6.20
Show that for the query affection, the relative ordering of the scores of the three documents in Figure 6.13 is the reverse of the ordering of the scores for the query jealous
gossip.
Exercise 6.21
In turning a query into a unit vector in Figure 6.13, we assigned equal weights to each of the query terms. What other principled approaches are plausible?
Exercise 6.22
Consider the case of a query term that is not in the set of M indexed terms; thus our
standard construction of the query vector results in ~ ( ) not being in the vector space
V q
created from the collection. How would one adapt the vector space representation to handle this case?
Exercise 6.23
Refer to the tf and idf values for four terms and three documents in Exercise 6.10. Compute the two top scoring documents on the query best car insurance for each of the following weighing schemes: (i) nnn.atc; (ii) ntc.atc.
Exercise 6.24
Suppose that the word coyote does not occur in the collection used in Exercises 6.10 and 6.23. How would one compute ntc.atc scores for the query coyote insurance?
Online edition (c) 2009 Cambridge UP