
Учебники / Hearing - From Sensory Processing to Perception Kollmeier 2007
.pdfSpatio-Temporal Representation of the Pitch of Complex Tones in the Auditory Nerve |
69 |
Shera CA, Guinan JJ, Jr., and Oxenham AJ (2002) Revised estimates of human cochlear tuning from otoacoustic and behavioral measurements. Proc Natl Acad Sci USA 99.
Shamma SA (1985) Speech processing in the auditory system. I: The representation of speech sounds in the responses of the auditory nerve. J Acoust Soc Am 78: 1612-1621.
Ruggero M and Temchin AN (2005) Unexceptional sharpness of frequency tuning in the human cochlea. Proc Natl Acad Sci USA 102: 18614-18619.
Zhang X, Heinz MG, Bruce IC, and Carney LH (2001) A phenomenological model for the responses of auditory-nerve fibers: I. Nonlinear tuning with compression and suppression.
J Acoust Soc Am 109: 648-670.
Zweig G (1976) Basilar membrane motion. Cold Spr Harb Symp Quant Biol 40: 619-633.
Comment by Greenberg
Could you describe how your model would handle the so-called “dominance region” for pitch? Ritsma (1967) and Plomp (1967) (as well as others) have shown that the spectral region generating the strongest sensation of pitch often varies as a function of fundamental frequency (f0). For an f0 of 500 Hz, the dominant harmonics are the second and third, while for an f0 of 100 Hz the sixth and seventh harmonics are dominant. In your model, the frequency resolution of the auditory periphery is logarithmically constant across frequency, which makes it difficult to accommodate findings such as those reported by Ritsma and Plomp. In other words, your model would seem to predict that the strongest pitch would be generated by a certain set of harmonics regardless of fundamental frequency (up to the limit of musical pitch). One way (of several) to resolve this issue would be to assume that the frequency selectivity of the auditory periphery is not constant Q (as in your model) but varies in a manner consistent with the tuning characteristics of auditory nerve fibers (e.g., Evans, 1975). In such studies, the Q10 dB of fibers varies between 0.5 for units with characteristic frequencies below 800 Hz to approximately 2 for the spectral region of 1.5 kHz (the upper limit of the dominance region).
References
Evans, E. (1975) The cochlear nerve and cochlear nucleus. In Handbook of Sensory Physiology. W. D. Keidel (ed.). Heidelberg: Springer, pp. 1-109.
Plomp, R. (1967) Pitch of complex tones. Journal of the Acoustical Society of America 41: 1526-1533.
Ritsma, R. (1967) Frequencies dominant in the perception of pitch of complex sounds. Journal of the Acoustical Society of America 42: 191-198.
Reply
Since our paper primarily reports single-unit data from the cat auditory nerve, the increase in cochlear frequency selectivity (as measured by Q) with CF is naturally included. We only assumed constant-Q filtering (as prescribed
70 |
L. Cedolin and B. Delgutte |
by scaling invariance) for the specific purpose of estimating the spatial derivative from the responses of a single AN fiber to a set of complex tones with varying F0. Since F0 was varied over only 1.6 octave, and the dependence of Q on CF is very gradual, the bandwidth errors resulting from this assumption are small. Specifically, the differences between the neural bandwidths (measured from reverse correlation functions by Carney and Yin, 1988) and those predicted from the constant-Q assumption never exceeded ±11% for any CF within the range investigated.
We don’t see how the increase in Q with CF could explain how the dominance region for pitch depends on F0 because higher-order harmonics are increasingly well resolved as F0 increases due to the increase in Q, while, psychophysically, low-order harmonics are increasingly dominant at higher F0s (Moore et al. 1985; Dai, 2000). The spatio-temporal representation offers a solution to this problem because, by requiring phase locking to the harmonics, it imposes an upper frequency limit (~3000 Hz) to which harmonics can contribute to pitch. Since higher-order harmonics will increasingly exceed this limit as F0 increases, pitch estimation from spatio-temporal cues has to rely increasingly on low-order harmonics, consistent with the psychophysics.
It is more difficult to account for the psychophysical observation that the dominant harmonics are not always the lowest ones for low F0s. In our data, the lowest harmonic present (Harmonic 2) is always the most prominent in the spatio-temporal representation. However, for each fiber, we selected stimulus levels for our complex tones relative to the pure-tone threshold at CF; this procedure effectively equalizes the middle-ear transfer function, which would otherwise attenuate low-frequency harmonics. Since the perceptual dominance of a harmonic increases with its relative amplitude (Moore et al., 1985), low-order harmonics may be sufficiently attenuated by the middle ear at low F0s that they can no longer contribute to pitch. Note that the dominant harmonics at low F0s vary substantially between studies depending on the method used (Ritsma, 1967; Plomp, 1967; Moore et al. 1985; Dai, 2000), and there can be large intersubject differences within the same study (Moore et al. 1985). For example, for one of the subjects of Moore et al. (1985), the fundamental was dominant for F0 = 200 Hz.
References
Carney, LH, and Yin, TCT (1988). Temporal coding of resonances by low-frequency auditorynerve fibers: Single-fiber responses and a population model. J Neurophysiol. 60: 1653-1677.
Dai, H. (2000). On the relative influence of individual harmonics on pitch judgment. J Acoust Soc Am 107: 953-959.
Moore, BCJ, and Glasberg BR (1985). Relative dominance of individual partials in determining the pitch of complex tones. J Acoust Soc Am 77:1853-1860.
Plomp, R. (1967) Pitch of complex tones. J Acoust Soc Am 41: 1526-1533.
Ritsma, R. (1967) Frequencies dominant in the perception of pitch of complex sounds. J Acoust Soc Am 42: 191-198.

9 Virtual Pitch in a Computational
Physiological Model
RAY MEDDIS AND LOWEL O’MARD
1Introduction
There are many different explanations of the origin of virtual pitches and these are often categorized as either ‘spectral’ or ‘temporal’. This chapter addresses a group of hypotheses in the ‘temporal’ category. These theories assume that virtual pitch arises from temporal regularity or periodicities in sounds and these regularities can be characterized using statistical methods such as autocorrelation. This approach makes many quantitatively detailed and often successful predictions concerning the outcome of a wide range of virtual pitch experiments.
The status of autocorrelation models remains controversial, however, because it appears to be physiologically implausible. There are no structures in the auditory brainstem that look capable of carrying out the delay and multiply operations required by autocorrelation. This is a major impediment to a general acceptance of an autocorrelation-type model of virtual pitch perception. We address this issue by showing that a model using physiologically plausible components can behave in some important respects like autocorrelation and can simulate a number of the most important virtual pitch phenomena.
This model is an expanded version of an older computational model that was originally developed to simulate the response of single units in the auditory brainstem to sinusoidally amplitude modulated (SAM) tones (Hewitt and Meddis 1993, 1994). In those studies it was shown that the model is able to simulate appropriate modulation transfer functions (MTFs) in cochlear nucleus (CN) neurons when stimulated using sinusoidally amplitude modulated (SAM) tones. It can also simulate appropriate rate MTFs in single inferior colliculus (IC) neurons. An earlier study has already shown that the response of the model CN units is a successful simulation of the neuron’s response to broadband pitch stimuli (Wiegrebe and Meddis 2004).
An additional cross-channel processing stage has been added to allow information to be aggregated across channels. This is essential for pitch processing because the stimuli with the clearest pitch consist of harmonics that
Department of Psychology, Essex University, Colchester, CO4 3SQ, UK, rmeddis@essex.ac.uk
Hearing – From Sensory Processing to Perception
B. Kollmeier, G. Klump, V. Hohmann, U. Langemann, M. Mauermann, S. Uppenkamp, and J. Verhey (Eds.) © Springer-Verlag Berlin Heidelberg 2007
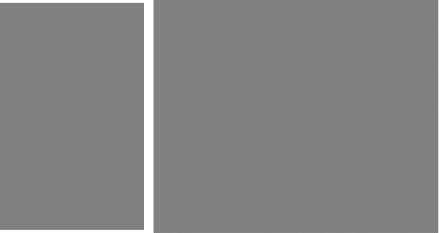
72 |
R. Meddis and L. O’Mard |
are resolved by the periphery. The overall architecture of the model is the same as that of an autocorrelation model (Meddis and O’Mard 1997). It consists of three stages: 1) peripheral segregation of sound into frequency bands, 2) extraction of periodicities on a within-channel basis, and 3) aggregation of periodicity information across BF-channels. The novelty in the model lies in the way in which periodicity is extracted; using physiologically plausible circuits rather than an artificial mathematical device.
2The Model
The model contains thousands of individual components but is modular in structure (Fig. 1). The basic building block of the system is a module consisting of a cascade of three stages: 1) auditory nerve (AN) fibers, 2) CN units, and 3) an IC unit. Each module has a single IC cell receiving input from 10 CN units all with the same saturated chopping rate. Each CN unit receives input from 30 AN fibers all with the same BF. All modules are identical except for BF and the saturated firing rate of the CN units. Within a module, it is the saturated firing rate of the CN units that determine the selectivity of the IC rate response to periodicity. The CN units are modeled on CN chopper units that chop at a fixed rate in response to moderately intense acoustic stimulation.
Fig. 1 A A single constituent module. A module consists of 10 CN units feeding one IC unit. Each CN unit receives input from 30 AN same-BF fibers. Within a module, all CN units have the same saturated firing (chopping) rate. B Arrangement of modules. There are 30 modules within each BF channel, each with a different characteristic firing rate. There are 40 channels with BFs ranging from 100 to 10,000 Hz. A stage-4 unit receives one input from each channel from modules with the same saturated chopping rate
Virtual Pitch in a Computational Physiological Model |
73 |
These modules are the same as those described in Hewitt and Meddis (1994) The extended model replicates this core module many times within a single BF channel using different chopping rates in different modules. There are 10 CN units in each block and, within a single channel, there are 30 blocks, each characterised by its chopping rate. This arrangement is replicated across 40 BF channels making a total of 12000 CN units and 1200 IC units.
This multi-rate, multi-BF architecture provides the basis for a further extension of the model, a fourth stage where periodicity information is aggregated across BF channels. This pitch-extraction stage is added to the model in the form of an array of hypothetical ‘stage 4’ units called the `stage 4 profile’. Each stage 4 unit receives input from one IC unit from each BF channel where all the contributing IC units have the same best modulation frequency and, hence, the same CN chopping rate.
The within-module details are largely unchanged from the original modelling studies. Improvements to the detail of the AN model have also been included in order to be consistent with recent modelling work from this laboratory. The AN section of the model has, for example, been recently updated and is fully described in Meddis (2006). The parameters of the dual resonance nonlinear (DRNL) filterbank are based on human psychophysical data (Lopez-Poveda and Meddis 2001).
3Implementation Details
Stage 1: auditory periphery. The basilar membrane was modelled as 40 channels whose best frequencies (BFs) were equally spaced on a log scale across the range 100–10,000 Hz. This was implemented as an array of dual resonance nonlinear (DRNL; Meddis et al. 2001) filters. All fibers were modelled identically, except for stochasticity, as high spontaneous rate (HSR) fibers with thresholds at 0 dB SPL at 1 kHz. The output of the auditory periphery was a stochastic stream of independent spike events in each auditory nerve fiber.
Stage 2: CN units. These are implemented as modified McGregor cells (MacGregor 1987) and are fully described in Hewitt and Meddis (1993) and Meddis (2006). The intrinsic (saturated) chopping rate of the CN units is determined in the model by the potassium recovery time constant (τGk). This time constant is varied systematically across the array of units in such a way as to produce 30 different chopping rates equally spaced between 60 and 350 spikes/s. The appropriate values of τGk were determined with an empirically derived formula; τGk=rate−1.441 based on the pure tone response at 65 dB SPL. Time constants varied between 2.8 ms (60 Hz) and 0.22 ms (350 Hz).
Stage 3: IC units. These are described in full in Hewitt and Meddis (1994) and are implemented here using the same MacGregor algorithm as used for the CN units. A single IC unit receives input from 10 CN units. It is a critical (and speculative) feature of the model that each IC unit receives input only
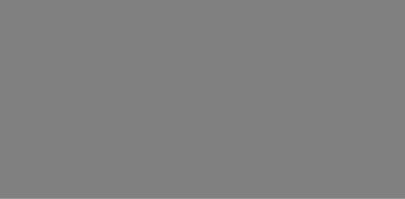
74 |
R. Meddis and L. O’Mard |
from CN units with the same intrinsic chopping rate. The thresholds of the IC units are set to require coincidental input from many CN units.
Stage 4 units. These units receive input from 40 IC units (one per BF-channel). All inputs to a single unit have the same rate-MTF as determined by the intrinsic chopping rate of the CN units feeding the IC unit. It is assumed that each spike input to the stage 4 unit provokes an action potential. Therefore, stage 4 units are not coincidence detectors but simply counters of all the spikes occurring in their feeder units. There are 30 stage 4 units, one for each CN rate. The output of the model is, therefore, an array of 30 spike counts called the ‘stage-4 profile’.
4Evaluation
4.1Pitch of a Harmonic Tone
Figure 2A shows the stage 4 profile to three ‘missing fundamental’ harmonic tones composed of harmonics 3 through 8 presented at 70 dB SPL for 500 ms. There is no spectral energy in the signals at their fundamental frequencies (F0 = 150, 200 and 250 Hz). The profiles shift systematically to the right as F0 increases. Figure 2B shows the profile for the same F0s where the tone is composed of harmonics 13–18 only. The effect of changing F0 is similar in that the profile shifts to the right as F0 increases. Despite differences in the overall shape, it is clear that the profiles in both figures discriminate easily among the three different pitches.
The left to right upward slope in Fig. 2A is explained in terms of the intrinsic chopping rates of the different modules. High chopping rates in the CN units give rise to more activity in those IC units that receive their input. It is
Fig. 2 Stage 4 rate profile for three 500-ms harmonic tones with F0=150, 200 and 250 Hz presented at 70 dB SPL. The x-axis is the saturated rate of firing of the CN units at the base of the processing chain. The y-axis is the rate of firing of the stage 4 units: A harmonics 3-8; B harmonics 13–18
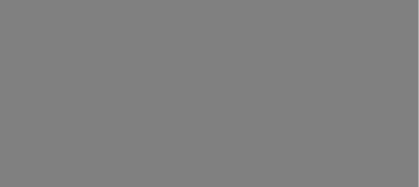
Virtual Pitch in a Computational Physiological Model |
75 |
significant that the stimuli with unresolved harmonics (Fig. 2B) do not show this continued upward slope. The horizontal nature of the function suggests that the stage 4 rate is not reflecting the intrinsic chopping rate of the CN units. In contrast, for higher F0 the plateau to the right hand of the function is higher and reflects the frequency of the envelope of the stimulus. The plateau is not present for resolved harmonics because the signal in the individual channels does not have a pronounced envelope.
4.2Inharmonic Tones
Patterson and Wightman (1976) showed that the pitch of a harmonic complex remained strong when the complex was made inharmonic by shifting the frequency of all components by an equal amount. The heard pitch of the complex shifts by a fraction of the shift of the individual components. This pitch shift is large when the stimulus is composed of low harmonics but small for complexes composed of only high harmonics. For example, Moore and Moore (2003) showed that a complex composed of three resolved harmonics would show a pitch shift of 8% when the individual harmonics were all shifted by 24%. On the other hand, a complex of three unresolved harmonics showed little measurable pitch shift. This was true for F0s of 50, 100, 200 and 400 Hz.
Moore and Moore’s stimuli were used in the following demonstration which used 70-dB SPL, 500-ms tones consisting of either three resolved harmonics (3, 4, 5) or three unresolved harmonics (13, 14, 15) with F0 = 200 Hz. Pitch shifts were produced by shifting all component tones by either 0, 24, or 48% and generating a stage 4 profile for each.
The resulting stage 4 profiles are shown in Fig. 3. The profiles for the resolved harmonics change with the shift. Shifting the frequencies of the unresolved
Fig. 3 Stage 4 rate profiles for shifted harmonic stimuli (F0 = 200 Hz). Shifts applied equally to all harmonics are 0, 24 or 48 Hz: A harmonics 4, 5 and 6 (resolved); B harmonics 13, 14 and 15 (unresolved). Shifting the harmonics has a larger effect when the harmonics are unresolved
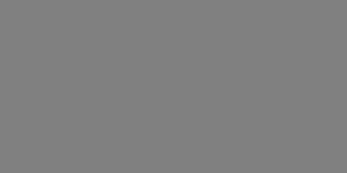
76 |
R. Meddis and L. O’Mard |
harmonics (Fig. 3B) had little effect however. Qualitatively at least, this replicates the results of Moore and Moore. When all harmonics are shifted by the same amount, the envelope of the signal is unchanged because it depends on the spacing between the harmonics which remains constant. The model reflects the unchanged periodicity of the envelope of the stimulus with unresolved harmonics in Fig. 3B. On the other hand, the model reflects the changing values of the resolved components when resolved stimuli are used (Fig. 3A).
4.3Iterated Ripple Noise
Iterated ripple noise (IRN) is of particular interest in the study of pitch because it produces a clear pitch percept but does not have the pronounced periodic envelope that is typical of harmonic and inharmonic tone complexes. When IRN is created by adding white noise to itself after a delay (d), the perceived pitch is typically matched to a pure tone or harmonic complex whose fundamental frequency is 1/d. The strength of the pitch percept is also proportional to the number of times the delay and add process is repeated. The model was evaluated using stimuli constructed using a delays of 6.67, 5 and 4 ms reciprocals and a gain of 1. These stimuli have pitches around 150, 200 and 250 Hz.
When only three iterations are used a clear shift in the stage 4 rate profile can be seen (Fig. 4A). When the number of iterations is increased, the differences become more obvious (Fig. 4B). These profiles can be compared with those produced with harmonic stimuli in Fig. 2 where the perceived pitches are the same. The comparison between IRN with 16 iterations in Fig. 4B and harmonic tones consisting of resolved components (Fig. 2A) is the most clear. However, the IRN profiles do not contain a plateau at high chopping rates previously seen in Fig. 2B. This is significant because the plateau signifies a response to the envelope of the stimulus and IRN stimuli do not have an envelope related to the perceived pitch.
Fig. 4 Stage 4 rate profiles produced by the model in response to iterated ripple noise stimuli with iteration delays corresponding to pitches of 150, 200 and 250 Hz: A 3 iterations; B 16
Virtual Pitch in a Computational Physiological Model |
77 |
5Discussion
The aim of this study was to show that a model using only physiological components could share some of the properties previously shown to characterize cross-channel autocorrelation models. The mathematical model and the new physiological model already have a great deal in common. Both use an auditory first stage to represent auditory nerve spike activity. Both extract periodicity information on a within-channel basis. Both models accumulate information across channels to produce a periodicity profile. They differ primarily only in the mechanism used to determine periodicities. In the physiological model periodicity detection uses CN units working together with their target IC units. This replaces the delay-and-multiply operations of the autocorrelation method.
The physiological mechanism depends on the synchronization properties of the model CN chopper units. When the choppers are driven by a stimulus periodicity that coincides with their intrinsic driven firing rate, all the CN units with the same firing rate will begin to fire in synchrony with the stimulus and with each other. This periodicity may originate equally from low frequency pure tones or from modulations of a carrier frequency. It is this synchrony that drives the receiving IC units. This is not exactly the same as autocorrelation, however, and it is likely that differences in the detail of the mathematical and physiological systems will produce some differences in the predictions they make. So far, however, the evaluation has not shown any major differences.
It would be premature to claim that the model described above is a complete pitch model. There are more pitch phenomena than those considered here and the new model needs to be tested on a much wider wide range of stimuli. Indeed some stimuli produce a pitch that is claimed not to be predicted by existing autocorrelation models. These are matters for further study. Nevertheless, the project has demonstrated that a cross-channel autocorrelation model can be simulated, to a first approximation, by a physiological model and is worthy of further consideration.
References
Hewitt MJ, Meddis R (1993) Regularity of cochlear nucleus stellate cells: a computational modelling study. J Acoust Soc Am 93:3390–3399
Hewitt MJ, Meddis R (1994) A computer model of amplitudemModulation sensitivity of single units in the inferior colliculus. J Acoust Soc Am 95:2145–2159
Lopez-Poveda EA, Meddis R (2001) A human nonlinear cochlear filterbank. J Acoust Soc Am 110:3107–3118
MacGregor RJ (1987) Neural and brain modeling. Academic Press, San Diego
Meddis R (2006) Auditory-nerve first-spike latency and auditory absolute threshold: a computer model. J Acoust Soc Am 119:406–417
Meddis R, O’Mard LP (1997) A unitary model of pitch perception. J Acoust Soc Am 102:1811–1820
78 |
R. Meddis and L. O’Mard |
Meddis R, O’Mard LP, Lopez-Poveda EA (2001) A computational algorithm for computing nonlinear auditory frequency selectivity. J Acoust Soc Am 109:2852–2861
Moore GA, Moore BCJ (2003) Perception of the low pitch of frequency-shifted complexes. J Acoust Soc Am 113:977–985
Patterson RD, Wightman FL (1976) Residue pitch as a function of component spacing. J Acoust Soc Am 59:1450–1459
Wiegrebe L, Meddis R (2004) The representation of periodic sounds in simulated sustained chopper units of the ventral cochlear nucleus. J Acoust Soc Am 115:1207–1218
Comment by Kollmeier
Your neural circuits seems to implement a modulation filterbank in a similar way to that in the Dissertation by Ulrike Dicke (Neural models of modulation frequency analysis in the auditory system, Universität Oldenburg, 2003, download at http://docserver.bis.uni-oldenburg.de/publikationen/dissertation/2004/ dicneu03/dicneu03.html) and Dicke et al. (2006). However, in your model the modulation tuning is critically dependent on the time constant of the model chopper unit which may not be a stable physiological quantity of a single cell and is also unlikely to vary over several octaves (as would be required to predict physiological and psychoacoustical data). In contrast, the Dicke approach uses a neural circuit that does not employ a continuously changing temporal parameter to obtain different best modulation frequencies (BMFs) of the IC modulation bandpass units. Instead, different BMFs are yielded from varying the number of input units projecting onto different bandpass units.
What evidence do you have that the chopper unit time constant is a stable and scalable property across different cells as opposed to the assumption that the modulation tuning is a network property rather than a property of a single neuron?
References
Dicke U, Ewert S, Dau T, Kollmeier B (2006) A neural circuit transforming temporal periodicity information into a rate-based representation in the mammalian auditory system (submitted)
Reply
We agree that there are aspects of the CN model that need further investigation. For example, it might be that the intrinsic chopping rate of a unit is controlled by some other factor such as the number of input AN fibers or inhibitory modulation. We are currently investigating this issue. We are also investigating the question of whether a full range of chopping frequencies is necessary to simulate the pitch results. These are open questions.