
- •Preface
- •Contents
- •Contributors
- •Modeling Meaning Associated with Documental Entities: Introducing the Brussels Quantum Approach
- •1 Introduction
- •2 The Double-Slit Experiment
- •3 Interrogative Processes
- •4 Modeling the QWeb
- •5 Adding Context
- •6 Conclusion
- •Appendix 1: Interference Plus Context Effects
- •Appendix 2: Meaning Bond
- •References
- •1 Introduction
- •2 Bell Test in the Problem of Cognitive Semantic Information Retrieval
- •2.1 Bell Inequality and Its Interpretation
- •2.2 Bell Test in Semantic Retrieving
- •3 Results
- •References
- •1 Introduction
- •2 Basics of Quantum Probability Theory
- •3 Steps to Build an HSM Model
- •3.1 How to Determine the Compatibility Relations
- •3.2 How to Determine the Dimension
- •3.5 Compute the Choice Probabilities
- •3.6 Estimate Model Parameters, Compare and Test Models
- •4 Computer Programs
- •5 Concluding Comments
- •References
- •Basics of Quantum Theory for Quantum-Like Modeling Information Retrieval
- •1 Introduction
- •3 Quantum Mathematics
- •3.1 Hermitian Operators in Hilbert Space
- •3.2 Pure and Mixed States: Normalized Vectors and Density Operators
- •4 Quantum Mechanics: Postulates
- •5 Compatible and Incompatible Observables
- •5.1 Post-Measurement State From the Projection Postulate
- •6 Interpretations of Quantum Mechanics
- •6.1 Ensemble and Individual Interpretations
- •6.2 Information Interpretations
- •7 Quantum Conditional (Transition) Probability
- •9 Formula of Total Probability with the Interference Term
- •9.1 Växjö (Realist Ensemble Contextual) Interpretation of Quantum Mechanics
- •10 Quantum Logic
- •11 Space of Square Integrable Functions as a State Space
- •12 Operation of Tensor Product
- •14 Qubit
- •15 Entanglement
- •References
- •1 Introduction
- •2 Background
- •2.1 Distributional Hypothesis
- •2.2 A Brief History of Word Embedding
- •3 Applications of Word Embedding
- •3.1 Word-Level Applications
- •3.2 Sentence-Level Application
- •3.3 Sentence-Pair Level Application
- •3.4 Seq2seq Application
- •3.5 Evaluation
- •4 Reconsidering Word Embedding
- •4.1 Limitations
- •4.2 Trends
- •4.4 Towards Dynamic Word Embedding
- •5 Conclusion
- •References
- •1 Introduction
- •2 Motivating Example: Car Dealership
- •3 Modelling Elementary Data Types
- •3.1 Orthogonal Data Types
- •3.2 Non-orthogonal Data Types
- •4 Data Type Construction
- •5 Quantum-Based Data Type Constructors
- •5.1 Tuple Data Type Constructor
- •5.2 Set Data Type Constructor
- •6 Conclusion
- •References
- •Incorporating Weights into a Quantum-Logic-Based Query Language
- •1 Introduction
- •2 A Motivating Example
- •5 Logic-Based Weighting
- •6 Related Work
- •7 Conclusion
- •References
- •Searching for Information with Meet and Join Operators
- •1 Introduction
- •2 Background
- •2.1 Vector Spaces
- •2.2 Sets Versus Vector Spaces
- •2.3 The Boolean Model for IR
- •2.5 The Probabilistic Models
- •3 Meet and Join
- •4 Structures of a Query-by-Theme Language
- •4.1 Features and Terms
- •4.2 Themes
- •4.3 Document Ranking
- •4.4 Meet and Join Operators
- •5 Implementation of a Query-by-Theme Language
- •6 Related Work
- •7 Discussion and Future Work
- •References
- •Index
- •Preface
- •Organization
- •Contents
- •Fundamentals
- •Why Should We Use Quantum Theory?
- •1 Introduction
- •2 On the Human Science/Natural Science Issue
- •3 The Human Roots of Quantum Science
- •4 Qualitative Parallels Between Quantum Theory and the Human Sciences
- •5 Early Quantitative Applications of Quantum Theory to the Human Sciences
- •6 Epilogue
- •References
- •Quantum Cognition
- •1 Introduction
- •2 The Quantum Persuasion Approach
- •3 Experimental Design
- •3.1 Testing for Perspective Incompatibility
- •3.2 Quantum Persuasion
- •3.3 Predictions
- •4 Results
- •4.1 Descriptive Statistics
- •4.2 Data Analysis
- •4.3 Interpretation
- •5 Discussion and Concluding Remarks
- •References
- •1 Introduction
- •2 A Probabilistic Fusion Model of Trust
- •3 Contextuality
- •4 Experiment
- •4.1 Subjects
- •4.2 Design and Materials
- •4.3 Procedure
- •4.4 Results
- •4.5 Discussion
- •5 Summary and Conclusions
- •References
- •Probabilistic Programs for Investigating Contextuality in Human Information Processing
- •1 Introduction
- •2 A Framework for Determining Contextuality in Human Information Processing
- •3 Using Probabilistic Programs to Simulate Bell Scenario Experiments
- •References
- •1 Familiarity and Recollection, Verbatim and Gist
- •2 True Memory, False Memory, over Distributed Memory
- •3 The Hamiltonian Based QEM Model
- •4 Data and Prediction
- •5 Discussion
- •References
- •Decision-Making
- •1 Introduction
- •1.2 Two Stage Gambling Game
- •2 Quantum Probabilities and Waves
- •2.1 Intensity Waves
- •2.2 The Law of Balance and Probability Waves
- •2.3 Probability Waves
- •3 Law of Maximal Uncertainty
- •3.1 Principle of Entropy
- •3.2 Mirror Principle
- •4 Conclusion
- •References
- •1 Introduction
- •4 Quantum-Like Bayesian Networks
- •7.1 Results and Discussion
- •8 Conclusion
- •References
- •Cybernetics and AI
- •1 Introduction
- •2 Modeling of the Vehicle
- •2.1 Introduction to Braitenberg Vehicles
- •2.2 Quantum Approach for BV Decision Making
- •3 Topics in Eigenlogic
- •3.1 The Eigenlogic Operators
- •3.2 Incorporation of Fuzzy Logic
- •4 BV Quantum Robot Simulation Results
- •4.1 Simulation Environment
- •5 Quantum Wheel of Emotions
- •6 Discussion and Conclusion
- •7 Credits and Acknowledgements
- •References
- •1 Introduction
- •2.1 What Is Intelligence?
- •2.2 Human Intelligence and Quantum Cognition
- •2.3 In Search of the General Principles of Intelligence
- •3 Towards a Moral Test
- •4 Compositional Quantum Cognition
- •4.1 Categorical Compositional Model of Meaning
- •4.2 Proof of Concept: Compositional Quantum Cognition
- •5 Implementation of a Moral Test
- •5.2 Step II: A Toy Example, Moral Dilemmas and Context Effects
- •5.4 Step IV. Application for AI
- •6 Discussion and Conclusion
- •Appendix A: Example of a Moral Dilemma
- •References
- •Probability and Beyond
- •1 Introduction
- •2 The Theory of Density Hypercubes
- •2.1 Construction of the Theory
- •2.2 Component Symmetries
- •2.3 Normalisation and Causality
- •3 Decoherence and Hyper-decoherence
- •3.1 Decoherence to Classical Theory
- •4 Higher Order Interference
- •5 Conclusions
- •A Proofs
- •References
- •Information Retrieval
- •1 Introduction
- •2 Related Work
- •3 Quantum Entanglement and Bell Inequality
- •5 Experiment Settings
- •5.1 Dataset
- •5.3 Experimental Procedure
- •6 Results and Discussion
- •7 Conclusion
- •A Appendix
- •References
- •Investigating Bell Inequalities for Multidimensional Relevance Judgments in Information Retrieval
- •1 Introduction
- •2 Quantifying Relevance Dimensions
- •3 Deriving a Bell Inequality for Documents
- •3.1 CHSH Inequality
- •3.2 CHSH Inequality for Documents Using the Trace Method
- •4 Experiment and Results
- •5 Conclusion and Future Work
- •A Appendix
- •References
- •Short Paper
- •An Update on Updating
- •References
- •Author Index
- •The Sure Thing principle, the Disjunction Effect and the Law of Total Probability
- •Material and methods
- •Experimental results.
- •Experiment 1
- •Experiment 2
- •More versus less risk averse participants
- •Theoretical analysis
- •Shared features of the theoretical models
- •The Markov model
- •The quantum-like model
- •Logistic model
- •Theoretical model performance
- •Model comparison for risk attitude partitioning.
- •Discussion
- •Authors contributions
- •Ethical clearance
- •Funding
- •Acknowledgements
- •References
- •Markov versus quantum dynamic models of belief change during evidence monitoring
- •Results
- •Model comparisons.
- •Discussion
- •Methods
- •Participants.
- •Task.
- •Procedure.
- •Mathematical Models.
- •Acknowledgements
- •New Developments for Value-based Decisions
- •Context Effects in Preferential Choice
- •Comparison of Model Mechanisms
- •Qualitative Empirical Comparisons
- •Quantitative Empirical Comparisons
- •Neural Mechanisms of Value Accumulation
- •Neuroimaging Studies of Context Effects and Attribute-Wise Decision Processes
- •Concluding Remarks
- •Acknowledgments
- •References
- •Comparison of Markov versus quantum dynamical models of human decision making
- •CONFLICT OF INTEREST
- •Endnotes
- •FURTHER READING
- •REFERENCES
suai.ru/our-contacts |
quantum machine learning |
Balanced Quantum-Like Model for Decision Making |
81 |
Table 1. Experimental results obtained in four di erent works of the literature for the prisoner’s dilemma game. The column p(¬y|¬x) corresponds to the probability of def ecting given that it is known that the other participant chose to def ect. The column p(¬y|x) corresponds to the probability of def ecting given that it is known that the other participant chose to cooperate. Finally, the column psub (¬y) corresponds to the subjective probability of the second participant choosing the def ect action given there is no information present about knowing if prisoner x cooperates or defects. The column p(¬y) corresponds to the classical probability.
Experiment |
p(¬y|¬x) |
p(¬y|x) |
psub (¬y) |
p(¬y) |
(a) [19] |
0.97 |
0.84 |
0.63 |
0.9050 |
|
|
|
|
|
(b) [17] |
0.82 |
0.77 |
0.72 |
0.7950 |
|
|
|
|
|
(c) [3] |
0.91 |
0.84 |
0.66 |
0.8750 |
|
|
|
|
|
(d) [10] |
0.97 |
0.93 |
0.88 |
0.9500 |
|
|
|
|
|
(e) Average |
0.92 |
0.85 |
0.72 |
0.8813 |
|
|
|
|
|
1.2Two Stage Gambling Game
In the two stage gambling game the participants were asked whether they want to play a gamble that has an equal chance of winning p(x) = 0.5 or losing p(¬x) = 0.5 [18]. The participants of the experiment were asked three di erent questions.
–What is the probability that they play the gamble y if they had lost the first gamble x, p(y|¬x)
–What is the probability that they play the gamble y if they had won the first gamble x, p(y|x)
–What is the probability that they play the gamble y given there is no information present knowing if they had won the first gamble x. This would by the law of total probability
p(y) = p(y|x) · p(x) + p(y|¬x) · p(¬x) |
(2) |
In Table 2, we summarise the results of several experiments of the literature concerned with the two stage gambling game.
2 Quantum Probabilities and Waves
Beside quantum cognition quantum physics was the only branch in science that evaluated a probability p(x) an state x as the mode-square of a probability amplitude A(x) represented by a complex number
p(x) = |A(x)|2 = A(x) 2 = A(x) · A(x). |
(3) |
This is because the product of complex number with its conjugate is always a real number. With
A(x) = α + β · i |
(4) |

suai.ru/our-contacts |
quantum machine learning |
82 A. Wichert and C. Moreira
Table 2. Experimental results obtained in three di erent works of the literature indicating the probability of a player choosing to make a second gamble for the two stage gambling game. The column p(y|¬x) corresponds to the probability when the outcome of the first gamble is known to be lose. The column p(y|x) corresponds to the probability when the outcome of the first gamble is known to be win. Finally, the column psub (y) corresponds to the subjective probability when the outcome of the first gamble is not known. The column p(y) corresponds to the classical probability.
|
Experiment |
p(y|¬x) |
p(y|x) |
psub (y) |
p(y) |
|
|
|
(i) [20] |
0.58 |
0.69 |
0.37 |
0.6350 |
|
|
|
|
|
|
|
|
|
|
|
(ii) [15] |
0.47 |
0.72 |
0.48 |
0.5950 |
|
|
|
|
|
|
|
|
|
|
|
(iii) [16] |
0.45 |
0.63 |
0.41 |
0.5400 |
|
|
|
|
|
|
|
|
|
|
|
(iv) Average |
0.50 |
0.68 |
0.42 |
0.5900 |
|
|
|
|
|
|
|
|
|
|
A(x) · A(x) = (α − β · i) · (α + β · i) = α2 + β2 = |A(x)|2. |
(5) |
Quantum physics by itself does not o er any justification or explanation beside the statement that it just works fine [2]. We can map the classical probabilities into amplitudes using the polar coordinate representation
a(x, θ1) = p(x) · ei·θ1 = A(x), a(y, θ2) = p(y) · ei·θ2 = A(y). (6)
The amplitudes represented in polar coordinate form contains a new free parameter θ, which corresponds to the phase of the wave.
2.1 Intensity Waves |
|
|
|
|
|
|
|
|
|
|
The intensity wave is defined as |
|
|
|
|
|
|
|
|
|
|
I (y, θ1, θ2) = |a(y, x, θ1) + a(y, ¬x, θ2)|2 |
(7) |
|||||||||
I (y, θ1, θ2) = p(y, x) + p(y, ¬x) + 2 · |
p(y, x) · p(y, ¬x) |
· cos(θ1 − θ2) |
(8) |
|||||||
Note that for simplification we can replace θ1 − θ2 with θ, |
|
|||||||||
θ = θ1 − θ2 |
|
|
|
|
cos(θ) |
(9) |
||||
I (y, θ) = p(y) + 2 |
|
p(y, x) |
· |
p(y, |
x) |
· |
||||
and |
· |
|
|
|
¬ |
|
|
|
||
|
|
|
|
|
|
|
|
|
|
|
I (¬y, θ¬1, θ¬2) = |a(¬y, x, θ¬1) + a(¬y, ¬x, θ¬2)|2 |
(10) |
with
θ¬ = θ¬1 − θ¬2
I (¬y, θ¬) = p(¬y) + 2 · p(¬y, x) · p(¬y, ¬x) · cos(θ¬) (11) and for certain phase values
I (y, θ) + I (¬y, θ¬) = 1. |
(12) |
In Fig. 2 (a) we see two intensity waves in relation to the phase with the parametrisation as indicated in corresponding to the values of Fig. 1 corresponding to the Table 1 values in (e).

suai.ru/our-contacts |
quantum machine learning |
|
|
Balanced Quantum-Like Model for Decision Making |
83 |
||||||
|
|
|
|
|
|
|
|
|
|
|
|
|
|
|
|
|
|
|
|
|
|
|
|
|
|
|
|
|
|
|
|
|
|
|
|
|
|
|
|
|
|
|
|
|
|
|
|
|
|
|
|
|
|
|
|
|
|
|
|
|
|
|
|
|
|
|
|
|
|
|
|
|
|
|
|
|
|
|
|
Fig. 2. (a) Two intensity waves I (y, θ), I (¬y, θ¬ ) in relation to the phase (−2 · π, 2 · π) with the parametrisation as indicated in corresponding to the values of Fig. 1. Note that the two waves oscillate around p(y) = 0.1950 and p(¬y) = 0.8050 (the two lines).
(b) The resulting probability waves as determined by the law of balance, the bigger wave is replaced by the negative smaller one.
2.2The Law of Balance and Probability Waves
Intensity waves I (y, θ) and I (¬y, θ¬) are probability waves p(y, θ) and p(¬y, θ¬) if:
1. they are positive
|
0 ≤ p(y, θ), |
0 ≤ p(¬y, θ¬); |
(13) |
2. |
they sum to one |
|
|
|
p(y, θ) + p(¬y, θ¬) = p(y) + p(¬y) = 1; |
(14) |
|
3. |
they are smaller or equal to one |
|
|
|
p(y, θ) ≤ 1, |
p(¬y, θ¬) ≤ 1. |
(15) |
Simply speaking, the law states that the bigger wave is replaced by a smaller negative one.
Probability Waves Are Positive. Since the norm is being positive or more precisely non-negative, we can represent a quadratic form by l2 norm
(a(x, θ1) + a(y, θ2) · a(x, θ1) + a(y, θ2)) = a(x, θ1) + a(y, θ2) 2
and it follows
0 ≤ a(x, θ1) + a(y, θ2) 2.
Probability Waves Sum to One According by the Law of Balance.
Instead of simple normalisation of the intensity we propose the law of balance. The interference is balanced, which means that the interference of p(y, θ) and p(¬y, θ¬) cancel each out.
|
p(y, x) · p(y, ¬x) |
· cos(θ) = − |
p(¬y, x) · p(¬y, ¬x) |
· cos(θ¬). |
(16) |
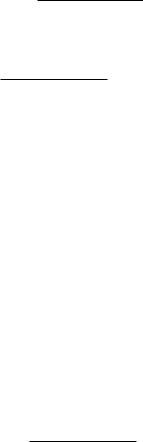
suai.ru/our-contacts |
quantum machine learning |
84 A. Wichert and C. Moreira
We can solve the Equation for the phase θ¬ or θ resulting in two possible cases. For θ¬ we get
θ |
¬ |
= cos−1 |
|
|
p(y|x) · p(y|¬x) |
· |
cos(θ) |
(17) |
||||||||||
|
− |
p( y x) |
· |
p( y |
|¬ |
x) |
|
|
||||||||||
|
|
|
|
|
|
|
¬ | |
|
¬ |
|
|
|
|
|
||||
Since cos−1(x) is only defined for x |
|
|
[ 1, 1] the Eq. 21 is valid for the constraint |
|||||||||||||||
|
|
|
|
|
|
− |
|
|
|
|
|
|
|
|
|
|
||
|
|
p(y|x) · p(y|¬x) |
≤ |
1. |
|
|
(18) |
|||||||||||
|
|
p( |
¬ |
y x) |
· |
p( y |
x) |
|
|
|
|
|
||||||
|
|
|
| |
|
|
¬ |
|¬ |
|
|
|
|
|
|
|
|
|||
corresponding to |
|
|
|
p(y) ≤ p(¬y). |
|
|
|
|
|
|
(19) |
|||||||
Since |
|
|
|
|
|
|
|
|
|
|||||||||
|
p(y|x) · p(y|¬x) ≤ p(¬y|x) · p(¬y|¬x) |
|
||||||||||||||||
|
|
|
||||||||||||||||
p(y|x) · p(y|¬x) ≤ (1 − p(y|x)) · (1 − p(y|¬x)) |
|
|||||||||||||||||
|
|
0 ≤ 1 − p(y|x) − p(y|¬x). |
|
|
|
|||||||||||||
p(y) ≤ p(¬y) is equivalent for p(x) = p(¬x) to |
|
|
|
|
|
|
|
|||||||||||
p(y|x) + p(y|¬x) ≤ (1 − p(y|x)) + (1 − p(y|¬x)) |
|
|||||||||||||||||
|
|
0 ≤ 1 − p(y|x) − p(y|¬x). |
|
|
|
|||||||||||||
The smaller probability wave determines the other probability wave as |
|
|||||||||||||||||
|
|
p(¬y, θ¬) = 1 − p(y, θ). |
|
|
(20) |
If the preceding constraint is not valid, then we solve the equation for the
phase θ. It has to be valid with |
|
|
|
|
|
|
|
|
|
|
|
|
|
|
|
|
|
|
|
|
|||||
θ = cos−1 |
|
|
p(¬y|x) · p(¬y|¬x) |
· |
cos(θ |
|
) |
(21) |
||||
− |
|
¬ |
||||||||||
|
|
p(y x) |
· |
p(y |
|¬ |
x) |
|
|
|
|||
|
|
| |
|
|
|
|
|
|
|
|||
and the constraint |
|
|
p(¬y) ≤ p(y) |
|
|
|
|
|
(22) |
|||
simplified as |
|
|
|
|
|
|
|
|||||
p(y, θ) = 1 − p(¬y, θ¬). |
|
|
|
|
(23) |
|||||||
|
|
|
|
|
For equality in the constraint p(y) = p(¬y) both cases become equal.