
Engineering and Manufacturing for Biotechnology - Marcel Hofman & Philippe Thonart
.pdf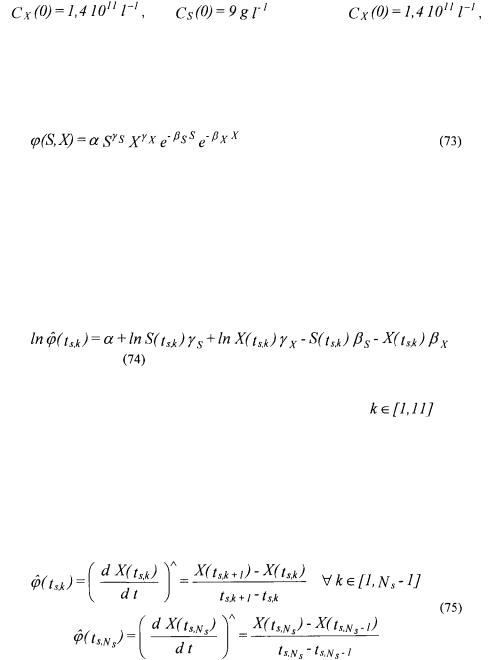
Macroscopic modelling of bioprocesses with a view to engineering applications
with 11 discrete measurement samples of S and X (initial conditions:
and
For each of the five specific growth rate laws {(68),...,(72)}, the two experiments obtained with the simulator {(66),(67)} (and the corresponding measurements of S and X) are used within the systematic identification methodology (second and third step presented above in Section 4) in order to estimate the kinetic parameters within the general model structure (16) which becomes here:
The aim being to test the flexibility of this structure in order to reproduce the behaviour obtained with 5 different kinetic laws, no noise is added on the “measurements” of X and S provided by the simulator {(66),(67)}. For the same reason, the first step of the identification procedure is not used here and the pseudo-stoichiometric coefficient vs is fixed at its “true” value in each case.
Concerning the identification of the kinetic coefficients and
in relation (73), a linearisation of the structure is performed thanks to a
logarithmic transformation:
where
• is the sampling time corresponding to the sample of the experiment
• is an estimation of the reaction rate.
In order to build the estimations one has to note that, in the mass balances (66), the reaction rate
corresponds directly to the derivative of the biomass concentration X w.r.t. time. Hence, an estimation of the reaction rate is given by an estimation of this derivative. Therefore, an Euler approximation is used:
101
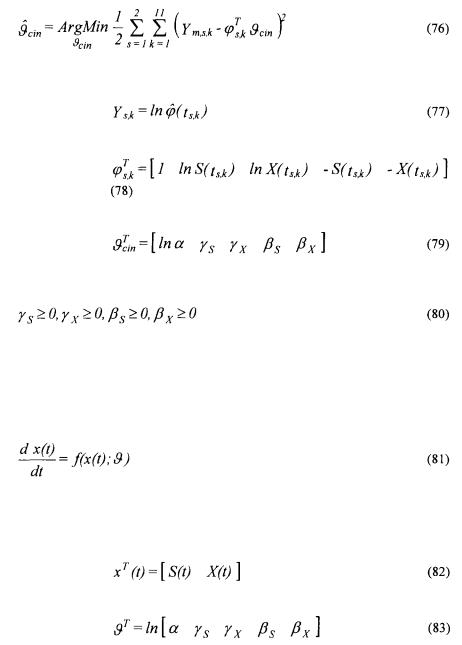
Ph. Bogaerts and R. Hanus
Note that this procedure is very rough but is acceptable in this ideal case in which the
“measurements” are supposed to be perfect, i.e. not corrupted by noise. The relations {(46),..., (50)} of the second step of the identification procedure become in this case:
where
•
•
•
and under the constraints
This optimisation problem leads to a unique solution, completely independent of any initial guess.
Due to the use of the approximations (75), it is necessary to use the third step of the identification procedure which is only based on the simulation model and on the available measurements. The relations {(52),...,(55)} reduce to:
where
•
•
102

Macroscopic modelling of bioprocesses with a view to engineering applications
• f is the model structure corresponding to the relations
{(66),(73)}.
The solution of this differential system (81), which is obtained numerically, is noted
In agreement with the relations {(59),(60)}, the measurements |
given by the |
simulator {(66),(67)} are compared with the numerical solution (84) evaluated at the sampled times
under the constraints
This nonlinear optimisation problem is initialised with the result (76). The covariance matrix (61) is not computed given the assumption of non noisy measurements. The obtained results are presented in Table 1. One can see that the cost functions (maximum
likelihood cost function |
for the final identification (85) of the kinetic |
coefficients) are significantly decreased when applying the third step on the basis of the results of the second one (hence only using the nonlinear simulation model instead of the Euler approximation which is used in the second step). Results are presented in
cross validation (Fig. 1 to 5) starting from the initial conditions
and (experiment which has not been used for parameter estimation).
In these figures, the straight lines correspond to the simulation obtained with the new general kinetic model structure whereas the circles represent “measurements” of the simulator using the Monod-rype law. Other simple and cross validation results are presented in Bogaerts (1999). These results are quite convincing and illustrate the flexibility of the general kinetic structure in the sense that it is able to reproduce by its own the behaviour of several different kinetic structures.
103
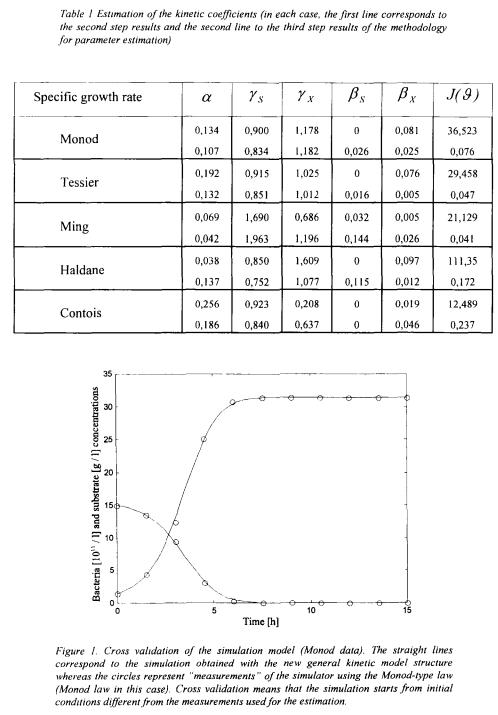
Ph. Bogaerts and R. Hanus
104
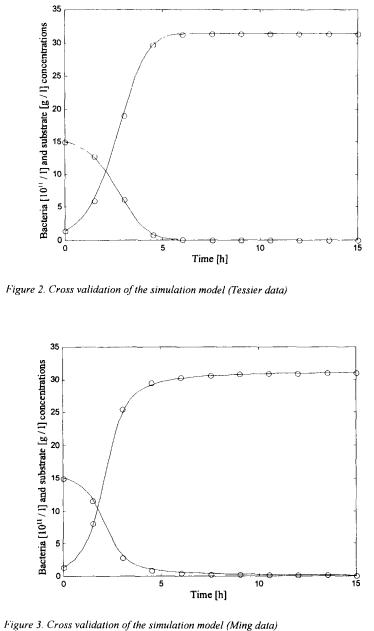
Macroscopic modelling of bioprocesses with a view to engineering applications
105
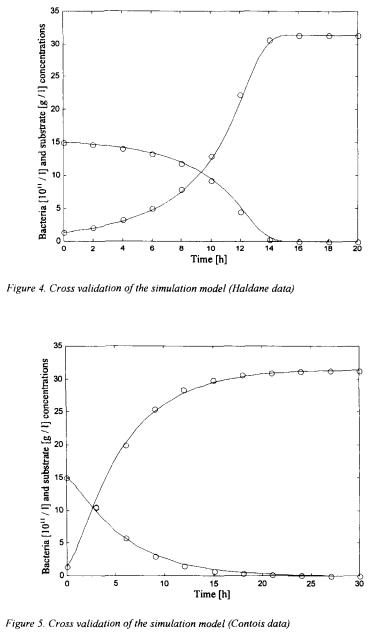
Ph. Bogaerts and R. Hanus
106
Macroscopic modelling of bioprocesses with a view to engineering applications
6. Conclusions and perspectives
Mathematical modelling is useful in order to build engineering tools like simulators, software sensors or controllers. For these aims, it is necessary to limit the complexity of the mathematical model structures. Although there exist some general frameworks for mathematical modelling of bioprocesses, they suffer of some significant drawbacks or limitations. In the same way, several drawbacks are present in the use of the usual members of the class of unstructured and unsegregated models, although the level of complexity is well appropriated regarding the modelling aims.
Therefore, a general kinetic model structure, overcoming these problems and exhibiting several interesting properties, is proposed. It has to be used within mass balances for the macroscopic species involved in a reaction scheme that describes the essential phenomena of the culture. Concerning the problem of the parameter identification (pseudo-stoichiometric coefficients and kinetic coefficients), there are also many problems and "lacks" that are encountered in the literature. Hence, a systematic three-step identification procedure has also been proposed. It takes into account the measurement errors (for each signal and at each sampling time, including the initial one) and gives estimation of the covariance for the errors on the identified parameters. Finally, necessary conditions for the reaction scheme validation can be tested on the basis of the identified parameters. The flexibility of the general kinetic model structure and a part of the identification methodology have been illustrated on simulated bacteria cultures.
A detailed illustration of the whole methodology in a real case study (CHO animal cell cultures in spinner flasks) can be found in Bogaerts (1999). Several other applications are currently in progress. In the same context of animal cell cultures, the use of this type of models for building software sensors (for instance for the biomass concentration) can be found in Bogaerts (1999) and in Bogaerts and Hanus (1999, 2000).
Based on the fact that a model has to be thought in terms of the modelling aim, the parameter estimation procedure (namely its third step) has been adapted for the case of models to be used in software sensors (Bogaerts and Vande Wouwer, 2000a, 2000b). A new identification cost function has been proposed, combining a classical maximum likelihood criterion and a scalar function of the state estimation sensitivity matrix. This latter quantifies the ability of the software sensor to reconstruct the state of the process on the basis of the available measurements.
As the macroscopic reaction scheme plays a key role in this mathematical modelling framework (and even in many other ones), the a priori determination of this scheme is very important and sometimes very tedious. Therefore, a method for the systematic generation of reaction networks (namely all the reaction networks which are C- identifiable) has been developed and will be published in the very near future.
Future research will focus on the problem of the a posteriori validation of the a priori assumptions on the measurement noises and on the way to handle this information. For instance, if the measurement noise is not really normally distributed some mathematical transformation of the measured signals could lead to new signals which would be normally distributed. Another perspective is to deal not only with the
107
Ph. Bogaerts and R. Hanus
measurement errors but also with some structural errors of the model. Finally, the possibility to extend the use of the kinetic structure and of the parameter estimation method in the field of structured and/or segregated models will also be studied.
References
Barford, J. P., Philips, P. J. and Harbour, C. (1992) Simulation of animal cell metabolism, Cytotechnology, 10, 63-74.
Bastin, G. and Dochain, D. (1990) On-line estimation and adaptive control of bioreactors, Elsevier, Amsterdam.
Bastin, G. and Van Impe, J. F. (1995) Nonlinear and adaptive control in biotechnology: a tutorial, European Journal of Control, I , 37-53.
Bogaerts, Ph. (1999) Contribution à la modélisation mathématique pour la simulation et l’observation d’états des bioprocédés, PhD thesis, Université Libre de Bruxelles (Belgium).
Bogaerts Ph. (1999b) A hybrid asymptotic - Kalman observer for bioprocesses, Bioprocess Eng., 20 (No 3), 249-255.
Bogaerts, Ph., Castillo, J. and Hanus, R. (1999) A general mathematical modelling technique for bioprocesses in engineering applications, System Analysis Modelling Simulation, 35, 87-113.
Bogaerts, Ph. and Hanus, R. (1999) On-line estimation of biomass concentration in CHO animal cell cultures, Proceedings of the Third International Symposium on Mathematical Modelling and Simulation in Agricultural and Bio-Industries Uppsala,97-102.
Bogaerts, Ph. and Hanus, R. (2000) Nonlinear and linearised full horizon state observers - Application to a bioprocess, IFAC International Symposium on Advanced Control of Chemical Processes (ADCHEM
2000), Pisa, 1, 371-376.
Bogaerts, Ph. and Vande Wouwer, A. (2000a) Parameter identification of a model to be used for state estimation - Application to a bioprocess, Proceedings of the IEE Control 2000 Conference, Cambridge, accepted for publication
Bogaerts, Ph. and Vande Wouwer, A. (2000b), Modellidentifikation zur zustandsschätzung - Anwendung auf einen bioprozess, Automatisierungstechnik, 48 (No 5), pp. 240-247
Cazzador, L. and Mariani, L. (1993) Growth and production modelling in hybridoma continuous cultures,
Biotechnol. Bioeng., 42, 1322-1330.
Chen, L. and Bastin, G. (1996) Structural identifiability of the yield coefficients in bioprocess models when the reaction rates are unknown, Math. Biosci., 132, 35-67.
Chotteau, V. (1995) A general modelling methodology for animal cell cultures, PhD thesis, Université Catholique de Louvain (Belgium).
Delgado, J., Meruane, J. and Liao, J. C. (1993) Experimental determination of flux control distribution in biochemical systems: in vitro model to analyse transient metabolite concentrations, Biotechnol. Bioeng., 41, 1121-1128.
Dhurjati, P., Ramkrishna, D., Flickinger, M. C. and Tsao, G. T. (1985) A cybernetic view of microbial growth: modelling of cells as optimal strategists, Biotechnol. Bioeng., 27, 1-9.
Gauthier, J. P., Hammouri, H. and Othman, S. (1992) A simple observer for nonlinear systems - Application to bioreactors, IEEE Trans. Automat. Contr., 37, 875-880.
Glacken, M. W., Adema, E. and Sinskey, A. J. (1988) Mathematical descriptions of hybridoma culture kinetics: I . Initial metabolic rates, Biotechnol. Bioeng., 32, 491-506.
Goergen, J. L., Marc, A. and Engasser, J. M. (1994) Biochemistry of cells in culture: modelling, in: Cells and tissue culture: laboratory procedures (Doyle A. et al., eds.), John Wiley and sons, Chichester,
8B:2.1-8B:2.19.
Holmberg, A. (1983) On the accuracy of estimating the parameters of models containing Michaelis-Menten type non-linearities, in: Modelling and data analysis in biotechnology and medical engineering
(Vansteenkiste G. C. and Young P. C., eds.), North-Holland, Amsterdam, 202.
Julien, S., Babary, J. P., Lessard, P. and Paul, E. (1998) Theoretical and practical identifiability of an activated sludge process model, Proceedings of the IMACS Multiconference on Computational Engineering in Systems Applications (CESA ‘98), Nabeul-Hammamet, 1, 988-992.
108
Macroscopic modelling of bioprocesses with a view to engineering applications
Martens, D. E., Sipkema, E. M., de Gooijer, C. D., Beuvery, E. C. and Tramper, J. (1995) A combined cellcycle and metabolic model for the growth of hybridoma cells in steady-state continuous culture,
Biotechnol. Bioeng., 48, 49-65.
Montague, G. and Morris, J. (1994) Neural-network contributions in biotechnology, Tibtech, 12, 312-323. Murray-Smith, D. J. (1998) Methods for the external validation of continuous system simulation models: a
review, Mathematical and Computer Modelling of Dynamical Systems, 4 (No 1), 5-31.
Nielsen L. K., Reid S. and Greenfield P. F. (1997) Cell cycle model to describe animal cell size variation and lag between cell number and biomass dynamics, Biotechnol. Bioeng., 56, 372-379.
Pirt, S. J. (1965) The maintenance energy of bacteria in growing cultures, Proc. Soc. Lond. Ser. B, 163, 224231.
Ramakrishna, R., Ramkrishna, D. and Konopka, A. E. (1996) Cybernetic modelling of growth in mixed, substitutable substrate environments: preferential and simultaneous utilisation, Biotechnol. Bioeng., 52, 141-151.
Roels, A. (1983) Energetics and kinetics in biotechnology, Elsevier Biomedical Press, Amsterdam.
Ryckaert, V. G. and Van Impe, J. F. (1996) Design methodologies for nonlinear observers - Application to biological models, Proceedings of the IMACS Multiconference on Computational Engineering in Systems Applications (CESA ‘96), Lille, 1, 1052-1057.
Savageau, M. A. (1969a) Biochemical systems analysis. I. Some mathematical properties of the rate law for the component enzymatic reactions, J. Theor. Biol., 25, 365-369.
Savageau, M. A. (1969b) Biochemical systems analysis. II. The steady-state solutions for an n-pool system using a power-law approximation, J. Theor. Biol., 25, 370-379.
Some, I., Bogaerts, Ph., Dubois, J., Hanus, R. and Hanocq, M. (1999) Nonlinear identification method for drug stability parameters estimation, Int. Journal of Pharmaceutics, 184 (n°2), 165-173.
Syu, M.-J. and Tsao, G. T. (1993) Neural network modelling of batch cell growth pattern, Biotechnol. Bioeng., 42, 376-380.
Tan, Y., Wang, Z.-X. and Marshall, K. C. (1996) Modelling substrate inhibition of microbial growth,
Biotechnol. Bioeng., 52, 602-608.
Torres, N. V., Voit, E. O. and González-Alcón, C. (1996) Optimisation of nonlinear biotechnological processes with linear programming: application to citric acid production by Aspergillus niger,
Biotechnol. Bioeng., 49, 247-258.
Torres N. V., Voit E. O., González-Alcón C. and Rodriguez F. (1997) An indirect optimisation method for biochemical systems: description of method and application to the maximisation of the rate of ethanol, glycerol, and carbohydrate production in Saccharomyces cerevisiae, Biotechnol. Bioeng., 55, 758-772.
Tsuchiya, H. M., Fredrickson, A. G. and Aris, R. (1966) Dynamics of microbial cell populations, Adv. Chem. Eng., 6, 125-206.
Tziampazis, E. and Sambanis, A. (1994) Modelling of cell culture processes, Cytotechnology, 14, 191-204. Van Impe, J. F. and Bastin, G. (1995) Optimal adaptive control of fed-batch fermentation processes, Contr.
Eng. Practice, 3 (No 7), 939-954.
Walter, E. and Pronzato, L. (1997) Identification of parametric models from experimental data, Springer, Paris
Westerhoff, H. V. and Kell, D. B. (1987) Matrix method for determining steps most rate-limiting to metabolic fluxes in biotechnological processes, Biotechnol. Bioeng., 30, 101-107.
Xie, L. and Wang, D. I. C. (1996a) Material balance studies on animal cell metabolism using a stoichiometrically based reaction network, Biotechnol. Bioeng., 52, 579-590.
Xie, L. and Wang, D. I. C. (1996b) Energy metabolism and ATP balance in animal cell cultivation using a stoichiometrically based reaction network, Biotechnol. Bioeng., 52, 591-601.
Zeng, A.-P. and Deckwer, W.-D. (1995) A kinetic model for substrate and energy consumption of microbial growth under substrate-sufficient conditions, Biotechnol. Prog., 11, 71-79.
109
A MODEL DISCRIMINATION APPROACH FOR DATA ANALYSIS AND EXPERIMENTAL DESIGN
R. TAKORS, D. WEUSTER-BOTZ*, W. WIECHERT**, C.
WANDREY
Institute ofBiotechnology, Research Centre Juelich, 52425 Juelich,
Germany
*Chair of Biochemical Engineering, Munich University of Technology,
Boltzmannstr. 15, 85748 Garching, Germany
** IMR, Department ofSimulation, University ofSiegen, Paul-Bonatz Str.
9-11, 57068 Siegen, Germany
Abstract
A general model discrimination approach is presented that enables data based model structure discrimination as well as model discriminating experimental design. Results of closed-loop controlled steady-state fermentations with the methylotrophic yeast Candida boidinii are used to clearly discriminate the “right” model out of a group of 10 competing models (53% model probability). Using the identified model the kinetics of batch and fed-batch fermentations with Candida boidinii could be described too. The applicability of the model discriminating experimental design approach is shown by simulation results using the kinetics of Zymomonas mobilis.
1. Introduction
Microbial fermentation kinetics like growth, substrate consumption and product formation are often described using unstructured kinetic models. These “simple” modelling approaches usually regard cells as “black-box” systems. Thus the kinetic cell behaviour is modelled by taking into account only a relatively few number of physiological state variables like cell-dry-weight, extracellular substrate or product concentrations and by using Monod-type modelling approaches. Due to their simplicity, these models could easily be used to quantitatively describe microbial fermentation courses, to implement model based fermentation control strategies or to simulate the scale-up of fermentation processes.
Unstructured models are usually identified by model parameter fit based on fermentation data that were derived from kinetic experiments. Batch experiments represent the simplest experimental method for the generation of kinetic data. However
111
M. Hofman and P. Thonart (eds.), Engineering and Manufacturing for Biotechnology, 111–128.
© 2001 Kluwer Academic Publishers. Printed in the Netherlands.