
- •Preface
- •Contents
- •Contributors
- •Modeling Meaning Associated with Documental Entities: Introducing the Brussels Quantum Approach
- •1 Introduction
- •2 The Double-Slit Experiment
- •3 Interrogative Processes
- •4 Modeling the QWeb
- •5 Adding Context
- •6 Conclusion
- •Appendix 1: Interference Plus Context Effects
- •Appendix 2: Meaning Bond
- •References
- •1 Introduction
- •2 Bell Test in the Problem of Cognitive Semantic Information Retrieval
- •2.1 Bell Inequality and Its Interpretation
- •2.2 Bell Test in Semantic Retrieving
- •3 Results
- •References
- •1 Introduction
- •2 Basics of Quantum Probability Theory
- •3 Steps to Build an HSM Model
- •3.1 How to Determine the Compatibility Relations
- •3.2 How to Determine the Dimension
- •3.5 Compute the Choice Probabilities
- •3.6 Estimate Model Parameters, Compare and Test Models
- •4 Computer Programs
- •5 Concluding Comments
- •References
- •Basics of Quantum Theory for Quantum-Like Modeling Information Retrieval
- •1 Introduction
- •3 Quantum Mathematics
- •3.1 Hermitian Operators in Hilbert Space
- •3.2 Pure and Mixed States: Normalized Vectors and Density Operators
- •4 Quantum Mechanics: Postulates
- •5 Compatible and Incompatible Observables
- •5.1 Post-Measurement State From the Projection Postulate
- •6 Interpretations of Quantum Mechanics
- •6.1 Ensemble and Individual Interpretations
- •6.2 Information Interpretations
- •7 Quantum Conditional (Transition) Probability
- •9 Formula of Total Probability with the Interference Term
- •9.1 Växjö (Realist Ensemble Contextual) Interpretation of Quantum Mechanics
- •10 Quantum Logic
- •11 Space of Square Integrable Functions as a State Space
- •12 Operation of Tensor Product
- •14 Qubit
- •15 Entanglement
- •References
- •1 Introduction
- •2 Background
- •2.1 Distributional Hypothesis
- •2.2 A Brief History of Word Embedding
- •3 Applications of Word Embedding
- •3.1 Word-Level Applications
- •3.2 Sentence-Level Application
- •3.3 Sentence-Pair Level Application
- •3.4 Seq2seq Application
- •3.5 Evaluation
- •4 Reconsidering Word Embedding
- •4.1 Limitations
- •4.2 Trends
- •4.4 Towards Dynamic Word Embedding
- •5 Conclusion
- •References
- •1 Introduction
- •2 Motivating Example: Car Dealership
- •3 Modelling Elementary Data Types
- •3.1 Orthogonal Data Types
- •3.2 Non-orthogonal Data Types
- •4 Data Type Construction
- •5 Quantum-Based Data Type Constructors
- •5.1 Tuple Data Type Constructor
- •5.2 Set Data Type Constructor
- •6 Conclusion
- •References
- •Incorporating Weights into a Quantum-Logic-Based Query Language
- •1 Introduction
- •2 A Motivating Example
- •5 Logic-Based Weighting
- •6 Related Work
- •7 Conclusion
- •References
- •Searching for Information with Meet and Join Operators
- •1 Introduction
- •2 Background
- •2.1 Vector Spaces
- •2.2 Sets Versus Vector Spaces
- •2.3 The Boolean Model for IR
- •2.5 The Probabilistic Models
- •3 Meet and Join
- •4 Structures of a Query-by-Theme Language
- •4.1 Features and Terms
- •4.2 Themes
- •4.3 Document Ranking
- •4.4 Meet and Join Operators
- •5 Implementation of a Query-by-Theme Language
- •6 Related Work
- •7 Discussion and Future Work
- •References
- •Index
- •Preface
- •Organization
- •Contents
- •Fundamentals
- •Why Should We Use Quantum Theory?
- •1 Introduction
- •2 On the Human Science/Natural Science Issue
- •3 The Human Roots of Quantum Science
- •4 Qualitative Parallels Between Quantum Theory and the Human Sciences
- •5 Early Quantitative Applications of Quantum Theory to the Human Sciences
- •6 Epilogue
- •References
- •Quantum Cognition
- •1 Introduction
- •2 The Quantum Persuasion Approach
- •3 Experimental Design
- •3.1 Testing for Perspective Incompatibility
- •3.2 Quantum Persuasion
- •3.3 Predictions
- •4 Results
- •4.1 Descriptive Statistics
- •4.2 Data Analysis
- •4.3 Interpretation
- •5 Discussion and Concluding Remarks
- •References
- •1 Introduction
- •2 A Probabilistic Fusion Model of Trust
- •3 Contextuality
- •4 Experiment
- •4.1 Subjects
- •4.2 Design and Materials
- •4.3 Procedure
- •4.4 Results
- •4.5 Discussion
- •5 Summary and Conclusions
- •References
- •Probabilistic Programs for Investigating Contextuality in Human Information Processing
- •1 Introduction
- •2 A Framework for Determining Contextuality in Human Information Processing
- •3 Using Probabilistic Programs to Simulate Bell Scenario Experiments
- •References
- •1 Familiarity and Recollection, Verbatim and Gist
- •2 True Memory, False Memory, over Distributed Memory
- •3 The Hamiltonian Based QEM Model
- •4 Data and Prediction
- •5 Discussion
- •References
- •Decision-Making
- •1 Introduction
- •1.2 Two Stage Gambling Game
- •2 Quantum Probabilities and Waves
- •2.1 Intensity Waves
- •2.2 The Law of Balance and Probability Waves
- •2.3 Probability Waves
- •3 Law of Maximal Uncertainty
- •3.1 Principle of Entropy
- •3.2 Mirror Principle
- •4 Conclusion
- •References
- •1 Introduction
- •4 Quantum-Like Bayesian Networks
- •7.1 Results and Discussion
- •8 Conclusion
- •References
- •Cybernetics and AI
- •1 Introduction
- •2 Modeling of the Vehicle
- •2.1 Introduction to Braitenberg Vehicles
- •2.2 Quantum Approach for BV Decision Making
- •3 Topics in Eigenlogic
- •3.1 The Eigenlogic Operators
- •3.2 Incorporation of Fuzzy Logic
- •4 BV Quantum Robot Simulation Results
- •4.1 Simulation Environment
- •5 Quantum Wheel of Emotions
- •6 Discussion and Conclusion
- •7 Credits and Acknowledgements
- •References
- •1 Introduction
- •2.1 What Is Intelligence?
- •2.2 Human Intelligence and Quantum Cognition
- •2.3 In Search of the General Principles of Intelligence
- •3 Towards a Moral Test
- •4 Compositional Quantum Cognition
- •4.1 Categorical Compositional Model of Meaning
- •4.2 Proof of Concept: Compositional Quantum Cognition
- •5 Implementation of a Moral Test
- •5.2 Step II: A Toy Example, Moral Dilemmas and Context Effects
- •5.4 Step IV. Application for AI
- •6 Discussion and Conclusion
- •Appendix A: Example of a Moral Dilemma
- •References
- •Probability and Beyond
- •1 Introduction
- •2 The Theory of Density Hypercubes
- •2.1 Construction of the Theory
- •2.2 Component Symmetries
- •2.3 Normalisation and Causality
- •3 Decoherence and Hyper-decoherence
- •3.1 Decoherence to Classical Theory
- •4 Higher Order Interference
- •5 Conclusions
- •A Proofs
- •References
- •Information Retrieval
- •1 Introduction
- •2 Related Work
- •3 Quantum Entanglement and Bell Inequality
- •5 Experiment Settings
- •5.1 Dataset
- •5.3 Experimental Procedure
- •6 Results and Discussion
- •7 Conclusion
- •A Appendix
- •References
- •Investigating Bell Inequalities for Multidimensional Relevance Judgments in Information Retrieval
- •1 Introduction
- •2 Quantifying Relevance Dimensions
- •3 Deriving a Bell Inequality for Documents
- •3.1 CHSH Inequality
- •3.2 CHSH Inequality for Documents Using the Trace Method
- •4 Experiment and Results
- •5 Conclusion and Future Work
- •A Appendix
- •References
- •Short Paper
- •An Update on Updating
- •References
- •Author Index
- •The Sure Thing principle, the Disjunction Effect and the Law of Total Probability
- •Material and methods
- •Experimental results.
- •Experiment 1
- •Experiment 2
- •More versus less risk averse participants
- •Theoretical analysis
- •Shared features of the theoretical models
- •The Markov model
- •The quantum-like model
- •Logistic model
- •Theoretical model performance
- •Model comparison for risk attitude partitioning.
- •Discussion
- •Authors contributions
- •Ethical clearance
- •Funding
- •Acknowledgements
- •References
- •Markov versus quantum dynamic models of belief change during evidence monitoring
- •Results
- •Model comparisons.
- •Discussion
- •Methods
- •Participants.
- •Task.
- •Procedure.
- •Mathematical Models.
- •Acknowledgements
- •New Developments for Value-based Decisions
- •Context Effects in Preferential Choice
- •Comparison of Model Mechanisms
- •Qualitative Empirical Comparisons
- •Quantitative Empirical Comparisons
- •Neural Mechanisms of Value Accumulation
- •Neuroimaging Studies of Context Effects and Attribute-Wise Decision Processes
- •Concluding Remarks
- •Acknowledgments
- •References
- •Comparison of Markov versus quantum dynamical models of human decision making
- •CONFLICT OF INTEREST
- •Endnotes
- •FURTHER READING
- •REFERENCES
suai.ru/our-contacts |
quantum machine learning |
72 J. B. Broekaert and J. R. Busemeyer
Table 1. Observed acceptance ratios and unpacking factors, partitioned by cue type, high frequency & concrete (HFC), high frequency & abstract (HFA), low frequency & concrete (LFC), low frequency & abstract (LFA). N = 70. Data set from Table 2, [8]. Predicted acceptance probabilities and unpacking factors from the Hamiltonian-QEM model, RMSE = 0.054737.
|
HFC |
|
|
|
HFA |
|
|
|
LFC |
|
|
|
LFA |
|
|
|
|
|
|
|
|
|
|
|
|
|
|
|
|
|
|
|
|
Obs. |
L1 |
L2 |
L3 |
L4 |
L1 |
L2 |
L3 |
L4 |
L1 |
L2 |
L3 |
L4 |
L1 |
L2 |
L3 |
L4 |
L1? |
0.52 |
0.31 |
0.30 |
0.15 |
0.52 |
0.32 |
0.40 |
0.25 |
0.59 |
0.31 |
0.34 |
0.11 |
0.58 |
0.44 |
0.43 |
0.19 |
L2? |
0.33 |
0.35 |
0.43 |
0.17 |
0.36 |
0.54 |
0.44 |
0.24 |
0.46 |
0.46 |
0.35 |
0.11 |
0.61 |
0.53 |
0.58 |
0.21 |
L3? |
0.38 |
0.35 |
0.42 |
0.21 |
0.37 |
0.38 |
0.48 |
0.24 |
0.41 |
0.34 |
0.49 |
0.13 |
0.53 |
0.38 |
0.52 |
0.17 |
L123? |
0.56 |
0.54 |
0.60 |
0.22 |
0.54 |
0.64 |
0.53 |
0.26 |
0.64 |
0.49 |
0.56 |
0.13 |
0.66 |
0.61 |
0.59 |
0.20 |
UF |
2.20 |
1.89 |
1.91 |
2.45 |
2.32 |
1.96 |
2.49 |
2.83 |
2.31 |
2.31 |
2.10 |
2.77 |
2.59 |
2.19 |
2.61 |
2.86 |
|
|
|
|
|
|
|
|
|
|
|
|
|
|
|
|
|
Pred. |
L1 |
L2 |
L3 |
L4 |
L1 |
L2 |
L3 |
L4 |
L1 |
L2 |
L3 |
L4 |
L1 |
L2 |
L3 |
L4 |
L1? |
0.45 |
0.36 |
0.36 |
0.20 |
0.49 |
0.39 |
0.39 |
0.19 |
0.49 |
0.39 |
0.39 |
0.19 |
0.57 |
0.47 |
0.47 |
0.18 |
L2? |
0.36 |
0.45 |
0.36 |
0.20 |
0.39 |
0.49 |
0.39 |
0.19 |
0.39 |
0.49 |
0.39 |
0.19 |
0.47 |
0.57 |
0.47 |
0.18 |
L3? |
0.36 |
0.36 |
0.45 |
0.20 |
0.39 |
0.39 |
0.49 |
0.19 |
0.39 |
0.39 |
0.49 |
0.19 |
0.47 |
0.47 |
0.57 |
0.18 |
L123? |
0.53 |
0.53 |
0.53 |
0.23 |
0.57 |
0.57 |
0.57 |
0.22 |
0.57 |
0.57 |
0.57 |
0.22 |
0.64 |
0.64 |
0.64 |
0.21 |
UF |
2.18 |
2.18 |
2.18 |
2.70 |
2.24 |
2.24 |
2.24 |
2.65 |
2.24 |
2.24 |
2.24 |
2.64 |
2.35 |
2.35 |
2.35 |
2.52 |
|
|
|
|
|
|
|
|
|
|
|
|
|
|
|
|
|
Table 2. Best-fit parameters for Hamiltonian-QEM (t1 = t2 = π/2).
ν |
ν |
γ |
γHFC |
γHFA |
γLFC |
γLFA |
κ |
−0.6885 |
0.40345 |
0.30631 |
−0.0099825 |
0.022938 |
0.027313 |
0.10107 |
−0.45978 |
4 Data and Prediction
We use the experimental 3-list data (N = 70), reported in Brainerd, Wang and Reyna (Table 2, [8]). The CPD model based ‘bias-correction’ of the acceptance probabilities has been omitted and appear in Table 1. The raw data set was provided by dr. Charles Brainerd. This same data set is used in the CMT model development by Denolf and Lambert-Mogiliansky [15, 16], (their Table 1 shows some typos for L2 cues in the HFA, LFC and LFA set). We optimised the RMSE for 64 data points and Hamiltonian-QEM predictions using 8 param-
eters {μ, μ , γ, γHFC , γHFA, γLFC , γLFA, κ}. Using a 38 grid in parameter space the best fit produced RMSE = 0.054737, for the parameter values in Table 2.
5 Discussion
We explored the over distribution predictions of a dynamical extension of Quantum Episodic Memory model by Brainerd, Wang, Reyna and Nakamura [8, 9] in the 3-list paradigm. The dynamic processing of the initial belief state in our Hamiltonian-QEM results, over time, in outcome states with adequate values of the acceptance probabilities and the unpacking factor for over distribution of memories. The model further predicts systematic higher acceptance probabilities of targets to their proper source list, p(Li?|Li) > p(Lj ?|Li) for (j = i), which
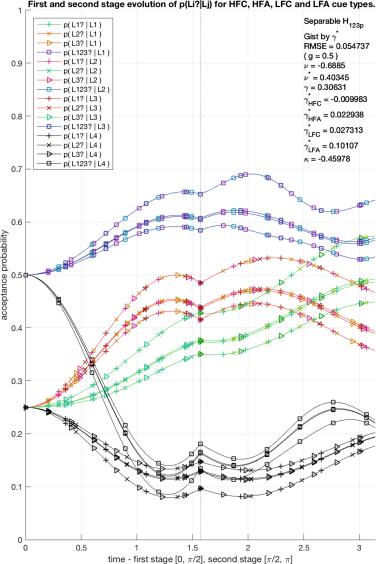
suai.ru/our-contacts |
quantum machine learning |
Hamiltonian-QEM 73
Fig. 2. Optimized two-stage temporal evolution of the acceptance probabilities p(Li ?|Lj ) according the Hamiltonian-QEM model with separable H123p for querying the joint-list, and with equal latent gist and verbatim support in the initial state (g = 0.5).
is curiously not consistently observed in the experimental data set (N = 70). The model relies on four types of transport embedded in the Hamiltonians; the parameters γ and γ regulate transport a ecting the gist-based component, and
suai.ru/our-contacts |
quantum machine learning |
74 J. B. Broekaert and J. R. Busemeyer
the parameters ν and ν regulate transport a ecting the verbatim-based components. The Hamiltonians of the first stage cue recognition phase receive the attenuation parameter κ in the second probe response stage.
The Hamiltonian-QEM model succeeds in a qualitatively good fit with 8 dynamical parameters for the 64 data points of the experimental data set Brainerd et al. [8]. Beyond this exploratory test of the Hamiltonian-QEM for source memory, the model must still be submitted to a comparative statistical test with recent generalisations and modifications of the QEM model, in particular the Generalized-QEM model by Trueblood and Hemmer [25] and Complementary Memory Types model by Denolf and Lambert-Mogiliansky [15, 16, 20].
Acknowledgements. We are grateful to Dr. Charles Brainerd for providing us with the raw data file of the 3-List experiment of [8].
We thank Dr. Peter Kvam for helpful discussions and comments on parts of this manuscript. This research was supported by AFOSR (FA9550-12-1-00397).
References
1.Aerts, D.: Quantum structure in cognition. J. Math. Psychol. 53, 314–348 (2009). https://doi.org/10.1016/j.jmp.2009.04.005
2.Aerts, D., Broekaert, J., Smets, S.: The Liar-paradox in a quantum mechanical perspective. Found. Sci. 4(2), 115–132 (1999). https://doi.org/10.1023/A: 1009610326206
3.Atmanspacher, H., Filk, T.: A proposed test of temporal nonlocality in bistable perception. J. Math. Psychol. 54, 314–321 (2010). https://doi.org/10.1016/j.jmp. 2009.12.001
4.Brainerd, C.J., Reyna, V.F., Mojardin, A.H.: Conjoint recognition. Psychol. Rev. 106(1), 160–179 (1999)
5.Brainerd, C.J., Reyna, V.F.: The Science of False Memory. Oxford UP, New York (2005)
6.Brainerd, C.J., Reyna, V.F.: Episodic over-distribution: a signature e ect of familiarity without recollection. J. Mem. Lang. 58, 765–786 (2008)
7.Brainerd, C.J., Reyna, V.F., Holliday, R.E., Nakamura, K.: Overdistribution in source memory. J. Exp. Psychol. Learn. Mem. Cogn. 38, 413–439 (2012)
8.Brainerd, C.J., Wang, Z., Reyna, V.F.: Superposition of episodic memories: overdistribution and quantum models. Top. Cogn. Sci. 5, 773–799 (2013)
9.Brainerd, C.J., Wang, Z., Ryena, V.F., Nakamura, K.: Episodic memory does not add up: verbatim-gist superposition predicts violations of the additive law of probability. J. Mem. Lang. 84, 224–245 (2015)
10.Broekaert, J, Basieva I, Blasiak P, Pothos EM.: Quantum-like dynamics applied to cognition: a consideration of available options. Phil. Trans. R. Soc. A 375 (2017). https://doi.org/10.1098/rsta.2016.0387
11.Broekaert, J., & Busemeyer, J.R.: A Hamiltonian driven quantum like model for overdistribution in episodic memory recollection. Front. Phys. 5 (2017). https:// doi.org/10.3389/fphy.2017.00023
12.Bruza, P., Kitto, K., Nelson, D., McEvoy, C.: Is there something quantum-like about the human mental lexicon? J. Math. Psy. 53(5), 362–377 (2009). https:// doi.org/10.1016/j.jmp.2009.04.004

suai.ru/our-contacts |
quantum machine learning |
Hamiltonian-QEM 75
13.Busemeyer, J.R., Pothos, E.M., Franco, R., Trueblood, J.S.: A quantum theoretical explanation for probability judgment errors. Psychol. Rev. 118(2), 193 (2011). https://doi.org/10.1037/a0022542
14.Busemeyer, J.R., Bruza, P.D.: Quantum Models of Cognition and Decision Making. Cambridge University Press, Cambridge (2012)
15.Denolf, J.: Subadditivity of episodic memory states: a complementarity approach. In: Atmanspacher, H., Bergomi, C., Filk, T., Kitto, K. (eds.) QI 2014. LNCS, vol. 8951, pp. 67–77. Springer, Cham (2015). https://doi.org/10.1007/978-3-319- 15931-7 6
16.Denolf, J., Lambert-Mogiliansky, A.: Bohr complementarity in memory retrieval.
J.Math. Psychol. 73, 28–36 (2016)
17.Jacoby, L.L.: A process dissociation framework: separating automatic from intentional uses of memory. J. Mem. Lang. 30, 513–541 (1991)
18.Kellen, D., Singmann, H., Klauer, K.C.: Modeling source-memory overdistribution.
J.Mem. Lang. 76, 216–236 (2014)
19.Kvam, P.D., Pleskac, T.J., Yu, S., Busemeyer, J.R.: Interference e ects of choice on confidence: quantum characteristics of evidence accumulation. PNAS 112(34), 10645–10650 (2015). https://doi.org/10.1073/pnas.1500688112
20.Lambert-Mogiliansky, A.: Comments on episodic superposition of memory states. Top. Cogn. Sci. 6, 63–66 (2014). https://doi.org/10.1111/tops.12067
21.Mandler, G.: Recognizing: the judgment of previous occurrence. Psychol. Rev. 87, 252–271 (1980)
22.Mart´ınez-Mart´ınez, I.: A connection between quantum decision theory and quantum games: the Hamiltonian of strategic interaction. J. Math. Psychol. 58, 33–44 (2014). https://doi.org/10.1038/srep23812
23.Nelson, D.L., Kitto, K., Galea, D., McEvoy, C.L., Bruza, P.D.: How activation, entanglement, and searching a semantic network contribute to event memory. Mem. Cogn. 41, 797–819 (2013)
24.Pothos, E.M., Busemeyer, J.R.: Can quantum probability provide a new direction for cognitive modeling? Behav. Brain Sci. 36, 255–327 (2013). https://doi.org/10. 1017/S0140525X12001525
25.Trueblood, J.S., Hemmer, P.: The generalized quantum episodic memory model. Cogn. Sci. 41, 2089–2125 (2017). https://doi.org/10.1111/cogs.12460
26.Tulving, E.: Precis of elements of episodic memory. Behav. Brain Sci. 7(2), 223–268 (1984)
27.Wang Z., Busemeyer, J.R.: A quantum question order model supported by empirical tests of an a priori and precise prediction. Top. Cogn. Sci. 1–22 (2013). https:// doi.org/10.1111/tops.12040
28.Yearsley, J.M., Pothos, E.M.: Zeno’s paradox in decision making. Proc. R. Soc. B 283, 20160291 (2016). https://doi.org/10.1098/rspb.2016.0291