
Kluwer - Handbook of Biomedical Image Analysis Vol
.3.pdf390 |
Kybic and Unser |
Switzerland, 2001, http://cmp.felk.cvut.cz/kybic/thesis/jkthesis.ps.gz,
No. 2439.
[89]Liouville, J., J. Math. Pures Applications, Vol. 20, (1855), unnumbered, in French.
[90]Unser, M. and Blu, T., SIAM Review, Vol. 42, No. 1, pp. 43–67, 2000.
[91]Flandrin, P., IEEE Transactions Inform. Th., Vol. 38, No. 2, pp. 910–917, March 1992.
[92]Poggio, T. and Girosi, F., Proceedings of IEEE, Vol. 78, No. 0, pp. 1481– 1497, September 1990.
[93]Kybic, J., Thevenaz,´ P., and Unser, M., Compensation of unidirectional geometric distortion in EPI using spline warping, In: ICIP’99: Proceedings of the 1999 IEEE International Conference on Image Processing, Vol. II, pp. 168–172, IEEE Computer Society, 445 Hoes Lane, Piscataway, NJ, U.S.A., October 1999, ftp://cmp.felk.cvut.cz/ pub/cmp/articles/kybic/Kybic-ICIP1999.pdf.
[94]Kybic, J. and Unser, M., Multidimensional elastic registration of images using splines, In: Proceedings of ICIP, Vol. 2, pp. 455–458, 2000, ftp://cmp.felk.cvut.cz/pub/cmp/articles/kybic/Kybic-ICIP2000.ps.gz.
[95]Kybic, J., Thevenaz,´ P., Nirkko, A., and Unser, M., IEEE Transactions on Medical Imaging, Vol. 19, No. 2, pp. 80–93, February 2000,
[96]Kybic, J. and Unser, M., IEEE Transactions on Image Processing, November 2003,
[97]Studholme, C., Constable, R. T., and Duncan, J. S., Incorporating an image distortion model in non-rigid alignment of EPI with conventional MRI, In: International Conference on Information Processing in Medical Imaging, Lecture Notes in Computer Science, pp. 454–459, SpringerVerlag, Visegrad,´ Hungary, June 1999.
[98]Kybic, J., Thevenaz,´ P., Nirkko, A., and Unser, M., IEEE Transactions on Medical Imaging, Vol. 19, No. 2, pp. 80–93, February 2000.
[99]Pluim, J. P. W., Maintz, J. B. A., and Viergever, M. A., Image Registration by Maximization of Combined Mutual Information and Gradient
Elastic Registration for Biomedical Applications |
391 |
Information, IEEE Transactions Medical Imaging, Vol. 19, No. 8, August
2000.
[100]Unser, M., Splines: A Perfect Fit for Signal and Image Processing, IEEE Signal Processing Magazine, Vol. 16, No. 6, pp. 22–38, November 1999.
[101]Blu, T. and Unser, M., Quantitative Fourier analysis of approximation techniques: Part I—Interpolators and projectors, IEEE Transactions on Signal Processing, 1999.
[102]Fornefett, M., Rohr, K., and Stiehl, H. S., Elastic registration of medical images using radial basis functions with compact support, In: Proc. Computer Vision and Pattern Recognition (CVPR’99), pp. 402–407, Fort Collins, Co, 1999.
[103]Wu, Y.-T., Image Registration using wavelet-based motion model and its applications, Ph.D. Dissertation, University of Pittsburgh, Saint Louis, Mississippi, 1997.
[104]Schoenberg, I., Proc. Nat. Acad. Sci., Vol. 52, pp. 947–950, 1964.
[105]Ahlberg, J. H., Nilson, E. N., and Walsh, J. L., The theory of splines and their applications, Academic Press, New York, 1967.
[106]Unser, M., Aldroubi, A., and Eden, M., Signal Processing, Vol. 30, No. 2, January 1993.
[107]Kybic, J., Thevenaz,´ P., and Unser, M., Compensation of unidirectional geometric distortion in EPI using spline warping, In: Proceedings of the IEEE Internation Conference on Image Processing, pp. 168–172, Kobe, Japan, 1999.
[108]Muller,¨ F., Brigger, P., Illgner, K., and Unser, M., IEEE Transactions on Signal Processing, Vol. 46, No. 9, pp. 2555–2558, September 1998.
[109]Unser, M., Aldroubi, A., and Eden, M., IEEE Transactions on Pattern Analysis Mach. Intelligance, Vol. 15, No. 4, April 1993.
[110]Cachier, P., Mangin, J.-F., Pennec, X., et al., Multisubject non-rigid registration of brain MRI using intensity and geometric features, In: Proceedings of MICCAI, pp. 734–742, Utrecht, The Netherlands, 2001.
392 |
Kybic and Unser |
[111]Hellier, P. and Barillot, C., Cooperation between local and global approaches to register brain images, In: Proceedings of IPMI, pp. 315–328, 2001.
[112]Kybic, J., Biomedical Image Processing by Elastic Warping, Ph.D. thesis, Ecole Polytechnique Fed´erale´ de Lausanne, Lausanne, Switzerland, 2001, No. 2439, http://www-sop.inria.fr/robotvis/personnel/Jan.Kybic/ thesis/index.html.
[113]Kara cali, B. and Davatzikos, C., Topology preservation and regularity in estimated deformation fields, In: Proceedings of IPMI, Lecture Notes in Computer Science, Springer, 2003.
[114]Kanno, I. and Lassen, N., Journal of Computer Assisted Tomography, Vol. 1, No. 3, pp. 71–76, 1979.
[115]Kybic, J. and Unser, M., Multidimensional elastic registration of images using splines, In: Proceedings of ICIP, Vol. 2, pp. 455–458, Vancouver, Canada, 2000.
[116]Ledesmay-Carbayo, M. J., Kybic, J., Desco, M., Santos, A., and Unser, M., Cardiac motion analysis from ultrasound sequences using non-Rigid Registration, In: Proceedings of MICCAI, Niessen, W. J. and Viergever, M. A., eds., pp. 889–896, Springer-Verlag, Utrecht, The Netherlands, October 2001.
[117]Vallee,´ J.-P. et al., Magnetic Resonance in Medicine, Vol. 38, pp. 981– 989, 1997.
[118]Vallee,´ J.-P. et al., Journal of Magnetic Resonance Imaging, Vol. 9, pp. 197–203, 1999.
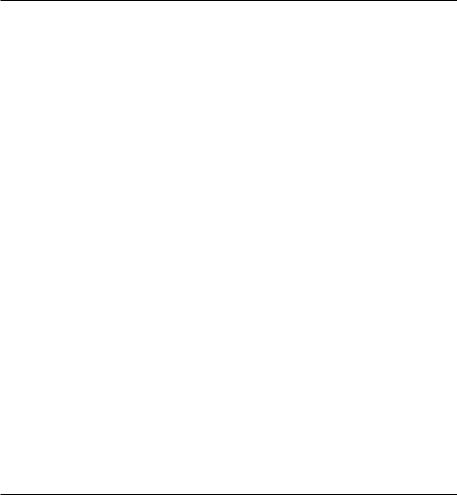
Chapter 10
Cross-Entropy, Reversed Cross-Entropy, and Symmetric Divergence Similarity Measures for 3D Image Registration: A Comparative Study
Yang-Ming Zhu1 and Steven M. Cochoff 1
10.1 Introduction
Image registration is a process that quantitatively relates the information in one image to that in another image by determining a one-to-one transformation between coordinates in the two image spaces. Medical image registration is becoming increasingly useful in research and patient care (see [1, 2, 3]). Different imaging modalities often times provide unique and complementary information. Multimodality image registration makes it possible to combine structural (computed tomography or magnetic resonance images) and functional (positron emission tomography or single photon emission tomography) information to improve diagnostic accuracy and aid surgical and/or radiotherapeutic planning. Registration of the same modality images acquired at different times allows clinicians to assess lesion progression/regression or treatment effectiveness. In an interactive, image-guided surgery environment, registration of preoperative images with the physical space is an overriding requirement.
Many algorithms have been employed to register medical images and have recently been reviewed and classified (see [4, 5]). Earlier work prior to 1993 has also been reviewed (see [6, 7]). Fitzpatrick and his colleagues evaluated 16 of
1 PET Engineering, Nuclear Medicine Division, Philips Medical Systems, 595 Miner Road,
Cleveland, Ohio 44143, USA
393
394 |
Zhu and Cochoff |
those algorithms visually as well as objectively [8, 9, 10]. Among those different registration algorithms, the voxel similarity approaches to image registration have attracted significant attention since these full-volume-based registration algorithms don’t rely upon data reduction or segmentation, and involve little or no user interaction. They can also be fully automated and offer quantitative assessment. Maintz et al. [4] lists the reported paradigms and Studholme et al.
[11], Penney et al. [12], and Holden et al. [13] compare many of them. Among various different similarity measures, mutual information is the most prominent (see [14, 15, 16], and [17]). Many papers and reports have been published on this similarity measure since its first publication and advances in this area have been recently reviewed in Pluim et al. [17].
A cross-entropy optimization approach to image registration was reported recently in Zhu [18]. Cross-entropy minimization as a principle was formally established by Shore and Johnson [19, 20]. They also studied the properties of cross-entropy minimization [21]. In addition to image registration, this measure has been applied to the areas of spectral analysis in Shore [22], image reconstruction in Zhuang et al. [23], biochemistry in Yee [24], process control in Alwan et al.
[25], non-linear programming in Das et al. [26], and electron density estimation in Antolin et al. [27], among many others.
Cross-entropy, also known as Kullback-Leibler divergence, is an informationtheoretic measure that quantifies the difference between two probability density functions (pdf). It can be either maximized or minimized, depending on how a priori pdf is given. Cross-entropy maximization degenerates to mutual information maximization, conditional entropy minimization or joint entropy minimization under certain conditions. Cross-entropy has two close relatives known as reversed cross-entropy and symmetric divergence, which have been applied to spectral analysis (see [28, 29]) and neural networks [30]. It is reported that cross-entropy, reversed cross-entropy, and symmetric divergence spectral analyses have comparable performance. However, it is not clear how reversed cross-entropy and symmetric divergence perform as registration measures. This chapter explores their use as similarity measures for medical image registration and compares their performance.
Since 1999, the imaging vendors have been developing new imaging devices which combine two different imaging modalities into a single apparatus [31]. General Electric Medical Systems, Philips Medical Systems, and Siemens Medical Solutions/CTI all have released PET/CT devices (Discovery LS, Biograph, and
Cross-Entropy, Reversed Cross-Entropy, and Symmetric Divergence |
395 |
Gemini, respectively, [32]. Given this hardware approach to image registration, the question arises as to the continued need for software registration techniques. It is our opinion however, that software image registration will continue to play a vital role in many instances and that the development of registration algorithms shall remain an important research area for years to come. In many cases, hardware registration is impractical or impossible and one must rely on software-based registration techniques. For example, when monitoring treatment effectiveness over time, software image registration is necessary since the single or multimodality images are acquired at different times. In addition, applications involving intersubject or atlas comparisons require software registration since the images originate from different subjects. Other applications for software registration include the correction of motion that occurs between sequential transmission and emission scans in PET and SPECT as well as the positioning of patients with respect to previously determined treatment plans. The need to offer multiple different combinations of imaging modalities (i.e., PET/MR, SPECT/MR, PET/CT, etc.) would be impractical. As most researchers agree, the hybrid devices will likely play a major role primarily in radiation oncology.
The remainder of this chapter is organized as following: section 10.2 defines all three measures and discusses how they can be used in an image registration context. Section 10.3 addresses the implementation issues. Section 10.4 details the experimental setup to test cross-entropy, reversed cross-entropy, and symmetric divergence image registration by both maximization and minimization. Section 10.5 presents the registration results, along with a discussion. Section 10.6 concludes with a brief summary.
10.2Cross-Entropy, Reversed Cross-Entropy, and Symmetric Divergence
Cross-entropy, reversed cross-entropy, and symmetric divergence can be defined for pdfs of any-dimensional random variables. To make it relevant to the image registration context, in the following equations only a vector variable (u, v) is considered. In these equations, u and v are voxel gray values at corresponding points in two images, f (x, y, z) and g(x, y, z) that are known as the reference image and the floating image, respectively. This gray value pair is considered in

396 |
Zhu and Cochoff |
almost all similarity measure-based image registration. Note that, if there are more than two images to be registered (see [17, 33]), these definitions can be easily extended to the n-dimensional case [18].
Assume the joint pdf of random variable (u, v) is p(u, v). Also assume a prior estimation of p(u, v) is available and denoted as q(u, v). The cross-entropy is
thus defined on a compact support D = Du × Dv , as in Shore and Johnson [19]
ηCE( p, q) = (10.1)
D q(u, v)
where Du and Dv are supports of u and v, respectively.
If the roles of q(u, v) and p(u, v) are switched, one has the reversed cross-
entropy, |
|
||
ηRCE( p, q) = D q(u, v) log |
q(u, v) |
(10.2) |
|
|
du dv. |
||
p(u, v) |
To make the definition symmetric with regard to p(u, v) and q(u, v), one can
combine cross-entropy and reversed cross-entropy, |
|
||
ηSD( p, q) = D [ p(u, v) − q(u, v)] log |
p(u, v) |
(10.3) |
|
|
du dv, |
||
q(u, v) |
which is the definition of symmetric divergence.
In the case where cross-entropy optimization is utilized to perform image registration, if a favorable (also known as desirable or likely) priori pdf is given, an estimate of the true pdf can be found by minimizing the cross-entropy. On the other hand, if an unfavorable priori pdf is given, an estimate of the true pdf can be obtained by maximizing the cross-entropy. The same would be true when reversed cross-entropy and symmetric divergence are used as similarity measures for image registration.
A favorable pdf can be computed based on previous registration results [34]. Theoretical analysis can also provide information regarding a favorable priori. For example, the voxel values in images of the same modality and of the same patient are linearly related and it has been shown that MR image can be used to simulate a PET image [35]. Since the true pdf is expected to be close to a likely priori, the cross-entropy, reversed cross-entropy, and symmetric divergence will be minimized. It is worthy to note that the cross-entropy, reversed cross-entropy, and symmetric divergence are not the only frameworks to exploit the priori knowledge in registration. The recently proposed likelihood maximization approach can also systematically use this knowledge (see [34, 36]).
Cross-Entropy, Reversed Cross-Entropy, and Symmetric Divergence |
397 |
When an undesirable prior q(u, v) is given, the cross-entropy, reversed crossentropy, or symmetric divergence are maximized. When images are in registration, we would expect that the voxel values at the same coordinates in the two images are related. Therefore, unfavorable pdfs can be uniform, proportional to one of the marginal pdfs, or the product of two marginal pdfs. The case where the unfavorable pdf is the product of two marginal pdfs is worth noting. In this case, the voxel values are statistically independent since their joint pdf is the product of their marginal pdfs. Cross-entropy maximization based on these three priori estimates degenerate to entropy minimization, conditional entropy
minimization, and mutual information maximization, respectively, [18].
Let’s assume the marginal pdfs of u and v are h f (u) and hg (v), respectively, and the unfavorable priori pdf q(u, v) is equal to the product of two marginal
pdfs. Putting q = h f (u)hg (v) into Eqs. (10.1) (10.2) and (10.3), one arrives at
ηCE = D p(u, v) log |
p(u, v) |
|
|
(10.4) |
||||
|
|
|
du dv, |
|||||
h f (u)hg (v) |
||||||||
ηRCE = D h f (u)hg (v) log |
h |
(u)hg (v) |
|
|
|
|||
|
|
f |
du dv, |
(10.5) |
||||
|
|
p(u, v) |
||||||
and |
|
|
|
|
|
|||
ηSD = D [ p(u, v) − h f (u)hg (v)] log |
|
p(u, v) |
|
du dv. |
(10.6) |
|||
|
||||||||
h f (u)hg (v) |
One may notice that Eq. (10.4) is the definition of mutual information. The measures defined in Eqs. (10.4–10.6) are maximzied when used in image registration.
10.3 Implementation
Figure 10.1 shows the overall flow chart of the registration process. It applies to all three similarity measures as well as the minimization and maximization cases. When the similarity measures are minimized, a favorable priori is used in the similarity calculation. When they are maximized, the priori estimate is calculated from the marginal pdfs on-the-fly. As can be seen, no matter which measure is used, the registration algorithm has the same structure and they can be implemented in the same manner. Since Eqs. (10.1)–(10.6) are in a continuous form, they must be discretized to be solved numerically.
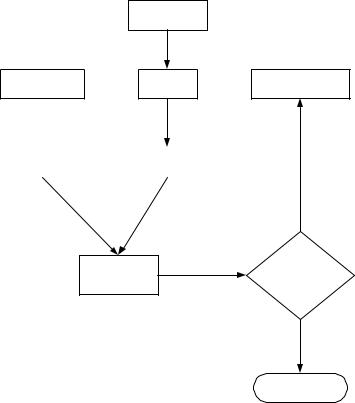
398 |
Zhu and Cochoff |
Floating Image
Initial Transform Transform
Update Transform
Reference Image |
|
Transformed Floating |
|
|
|
No
Compute
CE/RCE/SD
CE/RCE/SD
Optimal (min/max)?
Yes
Final Transform
Figure 10.1: Flow chart of image registration by the cross-entropy, reversed cross-entropy, and symmetric divergence optimization. CE: cross-entropy; RCE: reversed cross-entropy; SD: symmetric divergence.
The two images involved in the registration are identified as the reference image and the floating image. The floating image will undergo rotation and translation to match the reference image. Before the automatic registration starts, an initial set of registration parameters must be set and the floating image is appropriately transformed (i.e., rotated and translated). The similarity measures between the reference image and the transformed floating image are then computed. If the similarity number is not optimal, the registration parameters are updated, otherwise, the registration process stops and the optimal registration parameters are output. The scheme to update the registration parameters is determined by the optimization algorithm employed. In the following subsections, the key steps in the registration process are expanded and discussed in detail.
Cross-Entropy, Reversed Cross-Entropy, and Symmetric Divergence |
399 |
10.3.1 Transformation
Although all three registration measures can be applied to more general transformations, we restricted the transformation to rigid-body transformations. For the rigid-body registration, the registration parameter is a six-dimensional vector, (θx, θy, θz, tx, ty, tz), where θx, θy, and θz are rotation angles in degrees around the x-, y-, and z-axis, respectively, and tx, ty, and tz are translation offsets along the x-, y-, and z-axis, respectively.
There is a 4 × 4 matrix corresponding to any of the three rotations in a homogeneous coordinate system [37]. Since a successive application of the rotations amounts to matrix multiplication which is not commutative, the order of these rotations is important. It is assumed in this chapter that the rotation angles are applied around the x-, y-, and z-axis, in that order, and that the rotation is performed before the translation.
10.3.2Marginal, Joint, and Priori Probability Density Functions
The marginal and joint pdfs of gray value pairs were estimated from normalized histograms. The maximum voxel value of image f was found first. The voxel values in image f were then divided into 64 discrete levels. Similarly, the voxel values in image g were divided into 64 discrete values. The size of the joint histogram is thus 64 × 64. Alternatively, one can change the bin size adaptively [18]. In the overlapping volume, the histograms of voxel values in images f and g, and of the voxel pairs were calculated by binning the voxel values and value pairs. The normalized histograms then give estimations of the marginal as well as the joint distributions. To compute the cross-entropy, reversed cross-entropy, and symmetric divergence, these estimated pdfs can be directly substituted into the formulas defined in Eqs. (10.1)–(10.6).
When a favorable priori estimate is employed in the registration, it can be computed in the same fashion as that for the joint pdf. The priori estimate shall be calculated with the same size of histograms as that for the joint histogram.
10.3.3 Interpolation
A grid point in one volume will generally not exactly coincide with another grid point in the transformed space. Since the voxel values of the reference