
Учебники / Hearing - From Sensory Processing to Perception Kollmeier 2007
.pdf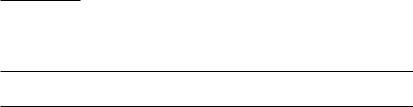
15 Psychophysically Driven Studies of Responses to Amplitude Modulation in the Inferior Colliculus: Comparing Single-Unit Physiology to Behavioral Performance
PAUL C. NELSON1 AND LAUREL H. CARNEY1,2
1Introduction
Psychophysical envelope processing has received renewed attention, largely in response to the success of the modulation filterbank model (Dau et al. 1997) in predicting perceptual data that are difficult to account for with the classical modulation low-pass filter (Viemeister 1979). In many behavioral studies, the stimulus modulation depth (m) is adaptively varied to determine thresholds for detection and discrimination of amplitude-modulated (AM) signals. To investigate directly and quantitatively the role of the inferior colliculus (IC) in the processing of such sounds, we recorded single-unit extracellular responses to AM stimuli with a wide range of modulation depths (from below psychoacoustic detection thresholds to 100% modulation), and in some neurons with resolution in depth finer than behavioral discrimination performance (~1–2 dB). Neural responses were quantified in terms of average firing rate and synchronization to the envelope; the significance of changes in the different metrics was determined by the slope of modulation depth functions (MDFs) and the across-repetition variability of the given response quantification (Nelson 2006).
Similar approaches have been used to relate auditory-nerve (AN) responses to audio-frequency tone detection and intensity discrimination (Siebert 1968; Young and Barta 1986; Delgutte 1987; Viemeister 1988; Colburn et al. 2003). The most sensitive (high spontaneous-rate) AN fibers exhibit rate changes at tone levels in line with psychophysical detection thresholds (e.g. Young and Barta 1986), while fine-structure phase-locking can emerge in fibers with low characteristic frequencies at SPLs 10–20 dB lower than those required to elicit a rate increase (Johnson 1980). Intensity discrimination thresholds at mid to high SPLs are more difficult to account for with single-fiber average-rate analyses because of saturation and increased spike-count variance at high SPLs (Colburn et al. 2003). Several schemes have been proposed to offset these effects, including (1) pooling of
1Department of Biomedical and Chemical Engineering and Institute for Sensory Research, Syracuse University, Syracuse, NY, USA, pcnelson@syr.edu
2Department of Electrical Engineering and Computer Science, Syracuse University, Syracuse, NY, USA, lacarney@syr.edu
Hearing – From Sensory Processing to Perception
B. Kollmeier, G. Klump, V. Hohmann, U. Langemann, M. Mauermann, S. Uppenkamp, and J. Verhey (Eds.) © Springer-Verlag Berlin Heidelberg 2007
134 |
P.C. Nelson and L.H. Carney |
rate responses (Delgutte 1987; Viemeister 1988), (2) spread of excitation (Siebert 1968; Heinz et al. 2001), and (3) the use of level-dependent response phase for low-frequency AN fibers (Colburn et al. 2003).
AM detection and discrimination performance predicted with central neural responses suggests a fundamentally different representation of modulation depth in the IC as compared to the representation of tone level in the AN. Specifically, at low modulation depths, changes in average rate cannot account for perceptual thresholds, while synchrony to the envelope can be significant at these low depths. In contrast, for higher modulation depths, rate-based MDFs are not as prone to saturation, whereas vector strength tends to saturate in most neurons and phase is not systematically depthdependent (Krishna and Semple 2000; Nelson 2006). These properties of the synchronized response are inconsistent with behavioral depth-discrimination thresholds which remain approximately constant above about −25 dB (Ewert and Dau 2004). In other words, a qualitative description of IC responses to AM suggests a transition from a temporal code at low depths to a rate-based code at high depths.
The current analysis was designed to further characterize IC responses with two specific encoding strategies in mind. The first hypothesis is that rate-based neural detection thresholds might improve by pooling information across a number of cells. The second hypothesis is that a single-neuron decision statistic incorporating both rate and temporal information will predict thresholds across a wider portion of the perceptually relevant range of AM depths than rate or synchrony alone.
2Methods
Detailed descriptions of the experimental methods are available elsewhere (Batra et al. 1989; Nelson 2006). Briefly, single-unit extracellular recordings over a wide range of AM depths were obtained in 152 single neurons in the ICs of three awake female Dutch-belted rabbits (oryctolagus cuniculus). Glass-coated tungsten microelectrodes were positioned with a stereotaxic system mounted on a steel cylinder that was affixed to the animal’s skull.
Parameters of the AM stimuli were designed for each neuron based on responses to a battery of simpler sounds. The best-frequency-tone carrier had an SPL on the ascending portion of the rate-level function. Sinusoidal AM was at the modulation frequency (fm) that elicited the largest value of synchronized rate (the product of vector strength and average rate) over a range of fm from 2 to 312 Hz. This fm almost always corresponded to the frequency that resulted in the highest value of the Rayleigh statistic (2nVS2, where n is the number of spikes and VS is the magnitude of vector strength at fm). Three repetitions of 2 s duration AM tones were presented at each tested frequency and depth. For statistical purposes, the responses were
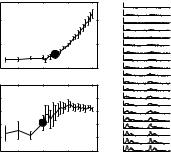
Psychophysically Driven Studies of Responses to Amplitude Modulation |
135 |
analyzed in 500-ms segments, omitting the initial 500 ms, resulting in nine estimates of each response metric at each fm and m. Neglecting the onset response avoided the calculation of significant synchrony to the AM period based solely on a transient burst at stimulus onset, but did not strongly affect the quantifications of most neural responses, which usually exhibited little temporal adaptation to AM stimulation. A t-test was used to establish significant differences in rates to variations in AM depth. The lowest modulation depth that resulted in a significant value of vector strength (Rayleigh statistic >13.8, p<0.001) was defined as synchrony threshold. Statistical analyses involving comparisons of two different synchrony measures took advantage of a transformation [–ln(1–VS)] that results in uniform variance of VS (Johnson 1974; Joris et al. 1994).
3Results
3.1Example Single-Unit Responses
Rateand synchrony-MDFs for one IC neuron are shown in Fig. 1, with period histograms of the spike times over a wide range of AM depths. Human AM detection thresholds for similar stimulus conditions approach −30 dB (e.g. Kohlrausch et al. 2000). Filled circles correspond to neural detection thresholds, or the first values of rate or synchrony that were significantly different from the response at the lowest tested depth (−35 dB). This cell had a rate threshold of −15 dB and a synchrony threshold of −20 dB. We consistently observed lower thresholds based on synchrony than on rate (93% of synchrony
) |
|
|
|
|
|
−1 |
|
|
|
|
|
(s |
200 |
|
|
|
|
rate |
|
|
|
|
|
|
|
|
|
|
|
average |
100 |
|
|
|
|
0 |
−30 |
−20 |
−10 |
0 |
|
|
1 |
||||
|
|
|
|
|
|
synchrony |
0.8 |
|
|
|
|
0.6 |
|
|
|
|
|
0.4 |
|
|
|
|
|
0.2 |
|
|
|
|
|
|
|
|
|
|
|
|
0 |
−30 −20 |
−10 |
0 |
|
|
|
||||
|
|
|
20 log m |
|
−15
−10
−5
0 |
0 1 2 cycle #
Fig. 1 Single-neuron response dependence on AM depth in terms of average rate, strength of synchrony, and raw period histograms (plotted twice for visual clarity). Error bars represent standard deviations across repetitions. Stimulus fm was 112 Hz, and the carrier was a 60-dB SPL, 3900-Hz tone
136 |
P.C. Nelson and L.H. Carney |
thresholds were equal to or lower than their rate-based counterparts; Nelson 2006). For supra-threshold AM depths, this cell’s average rate increased monotonically over the remaining 15-dB dynamic range.
In contrast, its VS was effectively constant for depths higher than −10 dB; the period histograms illustrate the emergence of a bimodal distribution of spike times that contributed to the saturation of this timing metric. Figure 1 also shows the across-repetition variability of rate and synchrony estimates as a function of stimulus m. Rate estimates were slightly more variable at higher driven rates (and higher m) in this neuron than at lower rates, while the variability of VS showed the opposite trend. The enhanced precision of VS at high depths was mainly a result of the increase in the number of spikes, as opposed to a transition into the compressive region of the metric’s dynamic range. Further analysis (not shown) supported this notion: mean VS did not change, but its variability increased, when spikes were deleted to match the average rates at different modulation depths.
3.2Relationship Between Mean Count and Count Variance
One strategy used in comparisons of AN responses to intensity discrimination psychophysical thresholds is the simulation of a population of fibers based on assumed rate functions and dependence of spike count variance on the mean spike count (e.g. Delgutte 1987; Viemeister 1988). This approach provides an estimate of the number of fibers with a stereotypical rate function that would be required to account for psychophysical performance, and is justifiable for peripheral responses because of the systematic variability of count estimates in the AN (Young and Barta 1986; Winter and Palmer 1991): count variance is slightly less than that expected from a Poisson process. Here, we show that a similar strategy is not appropriate in the IC because rate variability is not predictable from the number of spikes.
Count variance as a function of the mean count for the population of neurons tested with a range of modulation depths (1168 observations) is shown in Fig. 2. The thin line in Fig. 2 indicates the dependence that would be expected from a Poisson process, while the thicker lines represent the best linear fit to the data (in a minimized sum-of-squares error sense). The slope of the best-fit line is approximately 0.8 (consistent with Hancock and Delgutte 2004); however, the raw data make it clear that a simple relationship between variance and count does not adequately describe these data.
In contrast to more peripheral auditory neurons (see above) and visual cortical neurons (e.g. Geisler and Albrecht 1997), the spike-count variance of IC neurons in the awake rabbit is clearly not proportional to the mean count. An inspection of variance-vs-count functions for individual neurons also revealed no systematic relationship for most cells (not shown). The neuron illustrated in Fig. 1 was atypical in this sense, in that its spike-count variance increased monotonically with firing rate.
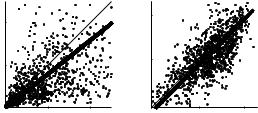
Psychophysically Driven Studies of Responses to Amplitude Modulation |
137 |
variance |
40 |
|
|
100 |
|
|
|
|
|
|
|
||
20 |
|
|
10 |
|
|
|
count |
|
|
|
|
||
|
|
|
|
|
||
|
|
|
|
|
|
|
|
0 |
|
|
1 |
|
|
|
|
|
|
|
|
|
|
0 |
20 |
40 |
1 |
10 |
100 |
mean spike count in 500 ms
Fig. 2 Spike count variance is not systematically dependent on the mean count in the IC. Linear axes are used in the left panel, focusing on measurements with counts and variances less than 50 (939/1168 observations); the logarithmic scale in the right panel allows for almost the entire population to be included (1115/1168)
The count-variance dependence of several subsets of cells was examined; no obvious differences were observed between groups with different puretone histogram types (onset or sustained), or rate-based sensitivities to AM depth (low versus high neural detection thresholds). Also, there were no clear trends that suggested a difference in this relationship for responses to stimuli with low vs high modulation depths (responses to all depths are included in Fig. 2).
3.3Combining Rate and Timing Information
The fact that synchronization to the envelope can become significant in the IC at AM depths near psychophysical thresholds and average rate often increases monotonically at higher values of m points towards a hybrid (e.g. synchronized-rate) metric as a decision statistic with the potential to account for perceptual thresholds across the entire AM depth dynamic range. We tested this idea by quantifying the average rate, synchrony, and the product of synchrony and rate. A transformation of synchrony, –ln(1–VS), compensated for the compressive nature of the vector strength metric and resulted in an equal-variance synchrony axis (Joris et al. 1994). Note that this transformation does not alter the count-dependence of across-repetition synchrony variance (Fig. 1).
Neural AM depth discrimination thresholds of one neuron for each tested standard depth and response metric are shown in Fig. 3; for reference, human psychophysical thresholds for high carrier frequencies and fm below 150 Hz are also included in the figure (from Ewert and Dau 2004). Two features of the neural thresholds are worth noting. First, predicted performance was essentially the same for all three of the tested decision statistics. Second, the trend toward higher thresholds at standard depths below −10 dB in the neural predictions was inconsistent with the human listeners’ ability to discriminate
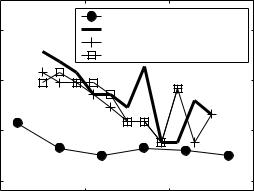
138 |
P.C. Nelson and L.H. Carney |
|
|
|
psychophysical thresholds |
|
|
20 |
|
rate |
|
) |
|
|
synchronized rate |
|
2 |
|
|
|
|
s |
|
|
synchrony |
|
)/m |
|
|
|
|
|
|
|
|
|
s |
|
|
|
|
2 |
10 |
|
|
|
m |
|
|
|
|
− |
|
|
|
|
c |
|
|
|
|
2 |
|
|
|
|
log ((m |
0 |
|
|
|
10 |
|
|
|
|
|
−10 |
|
|
|
|
−30 |
−20 |
−10 |
0 |
|
|
|
20 log ms |
|
Fig. 3 Comparison of neural and psychophysical AM depth discrimination thresholds across a wide range of standard depths. Perceptual data from Ewert and Dau (2004)
small changes in m for all standard depths above approximately −25 dB. Qualitatively similar results were observed for the 19 other neurons examined with high depth resolution.
One interpretation of these results (Fig. 3) is that a simple combination of rate and timing information does not carry adequate information to account for the low-depth psychophysical thresholds, as hypothesized above. Although synchrony was significant in the neuron at a depth of −19 dB, the variability of both synchrony and synchronized rate at depths near threshold was too high with respect to the slope of the modulation depth functions to predict the perceptual 1- or 2-dB discrimination thresholds (−6 to −2 dB on the ordinate axes shown in Fig. 3).
4Summary and Conclusions
The neural representation of AM stimuli in the IC was examined in terms of the mean and variance of several response quantifications. Spike-count variability had no systematic relationship with mean count. This finding has implications for modeling strategies that pool rate responses across a population of neurons. Rate codes are also affected by several other fundamental issues that raise serious questions regarding the feasibility of proposed decoding algorithms. For instance, spike counts computed over a finite duration depend on both the duration of the counting window and the absolute position of the window in time. Neural discharge rates exhibit temporal
Psychophysically Driven Studies of Responses to Amplitude Modulation |
139 |
correlations that can be modeled with a long-range dependent point process (e.g. Jackson and Carney 2005); therefore, to make efficient use of short-term changes in average rate, these slow temporal fluctuations must be correlated across populations of neurons that converge at a higher point in the system. There is no strong evidence either for or against the existence of such correlations in the central auditory system.
Although we conclude that a strict rate code is probably not used in the IC to represent AM (at least at low AM depths), we were also not able to identify an alternative single-neuron decision statistic that was capable of accounting for human performance across the entire perceptually relevant dynamic range of AM depths. The variability associated with estimates of VS computed using small numbers of spikes overwhelms the systematic changes in timing-based metrics that are present at lower AM depths. Taken together, these findings suggest one of three possibilities: (1) differences in percepts elicited by variations of AM depth are not directly mediated by the activity of single IC neurons, (2) rabbits and humans experience different AM-induced sensations, or (3) the system uses another single-neuron response metric that has yet to be identified. Future work will address these issues with simultaneous recordings of populations of IC neurons, measurement of behavioral rabbit AM detection and discrimination thresholds, and the testing of additional response quantifications.
Acknowledgements. We thank Anita Sterns for technical assistance and Shigeyuki Kuwada and Blagoje Filipovic for their generous contributions of time and electrode-making advice. This research was supported by NIHNIDCD F31-7268 (PCN) and NIH-NIDCD R01-01641 (LHC).
References
Batra R, Kuwada S, Stanford TR (1989) Temporal coding of envelopes and their interaural delays in the inferior colliculus of the unanesthetized rabbit. J Neurophysiol 61:257–268 Colburn HS, Carney LH, Heinz MG (2003) Quantifying the information in auditory-nerve
responses for level discrimination. J Assoc Res Otolaryngol 4:294–311
Dau T, Kollmeier B, Kohlrausch A (1997) Modeling auditory processing of amplitude modulation. I. Detection and masking with narrow-band carriers. J Acoust Soc Am 102:2892–2905 Delgutte B (1987) Peripheral auditory processing of speech information: implications from a physiological study of intensity discrimination. In: Schouten MEH (ed) The psychophysics
of speech perception. Dordrecht, Nijhoff, pp 333–353
Ewert SD, Dau T (2004) External and internal limitations in amplitude-modulation processing. J Acoust Soc Am 116:478–490
Geisler WS, Albrecht DG (1997) Visual cortex neurons in monkeys and cats: detection, discrimination, and identification. Vis Neurosci 14:897–919
Hancock KE, Delgutte B (2004) A physiologically based model of interaural time difference discrimination. J Neurosci 24:7110–7117
Heinz MG, Colburn HS, Carney LH (2001) Rate and timing cues associated with the cochlear amplifier: level discrimination based on monaural cross-frequency coincidence detection. J Acoust Soc Am 110:2065–2084
140 |
P.C. Nelson and L.H. Carney |
Jackson BS, Carney LH (2005) The spontaneous-rate histogram of the auditory nerve can be explained by only two or three spontaneous rates and long-range dependence. J Assoc Res Otolaryngol 6:148–159
Johnson DH (1974) The response of single auditory-nerve fibers in the cat to single tones: synchrony and average discharge rate. PhD dissertation, Cambridge, MA, MIT
Johnson DH (1980) The relationship between spike rate and synchrony in responses of auditory-nerve fibers to single tones. J Acoust Soc Am 68:1115–1122
Joris PX, Carney LH, Smith PH, Yin TCT (1994) Enhancement of neural synchronization in the anteroventral cochlear nucleus. I. Responses to tones at the characteristic frequency. J Neurophysiol 71:1022–1051
Kohlrausch A, Fassel R, Dau T (2000) The influence of carrier level and frequency on modulation and beat-detection thresholds for sinusoidal carriers. J Acoust Soc Am 108:723–734 Krishna BS, Semple MN (2000) Auditory temporal processing: responses to sinusoidally amplitude-
modulated tones in the inferior colliculus. J Neurophysiol 84:255–273
Nelson PC (2006) Physiological correlates of temporal envelope perception. PhD dissertation, Syracuse University
Siebert WM (1968) Stimulus transformations in the peripheral auditory system. In: Kolers PA (ed), Recognizing patterns. MIT Press, Cambridge, MA, pp 104–133
Viemeister NF (1979) Temporal modulation transfer functions based upon modulation thresholds. J Acoust Soc Am 66:1364–1380
Viemeister NF (1988) Intensity coding and the dynamic range problem. Hear Res 34:267–274 Winter IM, Palmer AR (1991) Intensity coding in low-frequency auditory-nerve fibers of the
guinea pig. J Acoust Soc Am 90:1958–1967
Young ED, Barta PE (1986) Rate responses of auditory nerve fibers to tones in noise near masked threshold. J Acoust Soc Am 79:426–442
Comment by Verhey
Would your data be in line with the following two interpretations?
1.The most sensitive units show similar thresholds as the psychophysical data on modulation detection.
2.Units with different thresholds are sensitive to different ranges of modulation depths, i.e. for the modulation discrimination task units may be used by the auditory system which have their thresholds close to modulation depth of the reference.
Reply
1.Although this aspect of the data was not emphasized here, it is true that a subset of neurons in our population with the lowest synchrony-based modulation depth thresholds can account for human AM detection performance [this is described more fully in Nelson (2006, PhD thesis), and in Nelson and Carney 2007]. One of the main points in the current presenta-
tion is that neural AM discrimination thresholds for standard depths below about −15 dB apparently cannot be predicted by changes in either
Psychophysically Driven Studies of Responses to Amplitude Modulation |
141 |
the average rate or strength of synchrony in single IC neurons when the across-repetition variability of these metrics is taken into account (this leads to Verhey’s second suggestion).
2.The recruitment of neurons with different AM depth sensitivities is certainly a reasonable mechanism to explain discrimination thresholds at low depths. We would only add that average rate alone in some neurons is suf-
ficient to predict discrimination psychoacoustics at high standard modulation depths (above −10 dB).
References
Nelson PC, Carney LH 2007 Neural rate and timing cues for detection and discrimination of amplitude-modulated tones in the awake rabbit inferior colliculus. J Neurophysiol 97:522–539
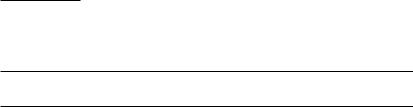
16 Source Segregation Based on Temporal Envelope Structure and Binaural Cues
STEVEN VAN DE PAR1, OTHMAR SCHIMMEL2, ARMIN KOHLRAUSCH1,2,
AND JEROEN BREEBAART1
1Introduction
The lateralization of a single auditory object is mediated by interaural time delays (ITDs) and interaural level differences (ILDs). In daily life, listeners regularly encounter multiple auditory objects simultaneously, and it is of interest to learn to what extent and how listeners can localize each object.
When the spectra of the objects differ sufficiently, the binaural cues within each pair of auditory filters would result from the auditory object that has most energy in the frequency range of that auditory filter. Although in principle this could provide cues for the separation of auditory objects, in experiments using simultaneously presented noises shaped to represent different vowels, subjects could not use ITD cues for segregation (Culling and Summerfield 1995).
In this chapter we want to investigate whether listeners are able to discern the lateralization of two simultaneously presented auditory objects with different temporal structures that are spectrally fully overlapping. Both objects (band-pass noise and a harmonic tone complex) resulted in nearly the same spectral excitation pattern, while due to their different temporal structures, the objects could be well distinguished when listened to in isolation. By presenting these two auditory objects simultaneously and with different binaural properties, binaural cues stemming from both objects are equally reflected within each auditory filter. Therefore, in order to correctly lateralize one of the two objects, listeners would need to somehow separate the binaural cues within each single auditory filter and couple these cues to the auditory objects.
Headphone lateralization experiments were done for different bandwidths and center frequencies in a similar way as in our earlier ISH contribution that was dealing with the discrimination of the same two auditory objects based on binaural cues (van de Par et al. 2005).
1Digital Signal Processing Group, Philips Research Laboratories, Eindhoven, Netherlands, Steven.van.de.Par@philips.com, Armin.Kohlrausch@philips.com, Jeroen.Breebaart@philips.com 2Human-Technology Interaction, Technische Universiteit Eindhoven, Netherlands, O.V.Schimmel @tm.tue.nl
Hearing – From Sensory Processing to Perception
B. Kollmeier, G. Klump, V. Hohmann, U. Langemann, M. Mauermann, S. Uppenkamp, and J. Verhey (Eds.) © Springer-Verlag Berlin Heidelberg 2007