
- •Contents
- •Preface
- •How to use this book
- •Chapter 1 Units, constants, and conversions
- •1.1 Introduction
- •1.2 SI units
- •1.3 Physical constants
- •1.4 Converting between units
- •1.5 Dimensions
- •1.6 Miscellaneous
- •Chapter 2 Mathematics
- •2.1 Notation
- •2.2 Vectors and matrices
- •2.3 Series, summations, and progressions
- •2.5 Trigonometric and hyperbolic formulas
- •2.6 Mensuration
- •2.8 Integration
- •2.9 Special functions and polynomials
- •2.12 Laplace transforms
- •2.13 Probability and statistics
- •2.14 Numerical methods
- •Chapter 3 Dynamics and mechanics
- •3.1 Introduction
- •3.3 Gravitation
- •3.5 Rigid body dynamics
- •3.7 Generalised dynamics
- •3.8 Elasticity
- •Chapter 4 Quantum physics
- •4.1 Introduction
- •4.3 Wave mechanics
- •4.4 Hydrogenic atoms
- •4.5 Angular momentum
- •4.6 Perturbation theory
- •4.7 High energy and nuclear physics
- •Chapter 5 Thermodynamics
- •5.1 Introduction
- •5.2 Classical thermodynamics
- •5.3 Gas laws
- •5.5 Statistical thermodynamics
- •5.7 Radiation processes
- •Chapter 6 Solid state physics
- •6.1 Introduction
- •6.2 Periodic table
- •6.4 Lattice dynamics
- •6.5 Electrons in solids
- •Chapter 7 Electromagnetism
- •7.1 Introduction
- •7.4 Fields associated with media
- •7.5 Force, torque, and energy
- •7.6 LCR circuits
- •7.7 Transmission lines and waveguides
- •7.8 Waves in and out of media
- •7.9 Plasma physics
- •Chapter 8 Optics
- •8.1 Introduction
- •8.5 Geometrical optics
- •8.6 Polarisation
- •8.7 Coherence (scalar theory)
- •8.8 Line radiation
- •Chapter 9 Astrophysics
- •9.1 Introduction
- •9.3 Coordinate transformations (astronomical)
- •9.4 Observational astrophysics
- •9.5 Stellar evolution
- •9.6 Cosmology
- •Index
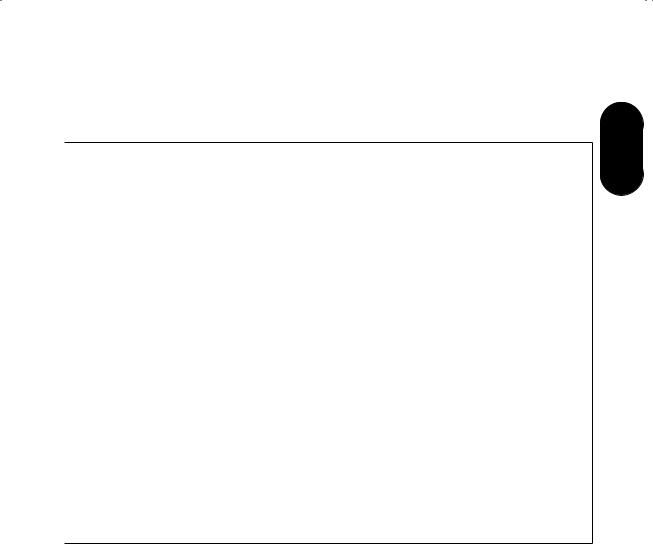
2.12 Laplace transforms |
55 |
|
|
2.12 Laplace transforms
Laplace transform theorems
|
Definitiona |
F(s) = L{f(t)} = 0 |
∞ f(t)e−st dt |
|
|
|
|
|
(2.514) |
|
||||||||||||||
|
|
|
|
|
|
|
||||||||||||||||||
|
Convolutionb |
F(s) · G(s) = L &0 |
∞ f(t− z)g(z) dz' |
|
|
|
(2.515) |
|
||||||||||||||||
|
|
|
|
|
|
|
= L{f(t) g(t)} |
|
|
|
|
|
|
|
(2.516) |
|
||||||||
|
|
|
|
|
1 |
|
γ+i∞ |
|
|
|
|
|
|
|
|
|
|
|
|
|
|
|
||
|
|
f(t) = |
|
|
γ−i∞ |
estF(s) ds |
|
|
|
|
|
|
|
(2.517) |
|
|||||||||
|
Inversec |
2πi |
|
|
|
|
|
|
|
|||||||||||||||
|
|
|
= residues |
|
|
(for t > 0) |
|
|
|
|
|
(2.518) |
|
|||||||||||
|
|
|
|
dnf(t) |
|
|
|
|
|
|
|
|
|
|
|
|
drf(t) |
|
|
|
||||
|
Transform of |
L & |
|
|
|
|
'= s |
|
L{f(t)} − r=0 s |
− − |
1 |
|
|
t=0 |
|
|||||||||
|
derivative |
|
dtn |
|
|
dtr |
|
|||||||||||||||||
|
|
|
|
|
|
|
|
|
|
|
|
|
|
|
|
|
|
|
|
|
|
|
(2.519) |
|
|
Derivative of |
|
dnF(s) |
|
|
|
|
|
|
|
|
|
|
|
|
|
|
|
|
|
||||
|
transform |
|
|
|
= L{(−t)nf |
(t)} |
|
|
|
|
|
|
|
|
(2.520) |
|
||||||||
|
|
dsn |
|
|
|
|
|
|
|
|
|
|||||||||||||
|
Substitution |
F(s− a) = L{eatf(t)} |
|
|
|
|
|
|
|
|
(2.521) |
|
||||||||||||
|
|
e−asF(s) = |
L{ |
u(t |
− |
a)f(t |
− |
a) |
} |
|
|
|
|
|
(2.522) |
|
||||||||
|
Translation |
|
|
|
|
|
|
|
|
|
|
|
|
|
|
|
|
|
||||||
|
|
|
|
|
|
|
|
0 (t < 0) |
|
|
|
|
|
|
|
|
|
|||||||
|
|
|
|
|
|
|
|
|
|
|
|
|
|
|
|
|
|
|||||||
|
|
where |
|
u(t) = "1 |
|
|
(t > 0) |
|
|
|
|
|
|
|
(2.523) |
|
L{} |
Laplace |
2 |
transform |
|
|
|
|
|
F(s) |
L{f(t)} |
|
G(s) |
L{g(t)} |
|
convolution
γconstant
ninteger > 0
aconstant
u(t) unit step function
aIf |
| |
e−s0tf(t) |
| |
is finite for su ciently large t, the Laplace transform exists for s > s0. |
|
b |
|
|
|
||
|
Also known as the “faltung (or folding) theorem.” |
cAlso known as the “Bromwich integral.” γ is chosen so that the singularities in F(s) are left of the integral line.

56 Mathematics
Laplace transform pairs
|
|
|
|
|
|
|
|
|
|
|
|
|
|
|
||
|
|
f(t) = F(s) = L{f(t)} = 0 |
∞ f(t)e−st dt |
(2.524) |
||||||||||||
|
|
δ(t) = 1 |
|
|
|
|
|
|
|
|
|
|
|
(2.525) |
||
|
|
1 |
= 1/s |
(s > 0) |
|
(2.526) |
||||||||||
|
|
tn |
= |
n! |
(s > 0, n > −1) |
(2.527) |
||||||||||
|
|
sn+1 |
|
|
|
|
|
|
||||||||
|
|
|
|
|
|
|
|
|
|
|
|
|
|
|
|
|
|
|
t1/2 |
= |
π |
|
|
|
(2.528) |
||||||||
|
|
|
|
|
|
|
|
|
|
|
|
|||||
|
|
4s3 |
|
|
|
|||||||||||
|
|
|
|
|
π |
|
|
|
|
|
||||||
|
|
t−1/2 |
= |
|
|
|
|
|
|
|
|
(2.529) |
||||
|
|
s |
|
|
|
|||||||||||
|
|
eat |
1 |
|
|
|
|
|
|
|
|
|
|
|
||
|
|
= |
|
|
|
|
(s > a) |
|
(2.530) |
|||||||
|
|
s− a |
|
|||||||||||||
|
|
teat |
= |
|
1 |
|
|
|
|
|
(s > a) |
|
(2.531) |
|||
|
|
(s− a)2 |
|
|
|
|||||||||||
(1 |
− |
at)e−at |
= |
|
s |
|
|
|
|
(2.532) |
||||||
|
+ a)2 |
|
|
|
||||||||||||
|
|
(s |
|
|
|
|
||||||||||
|
|
t2e−at |
= |
|
2 |
|
|
|
|
|
|
|
(2.533) |
|||
|
|
|
|
|
|
|
|
|
|
|
|
|
|
|||
|
|
|
+ a)3 |
|
|
|
||||||||||
|
|
|
(s |
|
|
|
|
|||||||||
|
|
sinat = |
|
a |
(s > 0) |
|
(2.534) |
|||||||||
|
|
|
|
|
||||||||||||
|
|
s2 + a2 |
|
|||||||||||||
|
|
cosat = |
|
s |
(s > 0) |
|
(2.535) |
|||||||||
|
|
|
|
|
||||||||||||
|
|
s2 + a2 |
|
|||||||||||||
|
|
sinhat = |
|
a |
(s > a) |
|
(2.536) |
|||||||||
|
|
|
|
|
||||||||||||
|
|
s2 − a2 |
|
|||||||||||||
|
|
coshat = |
|
s |
|
(s > a) |
|
(2.537) |
||||||||
|
|
s2 |
|
|
|
a2 |
|
|||||||||
e−bt sinat = |
|
− a |
|
|
|
(2.538) |
||||||||||
|
+ b)2 + a2 |
|
||||||||||||||
|
|
|
(s |
|
|
|||||||||||
e−bt cosat = |
|
s+ b |
|
(2.539) |
||||||||||||
|
|
|
|
|
|
|
|
|
|
|
|
|||||
|
+ b)2 + a2 |
|
||||||||||||||
|
|
|
(s |
|
|
|||||||||||
|
e−atf(t) = F(s+ a) |
|
|
|
(2.540) |
|||||||||||
|
|
|
|
|
|
|
|
|
|
|
|
|
|
|
|
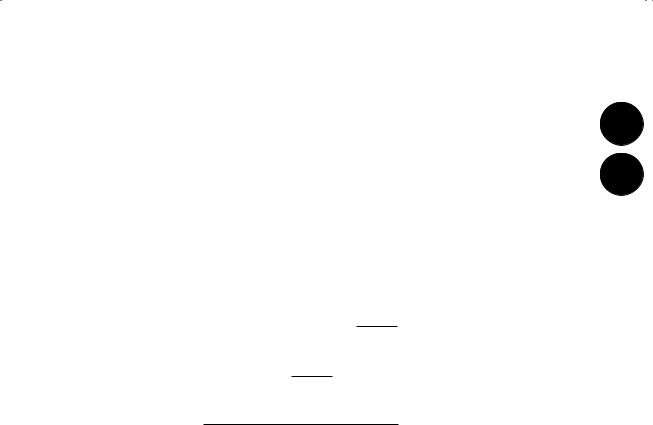
2.13 Probability and statistics |
57 |
|
|
2.13Probability and statistics
Discrete statistics |
|
|
|
|
|
|
|
|
|
|
|
|
|
|
|
|
|
|
|
|
|
|
|
|
|
|
|
|
2 |
|
|
|
1 |
|
|
N |
|
|
|
|
|
|
|
|
|
|
|
|
|
|
|
|
|
|
xi |
|
data series |
|
|
||
|
|
|
|
|
|
|
|
|
|
|
|
|
|
|
|
|
|
|
|
|
|
|
||||||||
Mean |
|
|
|
|
|
|
|
|
|
|
|
|
|
|
|
|
|
|
|
|
|
· |
|
series length |
|
|
||||
|
|
|
|
|
|
|
|
|
|
|
|
|
|
|
|
|
|
|
|
|
|
|
|
|
N |
|
|
|||
|
x = N i=1 xi |
|
|
|
|
|
|
|
|
|
|
|
|
|
|
(2.541) |
|
|
mean value |
|
||||||||||
|
|
|
|
|
|
|
|
− |
|
|
|
N |
|
|
|
|
|
|
|
|
|
|
|
|
|
|
|
|||
|
|
|
|
|
|
|
|
|
|
|
|
|
|
|
|
|
|
|
|
|
|
var[ |
] |
unbiased |
|
|
||||
|
|
|
|
|
|
|
|
1 |
|
|
|
|
|
|
|
|
|
|
|
|
|
|
|
|
|
|||||
Variancea |
var[x] = |
|
N |
|
|
1 |
|
|
(xi − x )2 |
|
|
|
|
|
|
(2.542) |
· |
|
variance |
|
|
|||||||||
|
|
|
|
|
|
|
|
|
|
|
|
i=1 |
|
|
|
|
|
|
|
|
|
|
|
|
|
|
|
|||
Standard |
σ[x] = (var[x])1/2 |
|
|
|
|
|
|
|
|
|
|
|
(2.543) |
σ |
|
standard |
|
|
||||||||||||
deviation |
|
|
|
|
|
|
|
|
|
|
|
|
|
|
|
|
|
|
|
|
|
|
|
|
|
|
|
deviation |
|
|
|
|
|
|
|
|
|
|
|
|
|
|
|
|
|
|
|
|
|
|
|
|
|
|
|
|
|
|
|
|
|
|
|
|
|
|
|
|
|
|
|
− |
|
|
|
− |
|
N |
|
|
|
|
|
|
3 |
|
|
|
|
|
||
Skewness |
skew[x] = |
|
|
|
N |
|
|
xi − x |
(2.544) |
|
|
|
|
|
||||||||||||||||
(N |
|
|
|
1)(N |
|
2) |
|
|
|
|
|
|
||||||||||||||||||
|
|
|
|
|
|
|
|
|
|
|
i=1 |
|
|
σ |
|
|
|
|
|
|
||||||||||
|
|
|
|
|
|
|
|
1 |
|
|
|
|
|
|
|
|
|
|
|
|
|
|
|
|
|
|
||||
Kurtosis |
kurt[x] |
|
|
|
|
|
|
|
|
|
|
|
xi − x |
|
|
|
3 |
|
(2.545) |
|
|
|
|
|
||||||
|
|
|
|
N i=1 |
|
|
σ |
|
− |
|
|
|
|
|
|
|
|
|
||||||||||||
|
|
|
|
|
|
|
|
|
N |
|
(xi − x )(yi − y ) |
|
|
|
|
x,y |
|
data series to |
|
|
||||||||||
Correlation |
|
|
|
|
|
|
|
|
|
|
|
|
|
|
|
correlate |
|
|
||||||||||||
r = |
|
|
|
|
|
|
|
i=1 |
|
|
|
(2.546) |
|
|
|
|||||||||||||||
|
|
|
N (xi |
|
|
y )2 |
|
|
|
|
|
|
||||||||||||||||||
coe cientb |
|
|
|
|
− |
x )2 |
|
N |
(yi |
− |
|
|
r |
|
correlation |
|
||||||||||||||
|
|
i=1 |
|
|
|
i=1 |
|
|
|
|
|
|
|
coe cient |
|
aIf x is derived from the data, {xi}, the relation is as shown. If x is known independently, then an unbiased estimate is obtained by dividing the right-hand side by N rather than N − 1.
bAlso known as “Pearson’s r.”
Discrete probability distributions
distribution |
pr(x) |
mean |
variance |
domain |
|
|
|
|||||
|
|
|
|
|
|
|
|
|
|
n |
binomial |
|
Binomial |
xn px(1 − p)n−x |
np |
np(1 − p) |
(x = 0,1,... ,n) |
(2.547) |
x |
||||||
coe cient |
||||||||||||
Geometric |
(1 |
− |
p)x−1p |
1/p |
(1 |
− |
p)/p2 |
(x = 1,2,3,...) |
(2.548) |
|
|
|
|
|
|
|
|
|
|
|
|
|
|||
Poisson |
λx exp(−λ)/x! |
λ |
λ |
|
|
(x = 0,1,2,...) |
(2.549) |
|
|
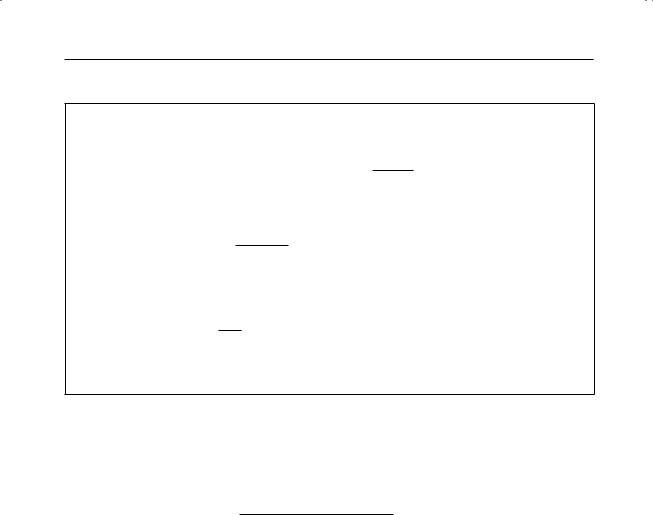
58 Mathematics
Continuous probability distributions
distribution |
|
pr(x) |
|
|
|
|
|
|
mean |
variance |
|
|
domain |
|
|
|
|
|||||||||||||||||
Uniform |
|
|
|
1 |
|
|
|
|
|
|
|
|
|
|
a+ b |
(b− a)2 |
|
|
(a |
≤ |
x |
≤ |
b) |
|
|
(2.550) |
||||||||
|
|
b |
− |
a |
|
|
|
|
|
|
|
|
2 |
|
|
|
|
|
|
|||||||||||||||
|
|
|
|
|
|
|
|
|
|
|
12 |
|
|
|
|
|
|
|
|
|
|
|
|
|||||||||||
|
|
|
|
|
|
|
|
|
|
|
|
|
|
|
|
|
1/λ2 |
|
|
|
|
|
|
|
|
|
|
|
|
|
|
|||
Exponential |
|
λexp(−λx) |
|
|
1/λ |
|
|
|
|
(x ≥ 0) |
|
|
|
(2.551) |
||||||||||||||||||||
Normal/ |
|
|
|
|
1 |
|
|
|
exp |
−(x− µ)2 |
µ |
|
|
σ2 |
|
|
|
|
( |
|
|
|
< x < |
|
) |
(2.552) |
||||||||
|
|
|
|
|
|
|
|
|
|
|
|
|
|
|
|
|
|
|
||||||||||||||||
Gaussian |
|
|
σ√2π |
|
|
|
2σ2 |
|
|
|
|
|
|
|
|
|
|
−∞ |
|
|
|
∞ |
|
|
||||||||||
Chi-squared |
a |
|
e−x/2x(r/2)−1 |
|
|
|
r |
2r |
|
|
|
|
(x ≥ 0) |
|
|
|
(2.553) |
|||||||||||||||||
|
|
2r/2Γ(r/2) |
|
|
|
|
|
|
|
|
|
|||||||||||||||||||||||
|
|
|
σ2 |
|
|
|
2σ2 |
|
|
( |
|
|
|
|
− |
4 ! |
|
|
≥ |
|
|
|
|
|
|
|
||||||||
Rayleigh |
|
|
x |
exp |
−x2 |
|
|
σ π/2 |
2σ2 |
1 |
|
π |
(x |
|
0) |
|
|
|
(2.554) |
|||||||||||||||
|
|
|
|
|
|
|
|
|
|
|
|
|||||||||||||||||||||||
Cauchy/ |
|
|
|
|
|
|
a |
|
|
|
|
|
|
(none) |
(none) |
|
|
|
(−∞ < x < ∞) |
(2.555) |
||||||||||||||
Lorentzian |
|
|
π(a2 + x2) |
|
|
|
|
|
aWith r degrees of freedom. Γ is the gamma function.
Multivariate normal distribution
|
|
|
|
|
|
|
|
$ |
|
pr |
probability density |
|
|
|
|
exp |
− |
1 (x |
µ)C−1(x |
µ)T |
|
k |
number of dimensions |
||
Density function |
pr(x) = |
|
2 |
|
|
|
|
|
||||
# |
(2π)k/−2[det(C)]1/−2 |
|
|
C |
covariance matrix |
|||||||
|
|
|
|
|
|
|
(2.556) |
x |
variable (k dimensional) |
|||
|
|
|
|
|
|
|
|
|
|
µ |
vector of means |
|
|
|
|
|
|
|
|
|
|
|
T |
transpose |
|
|
|
|
|
|
|
|
|
|
|
|||
|
|
|
|
|
|
|
|
|
|
|
||
Mean |
µ = (µ1,µ2,... ,µk) |
|
(2.557) |
det |
determinant |
|||||||
|
|
|
|
|
|
|
|
|
|
µi |
mean of ith variable |
|
Covariance |
C = σij = xixj |
− xi |
xj |
(2.558) |
σij |
components of C |
||||||
Correlation |
r = |
σij |
|
|
|
(2.559) |
r |
correlation coe cient |
||||
coe cient |
σiσj |
|
|
|
|
|||||||
|
|
|
|
1/2 |
|
|
|
|
xi |
normally distributed deviates |
||
Box–Muller |
x1 = (−2lny1)1/2 cos2πy2 |
(2.560) |
||||||||||
yi |
deviates distributed |
|||||||||||
transformation |
x2 = (−2lny1) |
sin2πy2 |
(2.561) |
|
uniformly between 0 and 1 |
|||||||
|
|
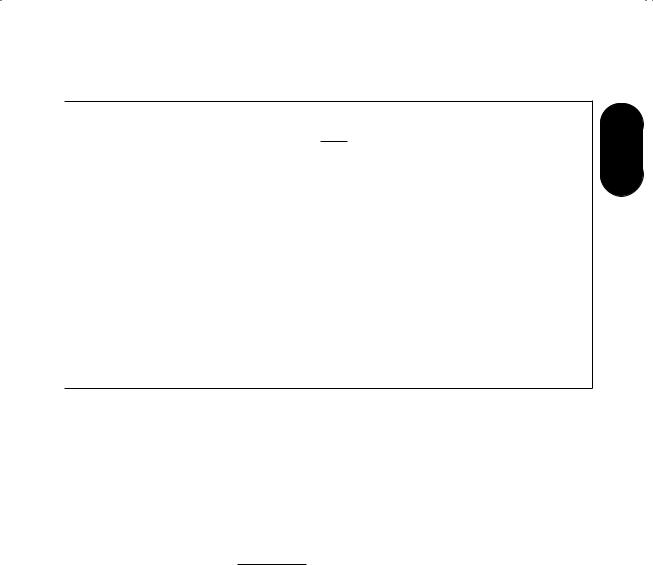
2.13 Probability and statistics |
59 |
|
|
Random walk
One- |
pr(x) = |
|
|
|
|
1 |
|
|
|
exp |
−x2 |
|
||
|
|
|
|
|
|
|
|
|
||||||
dimensional |
|
(2πNl2)1/2 |
|
|
2Nl2 |
|||||||||
|
|
|
|
|
|
|
|
|
|
|
|
|
|
(2.562) |
rms |
xrms = N1/2l |
|
|
|
|
|
|
|
|
(2.563) |
||||
displacement |
|
|
|
|
|
|
|
|
|
|
|
|
|
|
|
|
|
|
|
|
|
|
|
|
|
|
|
|
|
|
|
|
|
|
a |
|
|
|
3 |
|
|
|
|
|
|
pr(r) = |
|
|
|
|
|
exp(−a2r2) |
(2.564) |
||||||
Three- |
|
|
π1/2 |
|
||||||||||
dimensional |
|
|
|
|
|
! |
3 |
|
|
1/2 |
|
|
||
where a = |
|
|
|
|
|
|
||||||||
|
|
|
|
|
||||||||||
|
2Nl2 |
|
|
|
||||||||||
|
r = |
8 |
|
1/2 |
|
|
|
|
|
|
||||
Mean distance |
|
|
|
N1/2l |
|
|
(2.565) |
|||||||
|
|
|
|
|
|
|||||||||
3π |
|
|
|
|
|
|||||||||
rms distance |
rrms = N1/2l |
|
|
|
|
|
|
|
|
(2.566) |
||||
|
|
|
|
|
|
|
|
|
|
|
|
|
|
|
xdisplacement after N steps (can be positive or negative)
pr(x) probability density of x |
2 |
|
|
(%−∞∞ pr(x) dx = 1) |
|
N |
number of steps |
|
lstep length (all equal)
xrms root-mean-squared displacement from start point
rradial distance from start point
pr(r) probability density of r (%0∞ 4πr2 pr(r) dr = 1)
a(most probable distance)−1
r mean distance from start point
rrms |
root-mean-squared distance |
|
from start point |
Bayesian inference
Conditional |
|
|
|
|
|
|
|
|
pr(x) |
|
probability (density) of x |
|
pr(x) = |
pr(x |
y |
)pr(y |
) dy |
(2.567) |
pr(x |
y |
|
conditional probability of x |
|||
|
|
|||||||||||
probability |
|
|
| |
|
|
|
|
| |
|
) |
given y |
|
Joint |
pr(x,y) = pr(x)pr(y|x) |
|
|
(2.568) |
pr(x,y) |
joint probability of x and y |
||||||
probability |
|
|
||||||||||
Bayes’ theorema |
pr(y |
x) = |
pr(x|y) pr(y) |
|
|
(2.569) |
|
|
|
|
||
|
| |
|
pr(x) |
|
|
|
|
|
|
|
aIn this expression, pr(y|x) is known as the posterior probability, pr(x|y) the likelihood, and pr(y) the prior probability.