
Учебники / Hearing - From Sensory Processing to Perception Kollmeier 2007
.pdf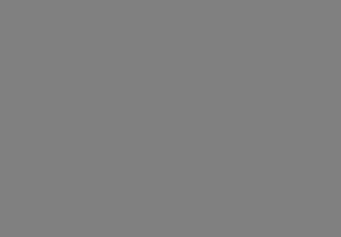
240 |
M.F.B. van Beurden and W.A. Dreschler |
Fig. 1 An example of an outcome of the loudness difference procedure. At each turning point the level differences between the variable and the variable +2 dB increase re. the reference are shown. The estimated equal loudness level is constructed from the level differences at the upper turning points of the variable and at the lower turning points of the variable +2 dB increase. The dotted lines represent the assumed loudness uncertainty region
The procedure consists out of two phases. In the first phase the limits of the auditory range are estimated by an interleaved ascending and descending stimulus sequence. In the second phase the four named intermediate categorical loudness levels are estimated. This last phase consists out of two blocks. In the first block the four named intermediate categorical loudness levels are estimated by linear interpolation between the two limits of the auditory range, which are the values at L5 (very soft) and L50 (too loud). In the second block the named intermediate categorical loudness levels are estimated by a modified least-squares fit of a linear model function. In this study three iterations of the final block were applied. In the analysis each ACALOS measurement was fitted with a model function consisting of two linear parts with independent slopes and a free cut-point. This model function is a slightly different function than the model function applied by Brand and Hohmann (2002), because their model function had a fixed cut-point at 25 CU.
2.3Subjects
In the loudness matching experiments nine normal-hearing subjects (four male, five female) participated. The age of the subjects ranged from 18 to 34 years. Two of the subjects were members of the Audiology department; the
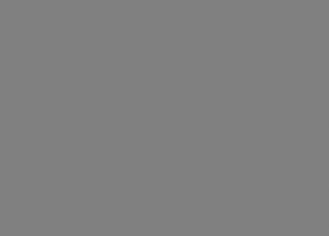
Duration Dependency of Spectral Loudness Summation |
241 |
other subjects were paid volunteers without previous experience with loudness matching experiments. In the loudness scaling experiment 12 other normalhearing subjects (5 male, 7 female) aged from 18 to 36 participated. The subjects were paid volunteers without previous experience with loudness scaling experiments. All subjects had auditory thresholds <15 dB HL and no previous history of any hearing problems.
3Results
Figure 2 shows the results of matching procedure 1 and matching procedure 2 at a center frequency of 2000 Hz and with a reference bandwidth of 800 Hz. The figures show the differences between the level of the test signal and the reference signal (∆L) at equal loudness as a function of the bandwidth of the test signal. A negative level difference means that the test signal needs a lower level to be judged as equally loud as the reference signal. Signal durations were 25 ms (circles) and 1000 ms (squares). The error bars indicate plus and minus one standard error of the mean.
The results of the loudness matching procedure are presented in Fig. 3. Each ACALOS measurement was fitted with a model function consisting of two linear parts with independent slopes and a free cut-point. Therefore, each fit was characterized by four parameters. The fits shown are based on the average of the parameters across subjects, where the variables per subject are based on all points obtained in the three tests.
Fig. 2 Results of the two procedures at 25 ms (circles) and 1000 ms (squares)
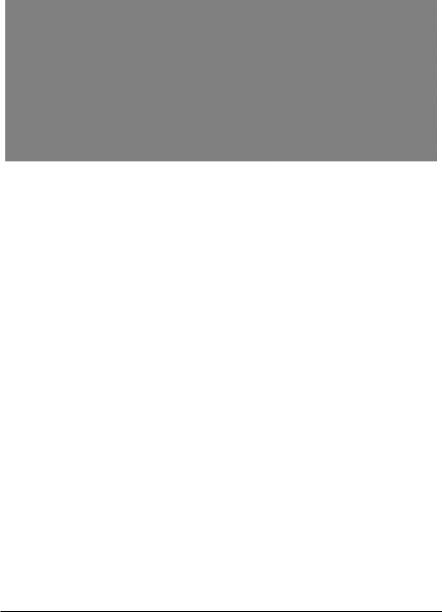
242 |
M.F.B. van Beurden and W.A. Dreschler |
Fig. 3 Results loudness scaling for 25 ms and 400 ms signals of different bandwidths
The figure shows that:
1.The slopes of the higher-intensity part are usually steeper than for the lowintensity part. This effect is found for all signal bandwidths and both signal durations.
2.The low-intensity slope is less steep for the 25-ms signals.
3.Furthermore, the loudness curves are ordered according to bandwidth, with a larger bandwidth leading to a higher loudness at the same level. This is what is expected from spectral loudness summation.
4.Finally, a comparison of both figures shows that corresponding levels yield a higher loudness level for the 400-ms signals than for the 25-ms signals, as would be expected from temporal integration.
In order to obtain a similar parameter of loudness summation from the loudness scaling data, we calculated for each of the stimuli the level differences relative to the level of the reference signal (60 dB), needed to obtain equal loudness as for the 400 Hz wide reference signal. Table 1 shows the calculated summation data.
At 25 ms the loudness of this signal is 11.6 CU and at 400 ms the loudness is 14.5 CU. So, there is a slight loudness differences between the reference signals
Table 1 Spectral loudness summation difference between short and long duration signals in dB SPL
|
Summation difference |
Summation difference |
Summation difference |
|
Matching 1 |
Matching 2 |
Scaling |
|
|
|
|
1600 Hz |
0.64 |
−0.14 |
−1.58 |
3200 Hz |
2.47 |
1.23 |
2.75 |
6400 Hz |
2.31 |
2.44 |
1.14 |
|
|
|
|
Duration Dependency of Spectral Loudness Summation |
243 |
at different durations. For the loudness scaling data the loudness ratings of a 800 Hz wide stimulus was used as a reference. This makes the data of the loudness matching not directly comparable to the data of the loudness scaling.
4Discussion
Although the matching procedures and the scaling procedure have been conducted with slightly different stimuli the same trends can be observed. First of all, in all three procedures spectral loudness summation is larger for short signals than for long signals. This corresponds well with the findings of Verhey and Kollmeier (2002) and Chalupper (2002). The fact that duration dependent spectral loudness summation has been found in three different measuring procedures provides extra support for the existence of this effect and excludes possible artifacts due to the measurement procedure.
The amount of spectral loudness summation difference depends on the amount of summation, which is in agreement with the results of Brand and Hohmann (2002). The duration-dependency of spectral loudness summation is small, when the loudness summation is small. As loudness summation increases, the loudness summation difference also increases. However, the maximum amount of loudness summation difference between short and long signals seems limited. In all three procedures the amount of loudness summation at a bandwidth of 3200 Hz and 6400 Hz is approximately the same. A further investigation with even broader bandwidths is needed to confirm the observation that a ceiling effect may be present. It would be interesting to determine the “critical” bandwidth at which the summation difference between long and short signals reaches the maximum value.
There are also differences between the procedures, especially with respect to the amount of summation found. This is probably a consequence of procedural differences. In the second matching procedure we assumed that interleaving of the different conditions was not necessary. Verhey (1999) found that an adaptive procedure with interleaved tracks leads to larger loudness summation. The differences we found between matching procedure 1 and matching procedure 2 correspond to the differences found between an interleaved and a non-interleaved procedure.
The long duration condition of the scaling procedure is conducted with a 400-ms signal instead of a 1000-ms signal. The influence of this difference in signal duration may be expected to be negligible, as the effect of temporal loudness integration is thought to be limited to approximately 200 ms. The scaling procedure is much less sensitive than the two matching procedures at one specific loudness. The results depend heavily on the definition of the fitting curve. Nevertheless, the results correspond reasonably well with the matching results and give also a hint towards the level dependency of the effect. At low levels there is almost no spectral loudness summation and
244 |
M.F.B. van Beurden and W.A. Dreschler |
therefore the summation difference is also very small. Around the cut-point of the fitting curve, which seems to lie at the lower side of the most comfortable loudness region, both the spectral summation and the summation difference are largest. At higher levels they tend to decrease again.
5Summary and Conclusion
This study shows with three different measuring procedures the effect that spectral loudness summation is larger at short signal durations. Although the amount of summation differs in the different procedures the summation difference is approximately the same. Our data show a possible ceiling effect in the amount of spectral loudness summation differences between short and long signals. Further research is needed in order to investigate the effect of bandwidth on the loudness summation difference between short and long signals.
An adapted version of the model of loudness applicable to time-varying sounds (Glasberg and Moore 2002) that increases the loudness of short signals at low levels has been proven to model these effects reasonably well.
References
Brand T, Hohmann V (2002) An adaptive procedure for categorical loudness scaling. J Acoust Soc Am 112:1597–1604
Chalupper J (2002) Perzeptive Folgen von Innenschwerhörigkeit: Modellierung, Simulation und Rehabilitation. Shaker, Aachen
Glasberg BR, Moore B C J (2002) A model of loudness applicable to time-varying sounds. J Audio Eng Soc 50:331–342
Ozimek E, Zwislocki JJ (1996) Relationships of intensity discrimination to sensation and loudness levels: dependence on sound frequency. J Acoust Soc Am 100:3304–3320
Kohlrausch A, Fassel R, van der Heijden M, Kortekaas R, van de Par S, Oxenham AJ, Puschel D (1997) Detection of tones in low-noise noise: further evidence for the role of envelope fluctuations. Acust Acta Acust 83:659–669
van Beurden MFB, Dreschler WA (2005) Bandwidth dependency of loudness in series of short noise bursts. AcustActa Acust 91:1020–1024
Verhey JL (1999) Psychoacoustics of spectro-temporal effects in masking and loudness perception. PhD thesis
Verhey JL, Kollmeier B (2002) Spectral loudness summation as a function of duration. J Acoust Soc Am 111:1349–1358
Comment by Verhey
In your talk you presented a model that predicts the duration-dependent spectral loudness summation as reported in, e.g., Verhey and Kollmeier (2002, JASA 111, 1349-1358). The model was based on the assumption of a
Duration Dependency of Spectral Loudness Summation |
245 |
larger gain applied to short signals than to long signals at low levels. Such a mechanism results in a higher compression at the medium to high levels. How does this assumption relate to Epstein and Florentine (2005a, b), and Anweiler and Verhey (2006) who showed that the loudness function of the short signals is essentially a vertically (downward) shifted version of the loudness function of the long signals? Such a vertical shift produces the same slope for different duration at the same intensity, i.e. no change in compression with duration.
References
Epstein M, Florentine M (2005a) Inferring basilar-membrane motion from tone-burst otoacoustic emissions and psychoacoustic measurements. J Acoust Soc Am 117:263–274
Epstein M, Florentine M (2005b) A test of the equal-loudness-ratio hypothesis using crossmodality matching functions. J Acoust Soc Am 118:907–913
Anweiler AK, Verhey JL (2006) Spectral loudness summation for short and long signals as a function of level. J Acoust Soc Am 119:2919–2928
Reply
First of all, an equal level difference at equal loudness for short and long signals over all levels is indeed in disagreement with my hypothesis. A small decrease in level difference at equal loudness is expected at low levels. In fact the data of Epstein and Florentine (2005b) is somewhat ambiguous and a small decrease in level difference at equal loudness can be seen. If the lowest point is neglected a decrease in loudness ratio at low levels is found. The ambiguity can also be seen in the individual data in which some subjects seem to show a clear level difference decrease at low levels (L5,L6,L9) In that case no contradiction between the adapted model and their data is present. The magnitude estimation data of Epstein and Florentine (2006a) show a clear increase in level difference at equal loudness at low levels, which is clearly in contrast with my hypothesis. But here the group data appear to be heavily influenced by subject L3 and the individual data again show also subjects with a decrease in level difference at low levels (L5, L6). The scaling data from Anweiler and Verhey (2006) and the present paper is not accurate enough at low levels to do well funded statements. Therefore I think it is not possible to say if these data agree, or disagree with my hypothesis.
Unfortunately Epstein and Florentine (2005a, b) have not presented data for broadband signals. For such signals the model predicts a larger decrease in level difference at low levels than for narrowband signals, which should be easier to measure.

27 The Correlative Brain: A Stream Segregation Model
MOUNYA ELHILALI AND SHIHAB SHAMMA
1Introduction
The question of how everyday cluttered acoustic environments are parsed by the auditory system into separate streams is one of the most fundamental in perceptual science. Despite its importance, the study of its underlying neural mechanisms remains in its infancy; with a lack of general frameworks to account for both psychoacoustic and physiological experimental findings. Consequently, the few attempts at developing computational models of auditory stream segregation remain highly speculative. This in turn has considerably hindered the development of such capabilities in engineering systems such as automatic speech recognition, or sophisticated interfaces for communication aids (hearing aids, cochlear implants, speech-based human-computer interfaces).
In the current work, we present a mathematical model of auditory stream segregation, which accounts for both perceptual and neuronal findings of scene analysis. By closely coordinating with ongoing perceptual and physiological experiments, the proposed computational approach provides a rigorous framework for facilitating the integration of these results in a mathematical scheme of stream segregation, for developing effective algorithmic implementations to tackle the “cocktail party problem” in engineering applications, as well as generating new hypotheses to better understand the neural basis of active listening.
2Framework and Foundation
2.1Premise of the Model
Numerous studies have attempted to reveal the perceptual cues necessary and/or sufficient for sound segregation. Researchers have identified frequency separation, harmonicity, onset/offset synchrony, amplitude and
Institute for Systems Research & Department of Electrical and Computer Engineering, University of Maryland, College Park MD, USA, mounya@isr.umd.edu, sas@isr.umd.edu
Hearing – From Sensory Processing to Perception
B. Kollmeier, G. Klump, V. Hohmann, U. Langemann, M. Mauermann, S. Uppenkamp, and J. Verhey (Eds.) © Springer-Verlag Berlin Heidelberg 2007
248 |
M. Elhilali and S. Shamma |
frequency modulations, sound timbre and spatial location as the most prominent candidates for grouping cues in auditory streaming (Cooke and Ellis 2001). It is, however, becoming more evident that any sufficiently salient perceptual difference along any auditory dimension (at the periphery or central auditory stages) may lead to stream segregation.
On the biophysical level, our knowledge of neural properties particularly in the auditory cortex indicates that cortical responses (Spectro-Temporal Receptive Fields, STRFs) exhibit elaborate selectivity to spectral shapes, symmetry and dynamics of sound (Kowalski et al. 1996; Miller et al. 2002). This intricate mapping of acoustic waveforms into a multidimensional space suggests a role of the cortical circuitry in representing sounds in terms of auditory objects (Nelken 2004). Moreover, this organizational role is supported by the correspondence between time scales of cortical processing and the temporal dynamics of stream formation and auditory grouping.
In this study, we formalize these principles in a computational scheme that emphasizes two critical stages of stream segregation: (1) mapping sounds into a multi-dimensional feature space; (2) organizing sound features into temporally coherent streams. The first stage captures the mapping of acoustic patterns onto multiple auditory dimensions (tonotopic frequency, spectral timbre and bandwidth, harmonicity and common onsets). In this mapping, acoustic elements that evoke sufficiently non-overlapping activity patterns in the multi-dimensional representation space are deemed perceptually distinguishable and hence may potentially form distinct streams. We assume that these features are rapidly extracted and hence this mapping simulates “instantaneous” organization of sound elements (over short time windows; e.g. <200 ms), thus evoking the notion of simultaneous auditory grouping processes (Bregman 1990).
The second stage simulates the sequential nature of stream segregation. It highlights the principle that sound elements belonging to the same stream tend to evolve together in time. Conversely, temporally uncorrelated features are an indication of multiple streams or a disorganized acoustic scene. Identifying temporal coherence among multiple sequences of features requires integration of information over relatively long time periods (e.g. >300 ms), consistent with known dynamics of streaming-buildup. Therefore, the current model postulates that grouping features according to their levels of temporal coherence is a viable organizing principle underlying cortical mechanisms in sound segregation.
2.2Stage 1: Multi-dimensional Cortical Representation
Current understanding of auditory cortical processing inspires our model for the multi-dimensional representation of sound. The model takes in as input an auditory spectrogram, and effectively performs a wavelet decomposition using a bank of linear spectro-temporal receptive fields (STRFs). The
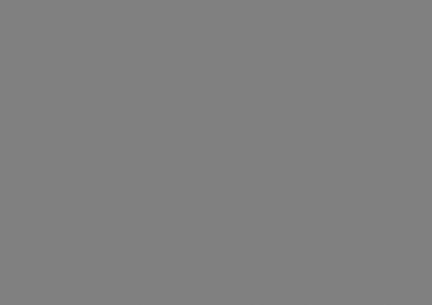
The Correlative Brain: A Stream Segregation Model |
249 |
analysis proceeds in two steps (as detailed in Chi et al. 2005): (i) a spectral step that maps each incoming spectral slice into a 2D frequency-scale representation. It is implemented by convolving the time-frequency spectrogram y(t,x) with a complex-valued spectral receptive field SRF, parametrized by spectral tuning Ωc and characteristic phase φc; (ii) a temporal step in which the time-sequence from each frequency-scale combination (channel) is convolved with a temporal receptive field TRF to produce the final 4D cortical mapping r. Each temporal filter is characterized by its modulation rate ωc and phase θc. This cortical mapping is depicted in Fig. 1A, and can be captured by
s (t, x; Ωc, fc) = y (t, x)*x SRF (x ; Ωc, fc) |
(1) |
r(t, x; wc, Ωc ,qc, fc) = s (t, x; Ωc, fc)*t TRF(t; wc,qc) |
|
We choose the model’s parameters to be consistent with cortical response properties, spanning the range Γ=[0.5–4] peaks/octave spectrally and Ψ = [1–30] Hz temporally. Clearly, other feature dimensions (such as spatial location and pitch) can supplement this multidimensional representation as needed.
Fig. 1 A,B Schematic of stream segregation model
250 |
M. Elhilali and S. Shamma |
2.3Stage 2: Temporal Coherence Analysis
The essential function of this stage is twofold: (i) estimate a pair-wise correlation matrix (C) among all scale-frequency channels, and then (ii) determine from it the optimal factorization of the spectrogram into two streams (foreground and background) such that responses within each stream are maximally coherent.
The correlation is derived from an instantaneous coincidence match between all pairs of frequency-scale channels integrated over time. Given that TRF filters provide an analysis over multiple time windows, this step is equivalent to an instantaneous pair-wise correlation across channels summed over rate filters (Fig. 1B):
Correlation Matrix = #si (t) s j (t) dt - / ri (~) r*j (~) _ Cij |
(2) |
~ ! } |
|
where (*) denotes the complex-conjugate. We can find the “optimal” factorization of this matrix into two uncorrelated streams, by determining the direction of maximal incoherence between the incoming stimulus patterns. Such a factorization is accomplished by a principal component analysis of the correlation matrix C (Golub and Van Loan 1996), where the principal eigenvector corresponds to a map labeling channels as positively or negatively correlated entries. The value of its corresponding eigenvalue reflects the degree to which the matrix C is decomposable into two uncorrelated sets, and hence reflects how ‘streamable’ the input is.
2.4Computing the Two Streams
Therefore, the computational algorithm for factorizing the matrix C is as follows:
1.At each time step, the matrix C(t) is computed from the cortical representation as in Eq. (2). The correlation matrix keeps evolving as the cortical output r(t) changes over time. However for stationary stimuli, the correlation pattern reaches a stable point after a buildup period.
2.Given its hermitian nature (since it is a correlation matrix), C can be expressed as C = lmm† + e, where m is the principal eigenvector of C, l its corresponding eigenvalue, and e(t) the residual energy in C not accounted for by the outer-product of m. (†) denotes the hermitian transpose. The ratio of l2 to the total energy in C corresponds to the proportion of the correlation matrix accounted for by its best factorization m. This ratio is an indicator of the separablity of the matrix C, and hence the streamability of the sound.
The principal eigenvector m can be viewed as a ‘mask’, which can differentially shape the scale-frequency input pattern at any given time instant. This mask