
Крючков Фундаменталс оф Нуцлеар Материалс Пхысицал Протецтион 2011
.pdfThe following types of measurements are identified for accounting and control systems:
depending on the objects measured: measurements of bulk forms (bulk samples) to determine the weight and volume and measure material samples (to find out isotopic and/or element composition);
depending on how the measured material is handled: destructive measurements (involve normally destruction of either the chemical or physical form of the material sample) and nondestructive measurements (quantitative or qualitative determination of the type and/or quantity of NM in a sample without producing significant changes to or getting into the sample);
depending on the technique employed to determine the measured quantity: direct and indirect measurements.
Any measurement, as mentioned earlier, involves a certain error. Therefore, formulating the decision to be made based on accounting data needs these errors to be taken into account. What are these and what are their potential sources will be discussed hereinafter.
Direct measurements have the measured and determined quantity compared to the measurement unit directly or using an instrument graduated in respective units.
Indirect measurements have the measured quantity determined (calculated) from direct measurements of other quantities that relate to the measured quantity through a functional dependence.
Measurement error
We will treat error as a deviation of an estimate from the true value of the characteristic estimated for. Then, as applied to measurements, this is a deviation of the measurement result from the true (actual) values of the measured quantity. Note that we do not normally know the true value since uncertainties exist, and properly describing and estimating errors requires that a suitable mathematical model of the error should be selected. Therefore, the actual error is the estimate of the respective uncertainties we have obtained (see p. 4.2.).
The simplest model for a single measurement is
X = T + E, |
(4.40) |
where X is the measurement result; T is the true value and E is the measurement error [5].
181
We have already talked about numerous sources of errors as may affect measurement data. All errors are categorized into random errors and systematic errors (also the so-called short-term systematic errors are occasionally introduced).
Indeed, the quantity E can be presented as random and having the mathematical expectation M (E) = S . Then (4.40) will be written as
X = T + S + R, |
(4.41) |
where R is the random quantity with the zero mathematical expectation M (R) = 0 conventionally referred to as random error; and S is the so-called systematic error often treated as an unknown constant shift of the measured quantity about the true value.
Here are several examples to consider for a better conception of this division.
Random error relates to the action of a series of random factors per each individual measurement, e.g. random nature of the nuclear decay process, air motion, sample position and so on.
Consider six UO2 pellets of nominally one composition to be tested for the uranium concentration. Let us denote: хij is the result of j–th uranium concentration measurement in the i– th pellet; μ is the nominal (declared) uranium content in the pellets; ρi is the uranium content deviation from the declared quantity in the i– the pellet; and is the deviation conditioned by the analytical error of the j–th measurement. With the aid of a multiplicative model we will arrive at
х11=μ+ρ1+ε1, х22=μ+ρ2+ε2,
………………
х66=μ+ρ6+ε6.
As ρi and εj differ for each of the six results, these are random errors. According to the classification we have introduced, ρi is the random error of the statistical sampling. If to consider this as a random variable with the average value equal to zero and the variance σρ2, then σρ2 is called the variance of the statistical sampling random error. Similarly, εj in the classification in question is an analytical random error, and σε2 is called the variance of the analytical random error.
182
First, since only one measurement was done for each pellet, and ε and ρ have become parts of the equation with one index, then, in the given case, these two errors cannot be separated. In such situation, one can consider them to be a single “random measurement error”. In the event of two or more measurements done for each pellet, errors are separable. Second, a feature of the random error model is that its index varies with each measurement, its influence on the eventual result decreasing with each new measurement. For this reason, random errors are relatively easy to control.
Systematic error is an error caused by the limited accuracy of the instrument, an improper installation of the instrument, the technique used to process data and the effects of external factors. Systematic errors determine the measurement group. Systematic errors are errors caused by the improperly reset scale and the rounding of numerical values.
Let us expand a bit the presented model by giving it one more summand: - a deviation from the nominal value conditioned by the analytical method. It will be the same for all measurements done by the given method. Then
х11=μ+ +ρ1+ε1, х22=μ+ +ρ2+ε2,
………………
х66=μ+ +ρ6+ε6.
The quantity that does not have an index and is common for all measurements is called systematic error or bias. These terms are normally used as equals, still they have a small difference. If measurement data is
adjusted for the known, somehow estimated quantity |
, then |
is called |
||||
bias. However, if |
is not |
known exactly |
and can |
be estimated |
only |
|
roughly, then measurement |
data cannot be |
adjusted |
precisely |
by |
. A |
residual bias so occurs, this being equal to the difference between the quantity and its estimate. It is this residual bias that is called systematic error. Not everyone differentiates so between systematic error and bias, still it is important to understand what do you mean exactly by .
If is a random variable with the average value equaling zero and the variance σ 2, then σ 2 will be called the systemic error variance.
Many aspects of accounting and control are more influenced by systematic errors than by random ones. The explanation is that, unlike random error, the systematic error effect does not decrease with each new measurement, thus reducing the efficiency of the NM accounting measures.
183
Sources of errors
An error in a given measurement results from several errors overlapping (superimposed). Consider some of the error sources that may affect measurement data.
1. Statistical sampling error. Consider a set of N items, e.g. containers with NM, each of these having a true value of a characteristic (net weight, uranium mass, enrichment, etc.). If several items have been selected randomly from the set (sample), then the average value of a characteristic calculated for these items will differ from the average value of the given characteristic for the whole set (say, from the average enrichment value). Let us determine the statistical sampling error as the difference between the average value of a characteristic for a randomly taken sample and the average value of the characteristic for the entire set.
This error is neglected in some cases, say, inventory taking has the inspector-obtained measurement data for an item compared against the operator data, so the true value of the measured characteristic does not matter and it is reduced in the difference.
In the event of attributive (quality) inventory taking, the true value for each item is equal either to 1, if there is a defect, or to 0, if there is none. And the average value for the group of interest is equal to the relation of the number of defective items in the group to the total number of items: 0<Ndef/Ntot<1. In the given case, therefore, there is a statistical sampling error in each selected item. The influence of this error on the inventory taking data (and so the necessity of taking this into account) will vanish only if all items are checked.
Depending on whether we do measurements for all nuclear material (a universe) or just for a portion of same (a sample), material is accounted for based on three measuring operations:
1)determination of the material net weight or volume (bulk measurements);
2)material sample taking;
3)analysis of the material sampled to find out its element and/or isotopic composition. So, normally, it will be convenient to divide the total measurement error into such components as will match the three measuring operations.
2. Error of measurements in bulk form (bulk measurements) will be defined by us as the difference between the true weight (or volume) of an item and its measured value. With only this definition considered, a bulk measurement error appears to be a directly obtainable simple value.
184
However, it may and even is most likely to result from a great deal of bulk measurement errors superimposed, some of these being capable to compensate each other.
3.Material sampling error is found from the relation to characteristic measured. This may be uranium or plutonium concentration, enrichment and so on.
A material sampling error is the difference between the average values of a characteristic for the sample taken and the respective average value of the given characteristic for all material. It is important to understand what “all material” means. If we are interested in the u ranium concentration to be found for the given container, then the sampling error is the difference between the uranium concentration in a small sample taken from this container, and the uranium concentration in the container. This may be referred to as the “sampling error for one containe r”. If the measurement data for a sample taken from the given container with the given uranium concentration needs to be extended to other, nominally the same containers, then the change in the concentration between containers is incorporated in the sampling error together with the “error for one container”.
4.Analysis error. As is the case with the material sampling error, the analytical error (analysis error) is determined for the characteristic of interest. An analytical measurement error is the difference between the true value of the sample characteristic in question and the measured value of this characteristic. Note that this error relates to the sample rather than to all material it characterizes. An error of determining the value of a characteristic for all material will be a combination of the analytical error and the material sampling error.
Where nondestructive measurements are used with no sampling involved, it is the error of measuring a characteristic that will be the analytical error of measurement; with nondestructive measurements done not for the whole of the item but for a sample of it, the sampling error should be also accounted for.
As noted earlier, the given error under determination results from many errors superimposed. For example, a weighing error may depend on how the item is placed on the scale, the type of the scale, the particular scale of the given type, the operator and the environment (air temperature, humidity and so on). The extent to which attention is to be given to the search for and estimation of factors that affect the error depends on a number of circumstances. For instance, if a weighing error at the measurement point of interest does not influence greatly the accounting data quality, there is no special need for identifying and evaluating every source contributing to this
185
error. And, vice versa, if the weight values observed at a measurement point turn out to have an error in excess of the level desired, a special study is required to find out why this has happened. And such a study needs at least some of the above-mentioned error sources to be identified, and individual contributions thereof to the error estimated. By a “special study” we mean, say, calibration.
Models of errors
We have discussed just some of many error sources. Though it is their cumulative effect that we are interested in the most, it is often convenient or even necessary to form a reasonable mathematical model to identify errors as such and find out how these are interlinked. Therefore, a model helps combine errors correctly, find their cumulative effect and identify sources of errors, and provides safeguards against potential skips of certain sources. Let us discuss this in more details and introduce two basic models: an additive model and a nonadditive (so-called multiplicative) model of error.
Additive model. This is the simplest model (4.40) we have already made use of. Despite being simple, additive models are often used in practical activities and, in many instances, give a nearly real result. A note should be made that any model is a mathematical description of reality. Experience shows that humans tend to employ simpler models in practice, occasionally even at a sacrifice of detailed and adequate description of reality. Simple models are therefore commonly used.
Let, say, хbe the measured gross weight value of a container with UO2
powder, g; μ the true value of this quantity, and ε the error, so then х= μ + ε .
Therefore, if summed up, the true value and the error would give the measured value. If measurements are done on standard samples of the known weight μ with their measured value хrecorded, then the distribution function ε can be constructed based on the measurements.
Multiplicative model. Describing a measurement result by a simple sum of the true value and the errors often gives an acceptable description of the real situation, though not altogether universally. If the absolute error of measurement grows with the measured value, the so-called multiplicative model is employed.
Assume that у is the measured value of the uranium concentration in UO2, α is the true quantity and η is the measurement error.
186
Then у= α + η. Let us be interested in the net weight of uranium in the container from the previous instance rather than in the weight of oxide. By multiplying х by у, we get ху = μα + μη + αε + εη, which no longer makes a simple additive model.
In this case, it will be more convenient to use a multiplicative model:
x = μ (1+ε), у= α (1+η).
Then the expression for the uranium weight will look as follows: ху= μα (1+η)(1+ε).The latter expression is apparently much simpler, more graphic and more convenient in terms of error estimates. So, though additive models are frequently convenient to use, one should avoid using these in all cases as the net effect of several errors is not always a simple sum thereof.
Estimation of NM measurement errors
Confident estimates of nuclear material measurement errors are required to estimate the significance of the inventory differences detected. These estimates depend on the models adopted to calculate errors and interpret «random» and «systematic» errors.
Test measurement data is expected to satisfy to accuracy and precision requirements. Precision is characterized by repeatability and reproducibility of measurement data.
Repeatability is determined by the variance (spread) of the data obtained by one operator when measuring one sample in similar conditions. Reproducibility is determined by the variance of the measurement data for a sample measured by more than one operator for several days in various conditions.
Accuracy of data depends on the quantity of the systematic measurement error. The systematic quantity error can be estimated as the average difference (in the sense of mathematical expectation) between the values measured and the true value (4.40). A small quantity of the systematic error in data is equivalent to a high accuracy thereof.
Basic principles of measurement error calculations:
1)the total measurement data errors are computed as the sum of the random error and the systematic error as reduced to the same confidential probability;
2)the values of all errors are normally reduced to the confidential probability of 0.95;
187

3)when computing the random error in data, one should treat the respective quantities as normally distributed;
4)one should consider the systematic error of a measurement result on the assumption of uniform (equally probable) law of distribution of constituent quantities.
Agreed notation.
1. According to GOST State Standards, the following designations should be used to denote errors of the quantity х as reduced to the confidential probability of 0.95: random error – S(x), systematic error – θ (x), total error – δ (x).
2.When choosing the confidence probability рother than equal to 0.95, one should use the record рS(x), рθ (x), рδ (x).
3.The absolute measurement error should be designated as DS, Dθ , D.
4.The ultimate measurement result should be presented as х= Х, δ =… %, θ =… %, р = 0,95, where Х is the root-mean-square value of the measured quantity х, and θ is the maximum boundary of the nonexceptional residue for the systematic error of the quantity хunder determination.
Direct measurements
1. Estimation of the result with a large number (n > 3) of measurements for the quantity х:
|
|
|
|
|
|
|
|
n |
|
|
|
|
|
|
|
|
|
|
X = |
∑ wi xi |
|
||||||
|
|
|
|
|
i =1 |
|
, |
|
|
(4.42) |
|||
|
|
|
|
|
n |
|
|||||||
|
|
|
|
|
|
|
|
|
|
|
|
|
|
|
|
|
|
|
|
|
|
∑ wi |
|
|
|
|
|
|
|
|
|
|
|
|
|
i =1 |
|
|
|
|
|
where wi = |
|
1 |
is the weight of the i measurement’s result; |
|
|||||||||
|
|
|
|||||||||||
S |
2 (x ) |
|
|||||||||||
|
i |
|
|
|
|
|
|
|
|
|
|||
|
|
|
|
|
|
|
|
|
|
|
|
|
|
|
|
|
|
|
|
|
n |
- X )2 |
|
||||
|
|
|
S (x) = |
1 |
|
|
∑wi (xi |
|
|||||
|
|
|
|
|
i=1 |
|
, |
(4.43) |
|||||
|
|
|
x |
(n -1)× |
|
||||||||
|
|
|
|
|
|
n |
|
||||||
|
|
|
|
|
|
|
∑wi |
|
|||||
|
|
|
|
|
|
|
|
|
i=1 |
|
188
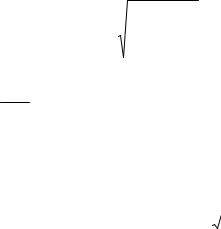
where рS(x) = pts×S(x) is the factor of reduction to the confidential probability P, Student’s coefficient (Table 4.2), [3] and [8].
|
|
|
|
|
Table 4.2 |
|
|
|
Student’s coefficients |
|
|
|
|
|
|
|
|
|
|
|
N |
|
|
P |
|
|
|
0.9 |
0.95 |
0.98 |
0.99 |
0.999 |
|
|
|
|
|||||
2 |
6.31 |
12.71 |
31.82 |
63.66 |
636.62 |
|
3 |
2.92 |
4.30 |
6.96 |
9.92 |
31.60 |
|
4 |
2.35 |
3.18 |
4.54 |
5.84 |
12.94 |
|
5 |
2.13 |
2.78 |
3.75 |
4.60 |
8.61 |
|
6 |
2.02 |
2.57 |
3.36 |
4.03 |
6.86 |
|
7 |
1.94 |
2.45 |
3.14 |
3.71 |
5.96 |
|
8 |
0.90 |
2.36 |
3.00 |
3.50 |
5.40 |
|
10 |
1.83 |
2.26 |
2.82 |
3.25 |
4.78 |
|
2. Estimation of the result with single measurements of the quantity х (n≤3). Single measurements of the quantity х should be preceded by a metrological test of the instrument to be used. Such test involves multiple (N > 20) measures of a certain quantity a similar to the quantity х, and determination of σ for the obtained value set аi:
|
|
|
N |
(ai − A)2 |
|
|
|
1 |
|
∑ |
|
||
s = |
|
i =1 |
|
, |
(4.44) |
|
A |
|
|
||||
|
|
|
N −1 |
|
||
N |
|
|
|
|
|
∑ai
where A = i =1 .
N
In further measurements of the quantity х, the result is estimated as follows:
|
N |
ωi xi |
|
|
|
|
|
|
|
∑ |
|
σ |
|
|
|
||
X = |
i =1 |
|
, S (x) = |
|
K , |
(4.45) |
||
∑ωi |
|
|
|
|||||
|
|
|
σ |
|
||||
|
|
|
n |
|
189
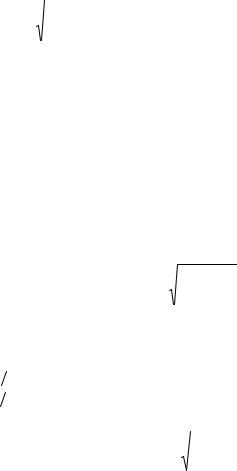
where Kσ is the coefficient that allows for various statistical significances of results when the instrument is tested and the quantity хis measured
K σ = |
|
wσ |
|
, |
wσ = |
1 |
, |
wx = |
1 |
are the weights of the measurement |
|
|
|
||||||||
|
|
wx |
|
s2 |
|
|
s2x |
results when the instrument is tested and хis determined
Indirect measurements
1. Estimation of the result in measuring the quantity y = f (x1, x2,…, xn) in a single experiment:
·the input is measured values xi, as well as the random error and the systematic error of the constituent quantities S(xk) and θ (xk);
·the errors of the constituent quantities that are part of the formula to determine the quantity у, but not measured in the given experiment
(constants, coefficients, certification results) shall be treated as θ (xk);
·the result Y is estimated by the following formula:
Y = y,
|
|
m |
|
|
|
|
S (Y ) = |
∑S 2y (xk ) , |
(4.46) |
||||
|
k =1 |
|
|
|
||
where |
|
|
|
|
|
|
S y (xk ) = |
xk |
× |
¶f |
× S (xk ) , |
(4.47) |
|
|
|
|||||
|
|
Y |
¶xk |
|
¶Y Y is the coefficient of the function Y sensitivity to the variation of the |
||||||||
¶x x |
|
|
|
|
|
|
||
quantity xk; |
|
|
|
|
|
|
||
|
|
|
|
|
|
|
|
|
|
|
|
m |
|
||||
θ (Y ) =1,1 ∑θ 2y (xk ) , |
(4.48) |
|||||||
|
|
k =1 |
|
|||||
where |
|
|
|
|
|
|
||
θ y (xk ) = |
xk |
|
× |
¶f |
×θ (xk ). |
(4.49) |
||
Y |
|
|
||||||
|
|
|
¶xk |
|
The total measurement data error is δ (Y) = S(Y)+θ (Y).
Often either S(Y)>>θ (Y) or θ (Y)>>S(Y). In these cases, the error calculation formula becomes simpler.
2. Estimation of the measurement result for the quantity y = f (x1, x2, …, xn) in a multiple experiment:
190