
- •List of Tables
- •List of Figures
- •Table of Notation
- •Preface
- •Boolean retrieval
- •An example information retrieval problem
- •Processing Boolean queries
- •The extended Boolean model versus ranked retrieval
- •References and further reading
- •The term vocabulary and postings lists
- •Document delineation and character sequence decoding
- •Obtaining the character sequence in a document
- •Choosing a document unit
- •Determining the vocabulary of terms
- •Tokenization
- •Dropping common terms: stop words
- •Normalization (equivalence classing of terms)
- •Stemming and lemmatization
- •Faster postings list intersection via skip pointers
- •Positional postings and phrase queries
- •Biword indexes
- •Positional indexes
- •Combination schemes
- •References and further reading
- •Dictionaries and tolerant retrieval
- •Search structures for dictionaries
- •Wildcard queries
- •General wildcard queries
- •Spelling correction
- •Implementing spelling correction
- •Forms of spelling correction
- •Edit distance
- •Context sensitive spelling correction
- •Phonetic correction
- •References and further reading
- •Index construction
- •Hardware basics
- •Blocked sort-based indexing
- •Single-pass in-memory indexing
- •Distributed indexing
- •Dynamic indexing
- •Other types of indexes
- •References and further reading
- •Index compression
- •Statistical properties of terms in information retrieval
- •Dictionary compression
- •Dictionary as a string
- •Blocked storage
- •Variable byte codes
- •References and further reading
- •Scoring, term weighting and the vector space model
- •Parametric and zone indexes
- •Weighted zone scoring
- •Learning weights
- •The optimal weight g
- •Term frequency and weighting
- •Inverse document frequency
- •The vector space model for scoring
- •Dot products
- •Queries as vectors
- •Computing vector scores
- •Sublinear tf scaling
- •Maximum tf normalization
- •Document and query weighting schemes
- •Pivoted normalized document length
- •References and further reading
- •Computing scores in a complete search system
- •Index elimination
- •Champion lists
- •Static quality scores and ordering
- •Impact ordering
- •Cluster pruning
- •Components of an information retrieval system
- •Tiered indexes
- •Designing parsing and scoring functions
- •Putting it all together
- •Vector space scoring and query operator interaction
- •References and further reading
- •Evaluation in information retrieval
- •Information retrieval system evaluation
- •Standard test collections
- •Evaluation of unranked retrieval sets
- •Evaluation of ranked retrieval results
- •Assessing relevance
- •A broader perspective: System quality and user utility
- •System issues
- •User utility
- •Results snippets
- •References and further reading
- •Relevance feedback and query expansion
- •Relevance feedback and pseudo relevance feedback
- •The Rocchio algorithm for relevance feedback
- •Probabilistic relevance feedback
- •When does relevance feedback work?
- •Relevance feedback on the web
- •Evaluation of relevance feedback strategies
- •Pseudo relevance feedback
- •Indirect relevance feedback
- •Summary
- •Global methods for query reformulation
- •Vocabulary tools for query reformulation
- •Query expansion
- •Automatic thesaurus generation
- •References and further reading
- •XML retrieval
- •Basic XML concepts
- •Challenges in XML retrieval
- •A vector space model for XML retrieval
- •Evaluation of XML retrieval
- •References and further reading
- •Exercises
- •Probabilistic information retrieval
- •Review of basic probability theory
- •The Probability Ranking Principle
- •The 1/0 loss case
- •The PRP with retrieval costs
- •The Binary Independence Model
- •Deriving a ranking function for query terms
- •Probability estimates in theory
- •Probability estimates in practice
- •Probabilistic approaches to relevance feedback
- •An appraisal and some extensions
- •An appraisal of probabilistic models
- •Bayesian network approaches to IR
- •References and further reading
- •Language models for information retrieval
- •Language models
- •Finite automata and language models
- •Types of language models
- •Multinomial distributions over words
- •The query likelihood model
- •Using query likelihood language models in IR
- •Estimating the query generation probability
- •Language modeling versus other approaches in IR
- •Extended language modeling approaches
- •References and further reading
- •Relation to multinomial unigram language model
- •The Bernoulli model
- •Properties of Naive Bayes
- •A variant of the multinomial model
- •Feature selection
- •Mutual information
- •Comparison of feature selection methods
- •References and further reading
- •Document representations and measures of relatedness in vector spaces
- •k nearest neighbor
- •Time complexity and optimality of kNN
- •The bias-variance tradeoff
- •References and further reading
- •Exercises
- •Support vector machines and machine learning on documents
- •Support vector machines: The linearly separable case
- •Extensions to the SVM model
- •Multiclass SVMs
- •Nonlinear SVMs
- •Experimental results
- •Machine learning methods in ad hoc information retrieval
- •Result ranking by machine learning
- •References and further reading
- •Flat clustering
- •Clustering in information retrieval
- •Problem statement
- •Evaluation of clustering
- •Cluster cardinality in K-means
- •Model-based clustering
- •References and further reading
- •Exercises
- •Hierarchical clustering
- •Hierarchical agglomerative clustering
- •Time complexity of HAC
- •Group-average agglomerative clustering
- •Centroid clustering
- •Optimality of HAC
- •Divisive clustering
- •Cluster labeling
- •Implementation notes
- •References and further reading
- •Exercises
- •Matrix decompositions and latent semantic indexing
- •Linear algebra review
- •Matrix decompositions
- •Term-document matrices and singular value decompositions
- •Low-rank approximations
- •Latent semantic indexing
- •References and further reading
- •Web search basics
- •Background and history
- •Web characteristics
- •The web graph
- •Spam
- •Advertising as the economic model
- •The search user experience
- •User query needs
- •Index size and estimation
- •Near-duplicates and shingling
- •References and further reading
- •Web crawling and indexes
- •Overview
- •Crawling
- •Crawler architecture
- •DNS resolution
- •The URL frontier
- •Distributing indexes
- •Connectivity servers
- •References and further reading
- •Link analysis
- •The Web as a graph
- •Anchor text and the web graph
- •PageRank
- •Markov chains
- •The PageRank computation
- •Hubs and Authorities
- •Choosing the subset of the Web
- •References and further reading
- •Bibliography
- •Author Index
178 |
9 Relevance feedback and query expansion |
9.1Relevance feedback and pseudo relevance feedback
RELEVANCE FEEDBACK The idea of relevance feedback (RF) is to involve the user in the retrieval process so as to improve the final result set. In particular, the user gives feedback on the relevance of documents in an initial set of results. The basic procedure is:
•The user issues a (short, simple) query.
•The system returns an initial set of retrieval results.
•The user marks some returned documents as relevant or nonrelevant.
•The system computes a better representation of the information need based on the user feedback.
•The system displays a revised set of retrieval results.
Relevance feedback can go through one or more iterations of this sort. The process exploits the idea that it may be difficult to formulate a good query when you don’t know the collection well, but it is easy to judge particular documents, and so it makes sense to engage in iterative query refinement of this sort. In such a scenario, relevance feedback can also be effective in tracking a user’s evolving information need: seeing some documents may lead users to refine their understanding of the information they are seeking.
Image search provides a good example of relevance feedback. Not only is it easy to see the results at work, but this is a domain where a user can easily have difficulty formulating what they want in words, but can easily indicate relevant or nonrelevant images. After the user enters an initial query for bike on the demonstration system at:
http://nayana.ece.ucsb.edu/imsearch/imsearch.html
the initial results (in this case, images) are returned. In Figure 9.1 (a), the user has selected some of them as relevant. These will be used to refine the query, while other displayed results have no effect on the reformulation. Figure 9.1 (b) then shows the new top-ranked results calculated after this round of relevance feedback.
Figure 9.2 shows a textual IR example where the user wishes to find out about new applications of space satellites.
9.1.1The Rocchio algorithm for relevance feedback
The Rocchio Algorithm is the classic algorithm for implementing relevance feedback. It models a way of incorporating relevance feedback information into the vector space model of Section 6.3.
Online edition (c) 2009 Cambridge UP
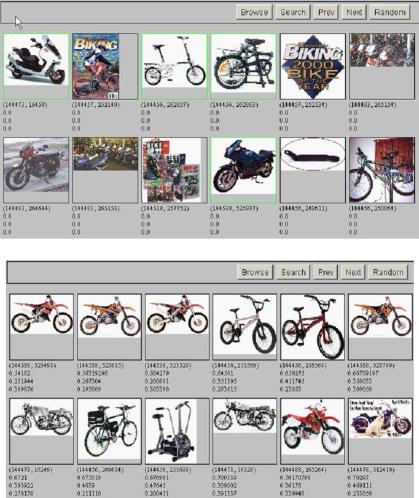
9.1 Relevance feedback and pseudo relevance feedback |
179 |
(a)
(b)
Figure 9.1 Relevance feedback searching over images. (a) The user views the initial query results for a query of bike, selects the first, third and fourth result in the top row and the fourth result in the bottom row as relevant, and submits this feedback. (b) The users sees the revised result set. Precision is greatly improved.
From http://nayana.ece.ucsb.edu/imsearch/imsearch.html (Newsam et al. 2001).
Online edition (c) 2009 Cambridge UP
180 |
9 Relevance feedback and query expansion |
(a)Query: New space satellite applications
(b)+ 1. 0.539, 08/13/91, NASA Hasn’t Scrapped Imaging Spectrometer
+2. 0.533, 07/09/91, NASA Scratches Environment Gear From Satellite Plan
3.0.528, 04/04/90, Science Panel Backs NASA Satellite Plan, But Urges Launches of Smaller Probes
4.0.526, 09/09/91, A NASA Satellite Project Accomplishes Incredible Feat: Staying Within Budget
5.0.525, 07/24/90, Scientist Who Exposed Global Warming Proposes Satellites for Climate Research
6.0.524, 08/22/90, Report Provides Support for the Critics Of Using Big Satellites to Study Climate
7.0.516, 04/13/87, Arianespace Receives Satellite Launch Pact From Telesat Canada
+8. 0.509, 12/02/87, Telecommunications Tale of Two Companies
(c) |
2.074 new |
15.106 space |
|
|
30.816 satellite |
5.660 application |
|
|
5.991 nasa |
5.196 eos |
|
|
4.196 launch |
3.972 aster |
|
|
3.516 instrument |
3.446 arianespace |
|
|
3.004 bundespost |
2.806 ss |
|
|
2.790 rocket |
2.053 scientist |
|
|
2.003 broadcast |
1.172 earth |
|
|
0.836 oil 0.646 measure |
(d)* 1. 0.513, 07/09/91, NASA Scratches Environment Gear From Satellite Plan
* 2. 0.500, 08/13/91, NASA Hasn’t Scrapped Imaging Spectrometer
3.0.493, 08/07/89, When the Pentagon Launches a Secret Satellite, Space Sleuths Do Some Spy Work of Their Own
4.0.493, 07/31/89, NASA Uses ‘Warm’ Superconductors For Fast
Circuit
*5. 0.492, 12/02/87, Telecommunications Tale of Two Companies
6.0.491, 07/09/91, Soviets May Adapt Parts of SS-20 Missile For Commercial Use
7.0.490, 07/12/88, Gaping Gap: Pentagon Lags in Race To Match the Soviets In Rocket Launchers
8.0.490, 06/14/90, Rescue of Satellite By Space Agency To Cost $90 Million
Figure 9.2 Example of relevance feedback on a text collection. (a) The initial query
(a). (b) The user marks some relevant documents (shown with a plus sign). (c) The query is then expanded by 18 terms with weights as shown. (d) The revised top results are then shown. A * marks the documents which were judged relevant in the relevance feedback phase.
Online edition (c) 2009 Cambridge UP
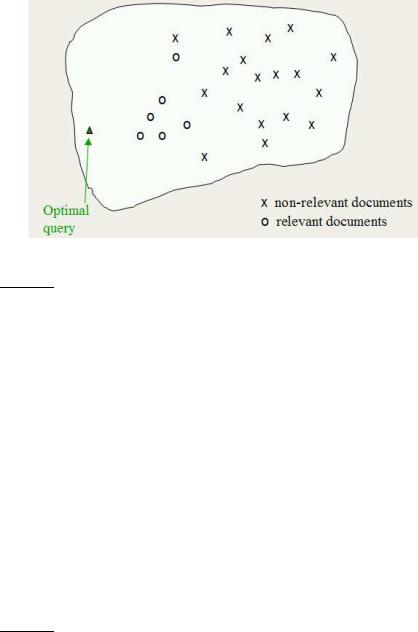
9.1 Relevance feedback and pseudo relevance feedback |
181 |
Figure 9.3 The Rocchio optimal query for separating relevant and nonrelevant documents.
The underlying theory. We want to find a query vector, denoted as ~q, that maximizes similarity with relevant documents while minimizing similarity with nonrelevant documents. If Cr is the set of relevant documents and Cnr is the set of nonrelevant documents, then we wish to find:1
(9.1) |
~qopt = arg max[sim(~q, Cr) − sim(~q, Cnr)], |
|||||||||||
|
|
~q |
|
|
|
|
|
|
|
|
||
|
where sim is defined as in Equation 6.10. Under cosine similarity, the optimal |
|||||||||||
|
query vector ~qopt for separating the relevant and nonrelevant documents is: |
|||||||||||
(9.2) |
|
|
|
1 |
|
|
|
~ |
1 |
|
|
~ |
~qopt = |
|
|
Cr |
|
~∑ dj − |
Cnr |
~ |
∑ dj |
||||
|
|
| |
|
| |
dj |
|
Cr |
| | |
dj |
|
Cnr |
|
|
|
|
|
|
That is, the optimal query is the vector difference between the centroids of the relevant and nonrelevant documents; see Figure 9.3. However, this observation is not terribly useful, precisely because the full set of relevant documents is not known: it is what we want to find.
ROCCHIO ALGORITHM The Rocchio (1971) algorithm. This was the relevance feedback mecha-
1. In the equation, arg maxx f (x) returns a value of x which maximizes the value of the function f (x). Similarly, arg minx f (x) returns a value of x which minimizes the value of the function f (x).
Online edition (c) 2009 Cambridge UP
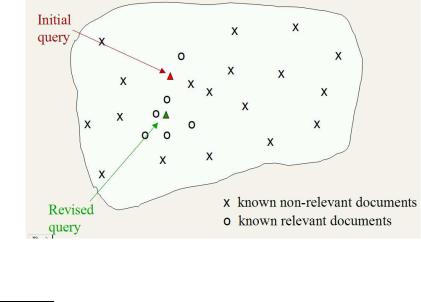
182 |
9 Relevance feedback and query expansion |
Figure 9.4 An application of Rocchio’s algorithm. Some documents have been labeled as relevant and nonrelevant and the initial query vector is moved in response to this feedback.
nism introduced in and popularized by Salton’s SMART system around 1970. In a real IR query context, we have a user query and partial knowledge of known relevant and nonrelevant documents. The algorithm proposes using the modified query ~qm:
(9.3) |
|
|
1 |
|
|
|
~ |
|
1 |
|
|
|
|
~ |
~qm = α~q0 + β |
|
Dr |
|
~ ∑ dj − γ |
|
Dnr |
~ |
∑ |
dj |
|||||
|
|
| |
|
| |
dj |
|
Dr |
| |
|
| dj |
|
Dnr |
|
|
|
|
|
|
|
|
|
where q0 is the original query vector, Dr and Dnr are the set of known relevant and nonrelevant documents respectively, and α, β, and γ are weights attached to each term. These control the balance between trusting the judged document set versus the query: if we have a lot of judged documents, we would like a higher β and γ. Starting from q0, the new query moves you some distance toward the centroid of the relevant documents and some distance away from the centroid of the nonrelevant documents. This new query can be used for retrieval in the standard vector space model (see Section 6.3). We can easily leave the positive quadrant of the vector space by subtracting off a nonrelevant document’s vector. In the Rocchio algorithm, negative term weights are ignored. That is, the term weight is set to 0. Figure 9.4 shows the effect of applying relevance feedback.
Relevance feedback can improve both recall and precision. But, in practice, it has been shown to be most useful for increasing recall in situations
Online edition (c) 2009 Cambridge UP