
Учебники / Hearing - From Sensory Processing to Perception Kollmeier 2007
.pdf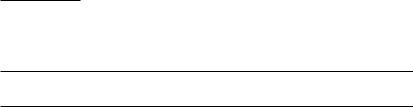
18 Forward Masking: Temporal Integration or Adaptation?
STEPHAN D. EWERT1,2, OLE HAU1, AND TORSTEN DAU1
1Introduction
When a short signal tone is presented after a noise or tone masker, the threshold for detecting the signal is raised the smaller the gap duration between the masker and the signal is. This phenomenon is termed forward masking and refers to the fact that a masker affects the signal threshold when both are presented in a non-simultaneous, consecutive manner. With increasing temporal separation, signal threshold usually drops to performance in silence when the gap is in the region of hundreds of milliseconds. As a possible explanation for forward masking, mainly two different mechanisms have been discussed in the literature: (i) continuation or persistence of neural activity (e.g., Plomp 1964; Oxenham and Moore 1994), referring to temporal integration of neural activity at presumably higher stages than the auditory nerve; (ii) neural adaptation (e.g., Duifhuis 1973; Nelson and Swain 1996), assuming adaptation at various levels of the auditory pathway (including high levels). A third possible source for interaction of masker and signal is linked to the ringing of the auditory filters but is generally assumed to be negligible for signal frequencies of 1 kHz or higher (e.g., Vogten 1978). It is still unclear whether temporal integration or adaptation can better account for forward masking in various stimulus configurations (Oxenham 2001), nor have both mechanisms been compared directly in a common modeling framework to investigate their relation.
The current study compares two well established models of temporal processing in the auditory system using a unified modeling framework: (i) the tem- poral-window model (e.g., Oxenham and Moore 1994) representing a temporal-integration mechanism and (ii) the adaptation-loop model (e.g., Dau et al. 1996) as the representative for the adaptation mechanism. The unified modeling framework shares a compressive, non-linear auditory filter stage and a template-based (optimal detector) decision stage. The question is, whether the temporal-window model and the adaptation-loop model can be considered
1Centre for Applied Hearing Research, Ørsted DTU, Technical University of Denmark, Denmark, se@oersted.dtu.dk, tda@oersted.dtu.dk
2Medizinische Physik, Fakultät V, Institut für Physik, Carl von Ossietzky Universität, Oldenburg, Germany, stephan.ewert@uni-oldenburg.de
Hearing – From Sensory Processing to Perception
B. Kollmeier, G. Klump, V. Hohmann, U. Langemann, M. Mauermann, S. Uppenkamp, and J. Verhey (Eds.) © Springer-Verlag Berlin Heidelberg 2007
166 |
S.D. Ewert et al. |
in a unified modeling framework while maintaining their predictive power. Specifically, it is investigated if the two models can help distinguishing between persistence and adaptation, the two hypothetical mechanisms underlying forward masking.
2Methods
2.1Procedure and Subjects
A three-interval, three-alternative forced-choice adaptive procedure (twodown, one-up rule) was used to determine detection thresholds in the simulations and experiments. The step size was 8 dB at the beginning and was halved after every two reversals, until it reached a minimum of 1 dB where eight reversals were obtained for threshold estimation. The starting level of the signal was 90 dB SPL. Two subjects participated as a control group. The stimuli were presented to one ear via headphones (AKG K-501) in a doublewalled, sound-attenuating booth.
2.2Stimuli
Two forward masking experiments with signal tones at 1 and 4 kHz were conducted to test the models. At 1 kHz, a 10-ms, Hanning-windowed signal was used. The masker was a 200-ms, 77-dB, 20to 5000-Hz frozen noise, no ramps were applied. The experimental design was the same as in Dau et al. (1996). At 4 kHz, a 12-ms signal, including 2-ms, raised-cosine ramps, was added to a 200-ms, 78-dB, 0- to 7000-Hz, frozen-noise masker. The masker included 2-ms, raised-cosine ramps. The design was the same as in Oxenham (2001). The offset-offset time of the signal and the masker was varied in the range from −10 to 150 ms, thus including conditions of simultaneous as well as nonsimultaneous masking.
3Models and Predictions
The processing modules of the temporal-window (TW) model according to Oxenham (2001) were implemented. The TW model uses a linear, timeinvariant integration after non-linear peripheral processing. The shape of the integration window relevant for forward masking results from two exponential functions with time constants of 4.6 ms and 16.6 ms, added with a weight of 0.17 for the longer time constant. In the nonlinear part, the model uses an instantaneous power-law compression with an exponent of 0.25 for
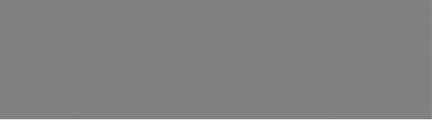
Forward Masking: Temporal Integration or Adaptation? |
167 |
levels higher than about 35 dB SPL after peripheral band-pass filtering. At the output of the temporal window, representations of the signal and masker are derived and detection is based on the best (signal+masker)- to-masker ratio at one instant in time.
The modules of the adaptation-loop (AD) model were implemented according to Dau et al. (1996). In the model, a series of five non-linear feedback loops mimics adaptation in the auditory system. The time constants of the adaptation loops are 5, 50, 129, 253, and 500 ms. In contrast to the TW model, the AD model (Dau et al. 1996) does not employ instantaneous compression of the output of the peripheral filters.
For the detector, the model calculates a template representation consisting of the normalized difference between the masker-plus-supra-threshold-signal representation and the masker-alone representation after adaptation. Detection is based on cross-correlation of the template and the output representation given by the difference between masker-plus-current-signal and masker alone.
For details of the TW and AD model the reader is referred to the respective publications.
3.1Unified Model Framework
Figures 1 and 2 show the modified TW and AD models as part of a common framework that shares the preprocessing and the decision stage. Peripheral filtering was simulated using the dual resonance non-linear (DRNL) model (Meddis et al. 2001). The DRNL model was adjusted to show a compression ratio of 0.25 for input levels in the region of about 40 to 70 dB SPL, comparable to the nonlinearity used in the original TW model. Stimuli were then subjected to half-wave rectification, lowpass filtering and squaring. The TW model structure has been modified to fit the optimal detector of the original AD model. Both model implementations in the unified framework have been
Fig. 1 Modified temporal-window (TW) model. The DRNL filter and the optimal detector were changed with respect to the original implementation. The optimal detector derives a template from the upper processing path. During the run of the experiment, the reference intervals, M/M, and the actual signal interval, (M+S)/M, are processed and correlated with the template
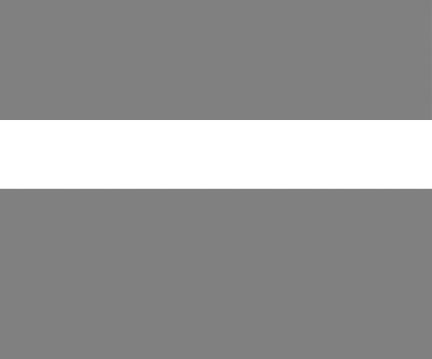
168 |
S.D. Ewert et al. |
Fig. 2 Modified adaptation-loop (AD) model. The DRNL filter and the squaring module were changed with respect to the original implementation
Fig. 3 Predictions of the original models (squares) and the unified TW and AD models (triangles) in the 1- and 4-kHz forward masking experiment. Left: TW model (black). Right: AD model (gray). The stars and crosses indicate empirical control data from two subjects
verified to match the predictions of the original models in the 1- and 4-kHz experiments described above. Results are shown in Fig. 3. The time constants of the unified TW model were fitted to the 4-kHz condition while the adaptation loops of the AD model were kept unchanged. For both models, a better agreement between the control data and the model predictions was observed at 4 kHz. Both models showed a too steep decay of forward masking in the 10to 30-ms offset-offset time region.
Overall, the TW model showed a slightly better agreement with the data than the AD model. Average deviations between the original and unified models were in the region of a few decibels.
4Model Analysis
In order to investigate how the modules of the two models account for forward masking, two simplified block diagrams of the TW and the AD model are shown in Figs. 4 and 5. In the TW model (Fig. 4), the internal representation of the
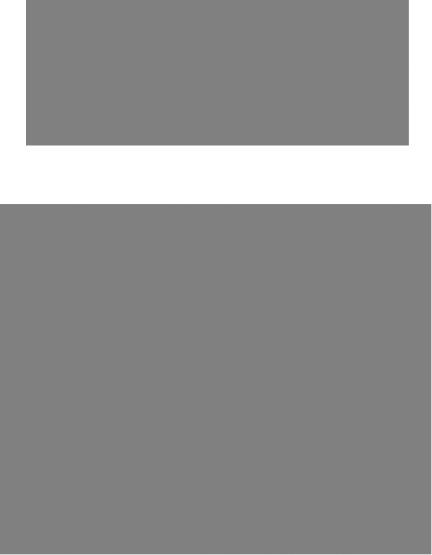
Forward Masking: Temporal Integration or Adaptation? |
169 |
Fig. 4 Simplified block diagram highlighting the division stage of the temporal-window model
Fig. 5 Simplified block diagram of the AD model (upper panel). For each input stimulus condition, the adaptation loops can be replaced by a division of the input waveform with an equivalent divisor as shown in the lower panel
masker+signal is divided by the representation of the masker alone which is referred to as “divisor” in the following. The TW model divisor is shown in Fig. 6 (black line). The TW model is able to account for forward masking since the divisor only declines gradually after masker offset at 0.2 s, reflecting persisting masker energy or the effect of temporal integration. However, in addition to the
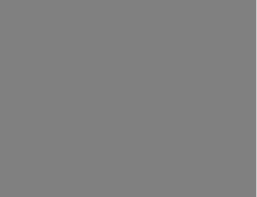
170 |
S.D. Ewert et al. |
Fig. 6 Comparison of the divisors in the temporal-window model (black) and adaptation-loop model (dashed gray)
persistence of masker energy, the fact that the TW model incorporates a division of both stimulus paths is crucial for the function of the model. The division module in the current model implementation equals the ratio detection criterion, (M+S)/M, in the original TW model.
A comparable analysis of the AD model (Fig. 5) reveals that the stages of the adaptation loops can be viewed as a similar division process (upper and middle panel). The difference is that the AD model incorporates feedback while the TW model is a pure feed-forward circuit. For each input stimulus condition, however, an equivalent adaptation devisor for a feed-forward mechanism can be derived (Fig. 5, lower panel). The equivalent AD model devisor is indicated by the dashed gray line in Fig. 6. In comparison to the TW divisor, it shows a bump at the temporal position of the signal, in this case at about 250 ms. This bump reflects a “self-suppression” effect of the signal.
4.1Simplified Adaptation-loop Model
The above analysis has shown that the prominent difference between the two models could be reduced to the effect of the signal on the divisor function which reflects a “self suppression” of the signal. The AD model was further simplified (see Fig. 7) to derive the equivalent AD devisor function from the masker only, similar to the processing in the TW model. The hypothesis was that if the parameters of the temporal window in the TW model were adjusted to produce a divisor function matching the divisor of the simplified AD model, both models should predict the same forward masking curve. Figure 8 shows the matched divisors (left) and the predictions obtained with the two models (right). Both models predicted essentially the same data when their divisors were matched.
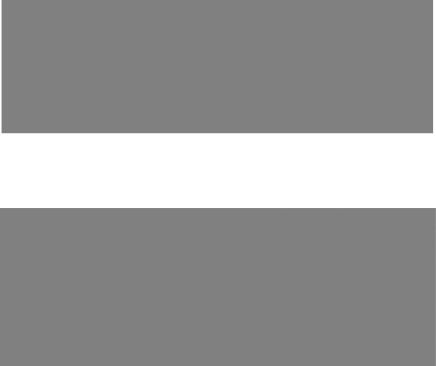
Forward Masking: Temporal Integration or Adaptation? |
171 |
Fig. 7 Simplified adaptation-loop model where only the adaptation effect originating from the masker is considered
Fig. 8 Left: Divisor of the TW model (black) with time constants adjusted to match the devisor of the simplified AD model (dashed gray). Right: Predictions of the TW model and simplified AD model at 4 kHz with the divisors shown in the left panel
5Discussion
The key mechanism responsible for the simulation of forward masking in both models is the division of the internal representation of the signal by the representation of the persisting or temporally smoothed masker. In the temporalwindow model, this division is realized in the detection process, while it is a part of the feedback loops in the adaptation-loop model. With regard to forward masking, the temporal-window model can be viewed as a simplified adaptation model neglecting “self suppression” of the signal. In fact, both models use the identical key mechanism to describe forward masking. Thus, these model realizations can not be used to critically separate between adaptation and persistence. The concept of persistence as realized in the TW model, cannot lead to successful predictions without the ratio-based decision criterion.
The temporal-window model has proven its strength as a very flexible and well “controllable” tool to investigate, e.g., effects of peripheral compressive non-linearity on forward masking in the normal versus the impaired auditory
172 |
S.D. Ewert et al. |
system (e.g., Plack and Oxenham 1998). The adaptation-loop model has proven its strength in a variety of experimental conditions in addition to forward masking, such as spectro-temporal masking and modulation detection. The model has also been used as front end in automatic speech recognition and objective speech quality assessment (whereby the detection stage was replaced by other post-processing devices). A possible modification of the adaptation stage might use a single low-pass filter in the feedback loops where the parameters of the impulse response could be adjusted in a similar way as the time constants in the temporal window model. The lack of goodness of fit in the 1-kHz case could be solved either by a variation of the parameters in the TW and AD models or by using alternative peripheral filter functions (with different ringing) at low frequencies.
6Conclusions
It was found that the TW and AD models can be considered as being essentially equivalent in predicting forward masking: the combination of integration and the signal-to-noise-ratio based detection criterion in the TW model act effectively as a simplified adaptation mechanism. However, since there is physiological evidence for adaptation along the auditory pathway, the AD model appears to be the more general approach. It shows the effect of adaptation in the internal representation of the stimuli and can be applied successfully to a broader class of masking conditions than the TW model.
Acknowledgments. This work was supported by the Danish Research Council.
References
Dau T, Püschel D, Kohlrausch A (1996) A quantitative model of the “effective” signal processing in the auditory system. I. Model structure. J Acoust Soc Am 99:3615–3622
Duifhuis H (1973) Consequences of peripheral frequency selectivity for nonsimultaneous masking. J Acoust Soc Am 54:1471–1488
Meddis R, O’Mard LP, Lopez-Poveda EA (2001) A computational algorithm for computing nonlinear auditory frequency selectivity. J Acoust Soc Am 109:2852–2861
Nelson DA, Swain AC (1996) Temporal resolution within the upper accessory excitation of a masker. Acust Acta Acust 82:328–334
Oxenham AJ (2001) Forward masking: adaptation or integration? J Acoust Soc Am 109:732–741 Oxenham AJ, Moore BC (1994) Modeling the additivity of nonsimultaneous masking. Hear Res
80:105–118
Plack CJ, Oxenham AJ (1998) Basilar-membrane nonlinearity and the growth of forward masking. J Acoust Soc Am 103:1598–1608
Plomp R (1964) The rate of decay of auditory sensation. J Acoust Soc Am 36:277–282
Vogten LLM (1978) Low-level pure-tone masking: a comparison of “tuning curves” obtained with simultaneous and forward masking. J Acoust Soc Am 63:1520–1527
Forward Masking: Temporal Integration or Adaptation? |
173 |
Comment by Kohlrausch
You provided evidence that, for one forward masking condition, the two schemes previously published to explain forward masking are conceptually equivalent and predict the same results.
My question: does this equality also apply to some of the additional properties of the adaptation loop scheme, which were considered to be important when this scheme was first proposed by Dirk Pueschel in his Ph.D. thesis? 1) The fact that forward masking curves become steeper for shorter maskers, and 2) that, in simultaneous masking, no such influence of masker duration on detection is observed, while, in contrast, signal duration has a considerable effect on detection.
In my understanding, the ability to model observation 1 lies in the different values of the time constants of the feedback loops, while observation 2 is primarily attributed to using a matched template for detection.
Reply
We would like to underline that our conclusion is that the temporal-window model can be viewed as a simplified adaptation model, not as a fully equivalent model. This implies that the temporal window model can not account for all effects that an adaptation model can cover. Particularly, changes in the forward masking curve as function of masker duration can, to our knowledge, not be accounted for by the temporal window model with a fixed set of parameters/time constants.
We do also attribute your second observation to the matched filter detection mechanism, which is conceptually different from the detector used in the temporal-window model as published in the literature. In our unified model, however, we used the matched filter detector for both the temporal-window processing scheme and the adaptation scheme. Thus, we disregarded potentially limiting effects of the original temporal-window model detector.
Comment by Plack
I agree that the TW model and the modified AD model are equivalent in most forward masking situations. However, there is considerable evidence to suggest that processes after the basilar membrane (BM) are effectively linear in the way they combine the energy of BM vibration over time, at least with respect to forward and backward masking (Plack et al. 2002, 2006). Hence, it may be advantageous to keep the non-linearity out of the adaptation loops if possible.
I’m not sure that I agree with the final statement in your paper that the AD model can be applied to a broader class of experimental masking conditions
174 |
S.D. Ewert et al. |
than the TW model. The TW model has been applied successfully to forward masking, backward masking, simultaneous masking, and increment and decrement detection. Being effectively a low-pass filter, the TW can also account for gap detection, temporal integration, and some aspects of modulation detection. Although the decision device in the AD model is more sophisticated than that in the TW model, my understanding is that the AD model is successful across the full range of temporal resolution and masking tasks only when combined with an additional processing stage, such as a modulation filterbank. I like the physiological realism of the adaptation stage, but do you know of any psychophysical result that requires a simulation of adaptation to model the data?
References
Plack CJ, Oxenham AJ, Drga V (2002) Linear and nonlinear processes in temporal masking. Acustica 88:348–358
Plack CJ, Oxenham AJ, Drga V (2006) Masking by inaudible sounds and the linearity of temporal summation. J Neurosci 26:8767–8773
Reply
The temporal-window (TW) model has been powerful to demonstrate the effects of cochlear compression on forward (and backward) masking and, in particular, to account for consequences of sensorineural hearing loss on forward masking functions.
We agree that the broad applicability of the original AD model is also related to its more general detector, while the TW model uses a more simple detection mechanism. Our point in the current study is that the TW model can only function correctly if there is the assumption of the (S+N)/N-ratio criterion in the decision process, i.e., the whole concept of persistence or temporal integration after compression only holds when it is connected with this specific criterion. This, in turn, is in principle equivalent to an adaptation mechanism. Thus, we argue that the TW model represents a concept which effectively provides correct predictions in these specific conditions, however, essentially being a simplified adaptation model. As such, it does not allow simulating an internal representation of the stimulus that reflects properties of adaptation as found in physiology. We agree that it might not be necessary for a model that is used for the prediction of psychophysical detection/masking data to resemble effects of neural adaptation in the internal representation. However, in our view, the AD model remains the more general approach and therefore seems applicable to a broader class of experiments.
Regarding your first point, we would like to point out that the non-linearity in the adaptation loops is, according to our analysis, to some extent equivalent to the division process in the ratio criterion of the TW model.