
- •Preface
- •Contents
- •Contributors
- •Modeling Meaning Associated with Documental Entities: Introducing the Brussels Quantum Approach
- •1 Introduction
- •2 The Double-Slit Experiment
- •3 Interrogative Processes
- •4 Modeling the QWeb
- •5 Adding Context
- •6 Conclusion
- •Appendix 1: Interference Plus Context Effects
- •Appendix 2: Meaning Bond
- •References
- •1 Introduction
- •2 Bell Test in the Problem of Cognitive Semantic Information Retrieval
- •2.1 Bell Inequality and Its Interpretation
- •2.2 Bell Test in Semantic Retrieving
- •3 Results
- •References
- •1 Introduction
- •2 Basics of Quantum Probability Theory
- •3 Steps to Build an HSM Model
- •3.1 How to Determine the Compatibility Relations
- •3.2 How to Determine the Dimension
- •3.5 Compute the Choice Probabilities
- •3.6 Estimate Model Parameters, Compare and Test Models
- •4 Computer Programs
- •5 Concluding Comments
- •References
- •Basics of Quantum Theory for Quantum-Like Modeling Information Retrieval
- •1 Introduction
- •3 Quantum Mathematics
- •3.1 Hermitian Operators in Hilbert Space
- •3.2 Pure and Mixed States: Normalized Vectors and Density Operators
- •4 Quantum Mechanics: Postulates
- •5 Compatible and Incompatible Observables
- •5.1 Post-Measurement State From the Projection Postulate
- •6 Interpretations of Quantum Mechanics
- •6.1 Ensemble and Individual Interpretations
- •6.2 Information Interpretations
- •7 Quantum Conditional (Transition) Probability
- •9 Formula of Total Probability with the Interference Term
- •9.1 Växjö (Realist Ensemble Contextual) Interpretation of Quantum Mechanics
- •10 Quantum Logic
- •11 Space of Square Integrable Functions as a State Space
- •12 Operation of Tensor Product
- •14 Qubit
- •15 Entanglement
- •References
- •1 Introduction
- •2 Background
- •2.1 Distributional Hypothesis
- •2.2 A Brief History of Word Embedding
- •3 Applications of Word Embedding
- •3.1 Word-Level Applications
- •3.2 Sentence-Level Application
- •3.3 Sentence-Pair Level Application
- •3.4 Seq2seq Application
- •3.5 Evaluation
- •4 Reconsidering Word Embedding
- •4.1 Limitations
- •4.2 Trends
- •4.4 Towards Dynamic Word Embedding
- •5 Conclusion
- •References
- •1 Introduction
- •2 Motivating Example: Car Dealership
- •3 Modelling Elementary Data Types
- •3.1 Orthogonal Data Types
- •3.2 Non-orthogonal Data Types
- •4 Data Type Construction
- •5 Quantum-Based Data Type Constructors
- •5.1 Tuple Data Type Constructor
- •5.2 Set Data Type Constructor
- •6 Conclusion
- •References
- •Incorporating Weights into a Quantum-Logic-Based Query Language
- •1 Introduction
- •2 A Motivating Example
- •5 Logic-Based Weighting
- •6 Related Work
- •7 Conclusion
- •References
- •Searching for Information with Meet and Join Operators
- •1 Introduction
- •2 Background
- •2.1 Vector Spaces
- •2.2 Sets Versus Vector Spaces
- •2.3 The Boolean Model for IR
- •2.5 The Probabilistic Models
- •3 Meet and Join
- •4 Structures of a Query-by-Theme Language
- •4.1 Features and Terms
- •4.2 Themes
- •4.3 Document Ranking
- •4.4 Meet and Join Operators
- •5 Implementation of a Query-by-Theme Language
- •6 Related Work
- •7 Discussion and Future Work
- •References
- •Index
- •Preface
- •Organization
- •Contents
- •Fundamentals
- •Why Should We Use Quantum Theory?
- •1 Introduction
- •2 On the Human Science/Natural Science Issue
- •3 The Human Roots of Quantum Science
- •4 Qualitative Parallels Between Quantum Theory and the Human Sciences
- •5 Early Quantitative Applications of Quantum Theory to the Human Sciences
- •6 Epilogue
- •References
- •Quantum Cognition
- •1 Introduction
- •2 The Quantum Persuasion Approach
- •3 Experimental Design
- •3.1 Testing for Perspective Incompatibility
- •3.2 Quantum Persuasion
- •3.3 Predictions
- •4 Results
- •4.1 Descriptive Statistics
- •4.2 Data Analysis
- •4.3 Interpretation
- •5 Discussion and Concluding Remarks
- •References
- •1 Introduction
- •2 A Probabilistic Fusion Model of Trust
- •3 Contextuality
- •4 Experiment
- •4.1 Subjects
- •4.2 Design and Materials
- •4.3 Procedure
- •4.4 Results
- •4.5 Discussion
- •5 Summary and Conclusions
- •References
- •Probabilistic Programs for Investigating Contextuality in Human Information Processing
- •1 Introduction
- •2 A Framework for Determining Contextuality in Human Information Processing
- •3 Using Probabilistic Programs to Simulate Bell Scenario Experiments
- •References
- •1 Familiarity and Recollection, Verbatim and Gist
- •2 True Memory, False Memory, over Distributed Memory
- •3 The Hamiltonian Based QEM Model
- •4 Data and Prediction
- •5 Discussion
- •References
- •Decision-Making
- •1 Introduction
- •1.2 Two Stage Gambling Game
- •2 Quantum Probabilities and Waves
- •2.1 Intensity Waves
- •2.2 The Law of Balance and Probability Waves
- •2.3 Probability Waves
- •3 Law of Maximal Uncertainty
- •3.1 Principle of Entropy
- •3.2 Mirror Principle
- •4 Conclusion
- •References
- •1 Introduction
- •4 Quantum-Like Bayesian Networks
- •7.1 Results and Discussion
- •8 Conclusion
- •References
- •Cybernetics and AI
- •1 Introduction
- •2 Modeling of the Vehicle
- •2.1 Introduction to Braitenberg Vehicles
- •2.2 Quantum Approach for BV Decision Making
- •3 Topics in Eigenlogic
- •3.1 The Eigenlogic Operators
- •3.2 Incorporation of Fuzzy Logic
- •4 BV Quantum Robot Simulation Results
- •4.1 Simulation Environment
- •5 Quantum Wheel of Emotions
- •6 Discussion and Conclusion
- •7 Credits and Acknowledgements
- •References
- •1 Introduction
- •2.1 What Is Intelligence?
- •2.2 Human Intelligence and Quantum Cognition
- •2.3 In Search of the General Principles of Intelligence
- •3 Towards a Moral Test
- •4 Compositional Quantum Cognition
- •4.1 Categorical Compositional Model of Meaning
- •4.2 Proof of Concept: Compositional Quantum Cognition
- •5 Implementation of a Moral Test
- •5.2 Step II: A Toy Example, Moral Dilemmas and Context Effects
- •5.4 Step IV. Application for AI
- •6 Discussion and Conclusion
- •Appendix A: Example of a Moral Dilemma
- •References
- •Probability and Beyond
- •1 Introduction
- •2 The Theory of Density Hypercubes
- •2.1 Construction of the Theory
- •2.2 Component Symmetries
- •2.3 Normalisation and Causality
- •3 Decoherence and Hyper-decoherence
- •3.1 Decoherence to Classical Theory
- •4 Higher Order Interference
- •5 Conclusions
- •A Proofs
- •References
- •Information Retrieval
- •1 Introduction
- •2 Related Work
- •3 Quantum Entanglement and Bell Inequality
- •5 Experiment Settings
- •5.1 Dataset
- •5.3 Experimental Procedure
- •6 Results and Discussion
- •7 Conclusion
- •A Appendix
- •References
- •Investigating Bell Inequalities for Multidimensional Relevance Judgments in Information Retrieval
- •1 Introduction
- •2 Quantifying Relevance Dimensions
- •3 Deriving a Bell Inequality for Documents
- •3.1 CHSH Inequality
- •3.2 CHSH Inequality for Documents Using the Trace Method
- •4 Experiment and Results
- •5 Conclusion and Future Work
- •A Appendix
- •References
- •Short Paper
- •An Update on Updating
- •References
- •Author Index
- •The Sure Thing principle, the Disjunction Effect and the Law of Total Probability
- •Material and methods
- •Experimental results.
- •Experiment 1
- •Experiment 2
- •More versus less risk averse participants
- •Theoretical analysis
- •Shared features of the theoretical models
- •The Markov model
- •The quantum-like model
- •Logistic model
- •Theoretical model performance
- •Model comparison for risk attitude partitioning.
- •Discussion
- •Authors contributions
- •Ethical clearance
- •Funding
- •Acknowledgements
- •References
- •Markov versus quantum dynamic models of belief change during evidence monitoring
- •Results
- •Model comparisons.
- •Discussion
- •Methods
- •Participants.
- •Task.
- •Procedure.
- •Mathematical Models.
- •Acknowledgements
- •New Developments for Value-based Decisions
- •Context Effects in Preferential Choice
- •Comparison of Model Mechanisms
- •Qualitative Empirical Comparisons
- •Quantitative Empirical Comparisons
- •Neural Mechanisms of Value Accumulation
- •Neuroimaging Studies of Context Effects and Attribute-Wise Decision Processes
- •Concluding Remarks
- •Acknowledgments
- •References
- •Comparison of Markov versus quantum dynamical models of human decision making
- •CONFLICT OF INTEREST
- •Endnotes
- •FURTHER READING
- •REFERENCES

suai.ru/our-contacts |
quantum machine learning |
28 A. Lambert-Mogiliansky et al.
information has globally no impact compared to the control group. None of the population variable has any impact. This suggests that the quantum model may indeed capture basic regularities of the mind relevant to decision-making.
2 The Quantum Persuasion Approach
Let us first briefly describe the classical approach (Bayesian persuasion) developed by Kamenica and Gentzkow [12]. We have a person call him Sender who tries to influence a decision-maker call her Receiver by means of an information structure or a measurement that generates information. Information a ects Receiver’s beliefs which in turn a ect her evaluation of uncertain choice alternatives and therefore the choice she makes. In the classical context Receiver updates her beliefs using Bayes rule and therefore the power of Sender is constrained by Bayesian plausibility - that is the expected posteriors must equal the priors.
The quantum persuasion approach has been developed in the same vein as the Bayesian persuasion. A central motivation is that persuasion seems much more e ective than what comes out of the Bayesian approach. So instead of assuming that agents are classical, it has been proposed that they are quantum-like. This means that the representation of reality upon which they make decision does not evolved according to Bayes rule but follows instead von Neumann-L˝uder’s rule (vNL). vNL updating has been shown to be an expression of dynamic consistency in such a context (see [7]).
The present paper aims at experimentally testing some predictions of the theory of quantum persuasion. More precisely as shown in [6], Sender can use ‘distracting’ measurements as tools to influence Receiver. A distracting measurement corresponds to a measurement that generates information that is incompatible (or Bohr complementary) with the information used by Receiver to evaluate the choice alternatives for decision-making. The objective is to switch the focus of Receiver’s mind (distract her) which changes her cognitive state or her beliefs although no information relevant to her concern is provided. The following example from [5] illustrates the point.
Example
A consumer is considering the purchase of a second hand smartphone at price 30eof uncertain value to her. What matters to her is its technical quality which may be standard or excellent. She holds beliefs about the quality of the smartphone. Based on those beliefs, she assigns an expected utility value to the smartphone which determines her decision whether or not to buy the item. Receiver’s expected utility for the smartphone in belief state B is represented by the trace of the product of operators A and B2:
Eu (A; B) = Tr (AB) = (1/5) 100 + (4/5) 0 = 20 < 30. |
(1) |
Given belief B Receiver does not want to buy the smartphone.
2 For a complete formulation of choice theory in the quantum context, see [7].
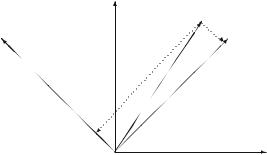
suai.ru/our-contacts |
quantum machine learning |
The Power of Distraction: An Experimental Test of Quantum Persuasion |
29 |
Can Sender persuade Receiver to buy by selecting an appropriate measurement? Consider another property (perspective) of the smartphone that we refer to as Glamour (i.e. whether celebrities have this brand or not). The two properties (perspectives) are assumed incompatible in the mind of Receiver. Receiver can think in terms of either one of the two perspectives but she cannot synthesize (combine in a stable way) pieces of information from the two perspectives. This is illustrated in Fig. 1.
|
e2 |
|
B |
B = |NG |
B = |G |
|
e1 |
Fig. 1. Receiver’s cognitive state.
Assume that Sender brings up the discussion to the Glamour perspective and performs the measurement so Receiver learns whether her preferred celebrity has this smartphone. With some probability p (say 0.9) the new cognitive state is B = G and with the complementary probability 1 − p = .1 it is B = N G.
We note that:
Eu (A; B ) = Tr (AB ) = 50 > 30. |
(2) |
Eu (A; B ) = Tr (AB ) = 50 > 30. |
(3) |
In both cases Receiver is persuaded to buy and Sender gets a positive utility. In the example above it is easy to show that the new belief state violates
Bayesian plausibility - the expected posteriors for the event ‘the smartphone is excellent’ are:
5p + .5(1 − p) = .5 |
(4) |
which is larger than the priors which equals 0.2. Moreover the measurement Glamour is not relevant to the beliefs about the quality of the used smartphone. It is a distraction. Yet it a ects the beliefs and the associated decision. In a Bayesian context irrelevant/uninformative data do not modify beliefs and therefore cannot be used as means of persuasion.