
Kluwer - Handbook of Biomedical Image Analysis Vol
.2.pdf182 |
Wong |
[128]Lange, N., Statistical approaches to human brain mapping by functional magnetic resonance imaging, Stat. Med., Vol. 15, pp. 389–428, 1996.
[129]Moser, E., Diemling, M., and Baumgartner, R., Fuzzy clustering of gradient-echo functional MRI in the human visual cortex. Part II: Quantification, J. Magn. Reson. Imaging, Vol. 7, pp. 1102–1108, 1997.
˚
[130] Goutte, C., Toft, P., Rostrup, E., Nielsen, F. A., and Hansen, L. K., On clustering fMRI time series, NeuroImage, Vol. 9, pp. 298–310, 1999.
[131] Fadili, M. J., Ruan, S., Bloyet, D., and Mazoyer, B., A multistep unsupervised fuzzy clustering analysis of fMRI time series, Hum. Brain Mapping, Vol. 10, pp. 160–178, 2000.
[132] Schmidt, K., Lucignani, G., Moresco, R. M., Rizzo, G., Gilardi, M. C., Messa, C., Colombo, F., Fazio, F., and Sokoloff, L., Errors introduced by tissue heterogeneity in estimation of local cerebral glucose utilization with current kinetic models of the [18F]fluorodeoxyglucose method, J. Cereb. Blood Flow Metab., Vol. 12, pp. 823–834, 1992.
[133] Popper, K. R., Normal science and its dangers, In: Criticism and the Growth of Knowledge, Lakatos, I. and Musgrave, A., eds., Cambridge University Press, Cambridge, pp. 51–58, 1970.
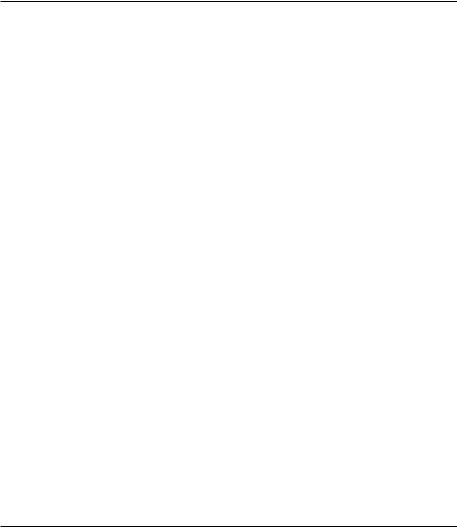
Chapter 4
Automatic Segmentation of Pancreatic
Tumors in Computed Tomography
Maria Kallergi,1 Marla R. Hersh,1 and Anand Manohar1
4.1 Introduction
Pancreatic cancer is the fourth leading cause of cancer deaths in the United States but only the tenth site for new cancer cases (estimated at 30,300 in 2002) [1, 2]. The reason for this major difference is that there are no clear early symptoms of pancreatic cancer and no screening procedures or screening policy for this disease. It is usually diagnosed at a late stage and has a poor prognosis with a 1-year survival rate of 20% and a 5-year survival rate of less than 5% [3]. Complete surgical resection is the only way to significantly improve prognosis and possibly lead to a cure. Unfortunately, only 15–20% of the patients can undergo resection with median survival rates from 12 to 19 months and a 5-year survival rate of 15–20%. A large majority of patients with pancreatic cancer receives palliative care or may follow a therapeutic approach the impact of which is currently quite limited and difficult to assess quantitatively [3].
Pancreatic cancer is a disease that is not extensively studied and is poorly understood. A significant risk factor associated with pancreatic cancers is age (frequency increases linearly after 50 years of age) with median age of diagnosis of 71 years. In addition to aging, other probable risk factors include family history, cigarette smoking, long-standing diabetes, and chronic and hereditary pancreatitis [2]. Studies have also implicated, without any consistency,
1 Department of Radiology, H. Lee Moffitt Cancer Center & Research Institute, University
of South Florida, Tampa, FL 33612
183
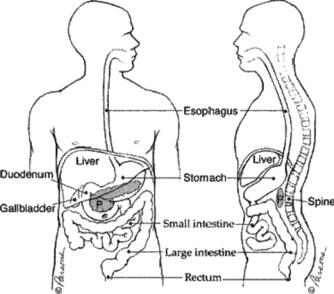
184 |
Kallergi, Hersh, and Manohar |
Figure 4.1: Human anatomy indicating the location of the pancreas and major neighboring organs (reprinted from http://pathology2.jhu.edu/pancreas/ pancreas1.cfm).
a number of other factors including alcohol consumption, diet and nutrition, occupational exposures, genetic predisposition, multiple endocrine neoplasia, hereditary nonpolyposis colorectal cancer, familial adenomatous polyposis and Gardner syndrome, familial atypical multiple mole melanoma syndrome, von Hippel-Lindau syndrome, and germline mutations in the BRCA2 gene [4–6]. Tumor markers have been used for pancreatic cancer including carcinoembryonic antigen (CEA) and carbohydrate antigen 19-9 (CA 19-9) but no conclusive results exist to date [2].
Figure 4.1 shows a drawing of the human anatomy, the location of the pancreas, and major surrounding organs and structures. The pancreas lies in the upper abdomen, transversely behind the stomach, with its tip very close to the hilum of the spleen. It is an elongated organ with three major parts: head, body, and tail as shown in Fig. 4.2. The pancreas is a gland that produces regulating hormones and enzymes for protein breakdown. The enzymes are secreted into the duodenum through a set of tubes called pancreatic ducts.
Pancreatic cancer mostly originates in the ductal system of the pancreas (95% of the cases) and is termed ductal adenocarcinoma [7]; pancreatic cancer that affects the endocrine cells is called islet cell cancer [8]. About 60% of all
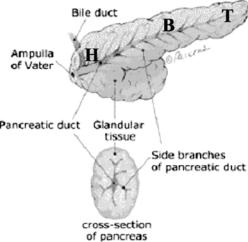
Automatic Segmentation of Pancreatic Tumors in Computed Tomography |
185 |
Figure 4.2: Detailed structure and main parts of the pancreas where H = head,
B = body, and T = tail (reprinted from http://pathology2.jhu.edu/pancreas/ pancreas1.cfm).
pancreatic cancers occur in the head or neck of the pancreas, about 15% occur in the body of the pancreas, about 5% in the tail, and about 20% are diffused through the gland [7]. Pancreatic cancer metastasizes rapidly even when the primary tumors are less than 2 cm. Metastasis most commonly occurs to regional lymph nodes, then to liver, and less commonly to the lungs. The tumors display a high degree of resistance to conventional chemotherapy and radiation therapy.
The National Cancer Institute (NCI) created a Review Group in 2001 to define an agenda for action for pancreatic cancer and the research priorities that could reduce morbidity and mortality from this difficult disease [2]. The research priorities set by NCI spanned a wide range of areas including
tumor biology
risk, prevention, detection, and diagnosis
therapy
health sciences research
In each of the areas above, research priorities were defined that could lead to the advancement of our knowledge of the disease and improved health care for the patients. Our interest in this chapter is on the role of imaging and computer
186 |
Kallergi, Hersh, and Manohar |
techniques on the detection, diagnosis, and surveillance of pancreatic cancer [9]. Hence, this chapter reviews the imaging priorities set by the Review Group [2], the currently used imaging techniques and state-of-the-art computer methodologies, the requirements for development of computer algorithms for the automatic diagnosis, management, and treatment of the disease, and the challenges posed to the development of computer methodologies. A novel algorithm is also described for the segmentation and classification of pancreatic tumor. Preliminary results are reported from its application on computed tomography (CT) images of the pancreas.
4.2 Imaging of the Pancreas
4.2.1 Imaging Modalities
Imaging of the pancreas is done for diagnosis, staging, and surveillance of benign or malignant conditions of the gland; surveillance includes monitoring of the disease, the effects of treatments, and the biological processes [10]. Imaging is faced with formidable obstacles in the case of pancreatic cancer because of the complexity of the disease and its high incidence of metastasis. Early neoplastic changes are difficult to detect and diagnose. Most of the available imaging techniques fail to detect early signs of pancreatic cancer. It is almost always detected after it has been spread beyond the gland. Imaging of the pancreas may be done in a variety of ways:
1.Computed tomography (CT); standard [11, 12], helical [7, 13, 14], and multidetector [8, 15] with the latter becoming the dominant modality of choice.
2.Magnetic resonance imaging (MRI) [9, 16, 17].
3.Magnetic resonance cholangiopancreatography (MRCP) [18, 19].
4.Endoscopic retrograde cholangiopancreatography (ERCP) [20]
5.Endoscopic ultrasonography (EUS) [21, 22]
6.Positron emission tomography (PET) [23, 24]
The usage of one technique over another depends on availability, purpose of
imaging, and expertise. Additional considerations in the selection of an imaging
Automatic Segmentation of Pancreatic Tumors in Computed Tomography |
187 |
modality include the desired accuracy of the procedure for providing staging information, or its ability to perform simultaneous biopsy of the tumor, or its capacity to facilitate therapeutic procedures. Detection usually starts with transabdominal sonography to identify causes of pain. After sonography, CT is used as the primary modality for diagnosis and staging. MRI is also used for staging. MRCP and ERCP imaging provide additional information on the level of obstruction of the biliary or pancreatic ductal systems. Fine-needle aspiration of suspected pancreatic lesions can be done with EUS for increased biopsy specificity. Specificity is a problem with all imaging modalities as they do not make it possible to distinguish between pancreatic cancer and other pancreatic pathology, e.g., chronic pancreatitis, mucinous cystadenoma, and intraductal papillary mucinous neoplasms [10].
Today the most common modality for pancreatic imaging is helical CT, which has significantly improved outcomes relative to the standard CT or the other imaging modalities. Standard abdominal CT scans can help detect 70–80% of pancreatic carcinomas [3, 13]. But 40–50% of tumors smaller than 3 cm are missed, and these are the tumors most likely to be resectable. Helical CT improved significantly the resolution of conventional CT for pancreatic tumor imaging [25]. Helical CT has also impacted staging and treatment monitoring procedures and is now probably the most useful imaging technique for such investigations. Helical CT is the technique that will be focused on in this chapter.
Priorities set by the NCI Review Group for pancreatic cancer imaging include [2] (a) increase specificity of current imaging modalities, (b) increase sensitivity of current imaging modalities for small invasive and preinvasive lesions in both normal and abnormal pancreas, (c) develop and test molecular imaging techniques, (e) develop and test screening and surveillance imaging protocols for high-risk patients, and (f) develop and test noninvasive techniques to accurately define the effect of treatment. Computer aided diagnosis (CAD) schemes are computer algorithms that could assist the physician (radiologist or oncologist) in the interpretation of the radiographic images and the evaluation of the disease. CAD could play several roles in the above imaging priorities and contribute in several recommendations and research directions. CAD using CT scans seems to be the logical first step in the development of computer tools for pancreatic cancer because of the major role of CT in this area, the large amount of information available in CT scans, and the considerable potential for improvements that could have significant clinical impact independent of magnitude.
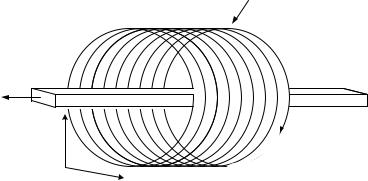
188 |
Kallergi, Hersh, and Manohar |
Continuous Source &
Detector Rotation
Continuous
Patient/Table
Translation
Z-axis
Y-axis
X-axis
Figure 4.3: Schematic diagram of the helical CT set-up and operation principle including cross-section (slice) plane (x,y), and z axis orientation.
4.2.2 Helical CT Imaging Characteristics
CT was developed in the early 1960s with the first clinical system installed in 1971 [26]. Today, there are five generations of CT scanners characterized by different scanning conditions and properties. Helical or spiral CT is the latest generation of scanners that combines a continuous rotation of the X-ray source and ring of detectors with a continuous movement of the examination table. Hence, data are acquired continuously while the patient is moved through the gantry [27, 28]. Figure 4.3 shows a drawing of the helical CT scanning principle and associated orientations [7]. Figure 4.4 shows a typical two-dimensional (2-D) CT slice through the abdomen. The major organs and structures are labeled including a tumor on the head of the pancreas (Arrow B).
Basic CT principles and operation description can be found in a variety of journal articles and dedicated books [27–29]; Van Hoe and Baert [7] provide a comprehensive summary of the advantages of helical CT relative to standard CT scans for pancreatic tumors, including a description of the various imaging parameters. Kopecky and colleagues from Indiana University Medical Center provide an excellent illustrative presentation of the physical characteristics of helical CT at http://www.indyrad.iupui.edu/public/lectures/multislice/sld001.htm. Herein we focus on the helical CT imaging parameters that may be of interest to the medical imaging scientist and engineer and pertinent to computer applications and CAD development. These parameters include the following:
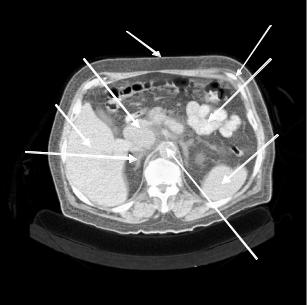
Automatic Segmentation of Pancreatic Tumors in Computed Tomography |
189 |
R
S
B
C
A
D
E
F
Figure 4.4: Contrast enhanced, helical CT scan through the abdomen and the head of the pancreas obtained with a reconstruction width of 8 mm (equal to slice thickness). A = liver; B = head of pancreas with tumor; C = bowel; D = spleen; E = right adrenal; F = aorta.
(a)Slice thickness (mm): This is equal to the collimation of the X-ray beam. Maximum slice thickness depends on the size of the detectors and is typically about 8–10 mm. Thick slices are used for general abdominal scans and they usually have better contrast resolution than do thin slices, which in turn have better spatial resolution and require higher radiation dose. Thin slices are used for small organs or to evaluate and review a region of interest in more detail. Pancreatic scans may be performed in either thick (8 mm) scans or thin (less than 4 mm) scans or combinations depending on the case and/or the protocol requirements. Different slice thicknesses result in different image properties and noise characteristics so they have to be carefully considered in computer applications including registration, reconstruction, and segmentation.
(b)Incrementation: This is related to the longitudinal or z-axis resolution and is also referred to as step or index. It defines the distance between consecutive slices. It is usually equal to the slice thickness leaving no gaps
190 |
Kallergi, Hersh, and Manohar |
between consecutive slices. In special cases, e.g., small organs or threedimensional (3-D) reconstructions, it may be smaller than slice thickness yielding an overlap between consecutive slices. Incrementation may be changed retrospectively in helical CT.
(c)Resolution: The field of view is related to the spatial resolution of the CT slices or the in-plane resolution. Based on the field of view and the size of the 2-D matrix (X and Y dimensions in pixels (see Fig. 4.3)) the spatial resolution or pixel size can be determined. The dynamic resolution or pixel depth is determined by the characteristics of the detector.
(d)Exposure: kVp and mAs are the two parameters that influence exposure with kVp defining the beam quality or the average intensity and mAs defining the quantity of the beam. For larger patients, the mAs may be increased.
(e)Pitch: This is the ratio of the distance traveled by the table during one full rotation of the detector (gantry) to the beam collimation. For example, a pitch of 1.0 corresponds to a scan with a beam collimation of 10 mm, 1-sec duration of a 360 degree rotation, and a rate of table movement of 10 mm/sec. By doubling the rate of table translation, the pitch is increased proportionally. In pancreatic screening scans the table speed is on the order of 15 mm/rotation and the pitch is 6. In diagnostic high-resolution scans the table speed is on the order of 6 with a pitch of 6. The higher the pitch the shorter the scan time. For multislice helical CT, pitch is also defined as the ratio of the table travel per gantry rotation to the nominal slice thickness. This is a more ambiguous definition, not applicable to single-slice helical CT, but often used by the manufacturers of multislice CT scanners [30].
(f)Contrast type and amount: For pancreatic scans, a contrast material (e.g., Omnipaque 320 or 350) is administered intravenously prior to imaging at a volume of 100–120 cc. Water, gastograffin, or barium is usually given as oral contrast. There is 50–60 sec scan delay to allow for optimum imaging of the pancreas after the administration of the contrast material.
(g)Data reconstruction interval: This is the thickness of the reconstructed slices and is usually equal to the scanned slice thickness.
Automatic Segmentation of Pancreatic Tumors in Computed Tomography |
191 |
Three-dimensional reconstructed CT volumes may be used to generate 2-D views of the organ in the coronal (XZ plane) and sagittal (YZ plane) modes in additional to the traditional transaxial view (XY plane) (Fig. 4.3).
A standard CT scan of the abdomen and pelvis consists of about 40 slices. An abdominal high-resolution scan of the pancreas may consist of 5–40 slices depending on the selected slice thickness and the orientation of the gland [31]. Slice thickness, slice interval, and slice starting point may be selected retrospectively in helical CT scans because of the continuous nature of the process. This is an advantage of the helical over the conventional CT imaging where these parameters need to be determined at the beginning of the scan at the risk of improper imaging of a lesion [32].
Despite its many advantages, e.g., high-spatial resolution and ability to identify vascular involvement, helical CT fails to detect small (<2cm) tumors, hepatic metastasis, and peritoneal implants, and also has low specificity to other pancreatic pathology [33]. Most patients with pancreatic cancer are evaluated by combinations of imaging tests rather than a single test. In addition to the limitations of the imaging technique, pancreatic tumor measurements and evaluation during treatment monitoring are done visually using subjective criteria by a single expert or, at best, a panel of experts. Hence, there is significant interobserver, and possibly intraobserver, variability in the evaluation of response to treatment and management of the patients with pancreatic cancer.
Important to the development of image processing techniques for pancreatic cancer applications is the knowledge of the clinical imaging characteristics of the normal and abnormal pancreas. The normal pancreas is relatively easy to delineate on CT slices. Understanding how the image of the normal pancreas may be distorted by disease and particularly pancreatic masses (benign or malignant) is the basis for selecting robust features for the development of automated segmentation, classification, registration, and reconstruction methodologies. The most important features used by the radiologists and oncologists in the evaluation of pancreatic adenocarcinoma on radiologic images are summarized in Table 4.1. These features are merely general observation that may not always hold.
There is variability in the imaging characteristics of pancreatic tumors on CT images that increases detection and diagnostic difficulty relative to other organ abnormalities. Table 4.1 summarizes the characteristics of adenocarcinoma