
Крючков Фундаменталс оф Нуцлеар Материалс Пхысицал Протецтион 2011
.pdf∙last name of the person approving the operation.
This data can be seen to include all NM batch parameters.
Documentation of transactions in the above form enables closing of the NM balance in the MBA, estimation of the NM shipper/receiver difference and adherence to regulatory NM accounting and control requirements
3.5. Federal automated NM accounting and control system
The federal automated information NM accounting and control information system (FIS) was established to provide information support to management of nuclear material for peaceful uses and counter illegal circulation thereof. The FIS software was developed by FSUE “TsNIIatominform”.
The FIS is a component of the SSAC and is intended to give information support for governing authorities in charge of planning and management of nuclear material. Among other things, the FIS is intended to:
∙give information support to Rosatom of Russia having powers in implementation of federal-level NM accounting and control;
∙communicate with agency information systems. The FIS is tasked to:
∙create and operate the state NM register;
∙deliver NM data to consumers concerned;
∙give information support to NM exports and imports;
∙give information support to NM transportation tasks.
The FIS is currently based at Rosatom’s Crisis Management Center (CMC) that includes an information and analytical center (IAC).
The IAC supports continuous communication with operators delivering data to FIS. It is exactly to the IAC that corporate information systems report on quantities and movements of NM. The IAC gathers reports on NM, checks these for conformity to specified requirements and regulations, tests data using all kinds of techniques, and, if found conforming to specified requirements, adds data to database. The IAC therefore forms the array of reporting data on NM, operates reference arrays, operates relevant versions of classifiers and communicates actively with data suppliers.
The current stage of the FIS evolution limits the range of data suppliers to Russian operators. To be able to deliver data, operators establish automated NM A&C systems to ensure corporate NM accounting, generation of regulatory NM reports and delivery of these to the FIS.
151
The IAC is also responsible for giving information support to the FIS users. It interacts with the FIS information suppliers and defines the required volume and quality composition of data submitted. Most of the information provided by the FIS is consumed by Rosatom executive staff and departments. No interactive user access to the FIS database is presently provided. However, this capability is expected to be made available at subsequent FIS evolution stages, provided information security features are in place.
Initially, the FIS was evolving towards gathering reports on material balance areas. So NM data transmission in the FIS was accordingly based on presentation of data from all MBAs at each enterprise. Operators reporting to the FIS sent in NM inventory listing for MBAs made out as of the reported period ending point and the NM physical inventory reports. This reporting pattern was looked upon as complete. In 1999-2004 the FIS had over 60 MBAs from more than 20 operators connected thereto using this reporting pattern. However, for all attractiveness thereof, this pattern should be understood to be feasible only if a number of conditions are fulfilled, including if corporate automated NM A&C information systems are in place. Besides, it takes quite a time to connect corporate NM A&C systems to the FIS. So, in 2000, a simplified (consolidated) pattern was implemented by Rosatom to connect operators to reports in the FIS. All operators circulating NM were sending annually to the FIS NM Inventory Listings (IL) and NM Inventory Change Reports (ICR) in the form consolidated for respective enterprises.
This dual-form (integrated and consolidated) reporting system had drawbacks (including duplication of NM data flows, loss of time to prepare reports and others), so in 2006 it was decided to switch to a “common reporting system”. As the result, the common corpor ate reporting system was launched into pilot operation in 2007 to replace, subsequently, the integrated and consolidated reporting systems. Common reporting of NMhandling operators hinges on reports based on presentation of data from MBAs merged into so-called reported areas (RA). The reported area is an area administratively established within an organization and formed by one or more MBAs to generate and deliver reports on the MBAs concerned to the SSAC federal level.
The IL RA and IRC RA reports from the area reported on are generated based on IL and ICR reports from the MBAs forming the RA with regard for the material holder and usage mode data.
IL RA/ICR RA reports should be prepared by the operator as a paper document or as its electronic version. An organization’s report coming into
152
the FIS IAC is handled at input to result in a data acceptance and error report which is forwarded to the organization concerned. If there are no errors in the organization’s report, the reported data are downloaded into the FIS database. In the event of errors, the IL RA/ICR RA data is subject to adjustment by the operator’s personnel or the FIS IAC personnel as approved by the operator and Rosatom’s respective department.
153
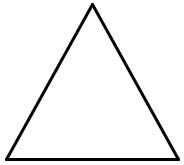
CHAPTER 4
STATISTICAL BASIS OF NM ACCOUNTING AND
CONTROL
If formulated in brief, the prime objective of an evolving NM physical protection, accounting and control (PPA&C) system may be said to consist in ensuring confident traceability of NM at all nuclear fuel cycle stages. Ensuring confidence is surely a problem of mathematical statistics [1].
We shall look at how the triad of accounting, control (A&C) and physical protection (PP) of nuclear material (NM) is organized to help achieve the objective in view (Fig. 4.1).
Control |
Accounting |
NM
Physical protection
Fig. 4.1. The NM PPA&C triad
It is perfectly obvious that an efficient solution of the NM nonproliferation and confident traceability task depends on how effective and sufficient each of the triad components (physical protection, accounting and control of NM) is. A number of questions arise, which can be answered only by those who are knowledgeable in probabilistic and statistical methods:
∙how to quantify the efficiency of a nuclear-hazardous facility and nuclear material physical protection system;
∙how often and permissibly accurately a nuclear material shall be controlled;
154
∙ how NM accounting measurements shall be properly and sufficiently arranged for?
Use of statistical procedures in inspections and physical inventory taking is dictated by limited time and funds. It happens sometimes that one cannot or finds it economically unreasonable to check all items or all nuclear material in the given material balance area. Statistical methods may however help plan the scope and sequence of sampling so that one could be confident about the characteristics of all material measured from a portion of it.
The prime objective of ensuring traceability of NM is achieved by analyzing and estimating measurement uncertainties and searching for how to bring these down, planning the scope and sequence of sampling, and estimating the statistical significance of measurement discrepancies.
Normally, indeed, the control measurement result deviates from the value declared for the measured characteristic of the nuclear material subject to control. The deviation may be caused either by a measurement error which is determined statistically or by the actually observed difference between the values measured and declared. No final conclusion can be made without the respective statistical analysis done.
Probabilistic and statistical methods do not only enable the efficiency of an accounting and control system to be estimated in quantity terms, but also offer the only means to quantify NM vulnerability, a major characteristic of the physical protection system efficiency, which may be undoubtedly identified as probabilistic.
A large-scale program currently under way to upgrade and improve the state system of accounting for and control of NM envisions to give the state NM A&C system such important feature as extensive use of mathematical statistics methods.
4.1. Basic determination and reduction concepts of probability theory and statistics
Probability theory (PT) and mathematical statistics (MS) stand as a single branch of mathematics. This deals with procedures to solve direct and inverse prediction and decision-making problems. What is the subject matter of MS? One of the simplest definitions is based on the comparison relating to the concept of sampling from a finite universe. Knowing the composition of a universe, one can get a distribution for the composition of a random sample. This is a generic direct PT problem.
Commonly, however, one has to deal with inverse problems when the sample composition is known and it is to be found from this what the
155
universe was. Problems of the kind form the subject matter of MS. It may be said: use of PT, with the nature of a phenomenon being known, helps us find out how some characteristics of interest observable in an experiment will behave (are distributed). It is vice versa in statistics where the input is experimental data (these are normally observations of random quantities), and the requirement is to derive a statement (or decide) on the identity of the phenomenon under study.
Probability
Probability of event is generically comprehended as an abstraction that originates in the idea of the relative frequency at which an event occurs in the sequence of a replicated experiment (test) with the “given set of conditions”.
Imagine a relative frequency of an event in an unlimitedly protracted series of a replicated experiment, and you will get close to giving a reasoned interpretation of the probability of an event. Complexities will however arise if you try to base PT on strictly formalizing such interpretation. A simpler and deeper formulation was proposed by A.N. Kolmogorov [2]. It relies on the following assumptions:
∙interval numbers [0,1] may be chosen as the probability of an event in a certain initial class of relatively simple events;
∙these initial probabilities, along with the axioms and rules to determine the probabilities of more complex events, help determine the probability of any event.
Note. Therefore, choosing the probability of an event is normally governed by hypothetical data based on predictions of relative frequencies. One may ask if we have chosen correctly the probability in a given problem. Formally, this question leads us to a problem in theory of testing statistical hypotheses. If solved, this problem brings us to an answer on how to choose probability. Let us introduce these axioms and rules.
1.For every event А there is a given number Р(А) satisfying the condition 0 ≤ P(A) ≤ 1 , which is called probability.
2.The probability of a credible event is equal to unity.
3.The probability of the sum of mutually exclusive events is equal to the sum of the probabilities thereof (the so-called axiom of addition of probabilities).
Let us note again that the system of axioms does not determine the principle of choosing the probability’s numerical value. It is found such
156

that all axioms be fulfilled and the laws to which the event occurs be described.
Probability is an objective measure and exists (can be known!) a priori, while relative frequency is always empirical.
We will treat event А as not dependent on event В if the probability of event А does not change when event В occurs. So the pairwise independence we have introduced can be generalized to an independence in a set for a reasonably broad series of events.
Total probability formula and Bayesian formula
The probability of event F that occurs only with each of the events А1, А2,…, Аn, forming a complete system, provided one knows the probabilities thereof and the conditional probabilities of event F relative to each of them PA1 (F )PA2 (F ),..., PAn1 (F ) and so forth, is calculated by the total probability formula:
P(F ) = P( A1)PA1 (F ) + P( A2 )PA2 (F ) + ... + P( An )PAn (F ) =
n
=∑P( Ai )PAi (F ).
i=1
One can obtain a Bayesian formula (a hypotheses formula) by generalizing the product rule so considering that if P(АВ)=Р(А)РА(В)=Р(В)РВ(А), then РА(В)=Р(В)РB(А)/Р(А), or by generalizing for п events, and by using the total probability formula, we have:
PF ( Ai ) = nP( Ai )PAi (F ) .
∑P( A j )PA j (F ) j =1
Bayesian formula is often referred to as formula of hypotheses because it can be used to re-estimate the probability of hypotheses as to the occurrence of the events A1, A2 , A3 ,...An after it became known that event F had occurred.
157
Random quantities, distribution and characteristics thereof
We will hold random quantity as a variable that is capable of taking any such value as the case may require. Random quantity is any variable x having values that form a set of elementary events or denote points in a sample space.
The respective distribution of probabilities is called probability of the random quantity x. All random quantities are intrinsically divisible into discrete and continuous quantities.
A discrete random quantity has the set of its values finite or countable (number of items in storage, number of fuel elements, number of containers with NM, etc.).
The function р(х) that relates the value of a random quantity to the corresponding probabilities is called random quantity law. Distribution law can be presented in a tabulated or graphic form. However, it is rather difficult to get a complete idea of random quantity from its law. So certain constants of its law are used. These are the so-called moments of various orders. They provide as complete characterization of random quantity as possible. The most important moments are mathematical expectation (firstorder moment about zero) and variance (second-order moment about mean) [3].
Mathematical expectation of discrete random quantity
Mathematical expectation of a discrete random quantity is the total of the products of all its values by corresponding probabilities:
n |
|
M ( X ) = x1 p1 + ... + xi pi + ... = ∑xi pi . |
(4.1) |
i =1
Mathematical expectation of a random quantity is a constant showing what value of the random quantity should be expected (on the average) during tests or observations.
We shall discuss the most important properties of mathematical expectation.
1.Mathematical expectation of a constant is equal to this constant: М(k) = k, where k = const.
2.Mathematical expectation of the sum (the difference) of a finite number of random quantities is equal to the sum (the difference) of the mathematical expectations thereof:
М(Х ± Y) = М(Х) ± М(Y).
158

3. Mathematical expectation of the product of a finite number of independent random quantities is equal to the product of the mathematical expectations thereof:
М(ХY) = М(Х)М(Y).
4. Reducing (increasing) all values of the random quantity Х by K = const gives the mathematical expectation a decrease (an increase) by K:
М(Х – K ) = М(Х) – K .
Variance of random quantity
The most commonly encountered measure (characteristic) of a deviation or spreading (scatter) of a random quantity is variance and the root-mean- square deviation (RMSD) obtained from this.
As the scatter (deviation) of a random quantity’s values from the mathematical expectation cannot be evidently characterized by linear differences, the adopted practice is to estimate deviation squares.
Therefore we will call the variance of the random quantity Х the mathematical expectation for the square of its value deviation from the mathematical expectation:
D( X ) = y2 ( X ) = M [X − M ( X )]2 , |
(4.2) |
for discrete random quantities we have: |
|
D(X ) = ∑(xi − a )2 pi , |
(4.3) |
where а = М(Х).
The RMSD is equal to:
y( X ) = |
D(x) |
. |
(4.4) |
The variance of a random quantity is a constant. If the variance is small, all numbers of the sum are also small (4.3). So if хi exists that deviates widely from а, these are unlikely. If the variance is great, this indicates to a big probability of the random quantity values being very remote from а.
159

The average root-mean-square deviation σ ( X ) is convenient in that, while characterizing the deviation (spreading) degree, it has the dimension of the quantity measured.
Let us look into the basic properties of variance.
1.The variance of a constant is equal to zero: D(k) = 0; k = const.
2.D(kx) = k 2 D(x) , where k = const.
3.The variance of a random quantity is equal to the mathematical expectation of its square with no mathematical expectation square:
D( X ) = M ( X 2 ) − M 2 ( X ) . |
(4.5) |
4. The variance of a sum (a difference) of independent random quantities is equal to the sum of the variances thereof:
D( X ± Y ) = D( X ) + D(Y ) . |
(4.6) |
It follows from 4 that the average standard deviation of the sum of independent random quantities is equal to the root of the sum of squares for the RMS deviations thereof:
σ (x) = |
n |
|
∑σ i2 . |
(4.7) |
|
|
i =1 |
|
Consider n similarly distributed random quantities:
if x1, x2 , x3 ,..., xn are similarly distributed random quantities, the mathematical expectation for each of these being equal to а, the mathematical expectation for the sum thereof is equal to na, and the mathematical expectation of the arithmetic mean is equal to а:
M |
x1 + x2 + ... + xn |
|
= |
1 |
M (x1 + x2 |
+ ... + xn ) = |
1 |
na = a ; |
|||
|
|
|
|||||||||
|
n |
|
|
|
n |
|
|
n |
|||
if x1, x2 , x3 ,..., xn |
are |
similarly |
distributed independent random |
quantities, the variance for each of these being σ 2 , the variance of the sum
thereof is nσ 2 , and the variance of the arithmetic mean is σ 2 : n
160