
aswath_damodaran-investment_philosophies_2012
.pdf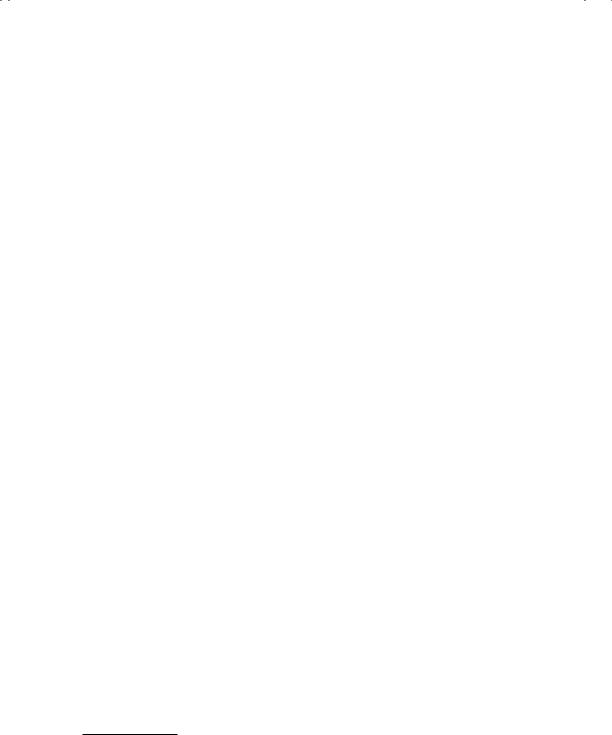
190 |
INVESTMENT PHILOSOPHIES |
S t r a t e g i e s f o r T e s t i n g M a r k e t E f f i c i e n c y
There are a number of different ways of testing for market efficiency, and the approach used will depend in great part on the investment scheme being tested. A scheme based on trading on information events (stock splits, earnings announcements, or acquisition announcements) is likely to be tested using an event study where returns around the event are scrutinized for evidence of excess returns. A scheme based on trading on an observable characteristic of a firm (price-earnings ratios, price-to-book value ratios, or dividend yields) is likely to be tested using a portfolio study approach, where portfolios of stocks with these characteristics are created and tracked over time to see if, in fact, they make excess returns. An alternative way of testing to see if there is a relationship between an observable characteristic and returns is to run a regression of the latter on the former. This approach allows for more flexibility if you are testing for interactions among variables. The following pages summarize the key steps involved in each of these approaches, and some potential pitfalls to watch out for when conducting or using these tests.
E v e n t S t u d y An event study is designed to examine market reactions to and excess returns around specific information events. The information events can be marketwide, such as macroeconomic announcements, or firmspecific, such as earnings or dividend announcements. The steps in an event study are:
1.The event to be studied is clearly identified, and the date on which the event was announced is pinpointed. The presumption in event studies is that the timing of the event is known with a fair degree of certainty. Since financial markets react to the information about an event rather than the event itself, most event studies are centered around the announcement date for the event.28
Announcement Date
___________________________________|___________________________________
2.Once the event dates are known, returns are collected around these dates for each of the firms in the sample. In doing so, two decisions have to be made. First, you have to decide whether to collect weekly, daily, or shorter-interval returns around the event. This will, in part, be decided
28In most financial transactions, the announcement date tends to precede the event date by several days, and sometimes weeks.

Too Good to Be True? Testing Investment Strategies |
191 |
by how precisely the event date is known (the more precisely it is known, the more likely it is that shorter return intervals can be used) and by how quickly information is reflected in prices (the faster the adjustment, the shorter the return interval to use). Second, you have to determine how many periods of returns before and after the announcement date will be considered as part of the event window. That decision also will be determined by the precision of the event date, since more imprecise dates will require longer windows.
R-jn |
....................................Rj0 |
...........................................R+jn |
_____________|_______________________|________________________|_________
Return window: –n to +n
where
Rjt = Returns on firm j for period t (t = –n, . . . , 0, . . . + n)
3.The returns, by period, around the announcement date are adjusted for market performance and risk to arrive at excess returns for each firm in the sample. You could use any of the risk and return models described in the preceding section to estimate excess returns. For instance, if the capital asset pricing model is used to control for risk:
Excess return on period t = Return on day t − (Risk-free rate
+ Beta Return on market on day t)
ER-jn ............................... |
ERj 0 |
.......................................ER+jn |
_____________|_______________________|________________________|_________
Return window: –n to +n
where
ERjt = Excess returns on firm j for period t (t = –n, . . . , 0, . . . + n)
You can also look at how a portfolio held over multiple periods would have done by measuring a cumulated abnormal return (CAR) by compounding the excess returns over the periods. Thus, if your excess return on day 1 is + 2 percent, day 2 is –1 percent, and day 3 is + 1.5 percent, your cumulative excess return over all three days would be:
Cumulated excess return = (1 + ER1)(1 + ER2)(1 + ER3) − 1
=(1.02)(0.99)(1.015) − 1 = 1.02495 or 2.495%
4.Once the excess returns are estimated for each firm in the sample, the average excess returns can be computed across the firms, and it will
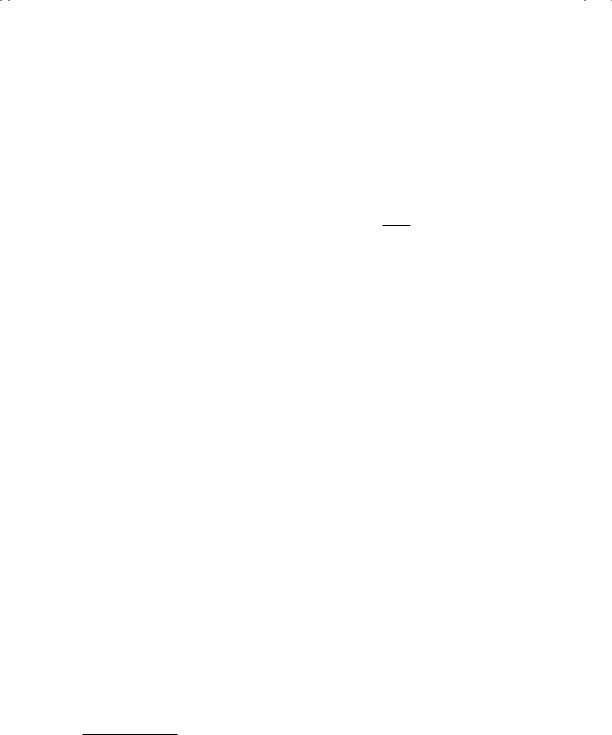
192 |
INVESTMENT PHILOSOPHIES |
almost never be equal to zero. To test to see whether this number is significantly different from zero, however, you need a statistical test. The simplest is to compute a standard deviation in the excess returns across the sampled firms, and to use this to estimate a t-statistic. Thus, if you have N firms in your sample and you have computed the excess returns each day for these firms:
J =N
Average excess return on day t =
ERJ t
N
J =1
T-statistic for excess return on day t = Average excess return/ Standard error
You can then check to see if the t-statistics are statistically significant. For instance, if the t-statistic is 2.33 or higher, there is a 99 percent chance that the average excess return is different from zero. If the average is positive, the event increases stock prices, whereas if the average is negative, the event decreases stock prices.
Consider the following illustrative example. Academics and practitioners have long argued about the consequences of option listing for stock price volatility. On the one hand, there are those who argue that options attract speculators and hence increase stock price volatility. This higher risk, they argue, should lead to lower stock prices. On the other hand, there are others who argue that options increase the available choices for investors and increase the flow of information to financial markets, and thus lead to lower stock price volatility and higher stock prices.
One way to test these alternative hypotheses is to do an event study, examining the effects of listing options on the underlying stocks’ prices. In 1989, Conrad did such a study, following these steps:29
Step 1: The dates on which the announcements were made that options would be listed on the Chicago Board Options Exchange on individual stocks was collected.
Step 2: The prices of the underlying stocks (j) were collected for each of the 10 days prior to the option listing announcement date, the day of the announcement, and each of the 10 days after.
Step 3: The returns on the stock (Rjt) were computed for each of these trading days.
29J. Conrad, “The Price Effect of Option Introduction,” Journal of Finance 44 (1989): 487–498.
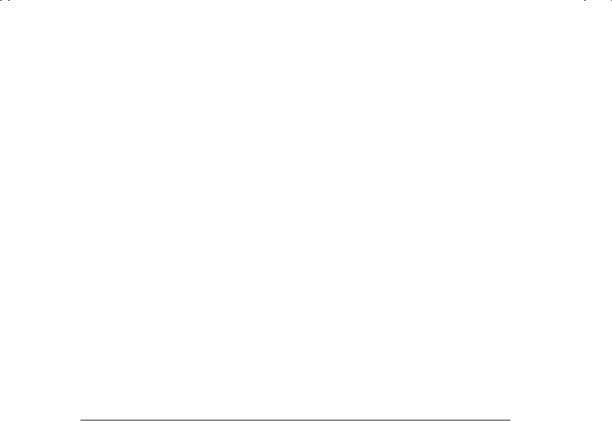
Too Good to Be True? Testing Investment Strategies |
193 |
Step 4: The beta for the stock ( j) was estimated using the returns from a time period outside the event window (using 100 trading days from before the event and 100 trading days after the event).
Step 5: The returns on the market index (Rmt) were computed for each of the 21 trading days.
Step 6: The excess returns were computed for each of the 21 trading days:
ER jt = Rjt − j Rmt . . . t = −10, −9, −8, . . . , +8, +9, +10
The excess returns were cumulated for each trading day.
Step 7: The average and standard error of excess returns across all stocks with option listings were computed for each of the 21 trading days. The t-statistics were computed using the averages and standard errors for each trading day. Table 6.4 summarizes the average excess returns and t-statistics around option listing announcement dates.
T A B L E 6 . 4 Excess Returns around Option Listing Announcement Dates
Trading Day |
Average Excess Return |
Cumulative Excess Return |
T-Statistic |
|
|
|
|
−10 |
0.17% |
0.17% |
1.30 |
−9 |
0.48% |
0.65% |
1.66 |
−8 |
−0.24% |
0.41% |
1.43 |
−7 |
0.28% |
0.69% |
1.62 |
−6 |
0.04% |
0.73% |
1.62 |
−5 |
−0.46% |
0.27% |
1.24 |
−4 |
−0.26% |
0.01% |
1.02 |
−3 |
−0.11% |
−0.10% |
0.93 |
−2 |
0.26% |
0.16% |
1.09 |
−1 |
0.29% |
0.45% |
1.28 |
0 |
0.01% |
0.46% |
1.27 |
1 |
0.17% |
0.63% |
1.37 |
2 |
0.14% |
0.77% |
1.44 |
3 |
0.04% |
0.81% |
1.44 |
4 |
0.18% |
0.99% |
1.54 |
5 |
0.56% |
1.55% |
1.88 |
6 |
0.22% |
1.77% |
1.99 |
7 |
0.05% |
1.82% |
2.00 |
8 |
−0.13% |
1.69% |
1.89 |
9 |
0.09% |
1.78% |
1.92 |
10 |
0.02% |
1.80% |
1.91 |
|
|
|
|
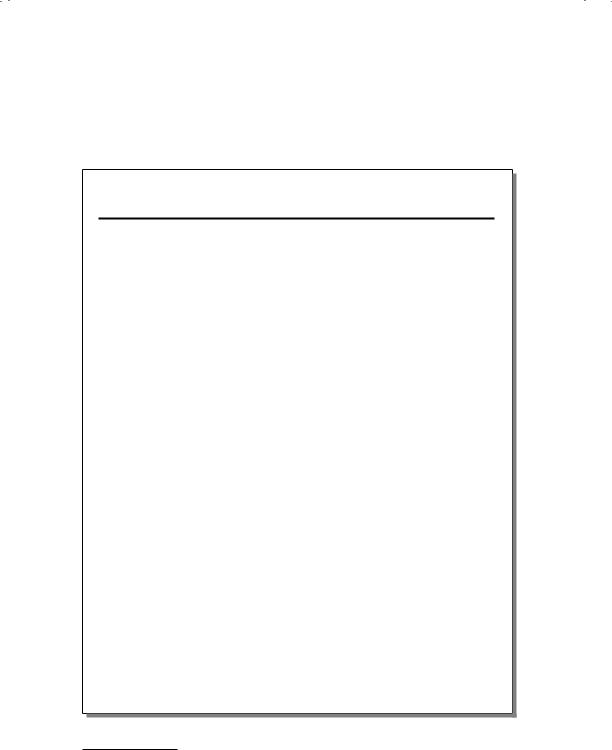
194 |
INVESTMENT PHILOSOPHIES |
Based on these excess returns, there is no evidence of an announcement effect on the announcement day alone, but there is mild30 evidence of a positive effect over the entire announcement period.
H O W D I F F E R E N T I S D I F F E R E N T ? S T A T I S T I C A L
V E R S U S E C O N O M I C S I G N I F I C A N C E
If you compare two samples on any dimension, you will get different results. Thus, you could compare the average returns on portfolios of companies with tall CEOs to the average returns of portfolios of companies with short CEOs, and you would find them to be different. But what should we read into the difference? If the average return on companies headed by tall CEOs is higher, should we rush out to buy stock in those companies? Not quite yet, because the differences often arise purely from chance.
The first test that you can run is a statistical test, where you apply the laws of probability to estimate the likelihood that the difference you are observing is purely random. This is what we do, for instance, when we compute a t-statistic on abnormal returns. If the t-statistic is 2.33, for example, we are saying that there is only a 1 percent chance that the difference we are observing is random and a 99 percent chance that returns are higher on companies with tall CEOs. If the t-statistic has been only 0.50, there would have been a 31 percent chance that the difference was purely random. In fact, it is common to test for statistical significance at the 1 percent or 5 percent levels; that is, only differences where the probability of randomness is less than 1 percent or 5 percent would be viewed as statistically significant.
The second test is for economic significance. When the difference between two samples is economically significant, you can make money off the difference. In the example that we have used, you could buy stocks with tall CEOs and sell stocks with short CEOs and make excess returns on your investment. Statistical significance does not always equal economic significance. First, you may have transaction costs that are much higher than the difference in returns between the two groups. Note that the larger the sample you use, the more likely it is that even small differences can be statistically significant. Thus, a
30The t-statistics are marginally significant at the 5 percent level.
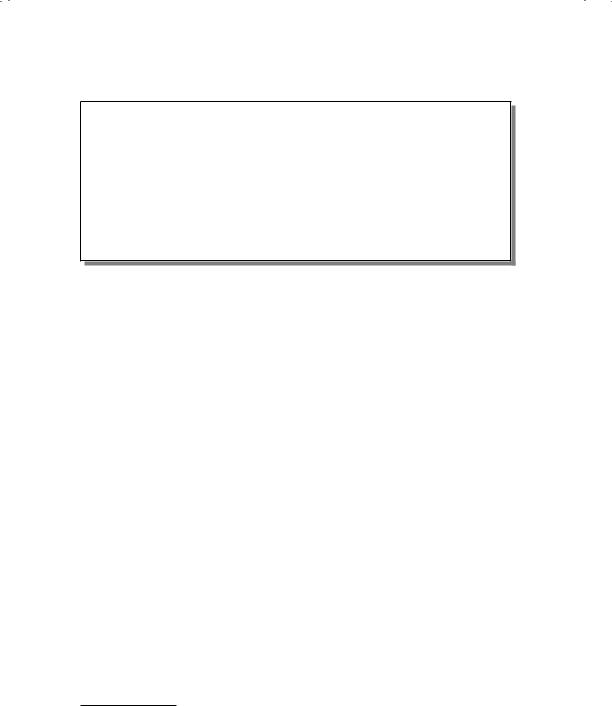
Too Good to Be True? Testing Investment Strategies |
195 |
difference of 0.20 percent may be statistically significant, but it clearly is not sufficient to cover execution costs. Second, you have the thorny issue of causation not being equal to correlation. In other words, all you have established is a correlation between returns and CEO height,
but you have not established causation. Do firms with tall CEOs earn higher returns or do firms with higher returns hire tall CEOs? If it is the latter, you may very well find statistical significance but not economic profits.
P o r t f o l i o S t u d y In some investment strategies, firms with specific characteristics are viewed as more likely to be undervalued, and therefore to have excess returns, than firms without these characteristics. In these cases, the strategies can be tested by creating portfolios of firms possessing these characteristics at the beginning of a time period and examining returns over the time period. To ensure that these results are not colored by the idiosyncrasies of one time period, this analysis is repeated for a number of periods. The seven steps in doing a portfolio study are:
1.The variable on which firms will be classified is defined, using the investment strategy as a guide. This variable has to be observable, though it does not have to be numerical. Examples would include market value of equity, bond ratings, stock prices, price-earnings ratios, and price-to- book value ratios.
2.The data on the variable is collected for every firm in the defined sample31 at the start of the testing period, and firms are classified into portfolios based on the magnitude of the variable. Thus, if the priceearnings ratio is the screening variable, firms are classified on the basis of P/E ratios into portfolios from lowest P/E to highest P/E classes. The number of classes will depend on the size of the sample, since there have to be sufficient firms in each portfolio to get some measure of diversification.
3.The returns are collected for each firm in each portfolio for the testing period, and the returns for each portfolio are computed, making an
31Though there are practicial limits on how big the sample can be, care should be taken to make sure that no biases enter at this stage of the process. An obvious one would be to pick only stocks that have done well over the time period for the universe.
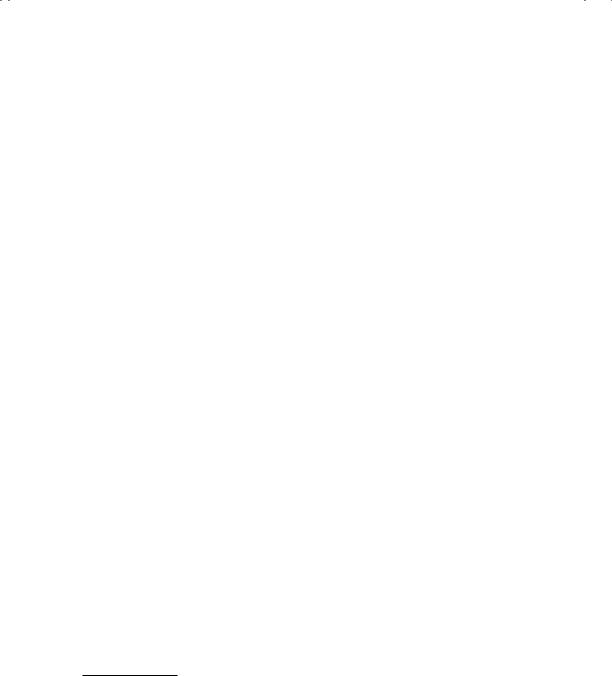
196 |
INVESTMENT PHILOSOPHIES |
assumption about how stocks will be weighted—some studies use equal weightings whereas others are value weighted.
4.The beta (if using a single-factor model like the CAPM) or betas (if using a multifactor model like the arbitrage pricing model) of each portfolio are estimated, either by taking the average of the betas of the individual stocks in the portfolio or by regressing the portfolio’s returns against market returns over a prior time period (for instance, the year before the testing period).
5.The excess returns earned by each portfolio are computed, in conjunction with the standard error of the excess returns.
6.There are a number of statistical tests available to check whether the average excess returns are, in fact, different across the portfolios. Some of these tests are parametric32 (they make certain distributional assumptions about excess returns) and some are nonparametric.33
7.As a final test, the extreme portfolios can be matched against each other to see whether there are statistically significant differences across these portfolios.
To illustrate this process, consider the following. Practitioners have claimed that low price-earnings ratio stocks are generally bargains and do much better than the market or stocks with high price-earnings ratios. This hypothesis can be tested using a portfolio approach:
Step 1: Using data on price-earnings ratios from the end of 1987, firms on the New York Stock Exchange were classified into five groups, the first group consisting of stocks with the lowest P/E ratios and the fifth group consisting of stocks with the highest P/E ratios. Firms with negative price-earnings ratios were ignored.
Step 2: The returns on each portfolio were computed using data from 1988 to 1992. Stocks that went bankrupt or were delisted were assigned a return of –100 percent.
Step 3: The betas for each stock in each portfolio were computed using monthly returns from 1983 to 1987, and the average beta for each
32One parametric test is an F test, which tests for equality of means across groups. This test can be conducted assuming either that the groups have the same variance or that they have different variances.
33An example of a nonparametric test is a rank sum test, which ranks returns across the entire sample and then sums the ranks within each group to check whether the rankings are random or systematic.
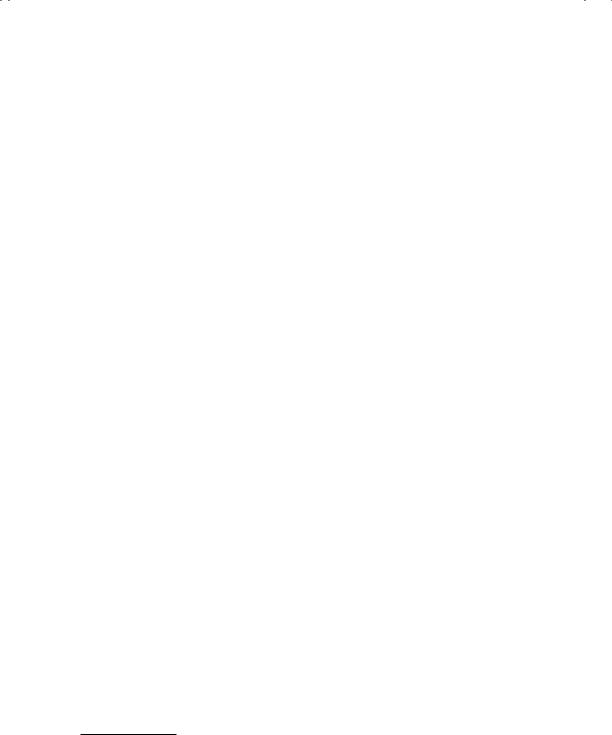
Too Good to Be True? Testing Investment Strategies |
|
|
197 |
|||
T A B L E 6 . 5 |
Excess Returns from 1988 to 1992 for P/E Ratio Portfolios |
|||||
|
|
|
|
|
|
|
P/E Class |
1988 |
1989 |
1990 |
1991 |
1992 |
1988–1992 |
|
|
|
|
|
|
|
Lowest |
3.84% |
−0.83% |
2.10% |
6.68% |
0.64% |
2.61% |
2 |
1.75% |
2.26% |
0.19% |
1.09% |
1.13% |
1.56% |
3 |
0.20% |
−3.15% |
−0.20% |
0.17% |
0.12% |
−0.59% |
4 |
−1.25% |
−0.94% |
−0.65% |
−1.99% |
−0.48% |
−1.15% |
Highest |
−1.74% |
−0.63% |
−1.44% |
−4.06% |
−1.25% |
−1.95% |
portfolio was estimated. The portfolios were assumed to be equally weighted.34
Step 4: The returns on the market index were computed each year from 1988 to 1992.
Step 5: The excess returns on each portfolio were computed each year, using the actual returns estimated from step 2, the betas estimated from step 3, and the market returns from step 4:
Excess return in year t = Actual return on portfolio in year t
−[Risk-free rate at the start of year t
−Beta (Return on market in year t
−Risk-free rate at the start of year t)]
Table 6.5 summarizes the excess returns each year from 1988 to 1992 for each portfolio.
Step 6: While the ranking of the returns across the portfolio classes seems to confirm our hypothesis that low-P/E stocks earn a higher return, we have to consider whether the differences across portfolios are statistically significant. There are several tests available, but these are a few:
An F test can be used to accept or reject the hypothesis that the average returns are the same across all portfolios. A high F score would lead us to conclude that the differences are too large to be random.
F statistic for difference across P/E portfolios between 1988 and 1992 = 14.75
34This will be a function of your strategy. If your strategy requires market- cap-weighted holdings, you would have to modify the test accordingly.

198 |
INVESTMENT PHILOSOPHIES |
This suggests that there is less than a 1 percent chance that the difference between the portfolios is random.
A chi-squared test is a nonparametric test that can be used to test the hypothesis that the means are the same across the five portfolio classes.
Chi-squared statistic for difference across P/E portfolios, 1988–1992 = 36.16
This confirms our conclusion from the F test that the differences are statistically significant.
We could isolate just the lowest P/E and highest P/E stocks and estimate a t-statistic that the averages are different across these two portfolios. In this case, the t-statistics that we obtain when we compare the returns on the lowest and highest P/E ratio classes is 5.61. This difference is also statistically significant.
R e g r e s s i o n s One of the limitations of portfolio studies is that they become increasing unwieldy as the number of variables that you use in your strategy increases. For instance, assume that you pick stocks that have low P/E ratios and low institutional investment, and whose stock prices have done well in the past six months. You could categorize all firms in your sample into five portfolios, based on each variable, but you would end up with 125 portfolios overall because of the potential interactions among the variables. The other problem with portfolio studies is that you group firms into classes and ignore differences across firms within each class. Thus, the stocks in the lowest P/E ratio class may have P/E ratios that range from 4 to 12. If you believe that these differences may affect the expected returns on your strategy, you could get a better measure of the relationship by running a multiple regression. Your dependent variable would be the returns on stocks, and the independent variables would include the variables that form your strategy. There are four steps to running a regression:
Step 1: Identify your dependent variable. This is the variable that you are trying to explain. In most investment schemes, it will be a measure of the return you would make on the investment, but you have to make at least two judgments. The first is whether you plan to use total returns or excess returns; with the latter, you would adjust the returns for risk and market performance, using one the measures discussed earlier in the chapter. The second decision you have to make is on the return interval you will be using—monthly,
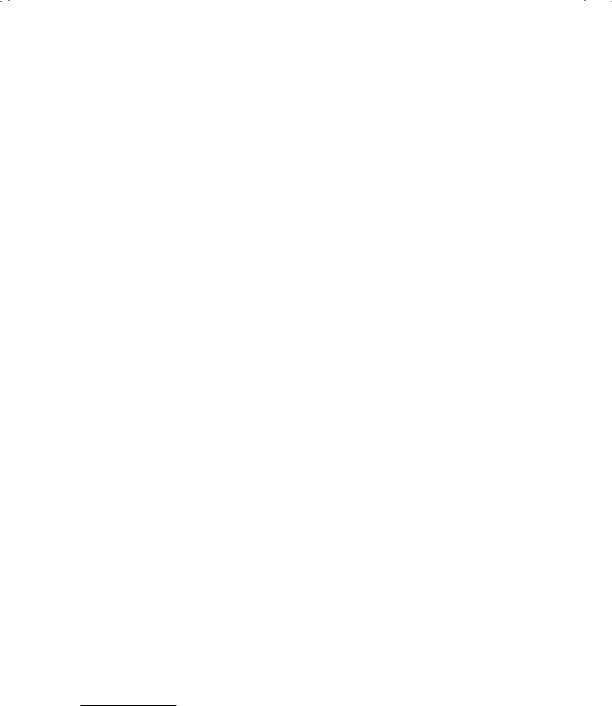
Too Good to Be True? Testing Investment Strategies |
199 |
quarterly, annual, or five-year, for instance. This choice will be determined both by your investment strategy—long-term strategies require long-term returns—and by the ease with which you can get data on your independent variables for the intervals. For instance, if you use accounting variables such as earnings or book value as independent variables, you will be able to get updates only once every quarter for these variables.
Step 2: Decide on how you will measure the variables that will underlie you strategy. For instance, in the example cited earlier, you will have to define P/E ratios, institutional investment, and stock price momentum with more specificity. With P/E ratios, you will have to choose between different measures of earnings—primary or diluted, before or after extraordinary items, current or trailing. With institutional investment, you could measure the institutional holdings as a percentage of outstanding stock or as a percentage of float (stock that is traded), and you will also have to decide whether you will consider all institutional investors or only certain kinds (mutual funds, pension funds, etc.). With stock price momentum, you may have to choose between percent changes over the previous six months, which will bias you toward lower-priced stocks, or absolute changes, which will bias you toward higher-priced stocks. Once you have determined your independent variables, you will have to collect information on them at the beginning of each of your testing periods. For instance, if you decide that annual returns in the year 2000 will be your dependent variable, you will have to collect information on P/E ratios and institutional holdings from January 1, 2000, and stock price momentum from June 30, 1999, to January 1, 2000.35
Step 3: You should check for the nature of the relationship between the dependent variable and each independent variable. A scatter plot provides a simple graphical tool for doing this. You are checking to see not only if there is a relationship but also for whether the relationship is linear. Figure 6.1 presents two scatter plots.
Panel A represents a scatter plot with a linear relationship, but Panel B is more consistent with a nonlinear relationship. If you
35For institutional holdings, you will have to use whatever values you would have been able to obtain from public sources as of January 1, 2000. Since there is a delay before institutions file with the SEC, you may not know the holdings as of January 1 until much later in the year.