
Учебники / Hearing - From Sensory Processing to Perception Kollmeier 2007
.pdfVirtual Pitch in a Computational Physiological Model |
79 |
Comment by Nelson
This comment concerns the continued use of cochlear nucleus (CN) chopper neurons as fundamental components of the neural circuitry in simulations of responses to AM in the inferior colliculus (IC). Two sets of empirical observations appear inconsistent with the assumptions of these models, such as the one described here (originally suggested by Hewitt and Meddis 1994).
First, real VCN chopper neurons do not typically exhibit maximum synchrony at stimulus AM rates equal to their inherent chopping rate. The correlation between synchrony best modulation frequency (BMF) and chopping frequency is weak (Frisina et al. 1990), but a one-to-one correspondence between these two metrics is an inherent feature of the Hewitt and Meddis model.
The second (more important) piece of physiological data is related to the assumed tight coupling between CN synchrony tuning and IC rate tuning to AM in the model: in Hewitt and Meddis’ implementation, IC rate-BMFs are equal to their input (CN chopper) synchrony-BMFs. This is not borne out in the data either, because the range of CN chopper synchrony-BMFs (~150–700 Hz; Rhode and Greenberg 1994; Frisina et al. 1990) does not match the distribution of IC cell rate-BMFs (~1–150 Hz; Krishna and Semple 2000).
An alternative physiologically based model (Nelson and Carney 2004) can extract periodicity information in the form of band-pass rate-MTFs without the use of an intermediate population of chopper neurons. Instead, temporal interactions between excitation and inhibition underlie rate tuning and enhanced synchronization at the level of the model IC cells.
References
Frisina RD, Smith RL, Chamberlain SC (1990) Encoding of amplitude modulation in the gerbil cochlear nucleus. I. A hierarchy of enhancement. Hear Res 44:99–122
Hewitt MJ, Meddis R (1994) A computer model of amplitude-modulation sensitivity of single units in the inferior colliculus. J Acoust Soc Am 95:2145–2159
Krishna BS, Semple MN (2000) Auditory temporal processing: responses to sinusoidally amplitude-modulated tones in the inferior colliculus. J Neurophysiol 84:255–273
Nelson PC, Carney LH (2004) A phenomenological model of peripheral and central neural responses to amplitude-modulated tones. J Acoust Soc Am 116:2173–2186
Rhode WS, Greenberg S (1994) Encoding of amplitude modulation in the cochlear nucleus of the cat. J Neurophysiol 71:1797–1825
Reply
We are aware of the excellent model of Nelson and Carney and also of the model presented at this conference by Bahmer and Langner. It is important to stress that either of these two models might be substituted for our own in stages two and three of the pitch model in so far as they simulate IC responses
80 |
R. Meddis and L. O’Mard |
to amplitude modulated sounds. Our choice of an old IC model was motivated primarily by simplicity, convenience and familiarity. The main thrust of our paper, of course, does not concern the choice of IC model. Rather it makes the point that large arrays of these units are sufficient to simulate a range of psychophysical pitch results.
When our IC model was developed 15 years ago, the decision to use sustained chopper units in the CN was made in the full knowledge of the physiology referred to in the comment. While the choice of this type of unit was not intuitively obvious, it was successful in the sense that it simulated the data (in which we put our trust). Whether the model is the correct one or not is an empirical issue that remains undecided. It is hoped that the availability of at least three competing models will stimulate further physiological enquiry.
Comment by Greenberg
Would your model be consistent with complex pitch discrimination (by human listeners) on the order of 0.2–0.5% for spectrally non-overlapping harmonics (Wightman 1981)?
References
Wightman FL (1981) Pitch perception: an example of auditory pattern recognition. In: Getty DJ, Howard JH Jr (eds) Auditory and visual pattern recognition. Hillsdale, NJ: Lawrence Erlbaum, pp 3–25
Reply
At present, I am doubtful whether a physiological model can produce that level of precision. However, I am not aware of any reason, in principle, why it should not work given enough units and computing power.
Comment by Carlyon
As you point out, your model is physiologically realizable and shares many properties similar to autocorrelation. A weakness of the most popular autocorrelation model (Meddis and O’Mard 1997) is that it fails to account for the effects of resolvability, independently of the frequencies of the harmonics used to convey pitch information (Carlyon 1998; Bernstein and Oxenham 2005). That is, for a given F0 it predicts poorer discrimination for high-numbered than for low-numbered harmonics, but does not capture the interaction between F0 and frequency region observed in the psy-
Virtual Pitch in a Computational Physiological Model |
81 |
chophysical literature (Shackleton and Carlyon 1994). Your Fig. 3 shows that it can capture the former finding; does it do any better than Meddis and O’Mard (1997) on the latter?
References
Bernstein JGW, Oxenham AJ (2005) An autocorrelation model with place dependence to account for the effect of harmonic number on fundamental frequency discrimination. J Acoust Soc Am 117:3816–3831
Carlyon RP (1998) Comments on “A unitary model of pitch perception” [J Acoust Soc Am 102:1811–1820 (1997)]”. J Acoust Soc Am 104:1118–1121
Meddis R, O’Mard L (1997) A unitary model of pitch perception. J Acoust Soc Am 102:1811–1820
Shackleton TM, Carlyon RP (1994) The role of resolved and unresolved harmonics in pitch perception and frequency modulation discrimination. J Acoust Soc Am 95:3529–3540
Reply
While the Shackleton and Carlyon (1994) study is frequently quoted in this context, it should be treated with caution with respect to establishing the status of autocorrelation as a reliable mathematical predictor of pitch percepts. That studied used pitch matches between simultaneously presented tones allowing for possible complex perceptual interactions between the tones. It would have been more convincing if the pitch matches had been made between non-simultaneous tones. Unfortunately this experiment has not been tried and, as a consequence, it would not be wise to abandon autocorrelation approaches on the basis of this one difficult-to-interpret study.
We accept that the Bernstein and Oxenham (2005) study was a successful challenge to the original autocorrelation architecture where each possible lag was given equal weight in each channel. Fortunately, the authors were able to solve the problem satisfactorily by changing the weights applied to different lags according to the CF of the channel. It is likely that a similar weighting function might work in the physiological model.
We also urge caution with respect to the concept of ‘resolvability’ in this context. It harkens back to a simpler age when it was enough to characterise the auditory periphery as a bank of narrowly tuned linear bandpass filters. Nonlinearity in both the electrical and mechanical responses of the cochlear has caused us to say ‘Goodbye’ to all that. What might be resolved near threshold is not resolved at higher listening levels while pitch remains largely insensitive to level.

10 Searching for a Pitch Centre in Human Auditory Cortex
DEB HALL1 AND CHRISTOPHER PLACK2
1Introduction
Recent data from human fMRI (Barrett and Hall 2006; Hall et al. 2005; Patterson et al. 2002; Penagos et al. 2004) and primate electrophysiological (Bendor and Wang 2005) studies have suggested that a region near the anterolateral border of primary auditory cortex may be involved in pitch processing. Collectively, these findings present strong support for a single central region of pitch selectivity. However, for a brain region to be referred to as a general pitch centre, its response profile should satisfy a number of criteria: i) Pitch selectivity: responses to the pitch-evoking stimulus should be greater than to a control stimulus that does not evoke a pitch percept, but is matched as closely as possible with respect to acoustic features; ii) Pitch constancy: selective responses should occur for all pitch-evoking stimuli, whatever their spectral, temporal or binaural characteristics and irrespective of whether there is spectral energy at the fundamental frequency (F0) (Tramo et al. 2005); iii) Covariation with salience: the response magnitude should covary with pitch salience; and iv) Elimination of peripheral phenomena: it must be possible to discount the contribution of peripheral effects, such as cochlear distortions (McAlpine 2004), to the pitch-evoked response.
In the current study we sought evidence for a pitch centre in humans that complies with these criteria. We combined psychophysical measurements of frequency and fundamental frequency difference limens (FDL) and fMRI measurements of the cortical response to five different pitch-evoking stimuli.
2Methods
2.1Stimuli
Five different pitch-evoking stimuli were generated:
(i)PT: Pure tone consisting of a 200-Hz pure tone and a Gaussian noise bandpass filtered between 500 Hz and 2 kHz
1MRC Institute of Hearing Research, University Park, Nottingham, UK, d.hall@ihr.mrc.ac.uk 2Department of Psychology, Lancaster University, Lancaster, UK, c.plack@lancaster.ac.uk
Hearing – From Sensory Processing to Perception
B. Kollmeier, G. Klump, V. Hohmann, U. Langemann, M. Mauermann, S. Uppenkamp, and J. Verhey (Eds.) © Springer-Verlag Berlin Heidelberg 2007
84 |
D. Hall and C. Plack |
(ii)WB: Wideband complex consisting of the harmonics of a 200-Hz F0 added in cosine phase and lowpass filtered at 2 kHz
(iii)Res: Resolved complex consisting of the harmonics of a 200-Hz F0 added in cosine phase and bandpass filtered between 1 and 2 kHz, together with a Gaussian noise masker lowpass filtered at 1 kHz to reduce the effect of combination tones
(iv)Unres: Unresolved complex consisting of the harmonics of a 100-Hz F0 added in alternating sine and cosine phase and bandpass filtered between 1 and 2 kHz, again with a Gaussian noise masker lowpass filtered at 1 kHz
(v)Huggins: Huggins pitch consisting of a Gaussian noise lowpass filtered at 2 kHz and presented diotically, except for a frequency region from 190
to 210 Hz (200 Hz±10%). This region was given a progressive phase shift, linear in frequency between 0 and 2π, in the left ear only.
Each of these five stimuli has a pitch equivalent to that of a pure tone at 200 Hz. For the fMRI experiment, a control stimulus consisting of a Gaussian noise lowpass filtered at 2 kHz was generated.
With the exception of Huggins, stimuli were presented diotically. For the behavioural experiments the overall level in each ear was fixed at 83 dB SPL, and the “average” spectrum level was held constant at 50 dB (re. 2 × 10−5 N/m2). In other words, the noise, when present, had a spectrum level of 50 dB, the pure tone had a level of 77 dB SPL [50 +10 log10(500)], the harmonics of the 200-Hz complexes had a level of 73 dB SPL, and the harmonics of the 100-Hz complex had a level of 70 dB SPL. For the behavioural experiment, the stimuli had a total duration of 200 ms including 10 ms onset and offset ramps. For the fMRI experiment, the stimuli were 500 ms, including 10 ms onset and offset ramps. These were repeated in a 15.5-s sequence, with 50-ms gaps between each stimulus. The sound levels delivered in the scanner were 87–88 dB SPL, measured at the ear.
2.2Subjects
We recruited 16 normally hearing listeners (≤ 25 dB HL between 250 Hz and 6 kHz) from the university population. Their mean age was 24.5 years, ranging from 18 to 41, and the group comprised seven females and nine males. A majority of listeners were musically trained; with only two listeners unable to read music or play an instrument (#10 and #14). All except one listener (#03) was strongly right handed. The study was approved by the University Medical School Ethics Committee and written informed consent was obtained from all participants.
2.3Pitch Discrimination
Pitch discrimination was measured using a two-down, one-up, adaptive procedure that estimates the 71% correct point on the psychometric function (Levitt 1971). The discrimination task was pitch direction (“in which interval was the
Searching for a Pitch Centre in Human Auditory Cortex |
85 |
pitch higher?”). On each trial there were two observation intervals. The frequency, fundamental frequency, or (in the case of Huggins) the centre frequency of the phase-shifted region, of the standard was fixed to produce a nominal pitch corresponding to 200 Hz. The pitch of the comparison was greater than this. The frequency difference between the standard and comparison intervals was varied using a geometric step size of 2 for the first four reversals, and 1.414 thereafter. In each block, 16 reversals were measured and the threshold taken as the geometric mean of the last 12. Five such estimates were made for each condition, and the final estimate was taken as the geometric mean of the last four. Two of the subjects (#10 and #12) could not hear the Huggins pitch and had thresholds greater than 100%. The thresholds for these subjects were assumed to be 100% for the purpose of subsequent analysis.
2.4fMRI Protocol
Scanning was performed on a Philips 3 T Intera using an 8-channel SENSE receiver head coil. For each listener, we first acquired a 4.5-min anatomical scan (1 mm3 resolution) of the whole head. Functional scans consisted of 20 slices taken in an oblique-axial plane, with a voxel size of 3 mm3. The anatomical scan was used to position the functional scan centrally on Heschl’s gyrus (HG). We took care to also include the superior temporal plane and superior temporal sulcus and to exclude the eyes. Functional scanning used a SENSE factor of 2 to reduce image distortions and a SofTone factor of 2 to reduce the background scanner noise level by 9 dB. Scans were collected at regular 8-s intervals, with the stimulus presented predominantly in the quiet periods between each scan. The functional experiment consisted of one 40-min listening session. In total it included 44 scans for each stimulus type and an additional 46 silent baseline scans, with the order of conditions randomised. Listeners were requested to attend to the sounds and to listen out for the pitch, but were not required to perform any task.
Analysis of the imaging data was conducted using SPM2 (www.fil.ion.ucl. ac.uk/spm) separately for each listener. Pre-processing steps included within-subject realignment and spatial normalization. For each subject, normalized images were up-sampled to a voxel resolution of 2 mm3 and smoothed by 4 mm FWHM. This procedure meets the smoothness assumptions of SPM without compromising much of the original spatial resolution, so preserving the precise mapping between structure and function.
3Results
3.1Pitch Discrimination
The geometric means of the pitch discrimination thresholds across subjects are shown in Fig. 1. Performance was best for WB and worst for Huggins. Interestingly, thresholds for Res and Unres were similar. It is usually reported
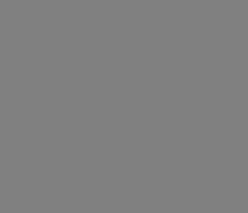
86 |
D. Hall and C. Plack |
Fig. 1 Discrimination thresholds across the group of 16 listeners (geometric mean and standard error)
that thresholds for unresolved harmonics are substantially higher than those for resolved harmonics (Shackleton and Carlyon 1994).
3.2Pitch Activation
Our first analysis confirmed that all listeners produced reliable sound-related activation (P < 0.05, FWE corrected) encompassing the primary auditory cortex on HG, posterior non-primary regions on lateral HG and the planum temporale, and anterior non-primary regions on the planum polare (PP).
Pitch selectivity. To determine regions of pitch selectivity, we contrasted each pitch condition against the control Gaussian noise condition for individual listeners. For exploratory analyses, we used a very lenient metric for false positives (P < 0.01, uncorrected for multiple testing). An overall map of pitch selectivity was generated by summing all pitch-related activations across the group. The white areas in Fig. 2 illustrate the spread of the pitch response that was present in at least 2/80 cases (5 pitch conditions × 16 listeners). The greatest overlap occurred in the left planum temporale at the x, y, z co-ordinate −58, −30, 12 mm, shown by the black dot. Even at this point activation was overlapping in only 11/80 cases, suggesting considerable variability across listeners. Despite the very relaxed statistical thresholding, our pitch-related activation did not extend across the anterolateral area (defined by the traced region in Fig. 2). It lay mostly posterior to HG, in the planum temporale. Although unexpected, this result is not wholly inconsistent with previous literature. Even though the peak activity typically lies in anterolateral HG, our own studies have shown that a pitch-evoking, iterated ripplednoise also engaged an anterior portion of the planum temporale (planned
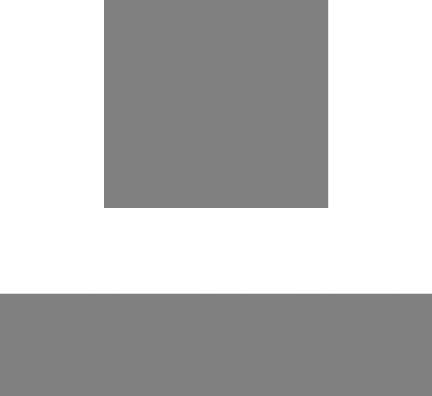
Searching for a Pitch Centre in Human Auditory Cortex |
87 |
Fig. 2 Coronal, sagittal and axial views positioned through the point of the most frequent pitchrelated activity (black dot) and showing the extent of all pitch-related activation (white areas). Activations are overlaid onto the mean anatomical image for the group
Fig. 3 View across the supratemporal plane illustrating the extent of pitch-related activation (white areas) in each of the pitch conditions. Those voxels plotted in white reached significance (P<0.01, uncorrected) for at least two listeners. Black dots indicate the significant peaks of activation occurring within each listener (P<0.01, FWE corrected for the volume of the auditory cortex)
comparison C in Barrett and Hall 2006). Furthermore, Penagos et al. (2004) also showed that resolved complex tones produced differential activation in posterior and lateral sites that were separate from those in anterolateral HG.
Pitch constancy. To explore the question of pitch constancy, we repeated the above procedure but generated separate maps for the five pitch-evoking stimuli (Fig. 3). The white areas represent activity present in at least 2/16 listeners. Although there were differences in the precise pattern, all five pitch conditions produced significant auditory activation. Planum temporale was most widely activated by the WB condition. Remarkably, the Huggins pitch also evoked a significant response, even though this pitch is generated using very different acoustic cues from the other types of pitch and has the greatest FDL.
The group maps in Figs. 2 and 3 conceal the fact that pitch-related activation rarely occurred at exactly the same point in the auditory cortex across
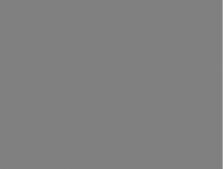
88 |
D. Hall and C. Plack |
Fig. 4 The pitch centre in three listeners; position is shown using x, y, z co-ordinates and each listener’s anatomical image
the different listeners. The spatial consistency was much more striking within listeners however.
Our data would remain compatible with the notion of a pitch centre if pitch constancy were to be confirmed (i.e. if a significant response to all five pitch-evoking stimuli occurred in any one listener). Given that separate contrasts are thresholded at P<0.01, the probability of this occurring by chance is very small (P<10−10). Most of our listeners (N=10) did indeed produce conjoint activation for at least four of the pitch contrasts (P<5 × 10−8). However, two observations differ from those predicted by previous literature. First, the location of the pitch centre varied a great deal from listener to listener (Fig. 4). In seven listeners (e.g. #14 and 15) it fell in different portions of the planum temporale, in one listener (#13) it fell in PP and in two listeners it was elsewhere. Second, the magnitude of the response within the pitch centre was unrelated to the perceptual salience of the pitch that had been measured psychophysically.
4Conclusion
To our knowledge, this is the first study that has sought to identify a pitch centre whose response satisfies the criterion of pitch constancy across a range of different pitch-evoking stimuli. In most listeners, we found small regions within posterior non-primary auditory cortex that responded selectively to the pitch-evoking stimuli, even to the Huggins pitch stimulus which evokes the weakest percept. This is the first time that a cortical response to a binaural pitch has been reported in humans. The two surprising caveats to our findings were that i) this apparent site for pitch processing occurred in different places in different listeners and, ii) the
Searching for a Pitch Centre in Human Auditory Cortex |
89 |
response did not vary consistently as a function of the pitch salience. Neither of these observations can be easily reconciled with current models of pitch coding and its neural representation within the auditory cortex. We were unable to find evidence for either pitch selectivity or pitch constancy in the anterolateral area of the human auditory cortex. Nevertheless, our data highlight the importance of other non-primary regions in pitch coding, a finding that has been reported, but perhaps not emphasized, by other researchers (e.g. Penagos et al. 2004). Our stringent criteria for inclusion could account for our failure to replicate previous findings. Not only was our pitch-related response required to generalize across the different pitchevoking stimuli – it also had to be significantly greater than for a control noise matched for spectral energy, and it had to occur reliably for pitchevoking stimuli that contained a noise masker around the missing F0 (Res and Unres conditions). Not all of these conditions have been met before. The present data perhaps raise more questions than they answer about the neural substrate of pitch processing. Apart from the questions that we have already raised it is important to gain a better understanding about how these pitch computations are affected by sound level, especially for those signals presented at high levels in the MR scanner. We hope this brief report will stimulate further neuroimaging and electrophysiological investigations to address these issues.
Acknowledgements. This work was supported by the Medical Research Council of the UK and a Knowledge Transfer Grant from the RNID.
References
Barrett DJK, Hall DA (2006) Response preferences for ‘what’ and ‘where’ in human nonprimary auditory cortex. NeuroIm (in press)
Bendor D, Wang X (2005) The neuronal representation of pitch in primate auditory cortex. Nature 436:1161–1165
Hall DA, Barrett DJK, Akeroyd MA, Summerfield AQ (2005) Cortical representations of temporal structure in sound. J Neurophys 94:3181–3191
Levitt H (1971) Transformed up-down methods in psychoacoustics. J Acoust Soc Am 49:467–477
McAlpine D (2004) Neural sensitivity to periodicity in the inferior colliculus: evidence for the role of cochlear distortions. J Neurophys 92:1295–1311
Patterson RD, Uppenkamp S, Johnsrude IS, Griffiths TD (2002) The processing of temporal pitch and melody information in auditory cortex. Neuron 36:767–776
Penagos H, Melcher JR, Oxenham AJ (2004) A neural representation of pitch salience in nonprimary human auditory cortex revealed with functional magnetic resonance imaging. J Neurosci 24:6810–6815
Shackleton TM, Carlyon RP (1994) The role of resolved and unresolved harmonics in pitch perception and frequency modulation detection. J Acoust Soc Am 95:3529–3540
Tramo MJ, Cariani PA, Koh CK, Makris N, Braida LD (2005) Neurophysiology and neuroanatomy of pitch perception: Auditory cortex. Ann NY Acad Sci 1060:148–174