
Engineering and Manufacturing for Biotechnology - Marcel Hofman & Philippe Thonart
.pdf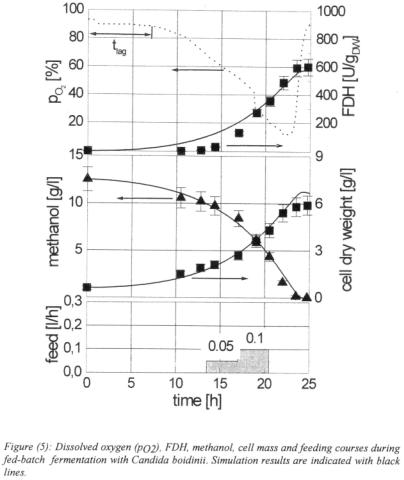
R. Takors, D. Weuster-Botz, W. Wiechert, C.
However a generally good agreement between measured and simulated values could be stated. This allows the conclusion that (at least for Candida boidinii) kinetic models derived from steady-state experiments could also be used to describe non steady-state fermentations if the “right” model is identified by model discrimination and (if necessary) model extensions are considered.
4.3 MODEL DISCRIMINATING DESIGN WITH ZYMOMONAS MOBILIS
As pointed out in section 2 the model discriminating approach could also be applied for model discriminating design. As an example simulations with the anaerobic bacterium Zymomonas mobilis were carried out using the following model (and corresponding parameters) as a basis for microbial kinetic simulations (Weuster-Botz, 1993).
122
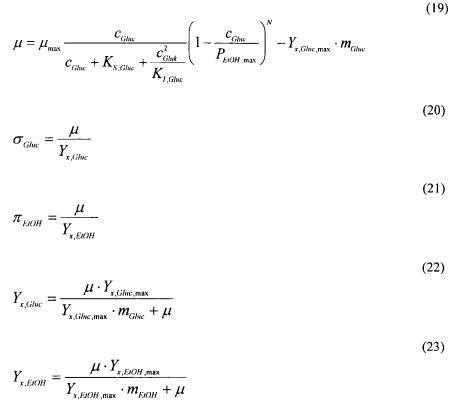
A model discrimination approach for data analysis and experimental design
Glucose is assumed to be the only carbon source and ethanol the fermentation product. High ethanol concentrations cause growth inhibitions as indicated by Levenspiel (1980). Measurements suggested by model discrimination design were simulated using this modelling approach in combination with balancing equations and a randomised “measurement” noise of 10%. Furthermore a set of competing models was defined consisting of different growth modelling approaches. Product inhibition was described using the approach of Jerusalimski and Engamberidiev (1969) and glucose effects were assumed to be saturating (Monod-type) or even growth inhibiting (Andrews-type).
Table (2) gives an overview about the set of competing models.
Simulations were started with the assumption that no experimental information should be available and all models possess equal model probabilities. Thus at the beginning model predictive design was used, based on model start parameters as indicated in figure (6). After 7 simulated experiments model predictive design was replaced by model discrimination design. In total 12 experiments were simulated. They are presented in figure (7). Additionally an overview of model probability development is given in figure (8).
123
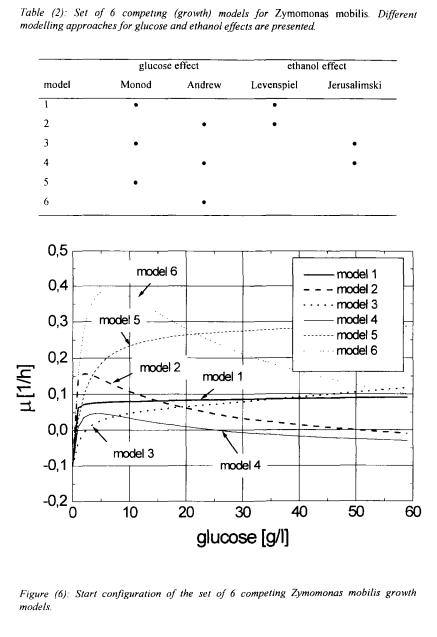
R. Takors, D. Weuster-Botz, W. Wiechert, C.
124
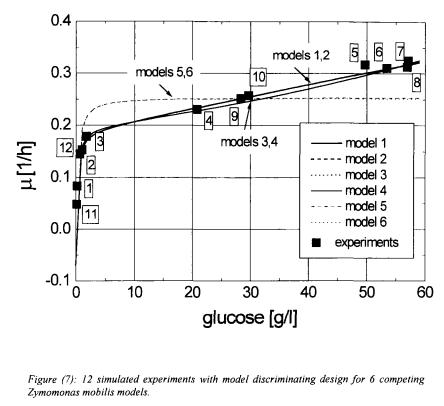
A model discrimination approach for data analysis and experimental design
After simulation of 7 experiments with model predictive design models (5) and (6) were identified as inappropriate modelling approaches (figure (8)). As a conclusion growth inhibition caused by ethanol must not be neglected for modelling. However no clear model discrimination was achieved with respect to models (l)-(4). This changed after the following three simulated experiments because a glucose inhibiting effect could not be detected (of the reference model was 913 g/l). Thence model probabilities of models (2) and (4) decreased down to 0%. Simulations (11) and (12) were aimed to discriminate the right product inhibition modelling approach. As most significant model discrepancies are observable at low growth rates, corresponding experimental designs were suggested (figure (7)). Finally the appropriate model could be discriminated with approximately 100% model probability
125
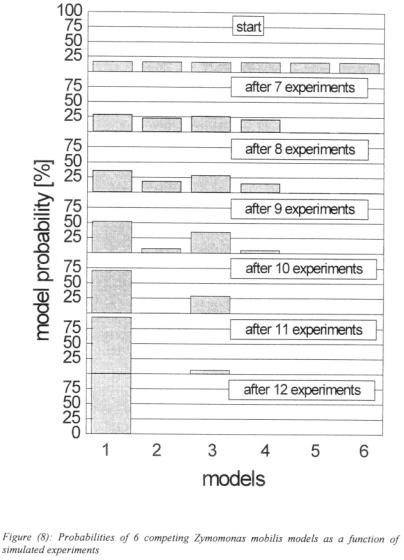
R. Takors, D. Weuster-Botz, W. Wiechert, C.
5. Conclusions
A general model discriminating strategy is presented that enables model discrimination based on already available experimental data as well as a model discriminating design. The methodology is generally applicable without limitation concerning model complexity, the number of model parameters or the number of competing models. As a
126
A model discrimination approach for data analysis and experimental design
result model probabilities are calculated that represent an expressive value for the suitability of a model.
As model variances are used for calculation, model discrimination based on entropy formulations could only be used if a non-singularity of the Fisher-information matrix is prevented. Thus sufficient experimental information for model parameter regression must be available. As a consequence a simplified model design approach is proposed to overcome this start problem.
Results of experimental data analysis using Candida boidinii steady-state fermentations show that the entropy based discrimination approach is well suited to discriminate an appropriate model among a set of competing models. Simulation results with competing Zymomonas mobilis models proof that the extended model discriminating design strategy could be used even under poor information start conditions. Furthermore it has been shown that the Candida boidinii model which was discriminated by steady-state fermentation results could also be used to describe batch and fed-batch fermentations.
References
Baltes, M.; Schneider, R.; Sturm, C.; Reuss, M. (1994) Optimal Experimental Design for Parameter Estimation in Unstructured Growth Models, Biolechnol. Prog. 10, 480-488.
Box, G.E.P. and Hill, W.J. (1967) Discrimination among mechanistic models, Technometrics 9, 57-71.
Cooney, M.J. and McDonald, K.A. (1995) Optimal dynamic experiments for bioreactor model discrimination, Appl. Microbiol. Bioelchnol. 43, 826-837.
Ejiofor, A.O.; Posten C.H.; Solomon B.O.; Deckwer, W.-D. (1994) A robust fed-batch feeding strategy for optimal parameter estimation for baker’s yeast production, Bioprocess Eng. 11, 135-144.
Goodwin, G.C. and Payne, R.L. (1973) Designs and characterisation of optimal test signals for linear SISO parameter estimation, 3rd IFAC Symposium on Identification and System Parameter Estimation, The Hague
Holmberg, A. (1981) On the practical identifiability of microbial growth models incorporating MichaelisMenten type non-linearities, Math. Biosci. 62, 23-43.
Jerusalimski, N.D. and Engamberidiev, N.B. (1969) Continuous Cultivation of Microorganisms, Academic Press, New York.
Levenspiel, O. (1980) The Monod equation: A requisit and generalisation to product inhibition situations,
Biotechnol. Bioeng. 22, 1671-1687.
Munack, A. (1989) Optimal feeding strategy for the identification of Monod-type models by fed-batch experiments. In: N.M. Fish (ed.) Reprints of the 4th International Congress on Computer Applications in Fermentation Technology, Ellis Horwood Ltd., Chichester.
Munack, A. (1991) Optimisation of sampling, In: Rehm and Reed (eds.) Biotechnology Vol. 4, Verlag Chemie, Weinheim, Germany.
Nihilitä, M. and Virkkunen, J. (1977) Practical identifiability of growth and substrate consumption models,
Biotechnol. Bioeng. 19, 1831-1850.
Roels, J. A.(1983) Energetics and kinetics in biotechnology, Elsevier Biomedical Press, Amsterdam. Schneider, R. and Munack A. (1995) Improvements in the on-line parameter identification of bioprocesses,
Preprints of the 6th Conference on Computer Applications in Biotchnology, Garmisch-Partenkirchen, Germany.
Takors, R. (1997) Entwicklung und Einsatz einer Versuchsplanungstechnik zur experimentellen
Makrokinetikbestimmung, Jül-Bericht 3420, Forschungszentrum Jülich GmbH, Jülich, Germany,, ISSN
0944-2952.
Takors, R.; Wiechert, W.; Weuster-Botz, D. (1997) Experimental Design for the Identification of
Macrokinetic Models and Model Discrimination, Biotechnol. and Bioeng. 56, 5, 564-576.
127
R. Takors, D. Weuster-Botz, W. Wiechert, C.
Van Impe, J.F.; Versyck, K.J.; Claes, J.E. (1997) Practical identification of unstructured growth kinetics by application of experimental design, Biotechnol. Prog. 13, 5, 524-531.
Wedy, M (1992) Reaktionskinetische und regelungstechnische Untersuchungen zur Produktion von
Formiatdehydrogenase mit Candida boidinii, Ph.D. thesis, RWTH, Aachen, Germany.
Weuster-Botz, D. (1993) Continuous ethanol production of Zymomonas mobilis in a fluidised bed reactor. Part I Kinetic Studies of immobilisation in macroporous glass beads, Appl. Microbiol. Biolechnol. 39, 679-684.
Weuster-Botz, D and Wandrey, C. (1995) Medium optimisation by genetic algorithm for continuous production of formate dehydrogenase, Process Biochemistry 30, 563-571.
Yoo, Y.J.; Marino-Galarraga, M.; Hong, J.; Hatch, R.T. (1986) Experimental design for parameter estimation from batch culture, Biotechnol. Bioeng. 28, 6, 836-841.
128
MODEL BASED SEQUENTIAL EXPERIMENTAL DESIGN FOR BIOPROCESS OPTIMISATION - AN OVERVIEW
RALPH BERKHOLZ1 AND REINHARD GUTHKE2
1BioControl Jena GmbH, 07745 Jena, Wildenbruchstr. 15, GERMANY 2 Hans Knoll Institute for Natural Products Research, 07745 Jena, Beutenbergstr. 11, GERMANY
Summary
Model based experimental design for bioprocess optimisation requires transparent, understandable, identifiable models considering the physiological states necessary to obtain high product yields. Knowledge and data based hybrid modelling techniques are suitable to build such models. Two concepts of model based experimental design called direct and indirect experimental design are established. The direct design focuses on experiments being optimal with respect to the process performance but ignoring the relevance of the parameter estimation accuracy. Contrarily the indirect design leads to precise parameter estimates, but may result in unproductive fermentation runs worthless with respect to model validation. Due to the disadvantages of these two design methods the concept of A-optimal experimental design was developed. This approach enables experimentalists to suggest experimental set-ups optimal in productivity and parameter estimation accuracy.
1. Introduction
Biotechnological processes are very complex and often poorly understood. Generally the knowledge of the underlying biochemical phenomena is incomplete. Therefore it is impossible to optimise bioprocesses on the basis of theoretical assumptions, only.
Experiments have to be performed. Due to the expensive bioprocesses it is necessary to design biotechnological experiments carefully with respect to their aim. For bioprocess optimisation a sequence of experiments has to be designed in order to achieve maximum productivity with minimum experimental effort.
In general the two basic approaches to handle this problem are the empirical experimental design and the model based experimental design. Empirical experimental design means the use of experience, intuition and process related analogies by skilled
129
M. Hofman and P. Thonart (eds.), Engineering and Manufacturing for Biotechnology, 129–141.
© 2001 Kluwer Academic Publishers. Printed in the Netherlands.
Ralph Berkholz and Reinhard Guthke
experimentalists to design experimental set-ups. Model based experimental design includes the use of mathematical models to obtain suitable suggestions for efficient experiments. Although it would be very interesting to analyse how skilled experimentalists often design nearly optimal experiments intuitively, in this overview the model based experimental design is considered only.
For model based experimental design the assumption is fundamental that the behaviour of a certain mathematical process model is similar to the process that should be optimised. A model fulfilling this assumption can be used for calculations in order to simulate the real process and to study the influence of different process strategies on the process numerically. So the model acts as a substitute for reality.
Experimental design is always a problem of optimisation concerning the convenient choice of the experimental conditions such as process regime and measurement set-up in order to maximise the efficiency of experiments with respect to the problem to be solved. For model based experimental design the optimisation of the experimental conditions will be carried out by simulations.
It is necessary to formulate a related objective function for the calculation of the efficiency of an experiment with regard to the experimental aim. During the optimisation procedure different experimental conditions will be evaluated according to the resulting value of these objective function. The experimental set-up leading to the maximum (or minimum) value of the objective function represents the optimal experimental design.
Actually there are two basic concepts established to formulate objective functions for the model based experimental design for bioprocess optimisation. The first one called direct experimental design method refers to the process productivity. The second one named indirect experimental design method focuses on the parameter estimation accuracy of the process model used. Both experimental design methods will be discussed later.
This review is organised as follows. Section 2 explains the demands on models concerning their application to experimental design and how to construct these models using knowledge and data based techniques. The two established methods of model based experimental design for bioprocess optimisation (i.e. direct and indirect experimental design method) will be discussed in the sections 3 and 4. A novel experimental design method for bioprocess optimisation called A optimal experimental design will be presented in section 5 as a conclusion of the analysis of the advantages and disadvantages of these two concepts. A short experimental example of the application of these design method will be shown in section 6.
1.Bioprocess modelling for experimental design procedures
1.1.BIOPROCESS MODELLING
Usually models for the simulation of dynamic bioprocesses consist of systems of ordinary differential equations for the overall mass balances that include kinetic expressions describing the rate limiting biochemical reactions of the considered process:
130
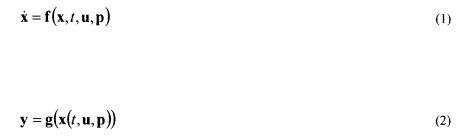
Model based sequential experimental design for bioprocess Optimisation - an overview
where x denotes the vector of state variables, u the vector of input variables and p the vector of kinetic parameters. Since the state variables are often not measurable directly it is necessary to calculate the vector of model output variables y:
Using equation (2) it is possible to compare the predicted model output y and the measured process output in order to evaluate the model performance or to calculate unknown model parameters.
Whereas it is mostly easy to formulate the overall mass balances of a biotechnological system in many cases it is quite difficult to find appropriate mathematical expressions for the biochemical reaction rates. There are two principle approaches to solve that problem, the deterministic and the hybrid modelling. Both concepts are based on the assumption, that only one or few biochemical reaction steps are rate limiting for the whole process. The so called formal kinetic expressions are used for the description of the rates of these reactions in deterministic bioprocess models.
Usually these expressions are simple nonlinear algebraic equations such as the wellknown Monod equation. Hybrid bioprocess models contain Artificial Neural Networks and/or fuzzy submodels for the calculation of reaction rates.
Generally bioprocess models can be built for different purposes such as process control, reactor design or scale-up. For each of these objectives a model has to satisfy specific requirements. In the following the features of models suitable for experimental design procedures are discussed.
1.2. TRANSPARENCY
The fundamental idea of model based experimental design is the assumption, that the predicted behaviour of the model is similar to that of the real process. That means the model has to predict phenomena of the process that are relevant to the experimental design problem. Generally the admissibility of that assumption can be proved using statistical approaches. However in biotechnological experiments the number and the accuracy of measurement data are mostly insufficient for the application of these statistical hypothesis testing methods. Therefore, the similarity of model behaviour to the real process is assessable empirically only. Skilled experts have to check the biological meaning of the bioprocess model and the underlying hypotheses concerning the rate limiting reaction steps. For that reason the model must be transparent, understandable and explainable.
131