
- •Preface
- •Contents
- •Contributors
- •Modeling Meaning Associated with Documental Entities: Introducing the Brussels Quantum Approach
- •1 Introduction
- •2 The Double-Slit Experiment
- •3 Interrogative Processes
- •4 Modeling the QWeb
- •5 Adding Context
- •6 Conclusion
- •Appendix 1: Interference Plus Context Effects
- •Appendix 2: Meaning Bond
- •References
- •1 Introduction
- •2 Bell Test in the Problem of Cognitive Semantic Information Retrieval
- •2.1 Bell Inequality and Its Interpretation
- •2.2 Bell Test in Semantic Retrieving
- •3 Results
- •References
- •1 Introduction
- •2 Basics of Quantum Probability Theory
- •3 Steps to Build an HSM Model
- •3.1 How to Determine the Compatibility Relations
- •3.2 How to Determine the Dimension
- •3.5 Compute the Choice Probabilities
- •3.6 Estimate Model Parameters, Compare and Test Models
- •4 Computer Programs
- •5 Concluding Comments
- •References
- •Basics of Quantum Theory for Quantum-Like Modeling Information Retrieval
- •1 Introduction
- •3 Quantum Mathematics
- •3.1 Hermitian Operators in Hilbert Space
- •3.2 Pure and Mixed States: Normalized Vectors and Density Operators
- •4 Quantum Mechanics: Postulates
- •5 Compatible and Incompatible Observables
- •5.1 Post-Measurement State From the Projection Postulate
- •6 Interpretations of Quantum Mechanics
- •6.1 Ensemble and Individual Interpretations
- •6.2 Information Interpretations
- •7 Quantum Conditional (Transition) Probability
- •9 Formula of Total Probability with the Interference Term
- •9.1 Växjö (Realist Ensemble Contextual) Interpretation of Quantum Mechanics
- •10 Quantum Logic
- •11 Space of Square Integrable Functions as a State Space
- •12 Operation of Tensor Product
- •14 Qubit
- •15 Entanglement
- •References
- •1 Introduction
- •2 Background
- •2.1 Distributional Hypothesis
- •2.2 A Brief History of Word Embedding
- •3 Applications of Word Embedding
- •3.1 Word-Level Applications
- •3.2 Sentence-Level Application
- •3.3 Sentence-Pair Level Application
- •3.4 Seq2seq Application
- •3.5 Evaluation
- •4 Reconsidering Word Embedding
- •4.1 Limitations
- •4.2 Trends
- •4.4 Towards Dynamic Word Embedding
- •5 Conclusion
- •References
- •1 Introduction
- •2 Motivating Example: Car Dealership
- •3 Modelling Elementary Data Types
- •3.1 Orthogonal Data Types
- •3.2 Non-orthogonal Data Types
- •4 Data Type Construction
- •5 Quantum-Based Data Type Constructors
- •5.1 Tuple Data Type Constructor
- •5.2 Set Data Type Constructor
- •6 Conclusion
- •References
- •Incorporating Weights into a Quantum-Logic-Based Query Language
- •1 Introduction
- •2 A Motivating Example
- •5 Logic-Based Weighting
- •6 Related Work
- •7 Conclusion
- •References
- •Searching for Information with Meet and Join Operators
- •1 Introduction
- •2 Background
- •2.1 Vector Spaces
- •2.2 Sets Versus Vector Spaces
- •2.3 The Boolean Model for IR
- •2.5 The Probabilistic Models
- •3 Meet and Join
- •4 Structures of a Query-by-Theme Language
- •4.1 Features and Terms
- •4.2 Themes
- •4.3 Document Ranking
- •4.4 Meet and Join Operators
- •5 Implementation of a Query-by-Theme Language
- •6 Related Work
- •7 Discussion and Future Work
- •References
- •Index
- •Preface
- •Organization
- •Contents
- •Fundamentals
- •Why Should We Use Quantum Theory?
- •1 Introduction
- •2 On the Human Science/Natural Science Issue
- •3 The Human Roots of Quantum Science
- •4 Qualitative Parallels Between Quantum Theory and the Human Sciences
- •5 Early Quantitative Applications of Quantum Theory to the Human Sciences
- •6 Epilogue
- •References
- •Quantum Cognition
- •1 Introduction
- •2 The Quantum Persuasion Approach
- •3 Experimental Design
- •3.1 Testing for Perspective Incompatibility
- •3.2 Quantum Persuasion
- •3.3 Predictions
- •4 Results
- •4.1 Descriptive Statistics
- •4.2 Data Analysis
- •4.3 Interpretation
- •5 Discussion and Concluding Remarks
- •References
- •1 Introduction
- •2 A Probabilistic Fusion Model of Trust
- •3 Contextuality
- •4 Experiment
- •4.1 Subjects
- •4.2 Design and Materials
- •4.3 Procedure
- •4.4 Results
- •4.5 Discussion
- •5 Summary and Conclusions
- •References
- •Probabilistic Programs for Investigating Contextuality in Human Information Processing
- •1 Introduction
- •2 A Framework for Determining Contextuality in Human Information Processing
- •3 Using Probabilistic Programs to Simulate Bell Scenario Experiments
- •References
- •1 Familiarity and Recollection, Verbatim and Gist
- •2 True Memory, False Memory, over Distributed Memory
- •3 The Hamiltonian Based QEM Model
- •4 Data and Prediction
- •5 Discussion
- •References
- •Decision-Making
- •1 Introduction
- •1.2 Two Stage Gambling Game
- •2 Quantum Probabilities and Waves
- •2.1 Intensity Waves
- •2.2 The Law of Balance and Probability Waves
- •2.3 Probability Waves
- •3 Law of Maximal Uncertainty
- •3.1 Principle of Entropy
- •3.2 Mirror Principle
- •4 Conclusion
- •References
- •1 Introduction
- •4 Quantum-Like Bayesian Networks
- •7.1 Results and Discussion
- •8 Conclusion
- •References
- •Cybernetics and AI
- •1 Introduction
- •2 Modeling of the Vehicle
- •2.1 Introduction to Braitenberg Vehicles
- •2.2 Quantum Approach for BV Decision Making
- •3 Topics in Eigenlogic
- •3.1 The Eigenlogic Operators
- •3.2 Incorporation of Fuzzy Logic
- •4 BV Quantum Robot Simulation Results
- •4.1 Simulation Environment
- •5 Quantum Wheel of Emotions
- •6 Discussion and Conclusion
- •7 Credits and Acknowledgements
- •References
- •1 Introduction
- •2.1 What Is Intelligence?
- •2.2 Human Intelligence and Quantum Cognition
- •2.3 In Search of the General Principles of Intelligence
- •3 Towards a Moral Test
- •4 Compositional Quantum Cognition
- •4.1 Categorical Compositional Model of Meaning
- •4.2 Proof of Concept: Compositional Quantum Cognition
- •5 Implementation of a Moral Test
- •5.2 Step II: A Toy Example, Moral Dilemmas and Context Effects
- •5.4 Step IV. Application for AI
- •6 Discussion and Conclusion
- •Appendix A: Example of a Moral Dilemma
- •References
- •Probability and Beyond
- •1 Introduction
- •2 The Theory of Density Hypercubes
- •2.1 Construction of the Theory
- •2.2 Component Symmetries
- •2.3 Normalisation and Causality
- •3 Decoherence and Hyper-decoherence
- •3.1 Decoherence to Classical Theory
- •4 Higher Order Interference
- •5 Conclusions
- •A Proofs
- •References
- •Information Retrieval
- •1 Introduction
- •2 Related Work
- •3 Quantum Entanglement and Bell Inequality
- •5 Experiment Settings
- •5.1 Dataset
- •5.3 Experimental Procedure
- •6 Results and Discussion
- •7 Conclusion
- •A Appendix
- •References
- •Investigating Bell Inequalities for Multidimensional Relevance Judgments in Information Retrieval
- •1 Introduction
- •2 Quantifying Relevance Dimensions
- •3 Deriving a Bell Inequality for Documents
- •3.1 CHSH Inequality
- •3.2 CHSH Inequality for Documents Using the Trace Method
- •4 Experiment and Results
- •5 Conclusion and Future Work
- •A Appendix
- •References
- •Short Paper
- •An Update on Updating
- •References
- •Author Index
- •The Sure Thing principle, the Disjunction Effect and the Law of Total Probability
- •Material and methods
- •Experimental results.
- •Experiment 1
- •Experiment 2
- •More versus less risk averse participants
- •Theoretical analysis
- •Shared features of the theoretical models
- •The Markov model
- •The quantum-like model
- •Logistic model
- •Theoretical model performance
- •Model comparison for risk attitude partitioning.
- •Discussion
- •Authors contributions
- •Ethical clearance
- •Funding
- •Acknowledgements
- •References
- •Markov versus quantum dynamic models of belief change during evidence monitoring
- •Results
- •Model comparisons.
- •Discussion
- •Methods
- •Participants.
- •Task.
- •Procedure.
- •Mathematical Models.
- •Acknowledgements
- •New Developments for Value-based Decisions
- •Context Effects in Preferential Choice
- •Comparison of Model Mechanisms
- •Qualitative Empirical Comparisons
- •Quantitative Empirical Comparisons
- •Neural Mechanisms of Value Accumulation
- •Neuroimaging Studies of Context Effects and Attribute-Wise Decision Processes
- •Concluding Remarks
- •Acknowledgments
- •References
- •Comparison of Markov versus quantum dynamical models of human decision making
- •CONFLICT OF INTEREST
- •Endnotes
- •FURTHER READING
- •REFERENCES
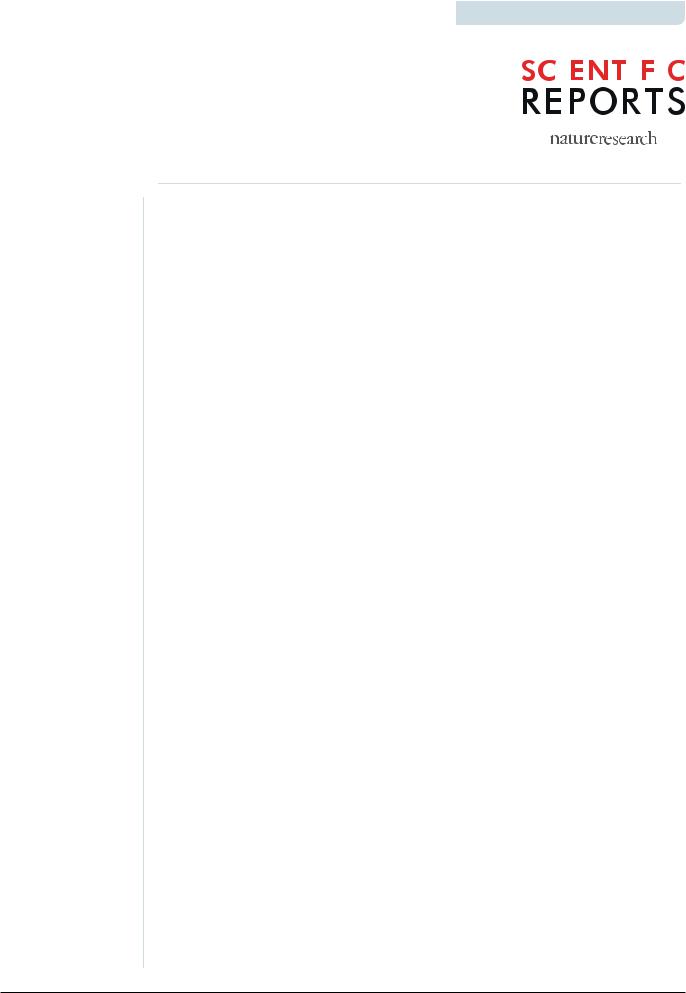
|
|
|
|
|
|
|
|
|
|
suai.ru/our-contacts |
www.nature.co /scientificr ports |
||||||||
quantum machine learning |
|||||||||
|
|
|
|
|
|
|
|
|
|
|
|
|
|
|
|
|
|
|
|
|
|
|
|
|
|
|
|
|
|
OPEN Markov versus quantum dynamic models of belief change during evidence monitoring
Jerome R. Busemeyer1 , Peter D. Kvam2 & Timothy J. Pleskac3
Twodiferentdynamicmodelsforbeliefchangeduringevidencemonitoringwereevaluated:Markov and quantum. They were empirically tested with an experiment in which participants monitored evidence for an initial period of time, made a probability rating, then monitored more evidence, before making a second rating. The models were qualitatively tested by manipulating the time intervalsinamannerthatprovidedatestforinterferenceefectsofthe rstratingonthesecond.The
Markov model predicted no interference, whereas the quantum model predicted interference. More importantly, a quantitative comparison of the two models was also carried out using a generalization criterionmethod:theparameterswere ttodatafromonesetoftimeintervals andthenthesesame parameters were used to predict data from another set of time intervals. The results indicated that some features of both Markov and quantum models are needed to accurately account for the results.
When monitoring evidence during decision making, a person’s belief about each hypothesis changes and evolves across time. For example, when watching a murder mystery lm, the viewer’s beliefs about guilt or innocence of each suspect change as the person monitors the evidence from the ongoing movie. What are the basic dynamics that underlie these changes in belief during evidence accumulation? Here we investigate two fundamentally different ways to understand the dynamics of belief change.
e “classical” way of modeling evidence dynamics is to assume that the dynamics follow a Markov process, such as a random walk or a dri di usion model (see, e.g., references1–3). ese models are essentially cognitive-neural versions of a Bayesian sequential sampling model in which the belief state at each moment corresponds to the posterior probability of the accumulated evidence4. According to one application of this view5–7, the decision maker’s belief state at any single moment is located at a speci c point on some mental scale of evidence.is belief state changes moment by moment from one location to another on the evidence scale, sketching out a sample path as illustrated in Fig. 1, le panel. At the time point that an experimenter requests a probability rating, the decision maker simply maps the pre-existing mental belief state onto an observed rating response.
A “non-classical” way of modeling evidence dynamics for belief change is to assume that the dynamics follow a quantum process8–10. According to one application of this view11, the decision maker’s belief state at a moment is not located at any speci c point on the mental evidence scale; instead, at any moment, the belief state is inde nite, which is represented by a superposition of beliefs over the mental evidence scale. is superposition state forms a wave that ows across time as illustrated in Fig. 1, right panel (technically, this gure shows the squared magnitude of the amplitude at each state). At the time point that an experimenter requests a judgment, the judge’s indefinite state must be resolved, and the wave is “collapsed” to probabilistically select an observed rating response (red bar at the top of Fig. 1, right panel). Note that we are not proposing that the brain is some type of quantum computer – we are simply using the basic principles of quantum probability theory to predict human behavior. At least one classical neural network model that could implement these principles has been proposed12.
Previous research11 tested and compared the predictions of these two models using a “dot motion” task for studying evidence monitoring13. is task has become popular among neuroscientists for studying evolution of con dence (see, e.g., reference3). e dot motion task is a perceptual task that requires participants to judge the le /right direction of dot motion in a display consisting of moving dots within a circular aperture (see le panel of Fig. 2). A small percentage of the dots move coherently in one direction (le or right), and the rest move randomly. Difculty is manipulated between trials by changing the percentage of coherently moving dots. e
1Psychological BrainSciences, IndianaUniversity, Bloominton, 47405, Indiana,USA. 2Psychology, University of Florida,Gainsville,32611,Florida,USA.3Psychology,UniversityofKansas,Lawrence,66045,Kansas,USA.*email: jbusemey@indiana.edu
Scientific Reports | (2019) 9:18025 | https://doi.org/10.1038/s41598-019-54383-9 |
1 |

www.nature.com/scientificreports/ |
www.nature.co /scientificr ports |
suai.ru/our-contacts |
quantum machine learning |
|
0.5 |
|
|
|
|
|
0.5 |
|
|
|
|
|
0.4 |
|
|
|
|
|
0.4 |
|
|
|
|
Time |
0.3 |
|
|
|
|
Time |
0.3 |
|
|
|
|
Processing |
|
|
|
|
Processing |
|
|
|
|
||
0.2 |
|
|
|
|
0.2 |
|
|
|
|
||
|
|
|
|
|
|
|
|
|
|
||
|
0.1 |
|
|
|
|
|
0.1 |
|
|
|
|
|
0 |
|
|
|
|
|
0 |
|
|
|
|
|
-1 |
-0.5 |
0 |
0.5 |
1 |
|
-1 |
-0.5 |
0 |
0.5 |
1 |
Evidence State |
Evidence State |
Figure 1. Illustration of Markov (Le ) and quantum (Right) processes for evolution of beliefs during evidence monitoring. e horizontal axis represents 101 belief states associated with subjective evidence scale values ranging from 0 to 100 in one unit steps. e vertical axis represents time that has passed during evidence monitoring. e Markov process moves from a belief state located at one value at one moment in time to another belief state at a later moment to produce a sample path across time. At the time of a request for a rating, the current belief state is mapped to a probability rating value (small circle). e quantum process assigns a distribution across the belief states at one moment, which moves to another distribution at a later moment (technically, this gure shows the squared magnitude of the amplitude at each state). At the time of a request for a rating, the current distribution is used to probabilistically select a rating (red vertical line).
Probabilityi Rating Request Location
35
30
25
20
15
10
5
0
0
|
Condition 3, Generalization Condition |
|
|
||
Model Calibration |
|
Condition 2 |
|
|
|
|
Condition 1 |
|
Model Calibration |
|
|
|
|
|
|
|
|
0.5 |
1 |
1.5 |
2 |
2.5 |
3 |
|
Time in sec |
|
|
|
Figure 2. Illustrations of dot motion task with probability rating scale and protocol for experiment (Le ), timing of judgments (right). On the le , a judge watches the dot motion and at the appointed time selects a rating response from the semicircle. e judge rst watches the dots motion for a period of time, then makes a rst choice response, and then observes the dot motion for a period of time again, and then makes a second
rating response. On the right, each condition had a di erent pair of time points for requests for ratings: the time intervals of the rst two conditions are contained within condition 3. Conditions 1 and 2 were used to estimate model parameters and then these same model parameters were used to predict the ratings for condition 3.
judge watches the moving dots for a period time at which point the experimenter requests a probability rating for a direction (see Fig. 2, le panel). In the study by Kvam et al.11, each of 9 participants received over 2500 trials on the dot motion task.
e experimental design used by Kvam et al.11 included 4 coherence levels (2%, 4%, 8%, or 16%) and two dif-
ferent kinds of judgment conditions. In the choice-con dence condition, participants were given t1 = 0.5 s to view the display, and then a tone was presented that signaled the time to make a binary (le /right) decision. A er
an additional ∆t = 0.05, 0.75, or 1.5 s following the choice, participants were prompted by a second tone to make a probability rating on a 0 (certain le ) to 100% (certain right) rating scale (see Fig. 2, le panel). In a confidence-only condition, participants didn’t have to make any decision and instead they simply made a pre-determined response when hearing the tone at time t1, and then later they made a probability rating at the same total time points t2 as the choice - con dence condition.
When comparing the choice-con dence condition to the con dence-only condition, a Markov model predicts that the marginal distribution of con dence at time t2 (pooled across choices at time t1 for the choice-con dence condition) should be the same between the two conditions. is is a general prediction and not restricted to a
Scientific Reports | (2019) 9:18025 | https://doi.org/10.1038/s41598-019-54383-9 |
2 |
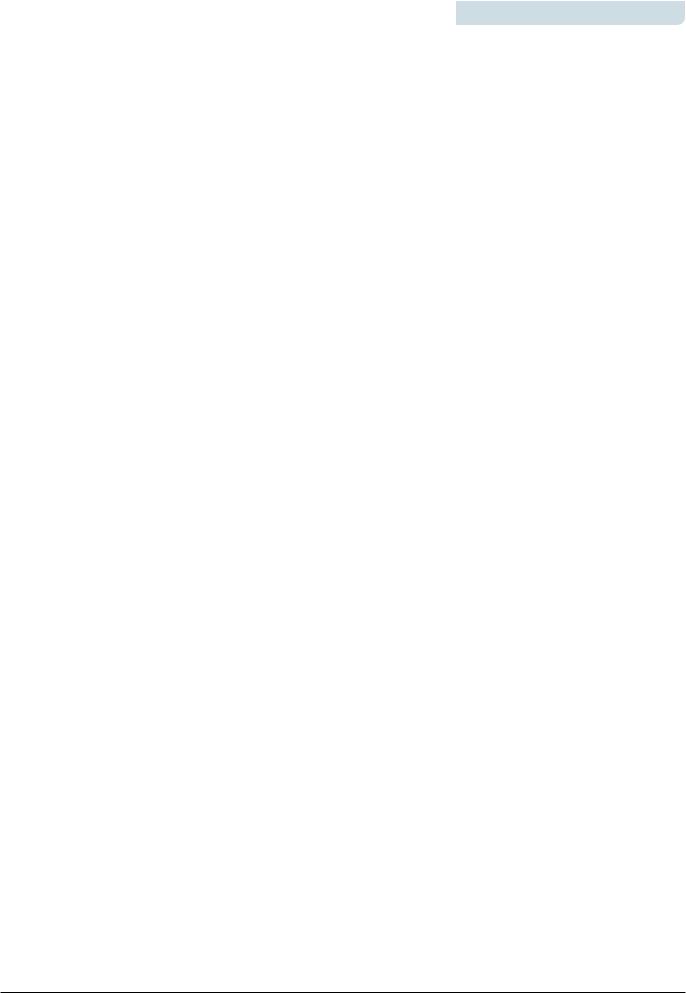
www.nature.com/scientificreports/ |
www.nature.co /scientificr ports |
suai.ru/our-contacts |
quantum machine learning |
particular version of a Markov model. e prediction even holds if the dynamics of the Markov process change between the rst and second intervals. We only need to assume that these dynamics are determined by the dot motion information a er time t1 rather than the type of response at time t1 (decision versus pre-planned response). See pages 247 to 248 in reference9,11 for a formal proof. In contrast, a quantum model predicts that these two distributions should be di erent, and the di erence between conditions is called an interference e ect.
e predictions for a Markov model are based on the following reasons. Markov processes describe the evolution of the probability distribution over evidence levels across time (see Methods). e probability of a response is computed from the sum of the probabilities of evidence levels associated with that response. Under the con - dence - only condition, the probability distribution evolves during the rst time interval, and then continues to evolve until the end of the second time interval, which is used to compute the probability of a judgment response. Under the choice-con dence condition, the probability distribution evolves across the rst time interval, which is used to compute the probability of a choice. Given the choice that is made at the end of the rst time interval, the probability distribution is conditioned (collapsed) on levels consistent with the observed choice. en this conditional probability distribution continues to evolve until the end of the second interval. e marginal probabilities of the judgments for the choice-con dence condition are computed by summing the joint probabilities of choices followed by judgments over choices. Finally, these marginal probabilities turn out to be equal to the sums across time intervals obtained from the con dence - only condition, because the Markov process obeys the Chapman - Kolmogorov equation, which is a dynamic form of law of total probability14.
e contrasting predictions for a quantum model are based on the following reasons. Quantum processes describe the evolution of an amplitude distribution over evidence levels across time (see Methods). e probability of a response is computed from the sum of the squared magnitudes of the amplitudes associated with that response. Under the con dence - only condition, the amplitude distribution evolves during the rst time interval, and then continues to evolve until the end of the second time interval, which is used to compute the probability of a judgment response. Under the choice-con dence condition, the amplitude distribution evolves across the rst time interval, which is used to compute the probability of a choice. Given the choice that is made at the end of therst time interval, the amplitude distribution is conditioned (collapsed) on levels consistent with the observed choice. en the conditional amplitude distribution continues to evolve until the end of the second interval. Once again, the marginal probabilities of the judgments for the choice-con dence condition are computed by summing the joint probabilities of choices followed by judgments over choices. However, these marginal probabilities di er from those obtained from the con dence - only condition, because the latter condition produces a sum of amplitudes rather than a sum of probabilities across time intervals, and taking the squared magnitudes of the sum of amplitudes to compute probabilities generates interference terms that violate of the law of total probability.
e results of the experiment strongly favored the quantum model predictions: the interference e ect was signi cant at the group level, and at the individual level, 6 out of the 9 participants produced signi cant interference e ects. Furthermore, parameterized versions of the Markov and quantum models were used to predict both the binary choices and as well as the con dence ratings using the same number of model parameters, and results of a Bayesian model comparison strongly favored the quantum over the Markov model for 7 out of 9 participants.
The current paper presents a new type of test for interference effects. The previous study examined the sequential e ects of a binary decision on a later probability judgment; in contrast, the present study examines the sequential e ects of a probability rating on a later probability judgment. A binary decision may evoke a stronger commitment, whereas a probability judgment does not force the decision maker to make any clear decision. e question is whether the rst probability judgment is sufcient to produce an interference e ect like that produced by committing to a binary decision.
Secondly, and more important, the present experiment provided a new generalization test15 for quantitatively comparing the predictions computed from parameterized versions of the competing models. ( e version of the Markov model that we test is a close approximation to a well-established di usion model5). e generalization test provides a di erent method than the Bayes factor previously used in11 for quantitatively comparing the two models because it is based on a priori predictions made to new experimental conditions.
A total of 11 participants (8 females, 3 males) were paid depending on their performance for making judgments on approximately 1000 trials across 3 daily sessions (see Methods for more details). Once again, the participants monitored dot motion using 4 coherence levels (2%, 4%, 8%, or 16%) with half of the trials presenting le moving dots and the remaining half of the trials presenting right moving dots. In this new experiment, two
probability ratings were made at a pair (t1, t2) of time points (see Fig. 2, right panel). e experiment included three main conditions: (1) requests for probability ratings at times t1 = 0.5 s and t2 = 1.5 s, (2) requests for rat-
ings at times t1 = 1.5 s and t2 = 2.5 s, and (3) requests for ratings at times t1 = 0.5 s and t2 = 2.5 s. is design provided two new tests of Markov and quantum models.
First of all, we tested for interference e ects by comparing the marginal distribution of probability ratings at time t2 = 1.5 s for condition 1 (pooled across ratings made at time t1 = 0.5 s) with the distribution of ratings at
time t1 = 1.5 s from condition 2. Note that at time t1 = 1.5 s, condition 2 was not preceded by any previous rating, whereas condition 1 was preceded by a rating. Once again, the Markov model predicts no di erence between conditions at the matching time points, and in contrast, the quantum model predicts an interference e ect of therst rating on the second.
Secondly, this design also provided a strong generalization test for quantitatively comparing the predictions computed from the competing models. e parameters from both models were estimated using maximum likelihood from the probability ratings distributions obtained from the rst two conditions for each individual; then these same parameters were used to predict probability rating distribution for each person on the third condition (see Fig. 2). Both models used two parameters to predict the probability rating distributions (see Methods for details): one that we call the “dri ” rate that a ects the direction and strength of change in the distribution of
Scientific Reports | (2019) 9:18025 | https://doi.org/10.1038/s41598-019-54383-9 |
3 |