
Kluwer - Handbook of Biomedical Image Analysis Vol
.3.pdf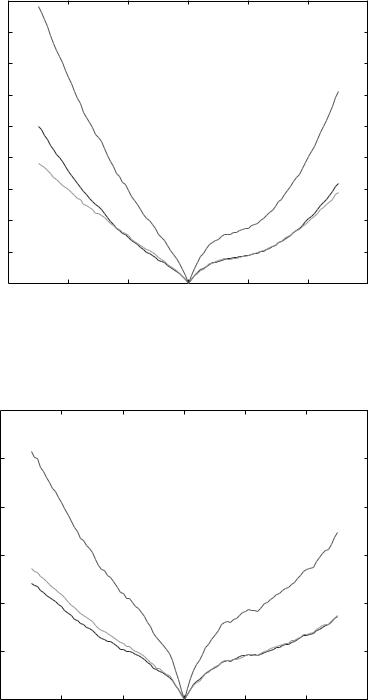
|
0.09 |
|
|
|
|
|
|
|
|
SD |
|
|
|
|
|
|
0.08 |
|
|
|
|
|
|
|
0.07 |
|
|
|
|
|
|
|
0.06 |
|
|
|
|
|
SD |
|
|
|
|
|
|
|
|
Values |
0.05 |
CE |
|
|
|
|
|
|
|
|
|
|
|
|
|
Similarity |
0.04 |
RCE |
|
|
|
|
|
|
|
|
|
|
|
||
|
|
|
|
|
|
CE |
|
|
|
|
|
|
|
|
|
|
0.03 |
|
|
|
|
|
RCE |
|
|
|
|
|
|
|
|
|
0.02 |
|
|
|
|
|
|
|
0.01 |
|
|
|
|
|
|
|
0 |
−10 |
−5 |
0 |
5 |
10 |
15 |
|
−15 |
||||||
|
|
|
|
Y Rotation (degrees) |
|
|
|
Figure 10.12: The cross-entropy (CE), reversed cross-entropy (RCE), and symmetric divergence (SD) profiles (with a likely priori) of an MR/Em image pair in the vicinity of a manual registration when the y-rotation changes independently.
|
0.06 |
|
|
|
|
|
|
|
0.05 |
SD |
|
|
|
|
|
|
|
|
|
|
|
|
|
|
0.04 |
|
|
|
|
|
|
Values |
|
|
|
|
|
|
SD |
|
|
|
|
|
|
|
|
Similarity |
0.03 |
|
|
|
|
|
|
|
RCE |
|
|
|
|
|
|
|
CE |
|
|
|
|
|
|
|
|
|
|
|
|
|
|
|
0.02 |
|
|
|
|
|
CE |
|
|
|
|
|
|
|
|
|
|
|
|
|
|
|
RCE |
|
0.01 |
|
|
|
|
|
|
|
0 |
−10 |
−5 |
0 |
5 |
10 |
15 |
|
−15 |
||||||
|
|
|
|
Z Rotation (degrees) |
|
|
|
Figure 10.13: The cross-entropy (CE), reversed cross-entropy (RCE), and symmetric divergence (SD) profiles (with a likely priori) of an MR/Em image pair in the vicinity of a manual registration when the z-rotation changes independently.
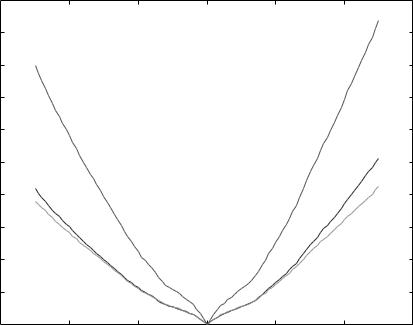
Cross-Entropy, Reversed Cross-Entropy, and Symmetric Divergence |
421 |
|
0.2 |
|
|
|
|
|
|
|
|
|
|
|
|
|
SD |
|
0.18 |
|
|
|
|
|
|
|
0.16 |
SD |
|
|
|
|
|
|
0.14 |
|
|
|
|
|
|
Values |
0.12 |
|
|
|
|
|
|
|
|
|
|
|
|
CE |
|
Similarity |
0.1 |
|
|
|
|
|
|
0.08 |
CE |
|
|
|
|
RCE |
|
|
|
|
|
|
|
|
|
|
|
RCE |
|
|
|
|
|
|
0.06 |
|
|
|
|
|
|
|
0.04 |
|
|
|
|
|
|
|
0.02 |
|
|
|
|
|
|
|
0 |
−10 |
−5 |
0 |
5 |
10 |
15 |
|
−15 |
||||||
|
|
|
|
X Translation (mm) |
|
|
|
Figure 10.14: The cross-entropy (CE), reversed cross-entropy (RCE), and symmetric divergence (SD) profiles (with a likely priori) of an MR/Em image pair in the vicinity of a manual registration when the x-translation changes independently.
substituted pdfs in the calculation. The small positive pdfs can be calculated in the same fashion as discussed in sections 10.3.6 and 10.5.1.4. Figures 10.17 and 10.18 show the cross-entropy profiles as the x-rotation angle and x-translation offset change independently. The profiles are labeled in the same manner as for the reversed cross-entropy maximization case. As those two figures reveal, all of the profiles have a similar shape. The only apparent difference is the value of the cross-entropy. Since the size of the basin around the optimal value indicates the size of the capture range, it seems that either using a small α or ignoring the zero pdf terms is preferred. We elected to ignore the zero pdf terms since the effect of the small α on the summation of all priori pdfs is hard to quantify (the sum has an upper bound of 1 + α1 ). This strategy worked fine in our experiment.
In the reversed cross-entropy minimization, the calculation is not stable when the joint pdfs are zero. Figures 10.19 and 10.20 show the reversed cross-entropy profiles when the x-rotation angle and the x-translation change independently
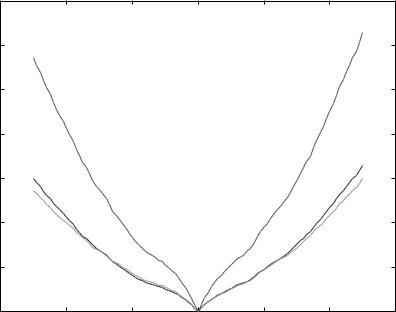
422 |
Zhu and Cochoff |
|
0.14 |
|
|
|
|
|
|
|
|
|
|
|
|
|
|
|
SD |
|
0.12 |
|
|
|
|
|
|
|
|
|
SD |
|
|
|
|
|
|
|
0.1 |
|
|
|
|
|
|
|
Values |
0.08 |
|
|
|
|
|
|
|
|
|
|
|
|
|
|
|
|
Similarity |
|
|
|
|
|
|
|
CE |
0.06 |
CE |
|
|
|
|
|
RCE |
|
RCE |
|
|
|
|
|
|||
|
|
|
|
|
|
|
||
|
|
|
|
|
|
|
|
|
|
0.04 |
|
|
|
|
|
|
|
|
0.02 |
|
|
|
|
|
|
|
|
0 |
|
−10 |
−5 |
0 |
5 |
10 |
15 |
|
−15 |
|||||||
|
|
|
|
|
Y Translation (mm) |
|
|
|
Figure 10.15: The cross-entropy (CE), reversed cross-entropy (RCE), and symmetric divergence (SD) profiles (with a likely priori) of an MR/Em image pair in the vicinity of a manual registration when the y-translation changes independently.
and different strategies to handle the zero joint pdfs are compared. The profiles are labeled in the same manner as was used for the cross-entropy minimization case. All of the resultant profiles are similar. Again, we elected to ignore the zero pdf terms since the corresponding profiles are relatively smooth and worked well in practice.
Since the symmetric divergence measure has cross-entropy and reversed cross-entropy components, the issues with numerical stability were addressed in a similar manner.
10.6 Concluding Remarks
We have applied the information-theoretic measures cross-entropy, reversed cross-entropy, and symmetric divergence to retrospective three-dimensional
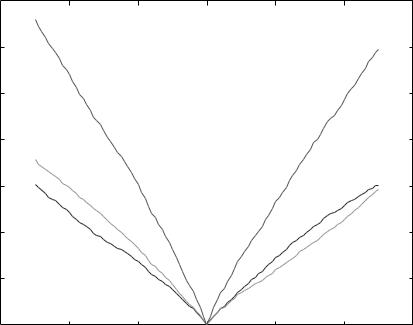
Cross-Entropy, Reversed Cross-Entropy, and Symmetric Divergence |
423 |
|
0.14 |
|
|
|
|
|
|
|
|
|
SD |
|
|
|
|
|
|
|
0.12 |
|
|
|
|
|
|
SD |
|
|
|
|
|
|
|
|
|
|
0.1 |
|
|
|
|
|
|
|
Values |
0.08 |
|
|
|
|
|
|
|
|
RCE |
|
|
|
|
|
|
|
Similarity |
|
|
|
|
|
|
|
|
0.06 |
CE |
|
|
|
|
|
CE |
|
|
|
|
|
|
|
RCE |
||
|
|
|
|
|
|
|
||
|
|
|
|
|
|
|
|
|
|
0.04 |
|
|
|
|
|
|
|
|
0.02 |
|
|
|
|
|
|
|
|
0 |
|
−10 |
−5 |
0 |
5 |
10 |
15 |
|
−15 |
|||||||
|
|
|
|
|
Z Translation (mm) |
|
|
|
Figure 10.16: The cross-entropy (CE), reversed cross-entropy (RCE), and symmetric divergence (SD) profiles (with a likely priori) of an MR/Em image pair in the vicinity of a manual registration when the z-translation changes independently.
image registration, which quantify the difference between the true joint distribution and its prior estimation of the voxel value pair in two images at corresponding points. The experimental results indicate that all three measures are suitable as registration criteria.
The cross-entropy, reversed cross-entropy, and symmetric divergence can be maximized and minimized, which is an unusual property for similarity measures. Almost all other similarity measures can be either maximized or minimized, but not both. If a likely priori pdf is given, we want our estimated pdf at registration to be as close to the priori as possible. Therefore, the similarity measures are minimized. If an unlikely priori pdf is given, we want our estimated pdf at registration to be different from the priori as much as possible. Thus, those similarity measures are maximized. There are many unlikely priori pdf. This chapter only considered the most popular one, i.e., the voxel values in two images at
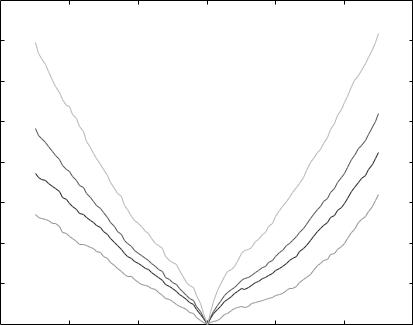
424 |
|
|
|
|
|
Zhu and Cochoff |
||
|
0.08 |
|
|
|
|
|
|
|
|
0.07 |
10000 |
|
|
|
|
|
10000 |
|
|
|
|
|
|
|
||
|
0.06 |
|
|
|
|
|
|
|
|
|
|
|
|
|
|
|
100 |
|
0.05 |
100 |
|
|
|
|
|
|
Cross–Entropy |
|
|
|
|
|
|
|
|
|
|
|
|
|
|
|
ignored |
|
0.04 |
ignored |
|
|
|
|
|
|
|
|
|
|
|
|
|
|
||
|
|
|
|
|
|
|
1 |
|
|
|
|
|
|
|
|
|
|
|
0.03 |
|
|
|
|
|
|
|
|
|
1 |
|
|
|
|
|
|
|
0.02 |
|
|
|
|
|
|
|
|
0.01 |
|
|
|
|
|
|
|
|
0 |
|
−10 |
−5 |
0 |
5 |
10 |
15 |
|
−15 |
|||||||
|
|
|
|
|
X Rotation (degrees) |
|
|
|
Figure 10.17: The cross-entropy profiles of an MR/Em image pair in the vicinity of a manual registration when the x-rotation angle changes independently. The numbers around the profiles indicate the α value used to compute a small probability value which is used to replace zero pdf. The profile that is labelled “ignored” represents the case where the contribution from zero joint pdfs was ignored in the profile computation.
registration are independent of each other. This priori joint pdf is not favorable since the voxel values in two different images reflect the physical properties of the same object and thus they are most likely related in some way, at least from a statistical point of view. If more than one likely and unlikely priori pdf is available, one can define cross-entropy, reversed cross-entropy, and symmetric divergence for them and maximize or minimize them collectively, where a multiobjective optimization approach would be useful, as already illustrated in Zhu [18].
The overall flow chart for the three similarity measures as applied to image registration is presented for both maximization and minimization cases. It shall be noted that this flow chart is also consistent with those of most
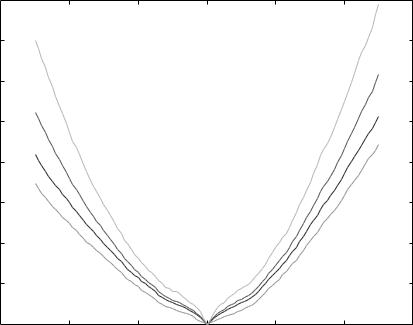
Cross-Entropy, Reversed Cross-Entropy, and Symmetric Divergence |
425 |
|
0.16 |
|
|
|
|
|
|
|
|
|
|
|
|
|
|
|
10000 |
|
0.14 |
10000 |
|
|
|
|
|
|
|
0.12 |
|
|
|
|
|
|
100 |
|
|
|
|
|
|
|
|
|
|
|
ignored |
|
|
|
|
|
ignored |
|
|
|
|
|
|
|
|
|
|
0.1 |
|
|
|
|
|
|
|
Cross–Entropy |
|
100 |
|
|
|
|
|
1 |
0.08 |
|
|
|
|
|
|
||
|
|
|
|
|
|
|
||
|
1 |
|
|
|
|
|
|
|
|
|
|
|
|
|
|
|
|
|
0.06 |
|
|
|
|
|
|
|
|
0.04 |
|
|
|
|
|
|
|
|
0.02 |
|
|
|
|
|
|
|
|
0 |
|
−10 |
−5 |
0 |
5 |
10 |
15 |
|
−15 |
|||||||
|
|
|
|
|
X Translation (mm) |
|
|
|
Figure 10.18: The cross-entropy profiles of an MR/Em image pair in the vicinity of a manual registration when the x-translation changes independently. The numbers around the profiles indicate the α value used to compute a small probability value which is used to replace zero pdf. Ignored means that those pdfs are ignored when computing the profile.
similarity-based registration techniques. Therefore, incorporating those measures to existed packages would be straightforward.
The major problem with applying cross-entropy, reversed cross-entropy, and symmetric divergence optimziation to image registration is the numerical stability when the estimated joint pdf or priori joint pdf is zero. If a large sample of successsful registrations are used to generate the desirable, statistically averaged pdf, it is unlikely that one would have many zero points in the priori joint pdf. Alternatively, one can assume a parametric form of the priori joint pdf. The unknown parameters can be estimated from available priori pdf data points and the pdf can be interpolated at the zero points. Unfortunately, there is no justification for one parametric form over others, particularly in the multimodality cases. To improve its accuracy and robustness, one can also use Paren
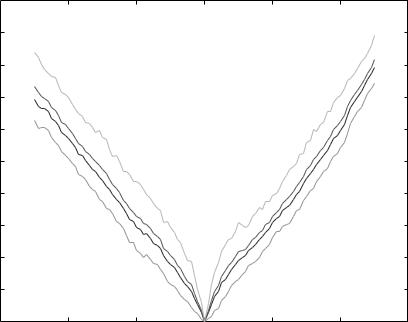
426 |
Zhu and Cochoff |
|
0.05 |
|
|
|
|
|
|
|
|
0.045 |
|
|
|
|
|
|
10000 |
|
|
10000 |
|
|
|
|
|
100 |
|
0.04 |
|
|
|
|
|
|
|
|
|
|
|
|
|
|
ignored |
|
|
0.035 |
100 |
|
|
|
|
|
1 |
|
ignored |
|
|
|
|
|
|
|
Cross–Entropy |
0.03 |
1 |
|
|
|
|
|
|
|
|
|
|
|
|
|
||
0.025 |
|
|
|
|
|
|
|
|
|
|
|
|
|
|
|
|
|
Reversed |
0.02 |
|
|
|
|
|
|
|
|
|
|
|
|
|
|
|
|
|
0.015 |
|
|
|
|
|
|
|
|
0.01 |
|
|
|
|
|
|
|
|
0.005 |
|
|
|
|
|
|
|
|
0 |
|
−10 |
−5 |
0 |
5 |
10 |
15 |
|
−15 |
|||||||
|
|
|
|
|
X Rotation (degrees) |
|
|
|
Figure 10.19: The reversed cross-entropy profiles of an MR/Em image pair in the vicinity of a manual registration when the x-rotation angle changes independently. The numbers around the profiles indicate the α value used to compute a small probability value which is used to replace zero pdf. Ignored means that those pdfs are ignored when computing the profile.
density estimation with a Gaussian kernel to add smoothness and stability to the similarity functions, as in Wells et al. [14]. Nevertheless, the simple, heuristic approach we used here proves to be effective. The main motivation for this heuristic approach is to make the similarity measure profiles smooth and to have a maximum (peak) or minimum (valley) around the unknown truth with a reasonble capture range. Both of these conditions are necessary for good registration measures. Moreover, it is important that the modified priori or estimated joint pdf do not deviate from a real pdf. That is, the sum of the modified pdfs shall still be close to 1 and the difference from the original values shall not be significant. To further improve the performance of these similarity measures, one can employ different interpolation methods. It was reported recently that different
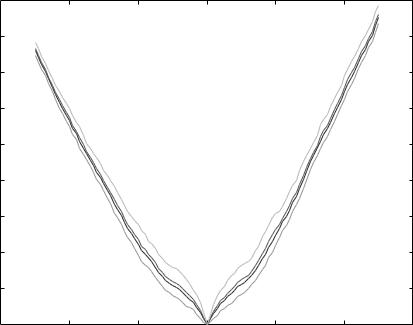
Cross-Entropy, Reversed Cross-Entropy, and Symmetric Divergence |
427 |
|
0.09 |
|
|
|
|
|
|
10000 |
|
|
|
|
|
|
|
|
|
|
|
|
|
|
|
|
|
100 |
|
|
10000 |
|
|
|
|
|
ignored |
|
0.08 |
|
|
|
|
|
1 |
|
|
|
100 |
|
|
|
|
|
|
|
|
ignored |
|
|
|
|
|
|
|
0.07 |
1 |
|
|
|
|
|
|
|
|
|
|
|
|
|
|
|
Cross–Entropy |
0.06 |
|
|
|
|
|
|
|
0.05 |
|
|
|
|
|
|
|
|
|
|
|
|
|
|
|
|
|
Reversed |
0.04 |
|
|
|
|
|
|
|
0.03 |
|
|
|
|
|
|
|
|
|
|
|
|
|
|
|
|
|
|
0.02 |
|
|
|
|
|
|
|
|
0.01 |
|
|
|
|
|
|
|
|
0 |
|
−10 |
−5 |
0 |
5 |
10 |
15 |
|
−15 |
|||||||
|
|
|
|
|
X Translation (mm) |
|
|
|
Figure 10.20: The reversed cross-entropy profiles of an MR/Em image pair in the vicinity of a manual registration when the x-translation changes independently. The numbers around the profiles indicate the α value used to compute a small probability value which is used to replace zero pdf. Ignored means that those pdfs are ignored when computing the profile.
interpolation methods can attribute to the different levels of smoothness of the similarity measures (see [16]). A smooth similarity function is desirable since the spurious peaks and valleys can trap the optimization to undesirable local optima.
Acknowledgements
The image files were provided by Dr. M. Hartshorne. Thanks are also due to B. Jones, D. Maniawski, Z. Mercer, and C. Nortmann who manually registered the MR/SPECT image pair used in the similarity minimization study.
428 |
Zhu and Cochoff |
Questions
1.What is image registration?
2.Why is the algorithm-based registration still required given the success of hybrid imaging devices?
3.What is cross-entropy? What is reversed cross-entropy? What is symmetric divergence?
4.Why the cross-entropy is a generalized similarity measure for image registration?
5.When is cross-entropy (reversed cross-entropy, symmetric divergence) maximized? When is it minimized?
6.How can one get a favorable priori pdf?
7.What are common unfavorable priori pdf’s?
8.What are the general steps for a similarity-based registration?
9.Why does cross-entropy, reversed cross-entropy, and symmetric divergence based registration suffer from numerical instability? How to deal with it?
10.What are the requirements for a good similarity measure?

Cross-Entropy, Reversed Cross-Entropy, and Symmetric Divergence |
429 |
Bibliography
[1]Vera, P., Kaminska, A., Cieuta, C., Hollo, A., Stievenart, J.L., Gardin, I., Ville, D., Mangin, J.F., Plouin, P., Dulac, O. and Chiron, C., Use of subtraction Ictal SPECT co-registration to MRI for optimizing the localization of seizure foci in children, Journal of Nuclear Medicine, Vol. 40, pp. 786–792, 1999.
[2]Brinkmann, B.H., O’Brien, T.J., Aharon, S., O’Connor, M.K. and Mullan, B.P., Quantitative and clinical analysis of SPECT image registration for epilepsy studies, Journal of Nuclear Medicine, Vol. 40, pp. 1098–1105, 1999.
[3]Pfluger, T., Vollmar, C., Widmuller, A., Dresel, S., Berger, F., Suntheim, P., Leinsinger, G. and Kahn, K., Quantitative comparison of automatic and interactive methods for MRI-SPECT image registration of the brain based on three-dimensional calculation of error, Journal of Nuclear Medicine, Vol. 41, pp. 1823–1829, 2000.
[4]Maintz, J.B.A. and Viergever, M.A., A survey of medical image registration, Medical Image Analysis, Vol. 2, No. 1, pp. 1–36, 1998.
[5]Hajnal, J.V., Hill, D.L.G. and Hawkes, D.J., eds., Medical Image Registration, CRC Press, Boca Raton, FL: 2001.
[6]Van den Elsen, P.A. and Viergever, M.A., Medical image matching—a review with classification, IEEE Engineering Medical Biology Vol. 12, pp. 26–39, March 1993.
[7]Maurer, C.R., Jr. and Fitzpatrick, J.M., A review of medical image registration, In: Interactive Image-guided Neurosurgery, Maciunas, R.J. ed., American Association Neurological Surgeons, Parkridge, IL, pp. 17–44, 1993.
[8]West, J., Fitzpatrick, J.M., Wang, M.Y., Dawant, B.M., Maurer, C.R., Jr., Kessler, R.M., Maciunas, R.J., Barillot, C., Lemoine, D., Collignon, A., Maes, F., Suetens, P., Vandermeulen, D., Van den Elsen, P.A., Napel, S., Sumanaweera, T.S., Harkness, B., Hemler, P.F., Hill, D.L.G., Hawkes, D.J., Studholme, C., Maintz, J.B.A., Viergever, M.A., Malaandain,