
Kluwer - Handbook of Biomedical Image Analysis Vol
.1.pdfWavelets in Medical Image Processing |
355 |
[79]Wang, J., Li, J., Gray, R., and Wiederhold, G., Unsupervised multiresolution segmentation for images with low depth of field, IEEE Trans. Pattern Anal. Mach. Intell., Vol. 23, No. 1, pp. 85–90, 2001.
[80]Etemad, K., Doermann, D., and Chellappa, R., Multi-scale segmentation of unstructured document pages using soft decision integration, IEEE Trans. Pattern Anal. Mach. Intell., Vol. 19, No. 1, pp. 92–96, 1997.
[81]Porter, R. and Canagarajah, N., A robust automatic clustering scheme for image segmentation using wavelets, IEEE Trans. Image Process., Vol. 5, No. 4, pp. 662–665, 1996.
[82]Zhang, J., Wang, D., and Tran, Q., A wavelet-based multiresolution statistical model for texture, IEEE Trans. Image Process., Vol. 7, No. 11, pp. 1621–1627, 1998.
[83]Choi, H. and Baraniuk, R., Multis-cale image segmentation using wavelet-domain hidden markov models, IEEE Trans. Image Process., Vol. 10, No. 9, pp. 1309–1321, 2001.
[84]Li, J. and Gray, R., Context-based multi-scale classification of document images using wavelet coefficient distributions, IEEE Trans. Image Process., Vol. 9, No. 9, pp. 1604–1616, 2000.
[85]Charalampidis, D. and Kasparis, T., Wavelet-based rotational invariant roughness features for texture classification and segmentation, IEEE Trans. Image Process., Vol. 11, No. 8, pp. 825–837, 2002.
[86]Chan, T. F. and Vese, L. A., Active controus without edges, IEEE Trans. Image Process., Vol. 10, No. 2, pp. 266–277, 2001.
[87]Yezzi, A., Tsai, A., and Willsky, A., A statistical approach to image segmentation for biomodal and trimodal imagery, ICCV, pp. 898–903, 1999.
[88]Canny, J., A computational approach to edge detection, IEEE Trans. Pattern Anal. Mach. Intell., Vol. 8, No. 6, pp. 679–698, 1986.
[89]Aydin, T., Yemez, Y., Anarim, E., and Sankur, B., Multi-directional and multi-scale edge detection via M-band wavelet Transform, IEEE Trans. Image Process., Vol. 5, No. 9, pp. 1370–1377, 1996.
356 |
Jin, Angelini, and Laine |
[90]Laine, A. F., Huda, W., Chen, D., and Harris, J. G., Local enhancement of masses using continuous scale representations, J. Math. Imaging Vis., Vol. 7, No. 1, 1997.
[91]Laine, A., and Zong, X., Border indentification of echocardiograms via multi-scale edge detection and shape modeling, In: IEEE International Conference on Image Processing, Lausanne, Switzerland, pp. 287–290, 1996.
[92]Koren, I., Laine, A. F., Fan, J., and Taylor, F. J., Edge detection in echocardiographic image sequences by 3-D multiscale analysis, IEEE International Conference on Image Processing, Vol. 1, No. 1, pp. 288– 292, 1994.
[93]Dima, A., Scholz, M., and Obermayer, K., Automatic segmentation and skeletonization of neurons from confocal microscopy images based on the 3-D wavelet transform, IEEE Trans. Image Process., Vol. 11, No. 7, pp. 790–801, 2002.
[94]Wilson, R., Calway, A., and Pearson, R., A generalized wavelet transform for Fourier analysis: The multiresolution Fourier transform and its application to image and audio signal analysis, IEEE Trans. Inf. Theory, Vol. 38, No. 2, pp. 674–690, 1992.
[95]Yoshida, H., Katsuragawa, S., Amit, Y., and Doi, K., Wavelet snake for classification of nodules and false positives in digital chest radiographs, In: IEEE EMBS Annual Conference, Chicago, IL, pp. 509–512, 1997.
[96]deRivaz, P. and Kingsbury, N., Fast Segmentation using level set curves of complex wavelet surfaces, In: IEEE International Conference on Image Processing, Vol. 3, pp. 29–32, 2000.
[97]Wu, H., Liu, J., and Chui, C., A wavelet frame based image force model for active contouring algorithms, IEEE Trans. Image Process., Vol. 9, No. 11, pp. 1983–1988, 2000.
[98]Sun, H., Haynor, D., and Kim, Y., Semiautomatic video object segmentation using VSnakes, IEEE Trans. Circuits Syst. Video Technol., Vol. 13, No. 1, pp. 75–82, 2003.
Wavelets in Medical Image Processing |
357 |
[99]Neves, S., daSilva, E., and Mendonca, G., Wavelet-watershed automatic infrared image segmentation method, IEEE Electron. Lett., Vol. 39, No. 12, pp. 903–904, 2003.
[100]Bello, M., A combined Markov random field and wave-packet transform-based approach for image segmentation, IEEE Trans. Image Process., Vol. 3, No. 6, pp. 834–846, 1994.
[101]Davatzikos, C., Tao, X., and Shen, D., Hierarchical active shape models using the wavelet transform, IEEE Trans. Med. Imaging, Vol. 22, No. 3,
pp.414–423, 2003.
[102]Strickland, R. N. and Hahn, H. I., Wavelet transforms for detecting microcalcifications in mammograms, IEEE Trans. Med. Imaging, Vol. 15, No. 2, pp. 218–229, 1996.
[103]Zhang, X. and Desai, M., Segmentation of bright targets using wavelets and adaptive thresholding, IEEE Trans. Image Process., Vol. 10, No. 7,
pp.1020–1030, 2001.
[104]Allen, R., Kamangar, F., and Stokely, E., Laplacian and orthogonal wavelet pyramid decompositions in coarse-to-fine registration, IEEE Trans. Signal Process., Vol. 41, No. 12, pp. 3536–3541, 1993.
[105]Unser, M., Thevenaz, P., Lee, C., and Ruttimann, U., Registration and statistical analysis of PET images using the wavelet transform, IEEE Eng. Med. Biol. (September/October), pp. 603–611, 1995.
[106]McGuire, M. and Stone, H., Techniques for multiresolution image registration in the presence of occlusions, IEEE Trans. Geosci. Remote Sensing, Vol. 38, No. 3, pp. 1476–1479, 2000.
[107]Zheng, Q. and Chellappa, R., A computational vision approach to image registration, IEEE Trans. Image Process., Vol. 2, No. 3, pp. 311–325, 1993.
[108]Moigne, J., Campbell, W., and Cromp, R., Automated parallel image registration technique based on the correlation of wavelet features, IEEE Trans. Geosci. Remote Sensing, Vol. 40, No. 8, pp. 1849–1864, 2002.
358 |
Jin, Angelini, and Laine |
[109]Dinov, I., Mega, M., Thompson, P., Woods, R., Sumners, D., Sowell, E., and Toga, A., Quantitative comparison and analysis of brain image registration using frequency-adaptive wavelet shrinkage, IEEE Trans. Inf. Technol. Biomed., Vol. 6, No. 1, pp. 73–85, 2002.
[110]Unser, M. and Blu, T., Mathematical properties of the JPEG2000 wavelet filters, IEEE Trans. Image Process., Vol. 12, No. 9, pp. 1080– 1090, 2003.
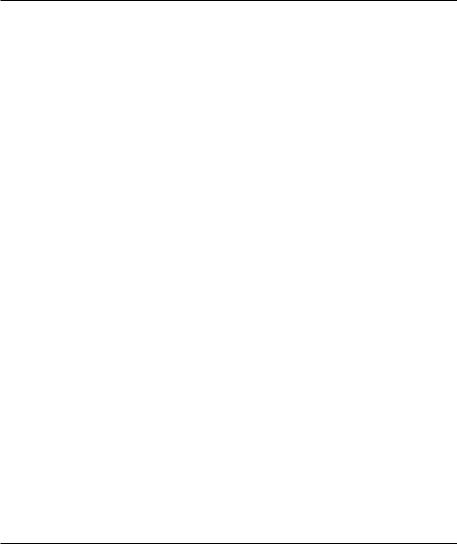
360 Giraldi, Rodrigues, Marturelli, and Silva
dependence of parametric snake models and can be extended to 3D through the theory of minimal surfaces [11, 57].
Implicit models, such as the formulation used in [46], consist of embedding the snake as the zero level set of a higher dimensional function and to solve the corresponding equation of motion. Such methodologies are best suited to the recovery of objects with unknown topologies.
Parametric deformable models are more intuitive than the implicit and geodesic ones. Their mathematical formulation makes it easier to integrate image data, initial estimated, desired contour properties and knowledge-based constraints, in a single extraction process [6].
However, parametric models also have their limitations. First, most of these methods can only handle objects with simple topology. The topology of the structures of interest must be known in advance since the mathematical model cannot deal with topological changes without adding extra machinery [21–47]. Second, parametric snakes are too sensitive to their initial conditions due to the nonconvexity of the energy functional and the contraction force which arises from the internal energy term [37, 79]. Several works have been done to address the mentioned limitations.
Topological restrictions can be addressed through a two-step approach: firstly, a method of identifying the necessity of a topological operation (split or merge) and secondly, a procedure of performing it. In [21] we found such a methodology that can split a closed snake into two closed parts. This is accomplished by first constructing a histogram of the image force norm along the snake to identify the appropriate region to cut it (region with weakest image field). Next, the method identifies two points in this region to be the end points of the segment which will cut the curve into two parts. The criterion to do this is based on the direction of an area force used to make the contour fit concave parts. This methodology has the disadvantages of not dealing with the contour merges and its extension to the 3D case is very difficult.
In [65] another approach is presented. It seeds particles on the surface of an object until their density on the surface is within some threshold value. Its components are a dynamical particle system and an efficient triangulation scheme which connects the particles into a continuous polygonal surface model consistent with the particles configuration. Particles are oriented; that is, each one has a position and a normal vector associated. The interparticle forces are used to encourage neighboring oriented particles to lie in each other’s tangent planes, and therefore favor smooth surfaces. This technique has the advantage of dealing
Improving the Initialization, Convergence, and Memory Utilization |
361 |
easily with open and closed surfaces. The topology of the particle-based surface can be modified during the triangulation step. However, this has the disadvantages of being expensive (O (N) log N ) where N is the number of particles) and that it may be difficult or cumbersome to find good initial seed particle sites, especially automatically [50].
A more general approach to incorporate topological changes in the parametric snake models is the T-snakes model [47–50]. The method embeds the snake model within a framework defined by a simplicial domain decomposition, using classical results in the field of numerical continuation methods [1]. The resulting model has the power of an implicit one without the need for a higher dimensional formulation [46]. Besides, it can be efficiently extended to 3D, generating the T-surfaces model [49].
The sensitivity to the initialization is a very common problem for deformable models. The use of simulated annealing for minimization was proposed in [62]. Despite the global optimization properties, the use of this technique is limited to both its computational complexity and memory requirements.
Levine et al. [44] applied hierarchical filtering methods, as well as a continuation method based on a discrete scale-space representation. At first, a scalespace scheme is used at a coarse scale to get closer to the global energy minimum represented by the desired contour. In further steps, the optimal valley or contour is sought at increasingly finer scales.
These methods address the nonconvexity problem but not the adverse effects of the internal normal force. This force is a contraction force which makes the curve collapse into a point if the external field is not strong enough. In Cohen [18] and Gang et al. [79] this problem is addressed by the addition of another internal force term to reduce the adverse effects of the contraction force. In both works the number of parameters is increased if compared with the original model and there are some trade-offs between efficiency and performance.
Another way to remove the undesired contraction force of the original snake model is to use the concept of invariance, which is well known in the field of computer vision [26, 36]. This concept has been applied to closed contours, and consists in designing an internal smoothing energy, biased toward some prior shape, which has the property of being invariant to scale, rotation, and translation. In these models, the snake has no tendency to expand or contract, but it tends to acquire a natural shape.
An example of a technique, which applies invariance concepts, is the dual active contour (dual ACM) [37]. This approach basically consists of one contour
362 Giraldi, Rodrigues, Marturelli, and Silva
which expands from inside the target feature, and another one which contracts from the outside. The two contours are interlinked to provide a driving force to carry the contours out of local minima, which makes the solution less sensitive to the initial position.
The sensitivity to initialization of snakes can also be addressed by a two-stage approach: (1) The region of interest is limited; and (2) a global minimization technique is used to find the object boundary. Bamford and Lovell [4] describe such a method to segment cell nucleus based on a dynamic programming algorithm ( Viterbi algorithm) to find the solution.
The use of dynamic programming (DP) for solving variational problems is discussed by Amini et al. [2]. Unlike the variational approach, DP ensures global optimality of the solution and does not require estimates of higher order derivatives, which improves the numerical stability. However, these techniques are limited by their storage requirements of O (N M2) and computational complexity of O (N M3), where N is the number of snaxels and M is the size of the neighborhood around each snaxel (given a discrete search space with N M points). These performance difficulties can be lowered with a method to reduce the search space. That is the main point addressed in [32, 34].
In those works, we propose to reduce the search space through the dual- T-snakes model [30] by its ability to get closer to the desired boundaries. The result is two contours close to the border bounding the search space. Hence, a DP algorithm [2, 4, 38] can be used more efficiently.
The sensitivity to the initial contour position can also be addressed by a method which initializes automatically the snake closer to the boundaries [43]. An efficient methodology in this field would be worthwhile, not only to save time/calculation, but also to facilitate the specification of parameters, a known problem for snake models [31].
In [29, 33] we propose a method to initialize deformable models, which is based on properties related to the topology and spatial scale of the objects in 2D or 3D scenes. We assume some topological and scale properties for the objects of interest. From these constraints we propose a method which first defines a triangulation of the image domain. After that, we take a subsampling of the image field over the grid nodes. This field is thresholded, generating a binary one, an “object characteristic function,” from which a rough approximation of the boundary geometry is extracted. This method was extended to 3D in [63].

Improving the Initialization, Convergence, and Memory Utilization |
363 |
Neural networks and Hough transforms have also been applied for initialization of deformable models [14, 74].
An other possibility to address the sensitivity to initialization is the gradient vector flow, which is a scheme based on a vector diffusion–reaction equation. It was introduced in [77] and can be used to obtain a more efficient image force field [78].
Deformable models can be extended to 3D, generating deformable surface models. Besides the described problems, a new one arises when considering these models: memory utilization.
In general, deformable surface models make use of only the data information along the surface when evolving the model toward the object boundary [48, 49]. However, state-of-the-art implementations of these models in general do not account for this fact and fetch the whole volume from disk at the initialization. Such a procedure brings limitations for large size image volumes, mainly if we consider that, in general, deformable models need not only the image intensity but also the image gradient [42, 49].
Nowadays, image volumes with 5123 sampling points can be acquired in CT scanners. Besides, other scanning techniques were developed allowing the acquisition of a huge amount of 3D color image volumes (www.nlm.nih. gov/research/visible/visible human.html). In these cases, the data set information (image intensity and gradient) can be too large to fit in main memory, even if we take the usual cut policy: In a first stage, select a subvolume (a bounding box) that contains the structure of interest, and then segment it. When the size of the data that must be accessed is larger than the size of main memory, some form of virtual memory is simply required, which leads to performance problems [20].
The analysis of large data sets is a known problem in the context of scientific visualization [15, 24, 71]. Out-of-core techniques have been developed for scalar and vector fields visualization and new proposals are still in progress. Among these methods, out-of-core isosurface extraction techniques are closely related with our work, as we shall see next.
These methods partition the data set into clusters that are stored in disk blocks, and build a data structure to index the blocks for information retrieval ( preprocessing step). At run-time, the data structure is read to main memory and traverse to find out the data blocks that must be read to main memory to perform the isosurface generation. The most commonly used data structures, for