
Учебники / Hearing - From Sensory Processing to Perception Kollmeier 2007
.pdf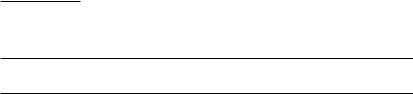
4 A Model of Ventral Cochlear Nucleus Units Based on First Order Intervals
STEFAN BLEECK1 AND IAN WINTER2
1Introduction
In the presence of a constant stimulus the arrival time of a spike has little meaning except in the context of the time of the preceding spike. Electrophysiological studies reveal a surprising degree of variability in neurons firing behaviour which can be described by the probability distribution function of consecutive spikes, the inter-spike intervals (ISI). A histogram (ISIH) can be constructed to represent the ISI-distribution (ISID) of intervals and a probability density function (PDF) of the intervals can be modelled by fitting it to the histogram. The continuous nature of the PDF is attractive since it avoids an arbitrary resolution of spike quantisation.
There is no generally accepted PDF to model ISIHs. Interval distributions that were fitted, with more or less success, to ISIHs of constant stimuli include normal, log normal, exponential, gamma, Weibull, bimodal, multimodal. With the exception of the normal distribution, these functions are asymmetric (due to their positive skewness) reflecting the tendency of slower firing neurons to show greater variability. These functions are all empirical fits and cannot be fully justified physiologically – exponential PDFs (which are a result of a Poisson process) can only fit parts of the curve and gamma functions (which are a result of a coincidence detector) ignore the decay times of EPSPs – and consequently there are many other possible candidates for empirical fitting functions.
Neuronal processing and all models based on physiological and biophysical bases include many noise sources that jitter the occurrences of spikes. These noise sources include the number and size of input synapses; the precise arrival time of input spikes and the location of synapses on dendrites and the thickness of dendrites which lead to differences in amplitude, width and time of arrival of EPSPs and IPSPs at the axon hillock. It is very difficult, if not impossible, to measure the influence of all these noise sources experimentally; therefore we have to rely on assumptions and simulations to investigate them in detail.
1Institute of Sound and Vibration Research, University of Southampton, UK, bleeck@gmail.com 2Department of Physiology, Development and Neuroscience, University of Cambridge, UK, imw1001@cam.ac.uk
Hearing – From Sensory Processing to Perception
B. Kollmeier, G. Klump, V. Hohmann, U. Langemann, M. Mauermann, S. Uppenkamp, and J. Verhey (Eds.) © Springer-Verlag Berlin Heidelberg 2007

28 |
S. Bleeck and I. Winter |
The idea that is developed in this chapter provides a possible solution to this problem. We demonstrate here that the huge number of noise sources can actually reduce the complexity of the system behaviour.
The “central limit theorem” states that any sum of many independent identically distributed random variables will be distributed normally (independent of the original distribution). The according theorem for probability distributions for our argument was formulated by Fischer and Tippett (1928) as the “central limit theorem of extreme value distributions”: the extreme values of many independent identically distributed random variables will take the form of an “extreme value distribution” (EVD) (independent of the original distribution). The EVD is a valid model under the following conditions: The membrane potential is affected in a complicated manner by many random processes. The event when the membrane potential exceeds the threshold can be seen as “extreme”, when the threshold is high compared to the noisy resting potential. This can be shown by intracellular recordings (Calvin and Stevens 1967). Furthermore, the resting potential and the underlying probability density function for spike generating must be stationary. To apply the EV theorem we do not have to know or assume any (physiologically motivated) models of the underlying spike generating process.
The resulting spike generating process then takes on the form of a Markov process in which the probability of the next spike explicitly depends on its immediate predecessor but is conditionally independent of all other preceding spikes (Tuckwell 1988). The shape of the PDF of such a process is determined by the EVD.
2Methods
All physiological recordings were obtained from the cochlear nucleus of the anaesthetized guinea pig (Cavia porcellus). The procedures are described in detail in Bleeck et al. (2006).
The normalised cumulative first order interval distributions of half the number of all intervals between stimulus onset and offset was fitted by a maximum likelihood method with the generalised extreme value distribution:
Z |
R |
|
V- |
1 |
_ |
] |
log (x) - a |
|
ζb |
||
] |
S |
W |
|
b |
|
F (x | a, b, ζ ) = exp [-S1 + ζ f |
|
pW |
|
` |
|
b |
|
||||
] |
S |
W |
|
b |
|
T |
|
X |
|
||
\ |
|
|
|
|
a |
where τ is the time since the last spike (ISI), a the its location, b the scale
and ζ the shape of the distribution. The shape parameter ζ governs the tail behaviour of the distribution, and the sub-families defined by ζ>0, ζ→0 and ζ <0 correspond, respectively, to the Fréchet, Gumbel and Weibull families, whose cumulative distributions are shown in Fig. 1. The quality of the
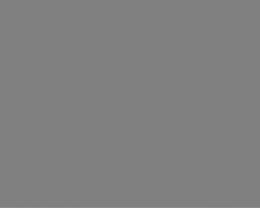
A Model of Ventral Cochlear Nucleus Units Based on First Order Intervals |
29 |
Fig. 1 The three families of extreme value distributions that constitute the generalized extreme value distribution. Note the different tail behaviour
fitting procedure was tested by performing a Kolgomorov-Smirnov test with a confidence interval of 5%. The other half of the intervals (that were not used for the fit) were used as the comparison distribution with the resulting fitting function.
3Results
In total, 1867 ISIHs from 877 units were investigated. A subset of these (323 ISIHs from 184 units) were also classified by one of the authors (IMW) using conventional criteria such as PSTH shape and regularity analysis (Young et al. 1988). The eight classification categories were: sustained chopper (CS), transient chopper (CT), wide chopper (CW), primary-like (PL), primary-like with notch (PN), onset (ON), low frequency (LF) and unusual (UN). Figure 2 shows data of six example units. The panels for each unit show a) the Post Stimulus Time Histogram (PSTH), b) the first order interval distribution (FOID) – the black line represents the GEV-fit to the data, c) the normalised
cumulative FOID and the fit, d) the hazard function PSpike=F′/(1−F) which is the probability that a spike happens after that interval, and the calculated
hazard function (calculated by the fitting parameters), and e) reconstruction of the PSTH using the hazard function. Neuronal responses to pure tones were simulated for 250 sweeps. At every point in time the probability that a spike happens is calculated as a Markov process given the values of µ, σ, ζ and the time of the last spike.
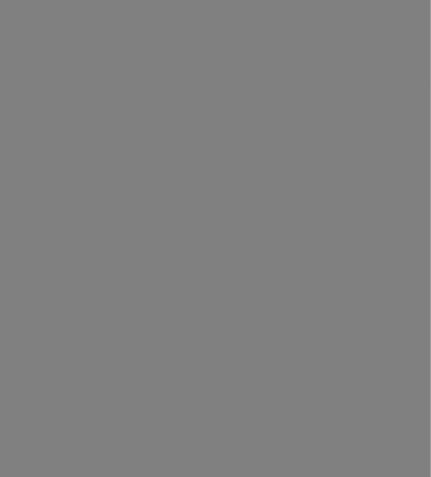
30 |
S. Bleeck and I. Winter |
Fig. 2 PSTH (time in ms), FOID, recovery function, hazard functions (time interval in ms) and reconstructions (time in ms) for six example neurons of different types. “Chop S” unit (characterized by very regular firing) has a relatively sharp FOID. In effect its recovery function is steep. “Chop T” unit (less regular firing pattern then Chop S) has a less steep recovery function. The hazard functions is complex. “Primary like” (similar to an auditory nerve fibre) and “Primary like with Notch” (characterized by a distinct notch after the initial peak) units show slower recovery and almost linear Hazard functions. Their FOID is very broad. “Onset” unit (has a low sustained rate) have a delayed recovery function and a hazard function that is slowly rising in the beginning and the very steep. “Unusual” units have different characteristics that prevent them from being classified as one of the others. The example shown here has recovery properties that places it between Chopper and Onset
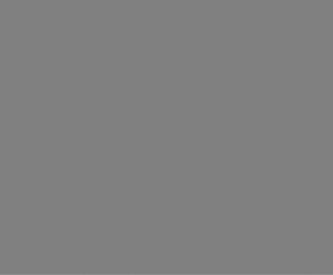
A Model of Ventral Cochlear Nucleus Units Based on First Order Intervals |
31 |
3.1Population Overview
Figure 3 shows the distribution of mean and standard deviation for fits to 1867 ISIHs from 877 units. Each histogram is represented by a symbol. The open symbols in the foreground represent the identity of the classified units. The grey circles in the background represent all other units that were not classified. The classified units mainly occupy different, although overlapping, areas. An “automatic classification” algorithm finds the mean of the parameter values of the response type that is closest. It classifies as the same by both the model and experimenter: 100% CS, 93% CT, 100% CW, 73% PL, 60% PN and 71% ON. Most “unusual” units are in areas not occupied by the major classes.
The Kolgomorov Smirnov test reveals that the logarithmic GEV fit was successful in 43% of all cases. This is significantly (p < 0.01) better than other distributions tested (GEV linear, gamma logarithmic and linear, Weibull logarithmic and linear, exponential, normal logarithmic and linear). Due to adaptation during the stimulus duration, the exact shape of the fitting function changes. If only intervals during parts of the stimulus are considered (e.g. the first 10 ms) the number of successfully fitted units is up to 78%.
Fig. 3 Population plot of the means and variances of all fits. Each dot indicates the fit of the recovery function of one unit to the fit. The open symbols correspond to a classified type, the grey circles in the background represent all units. the mean and standard deviation of each population is indicated by the black lines
32 |
S. Bleeck and I. Winter |
3.2The Neurons Journey Through Parameter Space
During the course of 50 ms stimulus the neurons behaviour and hence the fitting parameter changes due to adaptive processes (Fairhall et al. 2001). Not only the ISIs during the stimulus but also the latency of the first spike after stimulus onset and the firing patterns without a stimulus (spontaneous activity) can be described adequately using a GEV-fit. The number of neurons that can be fitted successfully as such are comparable to the interstimulus fit. The firing probability of a neuron during a stimulus (and also as a function of stimulus parameter like level) can therefore be traced in the parameter space spanned by the fitting parameters (data now shown).
4Discussion
Since the coding capacity of a spike train may be reflected in both the profile and the asymmetric dispersion of the ISIH, an information approach can be used to overcome the limitation of traditional second moment statistics. Recently, inter-spike intervals have formed the basis for quantifying the coding capacity of spike trains. Numerical methods have been developed based on logarithmic (Bhumbra et al. 2004) and linear (Reeke and Coop 2004) intervals. These measures suffer, however, from the absence of a generally accepted form of the distribution and must be calculated numerically for every case. It would therefore be extremely useful to have a general model to describe ISIHs as this allows direct access to the information content of spike trains.
Distinct cell types in the VCN can be associated with almost unique temporal response patterns (Rhode et al. 1983). However, we show here a method to represent neuronal responses in the VCN within overlapping parameter areas. The continuous filling of the space in the parameter map indicates that the different neuron classes have overlapping features. Therefore we argue here that it might be useful to reconsider unit classification as continuous in the descriptive parameter space rather then in distinct classes.
5Summary
The automatic algorithm allows classification of a neuron with good accuracy. Most of the errors made by the algorithm are actually between unit types that are similar by visual inspection as well. Additionally the automatic classifier is faster: Usually 40–100 intervals are enough to get a stable classification (data not shown). Analysed conventionally, many units are classified as unusual because they do not fit completely into the classic classification scheme. The
A Model of Ventral Cochlear Nucleus Units Based on First Order Intervals |
33 |
GEV fitting reveals that many of these neurons are actually highly regular in terms of their firing patterns and might well described in a continuous parameter space.
The spike probability at each time can be described by the hazard function as a function of the two main parameters of the ISI-distribution. The change of the ISI-distribution can be visualised as a journey through parameter space.
The advantage of using the extreme value distribution is that the outcome is determined by the statistical laws of big numbers independent of the actual distributions and properties of the underlying processes. It is nevertheless still possible to infer certain neuronal characteristics from the results. For instance the shape ζ is mainly constant for processes like amplitude changes and adaptation (data not shown). The location α and the scale β on the other hand are determined by the stimulus: the louder the stimulus, the smaller α, the longer its duration, the bigger β.
Acknowledgements. Supported by a grant to the second author from the BBSRC
References
Bhumbra GS, Dyball REJ (2004) Measuring spike coding in the rat supraoptic nucleus. J Physiol (London) 555(1):281–296
Bleeck S, Sayles M, Ingham NJ, Winter IM (2006) The time course of recovery from suppression and facilitation from single units in the mammalian cochlear nucleus. Hear Res 212:176–184 Calvin WH, Stevens CF (1967) Synaptic noise as a source of variability in the interval between
action potentials. Science 155(764):842–844
Fairhall AL, Lewen GD, Bialek W, de Ruyter Van Steveninck RR (2001) Efficiency and ambiguity in an adaptive neural code. Nature 412:787–792
Fisher RA, Tippett LHC (1928) Limiting forms of the frequency distribution of the largest or smallest member of a sample. Proc Cambridge Philos Soc 24:180–190
Reeke GN, Coop AD (2004) Estimating the temporal interval entropy of neuronal discharge. Neural Comput 16(5):941–970
Rhode WS, Oertel D, Smith PH (1983) Physiological response properties of cells labelled intracellularly with horseradish peroxidase in cat ventral cochlear nucleus. J Comp Neurol 213(4):448–463
Tuckwell HC (1988) Introduction to theoretical neurobiology: vol 2 – Nonlinear and stochastic theories. Cambridge University Press
Young ED, Robert JM, Shofner WP (1988) Regularity and latency of units in ventral cochlear nucleus – implications for unit classification and generation of response properties. J Neurophysiol 60:1–29
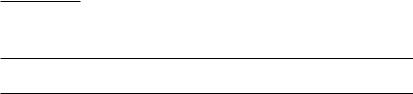
5 The Effect of Reverberation on the Temporal Representation of the F0 of Frequency Swept Harmonic Complexes in the Ventral Cochlear Nucleus
MARK SAYLES1, BERT SCHOUTEN2, NEIL J. INGHAM1, AND IAN M. WINTER1
1Introduction
When listening in an enclosed space, part of the sound has travelled to the listener directly from its source. In addition the listener receives multiple delayed and attenuated copies of the sound as it is reflected from the room’s surfaces, an effect referred to as reverberation. This series of reflections has a filtering effect, introducing distortion in both the spectral and temporal domains. Spectral transitions are smeared in time and the introduction of slowly decaying “tails” effectively applies a low-pass filter to the temporal envelope. Since each reflection is added back to the original “direct” sound with random phase, envelope periodicity tends to be disrupted.
Although reverberation is often exploited as a means of delivering the necessary sound level to audiences in auditoria it has long been acknowledged that the resulting distortion can have a deleterious effect on the intelligibility of complex time-varying stimuli such as speech (Knudsen 1929; Santon 1976; Nàb˘elek et al. 1989). Human psychophysical studies (Culling et al. 1994) have demonstrated that the combination of relatively mild reverberation and fundamental (F0) frequency modulation at rates commonly found in speech disrupts listeners’ ability to exploit differences in F0 to perceptually segregate two competing sound sources.
Despite the obvious importance of reverberation in the intelligibility of speech, to our knowledge this has yet to be explored from a physiological perspective. As a first attempt at understanding the effects of reverberation on the representation of complex sounds in the mammalian auditory system we have recorded the responses of single units in the ventral cochlear nucleus to the F0 of frequency swept harmonic complexes with and without reverberation. Here we show that in many single units in the ventral cochlear nucleus (VCN) reverberation degrades the temporal representation of F0. Only units responding to resolved harmonics are able to maintain a representation of the F0 through all reverberation conditions. These results are consistent with the hypothesis that reverberation disrupts the phase
1Centre for the Neural Basis of Hearing, The Physiological Laboratory, Downing Street, Cambridge, UK, imw1001@cam.ac.uk, ms417@cam.ac.uk, nji21@cam.ac.uk
2Universiteit Utrecht, The Netherlands, bert.schouten@let.uu.nl.
Hearing – From Sensory Processing to Perception
B. Kollmeier, G. Klump, V. Hohmann, U. Langemann, M. Mauermann, S. Uppenkamp, and J. Verhey (Eds.) © Springer-Verlag Berlin Heidelberg 2007
36 |
M. Sayles et al. |
relationship between unresolved harmonics sufficiently to abolish temporal responses to envelope periodicity. It is suggested that, under reverberant conditions, temporal information is limited to frequency channels containing resolved harmonics.
2Methods
Detailed methods describing the surgical approach and recording techniques can be found elsewhere (e.g. Bleeck et al. 2006) and will be briefly described below.
2.1Physiology and Recording
The data reported in this chapter were recorded from pigmented guinea pigs anaesthetised with urethane (1.0 g/kg i.p.). Supplementary analgesia was provided by Hypnorm (fentanyl/fluanisone) (1.0 ml/kg i.m.). Additional doses of urethane and Hypnorm were given when required. The surgical preparation and stimulus presentation took place in a sound attenuating chamber (IAC). All animals were tracheotomised and core temperature was maintained at 38ºC with a thermostatically controlled heating blanket. The electrocardiogram and respiration rate were monitored throughout and on signs of suppressed respiration the animal was artificially ventilated.
Recordings were made using tungsten-in-glass microelectrodes (Merrill and Ainsworth 1972). Broadband noise was used as a search stimulus. Upon isolation of a single unit, estimates of best frequency (BF) and threshold were made using audio-visual criteria. Single units were classified according to peri-stimulus time histogram shape in response to suprathreshold BF tonebursts, interspike interval distribution and discharge regularity.
2.2Complex Stimuli
The stimuli were linearly swept harmonic complex tones summed in cosine phase (Fig. 1). The complexes were constructed from harmonics 1–20 with starting F0 frequencies spaced at 1/3 octave intervals between 100 and 400 Hz and ending F0 frequencies one octave above that at the start of the sweep. Sweep duration was 500 ms.
Each sweep was convolved with impulse responses provided to us by Dr Tony Watkins (University of Reading). These were recorded at 0.32, 0.63, 1.25, 2.5, 5.0 and 10.0 m from a sound source in a corridor measuring approximately 2 m wide, 35 m long and with a ceiling height of 3.4 m and are the same impulse responses as used in previous psychophysical studies of
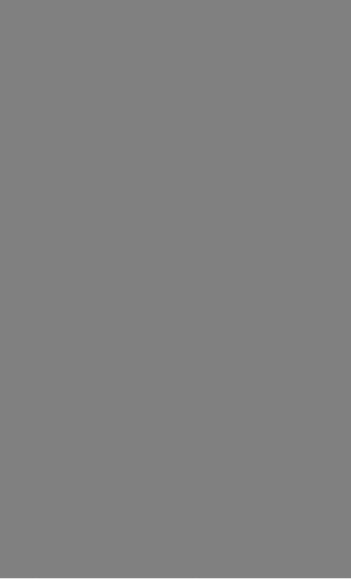
The Effect of Reverberation on the Temporal Representation of the F0 |
37 |
Fig. 1 Waveforms and spectrograms of harmonic complex sweeps with F0 100–200 Hz
reverberation (Watkins 2005). In this chapter we show only responses to the dry condition and the 32, 125, and 500 cm distance conditions. All stimuli were normalised after convolution for equal rms voltage and were presented monaurally with typically 25 repetitions and a 1 s repetition period. All stimuli were gated on and off with a 1 ms cos2 ramp.