
- •Preface
- •Contents
- •Contributors
- •Modeling Meaning Associated with Documental Entities: Introducing the Brussels Quantum Approach
- •1 Introduction
- •2 The Double-Slit Experiment
- •3 Interrogative Processes
- •4 Modeling the QWeb
- •5 Adding Context
- •6 Conclusion
- •Appendix 1: Interference Plus Context Effects
- •Appendix 2: Meaning Bond
- •References
- •1 Introduction
- •2 Bell Test in the Problem of Cognitive Semantic Information Retrieval
- •2.1 Bell Inequality and Its Interpretation
- •2.2 Bell Test in Semantic Retrieving
- •3 Results
- •References
- •1 Introduction
- •2 Basics of Quantum Probability Theory
- •3 Steps to Build an HSM Model
- •3.1 How to Determine the Compatibility Relations
- •3.2 How to Determine the Dimension
- •3.5 Compute the Choice Probabilities
- •3.6 Estimate Model Parameters, Compare and Test Models
- •4 Computer Programs
- •5 Concluding Comments
- •References
- •Basics of Quantum Theory for Quantum-Like Modeling Information Retrieval
- •1 Introduction
- •3 Quantum Mathematics
- •3.1 Hermitian Operators in Hilbert Space
- •3.2 Pure and Mixed States: Normalized Vectors and Density Operators
- •4 Quantum Mechanics: Postulates
- •5 Compatible and Incompatible Observables
- •5.1 Post-Measurement State From the Projection Postulate
- •6 Interpretations of Quantum Mechanics
- •6.1 Ensemble and Individual Interpretations
- •6.2 Information Interpretations
- •7 Quantum Conditional (Transition) Probability
- •9 Formula of Total Probability with the Interference Term
- •9.1 Växjö (Realist Ensemble Contextual) Interpretation of Quantum Mechanics
- •10 Quantum Logic
- •11 Space of Square Integrable Functions as a State Space
- •12 Operation of Tensor Product
- •14 Qubit
- •15 Entanglement
- •References
- •1 Introduction
- •2 Background
- •2.1 Distributional Hypothesis
- •2.2 A Brief History of Word Embedding
- •3 Applications of Word Embedding
- •3.1 Word-Level Applications
- •3.2 Sentence-Level Application
- •3.3 Sentence-Pair Level Application
- •3.4 Seq2seq Application
- •3.5 Evaluation
- •4 Reconsidering Word Embedding
- •4.1 Limitations
- •4.2 Trends
- •4.4 Towards Dynamic Word Embedding
- •5 Conclusion
- •References
- •1 Introduction
- •2 Motivating Example: Car Dealership
- •3 Modelling Elementary Data Types
- •3.1 Orthogonal Data Types
- •3.2 Non-orthogonal Data Types
- •4 Data Type Construction
- •5 Quantum-Based Data Type Constructors
- •5.1 Tuple Data Type Constructor
- •5.2 Set Data Type Constructor
- •6 Conclusion
- •References
- •Incorporating Weights into a Quantum-Logic-Based Query Language
- •1 Introduction
- •2 A Motivating Example
- •5 Logic-Based Weighting
- •6 Related Work
- •7 Conclusion
- •References
- •Searching for Information with Meet and Join Operators
- •1 Introduction
- •2 Background
- •2.1 Vector Spaces
- •2.2 Sets Versus Vector Spaces
- •2.3 The Boolean Model for IR
- •2.5 The Probabilistic Models
- •3 Meet and Join
- •4 Structures of a Query-by-Theme Language
- •4.1 Features and Terms
- •4.2 Themes
- •4.3 Document Ranking
- •4.4 Meet and Join Operators
- •5 Implementation of a Query-by-Theme Language
- •6 Related Work
- •7 Discussion and Future Work
- •References
- •Index
- •Preface
- •Organization
- •Contents
- •Fundamentals
- •Why Should We Use Quantum Theory?
- •1 Introduction
- •2 On the Human Science/Natural Science Issue
- •3 The Human Roots of Quantum Science
- •4 Qualitative Parallels Between Quantum Theory and the Human Sciences
- •5 Early Quantitative Applications of Quantum Theory to the Human Sciences
- •6 Epilogue
- •References
- •Quantum Cognition
- •1 Introduction
- •2 The Quantum Persuasion Approach
- •3 Experimental Design
- •3.1 Testing for Perspective Incompatibility
- •3.2 Quantum Persuasion
- •3.3 Predictions
- •4 Results
- •4.1 Descriptive Statistics
- •4.2 Data Analysis
- •4.3 Interpretation
- •5 Discussion and Concluding Remarks
- •References
- •1 Introduction
- •2 A Probabilistic Fusion Model of Trust
- •3 Contextuality
- •4 Experiment
- •4.1 Subjects
- •4.2 Design and Materials
- •4.3 Procedure
- •4.4 Results
- •4.5 Discussion
- •5 Summary and Conclusions
- •References
- •Probabilistic Programs for Investigating Contextuality in Human Information Processing
- •1 Introduction
- •2 A Framework for Determining Contextuality in Human Information Processing
- •3 Using Probabilistic Programs to Simulate Bell Scenario Experiments
- •References
- •1 Familiarity and Recollection, Verbatim and Gist
- •2 True Memory, False Memory, over Distributed Memory
- •3 The Hamiltonian Based QEM Model
- •4 Data and Prediction
- •5 Discussion
- •References
- •Decision-Making
- •1 Introduction
- •1.2 Two Stage Gambling Game
- •2 Quantum Probabilities and Waves
- •2.1 Intensity Waves
- •2.2 The Law of Balance and Probability Waves
- •2.3 Probability Waves
- •3 Law of Maximal Uncertainty
- •3.1 Principle of Entropy
- •3.2 Mirror Principle
- •4 Conclusion
- •References
- •1 Introduction
- •4 Quantum-Like Bayesian Networks
- •7.1 Results and Discussion
- •8 Conclusion
- •References
- •Cybernetics and AI
- •1 Introduction
- •2 Modeling of the Vehicle
- •2.1 Introduction to Braitenberg Vehicles
- •2.2 Quantum Approach for BV Decision Making
- •3 Topics in Eigenlogic
- •3.1 The Eigenlogic Operators
- •3.2 Incorporation of Fuzzy Logic
- •4 BV Quantum Robot Simulation Results
- •4.1 Simulation Environment
- •5 Quantum Wheel of Emotions
- •6 Discussion and Conclusion
- •7 Credits and Acknowledgements
- •References
- •1 Introduction
- •2.1 What Is Intelligence?
- •2.2 Human Intelligence and Quantum Cognition
- •2.3 In Search of the General Principles of Intelligence
- •3 Towards a Moral Test
- •4 Compositional Quantum Cognition
- •4.1 Categorical Compositional Model of Meaning
- •4.2 Proof of Concept: Compositional Quantum Cognition
- •5 Implementation of a Moral Test
- •5.2 Step II: A Toy Example, Moral Dilemmas and Context Effects
- •5.4 Step IV. Application for AI
- •6 Discussion and Conclusion
- •Appendix A: Example of a Moral Dilemma
- •References
- •Probability and Beyond
- •1 Introduction
- •2 The Theory of Density Hypercubes
- •2.1 Construction of the Theory
- •2.2 Component Symmetries
- •2.3 Normalisation and Causality
- •3 Decoherence and Hyper-decoherence
- •3.1 Decoherence to Classical Theory
- •4 Higher Order Interference
- •5 Conclusions
- •A Proofs
- •References
- •Information Retrieval
- •1 Introduction
- •2 Related Work
- •3 Quantum Entanglement and Bell Inequality
- •5 Experiment Settings
- •5.1 Dataset
- •5.3 Experimental Procedure
- •6 Results and Discussion
- •7 Conclusion
- •A Appendix
- •References
- •Investigating Bell Inequalities for Multidimensional Relevance Judgments in Information Retrieval
- •1 Introduction
- •2 Quantifying Relevance Dimensions
- •3 Deriving a Bell Inequality for Documents
- •3.1 CHSH Inequality
- •3.2 CHSH Inequality for Documents Using the Trace Method
- •4 Experiment and Results
- •5 Conclusion and Future Work
- •A Appendix
- •References
- •Short Paper
- •An Update on Updating
- •References
- •Author Index
- •The Sure Thing principle, the Disjunction Effect and the Law of Total Probability
- •Material and methods
- •Experimental results.
- •Experiment 1
- •Experiment 2
- •More versus less risk averse participants
- •Theoretical analysis
- •Shared features of the theoretical models
- •The Markov model
- •The quantum-like model
- •Logistic model
- •Theoretical model performance
- •Model comparison for risk attitude partitioning.
- •Discussion
- •Authors contributions
- •Ethical clearance
- •Funding
- •Acknowledgements
- •References
- •Markov versus quantum dynamic models of belief change during evidence monitoring
- •Results
- •Model comparisons.
- •Discussion
- •Methods
- •Participants.
- •Task.
- •Procedure.
- •Mathematical Models.
- •Acknowledgements
- •New Developments for Value-based Decisions
- •Context Effects in Preferential Choice
- •Comparison of Model Mechanisms
- •Qualitative Empirical Comparisons
- •Quantitative Empirical Comparisons
- •Neural Mechanisms of Value Accumulation
- •Neuroimaging Studies of Context Effects and Attribute-Wise Decision Processes
- •Concluding Remarks
- •Acknowledgments
- •References
- •Comparison of Markov versus quantum dynamical models of human decision making
- •CONFLICT OF INTEREST
- •Endnotes
- •FURTHER READING
- •REFERENCES
suai.ru/our-contacts |
quantum machine learning |
44 P. D. Bruza and L. Fell
This sum is essentially based on the sum of di erences between marginal prob-
abilities. If |
0 ≥ 1, |
then the di erences between the marginal probabilities |
is deemed |
too great |
for any meaningful conception of contextuality. When |
0 ≤ 0 < 1, then failure of any of the four inequalities signifies the presence of contextuality. Note that these inequalities revert to the standard CHSH inequal-
ities when signalling is not present ( 0 = 0). |
|
|
|
|C11R11 |
+ C12R12 + C21R21 − C22R22| ≤ 2(1 + |
0) |
(11) |
|C11R11 |
+ C12R12 − C21R21 + C22R22| ≤ 2(1 + |
0) |
(12) |
|C11R11 |
− C12R12 + C21R21 + C22R22| ≤ 2(1 + |
0) |
(13) |
| − C11R11 |
+ C12R12 − C21R21 + C22R22| ≤ 2(1 + |
0) |
(14) |
where |
|
|
|
C11R11 = (p1 |
+ p4) − (p2 + p3) |
(15) |
|
C12R12 |
= (p5 |
+ p8) − (p6 + p7) |
(16) |
C21R21 |
= (p9 |
+ p12) − (p10 + p11) |
(17) |
C22R22 |
= (p13 + p16) − (p14 + p15) |
(18) |
4 Experiment
4.1Subjects
Participants consisted of 34 workers using the online crowdsourcing platform, Amazon Mechanical Turk (AMT), and 85 workers using the online crowdsourcing platform, Prolific. Both platforms are used by researchers, amongst others, to post experiments and surveys to a wide audience of thousands of potential participants to view and complete. Participants involved in the present study were able to view any project before agreeing to participate, and were paid a small but standard amount ($1.50 for AMT participants, £1.00 for Prolific participants) per HIT. Both AMT and Prolific platforms allow workers of certain skill, ability or reputation to be specified for a HIT. In this experiment, workers of at least 96% or greater approval rating were selected to balance worker quality with the need to attract a su cient number of participants. De-identified worker IDs were used to ensure participants did not participate in more than one condition. Participants were sourced from a wide variety of countries, including the US, UK, France, Italy, Australia, New Zealand, and South Africa. A test question was first presented to participants to ensure they could properly view the experiment on their browsers. Data collected from participants who could not correctly answer this test question, did not respond to written segments of the experiment in English, or who were deemed not to have understood instructions based on the relevancy/quality of their responses, were excluded from the study. In total, 3 workers were excluded.

suai.ru/our-contacts |
quantum machine learning |
Are Decisions of Image Trustworthiness Contextual? A Pilot Study |
45 |
4.2Design and Materials
Images were selected to fall into two subject categories: untrustworthy subjects and neutral subjects. The first two images depicted infamously untrustworthy subjects: an image of Vladimir Putin2, and an image of a shark3. Figure 2 depicts the images of the neutral subjects: an unknown woman4, and an antelope with her calf 5. All images were manipulated in a detectable way. Image presentation was counter-balanced, and each image was accompanied by three responses: A question-pair, a confidence rating, and a reasoning explanation. The question pairs consisted of combinations of the following four questions:
Fig. 2. Image stimuli
–Do you think the person in this image is honest? (for a human image subject)/ Do you think the animal in this image is safe? (for an animal image subject) (Honest/Safe)
–Do think this image has been altered? (Altered)
–Do you think the person in this image is dishonest? (for a human image subject)/ Do you think the animal in this image is unsafe? (for an animal image subject) (Dishonest/Unsafe)
–Do think this image has been unaltered? (Unaltered)
The questions were intended to capture a decision about the subject of the image (human or animal) as well as the features of the image itself (the representation of the subject). The first two questions are the opposite counterparts of the second two. Responses to opposing questions were expected to be unique to each other, based on research showing asymmetry between positive and negatively worded questions [14]. The pairs were combined in the following four ways to form the 4 measurement contexts in a Bell scenario design where two variables (H and D) are related to the content of the image and two variables (A and U ) are related to its representation.
2https://www.iol.co.za/news/world/us-releases-putin-list-13013292.
3https://www.complex.com/life/2017/11/scientists-discover-ancient-shark-with-300- teeth-and-people-want-no-part-of-it.
4Sourced from Pixabay.
5Sourced from Flickr (Igor Shpilenok) under the following license: https:// creativecommons.org/licenses/by/2.0/.
suai.ru/our-contacts |
quantum machine learning |
46 P. D. Bruza and L. Fell
–Honest/Safe (H ) and Altered (A)
–Honest/Safe (H ) and Unaltered (U )
–Dishonest/Unsafe (D) and Altered (A)
–Dishonest/Unsafe (D) and Unaltered (U )
Each image was displayed for 1 s. Placed in a Dual Process context, decisions of trust are assumed to be based on a ective characteristics (the ‘Hot’ system) as well as more rational characteristics (the ‘Cold’ system). The time pressure was designed to access the ‘Hot’ system.
4.3Procedure
Participants were instructed to peruse the task (one condition of the experiment) before agreeing to participate. They were given a test question to ensure they understood instructions and could properly view the images displayed in the task before continuing. This was done by presenting an image of a cat, along with a question asking what animal featured in the image. If the participant could correctly answer this, they were asked to continue to the rest of the task.
Following this question were instructions aimed at introducing the question pair as well as preparing users for the shortness of the image display time. Once understood, users were asked to scroll to the first image. Each image and question group was structured in the following way:
1.A short repeat of image reveal instructions
2.A blank image with the text ‘Click to show image’ Once clicked, this changed to one of the images outlined earlier, with a number beneath counting down from 1 s. Once 1 s had elapsed, the image disappeared.
3.First response: The full question pair is displayed again (the same question pair as in the instructions and accompanying each image of the HIT), as well as a short version (e.g., Safe/Altered). Beneath this was a multiple choice format with the answers:
(a)Yes, Yes
(b)Yes, No
(c)No, Yes
(d)No, No
These were aligned to the short version of the questions to avoid confusion about what each answer referred to.
4.Second response: Participants were then asked to rate, on a slider with a scale from 1 to 7, their confidence in the answers they gave.
5.Third response: Lastly, participants were asked to provide reasoning for the answers they gave in written form. This was to gather further insight into the first response, as well as to determine whether participants correctly understood the questions being asked.
This was repeated for all 4 images, and was followed by a question asking for the country the participants resided in, and a ‘Submit’ button.
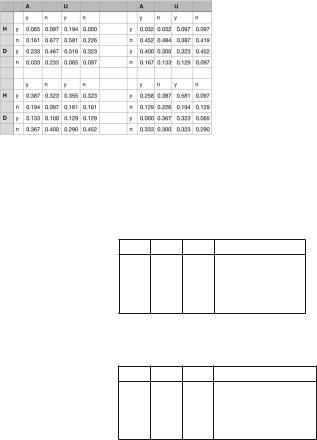
suai.ru/our-contacts |
quantum machine learning |
Are Decisions of Image Trustworthiness Contextual? A Pilot Study |
47 |
Fig. 3. Pairwise probability distributions corresponding to Eq. (1) for Putin (top left), Shark (top right), Girl (bottom left), antelope (bottom right). Variables H - honest/safe, D - dishonest/unsafe, A -altered, U - unaltered
Table 1. Di erences in marginal probabilities: Putin (L) and Shark (R)
|
|
|di | |
z−score (p−val) |
0.161 |
0.194 |
0.032 |
-0.894 (0.896) |
0.733 |
0.839 |
0.105 |
-0.894 (0.381) |
0.226 |
0.300 |
0.074 |
-0.333 (0.751) |
0.774 |
0.581 |
0.194 |
1.344 (0.192) |
|di | z−score (p−val)
0.065 0.194 0.129 -0.430 (0.742)
0.700 0.774 0.074 -0.566 (0.578)
0.484 0.567 0.083 -0.468 (0.647)
0.484 0.452 0.032 0.174 (0.864)
Table 2. Di erences in marginal probabilities: Girl (L) and Antelope (R)
|
|
|di | |
z−score (p−value) |
0.710 |
0.677 |
0.032 |
0.229 (0.821) |
0.233 |
0.258 |
0.025 |
-0.111 (0.915) |
0.581 |
0.5 |
0.081 |
-0.463 (0.649) |
0.516 |
0.419 |
0.097 |
0.519 (0.611) |
|
|
|
|
|di | z−score (p−value)
0.645 0.677 0.032 -0.218 (0.830)
0.367 0.387 0.020 -0.101 (0.922)
0.387 0.333 0.053 0.261 (0.799)
0.774 0.645 0.129 0.945 (0.355)
4.4Results
Tables 1 and 2 show that di erences in marginal probabilities vary across all four images. Z–scores were computed to determine whether the di erences between marginal probabilities were significant. None of these di erences where found to be significant. Table 3 reveals that 0 < 1 for all images which means that the system of CHSH inequalities (11)–(14) can be meaningfully applied to determine the presence of contextuality. The resulting analyses revealed no violations as none of the maximum CHSH values exceeded the degree of contextuality required.
4.5Discussion
The di erences in marginal probabilities reveal that there is signalling between the content and representation decision systems. This is despite the fact the experimental protocol was designed to isolate these sub-systems.