
aswath_damodaran-investment_philosophies_2012
.pdf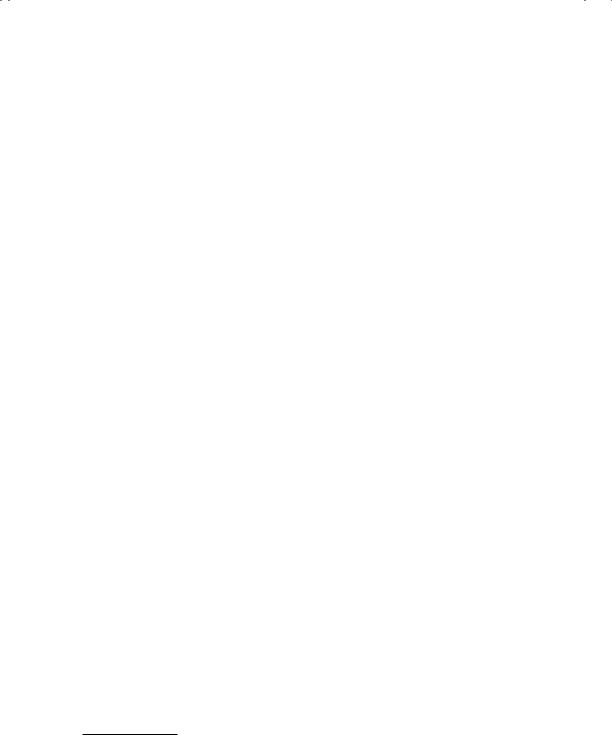
30 |
INVESTMENT PHILOSOPHIES |
and earnings potential to make an assessment of whether it is a good investment. If your analysis leads you to conclude that you can earn a higher return than your hurdle rate, you have a good investment. In other words, a great investment can come with a high beta (because the return that you expect to earn on that investment is much higher than what you would require, given its risk), and awful investments can have low betas.
A l t e r n a t i v e s t o t h e C a p i t a l A s s e t P r i c i n g M o d e l The restrictive assumptions on transaction costs and private information in the capital asset pricing model and the model’s dependence on the market portfolio have long been viewed with skepticism by both academics and practitioners. Within the confines of economic theory, there are two alternatives to the CAPM that have been developed over time.
1.Arbitrage pricing model. To understand the arbitrage pricing model, we need to begin with a definition of arbitrage. The basic idea is a simple one. Two portfolios or assets with the same exposure to market risk should be priced to earn exactly the same expected returns. If they are not, you could buy the less expensive portfolio, sell the more expensive portfolio, have no risk exposure, and earn a return that exceeds the riskless rate. This is arbitrage. If you assume that arbitrage is not possible and that investors are diversified, you can show that the expected return on an investment should be a function of its exposure to market risk. While this statement mirrors what was stated in the capital asset pricing model, the arbitrage pricing model does not make the restrictive assumptions about transaction costs and private information that lead to the conclusion that one beta can capture an investment’s entire exposure to market risk.8 Instead, in the arbitrage pricing model you can have multiple sources of market risk and different exposures (betas) to each market risk, and your expected return on an investment can be written as:
Expected return = Risk-free rate
+Beta for factor 1(Risk premium for factor 1)
+Beta for factor 2(Risk premium for factor 2) · · ·
+Beta for factor n(Risk premium for factor n)
8Stephen A. Ross, “The Arbitrage Theory of Capital Asset Pricing,” Journal of Economic Theory 13, no. 3 (1976): 341–360.
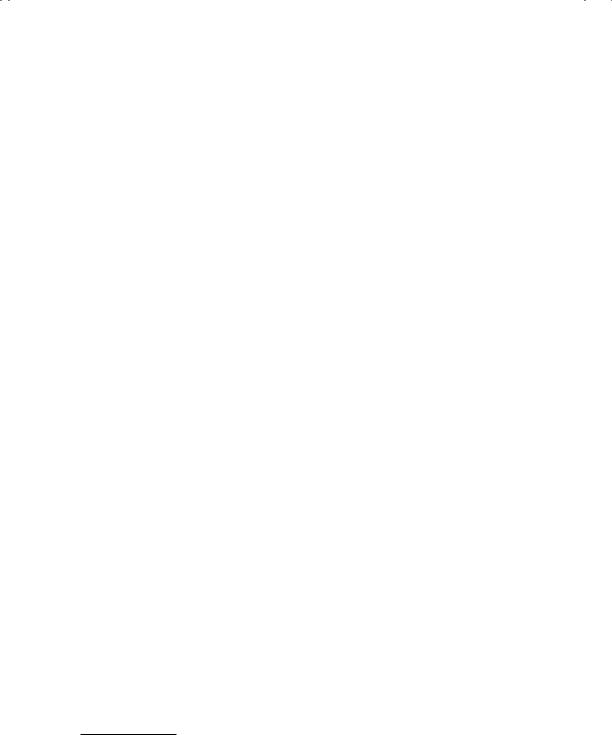
Upside, Downside: Understanding Risk |
31 |
The practical questions then become knowing how many factors there are that determine expected returns and what the betas for each investment are against these factors. The arbitrage pricing model estimates both by scanning past data on stock returns for common patterns (since market risk affects most or all stocks at a given time) and estimating each stock’s exposure to these patterns in a process called factor analysis. A factor analysis provides two output measures:
1.It specifies the number of common factors that affected the historical return data.
2.It measures the beta of each investment relative to each of the common factors and provides an estimate of the actual risk premium earned by each factor.
The factor analysis does not, however, identify the factors in economic terms—the factors remain unnamed. In summary, in the arbitrage pricing model, the market risk is measured relative to multiple unspecified macroeconomic variables, with the sensitivity of the investment relative to each factor being measured by a beta. The number of factors, the factor betas, and the factor risk premiums can all be estimated using the factor analysis.
2.Multifactor models for risk and return. The arbitrage pricing model’s failure to identify the factors specifically in the model may be a statistical strength, but it is an intuitive weakness. The solution seems simple: replace the unidentified statistical factors with specific economic factors, and the resultant model should have an economic basis while still retaining much of the strength of the arbitrage pricing model. That is precisely what multifactor models try to do. Multifactor models generally are built from historical data, rather than economic theory. Once the number of factors has been identified in the arbitrage pricing model, the behavior of these factors over time can be extracted from the data. The behavior of the unnamed factors over time can then be compared to the behavior of macroeconomic variables over that same period to see whether any of the variables are correlated, over time, with the identified factors.
For instance, Chen, Roll, and Ross suggest that the following macroeconomic variables are highly correlated with the factors that come out of factor analysis: industrial production, changes in default premium, shifts in the term structure, unanticipated inflation, and changes in the real rate of return.9 These variables can then be correlated
9N. Chen, R. Roll, and S. A. Ross, “Economic Forces and the Stock Market,” Journal of Business 59 (1986): 383–404.
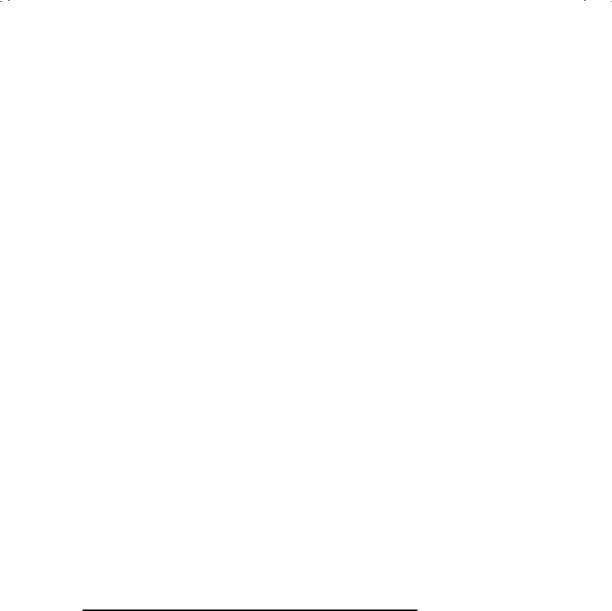
32 |
INVESTMENT PHILOSOPHIES |
with returns to come up with a model of expected returns, with firmspecific betas calculated relative to each variable.
E(R) = Rf + GNP[E(RGNP) − Rf ] + I [E(RI ) − Rf ] + · · ·
+ ∂ [E(R∂ ) − Rf ]
where GNP = Beta relative to changes in industrial production E(RGNP) = Expected return on a portfolio with a beta of 1 on
the industrial production factor and zero on all other factors
I = Beta relative to changes in inflation
E(RI) = Expected return on a portfolio with a beta of 1 on the inflation factor and zero on all other factors
The costs of going from the arbitrage pricing model to a macroeconomic multifactor model can be traced directly to the errors that can be made in identifying the factors. The economic factors in the model can change over time, as will the risk premiums associated with each one. For instance, oil price changes were a significant economic factor driving expected returns on stocks in the 1970s but were not significant in subsequent time periods. Using the wrong factor or missing a significant factor in a multifactor model can lead to inferior estimates of expected return.
In summary, multifactor models, like the arbitrage pricing model, assume that market risk can be captured best using multiple macroeconomic factors and estimating betas relative to each. Unlike the arbitrage pricing model, multifactor models do attempt to identify the macroeconomic factors that drive market risk.
A S S E S S I N G C O N V E N T I O N A L R I S K
A N D R E T U R N M O D E L S
All the risk and return models developed hitherto in this chapter are built on a common foundation. They all assume that only market risk is rewarded and they derive the expected return as a function of measures of this risk. The capital asset pricing model makes the most restrictive assumptions about how markets work but arrives at the simplest model, with only one factor driving risk and requiring estimation. The arbitrage pricing model makes fewer assumptions but arrives at a more complicated model, at least in terms of the parameters that require estimation. The capital asset pricing model
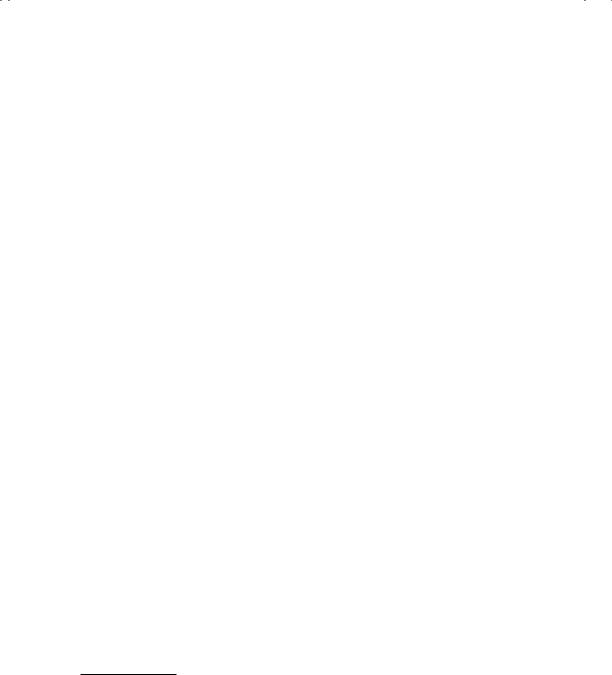
Upside, Downside: Understanding Risk |
33 |
can be considered a special case of the arbitrage pricing model, where there is only one underlying factor and it is completely captured by the market index. In general, the CAPM has the advantage of being a simpler model to estimate and to use, but it will underperform the richer multifactor models when an investment is sensitive to economic factors not well represented in the market index. For instance, oil company stocks, which derive most of their risk from oil price movements, tend to have low CAPM betas and low expected returns. An arbitrage pricing model, where one of the factors may measure oil and other commodity price movements, will yield a better estimate of risk and a higher expected return for these firms.10
Notwithstanding the impressive economic underpinnings of these models for risk, key questions remain: Do these models work at measuring what they claim to measure? Should investors assess the betas of investments and use them in investment decisions? Are there alternative measures of risk that are less dependent upon stock prices? The answers to these questions have been debated widely among academics and practitioners in the past two decades. The first tests of the CAPM suggested that betas and returns were positively related, though other measures of risk (such as variance in stock prices) continued to explain differences in actual returns. This discrepancy was initially attributed to limitations in the testing techniques, but Fama and French examined the relationship between betas and returns between 1962 and 1989 and concluded that there is little or no relationship between the two.11 While the early tests of the arbitrage pricing model and multifactor models suggested that they might provide more promise in terms of explaining differences in returns, a distinction has to be drawn between the use of these models to explain differences in past returns and their use to predict expected returns in the future. The competitors to the CAPM clearly do a much better job at explaining past returns since they do not constrain themselves to one factor, as the CAPM does. This extension to multiple factors does become more of a problem when we try to forecast expected returns for the future, since the betas and premiums of each of these factors now have to be estimated. Because the factor premiums and betas are themselves volatile, the estimation error may eliminate the benefits that could be gained by moving from the CAPM to more complex models.
10Weston and Copeland used both approaches to estimate the cost of equity for oil companies in 1989 and came up with 14.4 percent with the CAPM and 19.1 percent using the arbitrage pricing model.
11E. F. Fama and K. R. French, “The Cross-Section of Expected Returns,” Journal of Finance 47 (1992): 427–466.
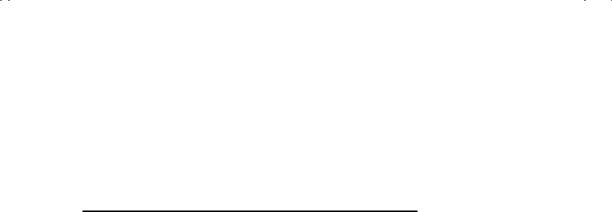
34 |
INVESTMENT PHILOSOPHIES |
In summary, after 60 years of research, we are still faced with a quandary. Our models for risk are flawed, and we estimate the risk parameters within each of these models with substantial error. In the next section, we look at alternatives to conventional risk and return models.
E Q U I T Y R I S K : A L T E R N A T I V E M E A S U R E S
The discomfort that practitioners and many academics feel with the theorybased risk and return models described in the preceding section has several roots. First, by starting with the premise that risk is symmetric—the upside and downside are balanced—it already seems to concede the fight to beat the market. After all, a good investment should have more upside than downside; value investors in particular build their investment strategies around the ethos of minimizing downside risk while expanding upside potential. Second, the model’s dependence upon past market prices to get a measure of risk (betas come from regressions) should make anyone wary: after all, markets are often volatile for no good fundamental reason. Third, the focus on breaking down risk into diversifiable and undiversifiable risk, with only the latter being relevant for investors, does not convince some, who believe that the distinction is meaningless or should not be made.
In this section, we look at alternative approaches to assessing risk in investments. The first approach draws on information in accounting statements to evaluate the risk in a business. The second and the third approaches draw on the assumption that the market prices assets based on their risk, at least on average and over the long term. In the second, we look at the shared characteristics of companies that have generated high returns in the past, and use these characteristics as proxies for risk. In the third, we back out the returns implied in current stock prices and use these expected returns as measures of risk: riskier investments will have higher expected returns. In the fourth approach, we look at the option of adjusting cash flows for risk, either subjectively or with a model-based approach, rather than adjusting expected returns for risk. Finally, we look at building risk into a margin of safety (MOS) when making the decision on whether to buy a stock.
A c c o u n t i n g - B a s e d M e a s u r e s
For those who are inherently suspicious of any market-based measure, there is always accounting information that can be used to come up with a measure of risk. In particular, firms that have low debt ratios, high dividends, stable and growing earnings, and large cash holdings should be less risky to

Upside, Downside: Understanding Risk |
35 |
equity investors than firms without these characteristics. While the intuition is impeccable, converting it into an expected return can be problematic; however, here are three choices:
1.Pick one accounting ratio and create scaled risk measures around that ratio. Assume that you are assessing the risk in 3M. The median book debt-to-capital ratio for U.S. companies at the start of 2011 was 51 percent. The book debt-to-capital ratio for 3M at that time was 30.91 percent, yielding a relative risk measure of 0.61 for the company, obtained by dividing 3M’s debt ratio (30.91 percent) by the market average (51 percent). The perils of this approach should be clear when applied to Apple, since the firm has no debt outstanding, yielding a relative risk of zero (which is an absurd result).
2.Compute an accounting beta. Rather than estimate a beta from market prices, an accounting beta is estimated from accounting numbers. One simple approach is to look at changes in accounting earnings at a firm relative to accounting earnings for the entire market. Firms that have more stable earnings than the rest of the market or whose earnings movements have nothing to do with the rest of the market will have low accounting betas. An extended version of this approach would be to estimate the accounting beta as a function of multiple accounting variables, including dividend payout ratios, debt ratios, cash balances, and earnings stability for the entire market. Plugging the values for an individual company into this regression will yield an accounting beta for the firm. While this approach looks promising, here are a couple of cautionary notes: accounting numbers are smoothed out and can hide risk, and they are estimated at most four times a year (as opposed to market numbers, which get minute-by-minute updates).
3.Use screens. If you are unconvinced by either of the first two approaches, the most flexible approach for incorporating accounting information into investment decisions is to screen companies that meet pre-specified screens, and choose only those among those firms that pass these screens. Thus, you may decide that you will invest only in firms that trade at less than book value, have returns on equity that exceed 20 percent, have no debt and whose earnings have grown at least 10 percent a year for the last 5 years.
In summary, with accounting risk measures, the risk measure is related to a company’s fundamentals, which seems more in keeping with an intrinsic valuation view of the world. On the flip side, accounting numbers can be misleading, and the estimates can have significant errors associated with them.

36 |
INVESTMENT PHILOSOPHIES |
P r o x y M o d e l s
The conventional models for risk and return in finance (CAPM, the arbitrage pricing model, and even multifactor models) start by making assumptions about how investors behave and how markets work to derive models that measure risk and link those measures to expected returns. While these models have the advantage of having a foundation in economic theory, they seem to fall short in explaining differences in returns across investments. The reasons for the failure of these models run the gamut: the assumptions made about markets are unrealistic (no transaction costs, perfect information) and investors don’t behave rationally (and behavioral finance research provides ample evidence of this).
With proxy models, we essentially give up on building risk and return models from economic theory. Instead, we start by looking at the actual returns earned by different investments and relate returns earned to observable variables. In the earlier-referenced study by Fama and French, they examined returns earned by individual stocks from 1962 to 1989, looking for company-specific variables that did a better job of explaining return differences across firms than betas, and pinpointed two variables: the market capitalization of a firm and its price-to-book ratio (the ratio of market capitalization to accounting book value for equity). Specifically, they concluded that small market cap stocks earned much higher annual returns than large market cap stocks and that low price-to-book ratio stocks earned much higher annual returns than stocks that traded at high price-to-book ratios. Rather than view this as evidence of market inefficiency, which is what prior studies that had found the same phenomena had done, they argued that if these stocks earned higher returns over long time periods, they must be riskier than stocks that earned lower returns. In effect, market capitalization and price-to-book ratios were better proxies for risk, according to their reasoning, than betas. In fact, they regressed returns on stocks against the market capitalization of a company and its price-to-book ratio to arrive at the following regression for U.S. stocks:
Expected monthly return = 1.77%
− 0.11[ln(Market capitalization in millions)]
+ 0.35[ln(Book-to-price ratio)]
In a pure proxy model, you could plug the market capitalization and book-to-price ratio for any company into this regression to get expected returns.
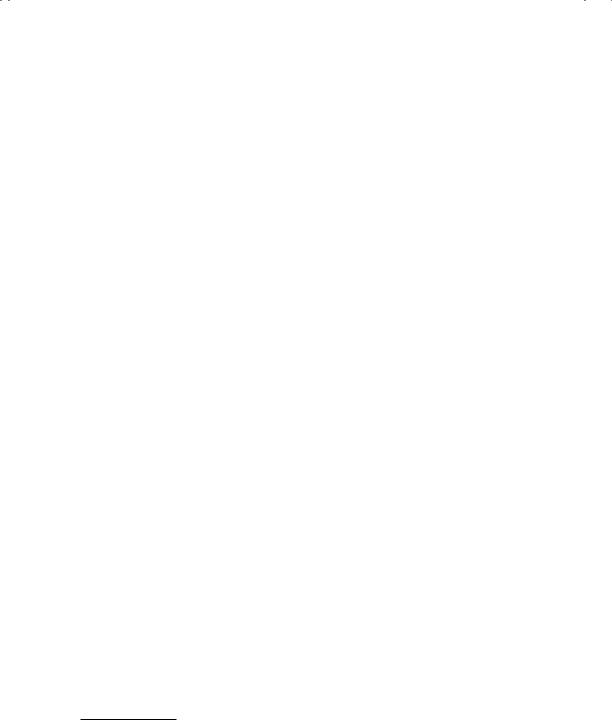
Upside, Downside: Understanding Risk |
37 |
In the two decades since the Fama-French paper brought proxy models to the fore, researchers have probed the data (which has become more detailed over time) to find better and additional proxies for risk. Three of the proxies are:
1.Earnings momentum. Equity research analysts will find vindication in research that seems to indicate that companies that have reported stronger than expected earnings growth in the past earn higher returns than the rest of the market.
2.Price momentum. Chartists will smile when they read this, but researchers have concluded that price momentum carries over into future periods. Thus, the expected returns will be higher for stocks that have outperformed markets in recent time periods and lower for stocks that have lagged.
3.Liquidity. In a nod to real-world costs, there seems to be clear evidence that stocks that are less liquid (lower trading volume, higher bid-ask spreads) earn higher returns than more liquid stocks.
Many analysts have melded the CAPM with proxy models to create composite or melded models. For instance, analysts who value small companies derive expected returns for these companies by adding a small cap premium to the CAPM expected return:
Expected return = Risk-free rate + Market beta Equity risk premium
+ Small cap premium
The threshold for small capitalization varies across time but is generally set at the bottom decile of publicly traded companies and the small cap premium itself is estimated by looking at the historical premium earned by small-cap stocks over the market. In a 2012 paper on equity risk premiums, I estimate that companies in the bottom market cap decile earned 4.64 percent more than the overall market between 1928 and 2011.12 Thus, the expected return (cost of equity) for a small-cap company with a beta of 1.20 (using a risk-free rate of 2% and an equity risk premium of 6%) would be:
Expected return = 2% + 1.2(6%) + 4.64% = 13.84%
12A. Damodaran, “Equity Risk Premiums: Determinants, Estimation and Implications—The 2012 Edition” (SSRN Working Paper, http://papers.ssrn.com/ sol3/papers.cfm?abstract_id=2027211).

38 |
INVESTMENT PHILOSOPHIES |
In an alternate strategy, using the Fama-French findings, the CAPM has been expanded to include market capitalization and price-to-book ratios as additional variables, with the expected return stated as:
Expected return = Risk-free rate + Market beta Equity risk premium
+Size beta Small cap risk premium
+Book-to-market beta Book-to-market premium
The size factor and the book-to-market betas are estimated by regressing a stock’s returns against the size premium and book-to-market premiums over time; this is analogous to the way we get the market beta, by regressing stock returns against overall market returns.
While the use of proxy and melded models offers a way of adjusting expected returns to reflect market reality, there are three dangers in using these models.
1.Data mining. As the amount of data that we have on companies increases and becomes more accessible, it is inevitable that we will find more variables that are related to returns. It is also likely that most of these variables are not proxies for risk and that the correlation is a function of the time period that we look at. In effect, proxy models are statistical models and not economic models. Thus, there is no easy way to separate the variables that matter from those that do not.
2.Standard error. Since proxy models come from looking at historical data, they are burdened by the noise in the data. Stock returns are extremely volatile over time, and any historical premiums that we compute (for market capitalization or any other variable) are going to have significant standard errors. For instance, the small cap premium of 4.64 percent between 1928 and 2011 has a standard error of 2.01 percent; put simply, the true premium may be less than 1 percent or higher than 7 percent. The standard errors on the size and book-to-market betas in the three-factor Fama-French model are so large that using them in practice creates more noise as it adds in precision.
3.Pricing error or risk proxy. For decades, value investors have argued that you should invest in stocks with low price-earnings (P/E) ratios that trade at low multiples of book value and have high dividend yields, pointing to the fact that you will earn higher returns by doing so. In fact, a scan of Benjamin Graham’s screens from Security Analysis for cheap companies unearths most of the proxies that you see in use today. Proxy models incorporate all of these variables into the expected return and thus render these assets to be fairly priced. Using the circular logic of these models, markets are always efficient because any inefficiency that exists is just another risk proxy that needs to get built into the model.

Upside, Downside: Understanding Risk |
39 |
There is a broader critique of this approach. If you believe that smallcap stocks are riskier than large-cap stocks, you have an obligation to think of fundamental or economic reasons why and build those into your risk and return model or into the parameters of the model. Adding a small cap premium is not only a sloppy (and high-error) way of adjusting expected returns but it is an abdication of the mission in intrinsic valuation, which is to build up your numbers from fundamentals.
M a r k e t - I m p l i e d M e a s u r e s
As you can see from each of the alternatives laid out, there are assumptions underlying each alternative that can make users uncomfortable. So, what if you want to estimate a model-free cost of equity? There is a choice, but it comes with a catch. To see the choice, assume that you have a stock that has an expected annual dividend of $3 per share next year, with growth at 4 percent a year, and that the stock trades at $60. Using a very simple dividend discount model, you can back out the cost of equity for this company from the existing stock price:
Value of stock = Dividends next year/(Cost of equity − Growth rate)
$60 = $3.00/(Cost of equity − 4%)
Cost of equity = 9%
The mechanics of computing implied cost of equity become messier as you go from dividends to estimated cash flows and from stable-growth models to high-growth models, but the principle remains the same. You can use the current stock price and solve for the cost of equity. This cost of equity is a market-implied cost of equity. If you were required to value this company, though, using this cost of equity to value the stock would be pointless since you would arrive at a value of $60 and the not surprising conclusion that the stock is fairly priced.
So, what point is there to computing an implied cost of equity? There are three ways of incorporating these numbers into investing/valuation.
1.One is to use a conventional cost of equity in the valuation and to compare the market-implied cost of equity to the conventional one to see how much margin for error you have in your estimate. Thus, if you find your stock to be undervalued with an 8 percent cost of equity, but the implied cost of equity is 8.5 percent, you may very well decide not to buy the stock because your margin for error is too narrow; with an implied cost of equity of 14 percent, you may be more comfortable buying the stock. Think of it as a marriage of discounted cash flow valuation with a margin of safety.