
- •Preface
- •Contents
- •Contributors
- •Modeling Meaning Associated with Documental Entities: Introducing the Brussels Quantum Approach
- •1 Introduction
- •2 The Double-Slit Experiment
- •3 Interrogative Processes
- •4 Modeling the QWeb
- •5 Adding Context
- •6 Conclusion
- •Appendix 1: Interference Plus Context Effects
- •Appendix 2: Meaning Bond
- •References
- •1 Introduction
- •2 Bell Test in the Problem of Cognitive Semantic Information Retrieval
- •2.1 Bell Inequality and Its Interpretation
- •2.2 Bell Test in Semantic Retrieving
- •3 Results
- •References
- •1 Introduction
- •2 Basics of Quantum Probability Theory
- •3 Steps to Build an HSM Model
- •3.1 How to Determine the Compatibility Relations
- •3.2 How to Determine the Dimension
- •3.5 Compute the Choice Probabilities
- •3.6 Estimate Model Parameters, Compare and Test Models
- •4 Computer Programs
- •5 Concluding Comments
- •References
- •Basics of Quantum Theory for Quantum-Like Modeling Information Retrieval
- •1 Introduction
- •3 Quantum Mathematics
- •3.1 Hermitian Operators in Hilbert Space
- •3.2 Pure and Mixed States: Normalized Vectors and Density Operators
- •4 Quantum Mechanics: Postulates
- •5 Compatible and Incompatible Observables
- •5.1 Post-Measurement State From the Projection Postulate
- •6 Interpretations of Quantum Mechanics
- •6.1 Ensemble and Individual Interpretations
- •6.2 Information Interpretations
- •7 Quantum Conditional (Transition) Probability
- •9 Formula of Total Probability with the Interference Term
- •9.1 Växjö (Realist Ensemble Contextual) Interpretation of Quantum Mechanics
- •10 Quantum Logic
- •11 Space of Square Integrable Functions as a State Space
- •12 Operation of Tensor Product
- •14 Qubit
- •15 Entanglement
- •References
- •1 Introduction
- •2 Background
- •2.1 Distributional Hypothesis
- •2.2 A Brief History of Word Embedding
- •3 Applications of Word Embedding
- •3.1 Word-Level Applications
- •3.2 Sentence-Level Application
- •3.3 Sentence-Pair Level Application
- •3.4 Seq2seq Application
- •3.5 Evaluation
- •4 Reconsidering Word Embedding
- •4.1 Limitations
- •4.2 Trends
- •4.4 Towards Dynamic Word Embedding
- •5 Conclusion
- •References
- •1 Introduction
- •2 Motivating Example: Car Dealership
- •3 Modelling Elementary Data Types
- •3.1 Orthogonal Data Types
- •3.2 Non-orthogonal Data Types
- •4 Data Type Construction
- •5 Quantum-Based Data Type Constructors
- •5.1 Tuple Data Type Constructor
- •5.2 Set Data Type Constructor
- •6 Conclusion
- •References
- •Incorporating Weights into a Quantum-Logic-Based Query Language
- •1 Introduction
- •2 A Motivating Example
- •5 Logic-Based Weighting
- •6 Related Work
- •7 Conclusion
- •References
- •Searching for Information with Meet and Join Operators
- •1 Introduction
- •2 Background
- •2.1 Vector Spaces
- •2.2 Sets Versus Vector Spaces
- •2.3 The Boolean Model for IR
- •2.5 The Probabilistic Models
- •3 Meet and Join
- •4 Structures of a Query-by-Theme Language
- •4.1 Features and Terms
- •4.2 Themes
- •4.3 Document Ranking
- •4.4 Meet and Join Operators
- •5 Implementation of a Query-by-Theme Language
- •6 Related Work
- •7 Discussion and Future Work
- •References
- •Index
- •Preface
- •Organization
- •Contents
- •Fundamentals
- •Why Should We Use Quantum Theory?
- •1 Introduction
- •2 On the Human Science/Natural Science Issue
- •3 The Human Roots of Quantum Science
- •4 Qualitative Parallels Between Quantum Theory and the Human Sciences
- •5 Early Quantitative Applications of Quantum Theory to the Human Sciences
- •6 Epilogue
- •References
- •Quantum Cognition
- •1 Introduction
- •2 The Quantum Persuasion Approach
- •3 Experimental Design
- •3.1 Testing for Perspective Incompatibility
- •3.2 Quantum Persuasion
- •3.3 Predictions
- •4 Results
- •4.1 Descriptive Statistics
- •4.2 Data Analysis
- •4.3 Interpretation
- •5 Discussion and Concluding Remarks
- •References
- •1 Introduction
- •2 A Probabilistic Fusion Model of Trust
- •3 Contextuality
- •4 Experiment
- •4.1 Subjects
- •4.2 Design and Materials
- •4.3 Procedure
- •4.4 Results
- •4.5 Discussion
- •5 Summary and Conclusions
- •References
- •Probabilistic Programs for Investigating Contextuality in Human Information Processing
- •1 Introduction
- •2 A Framework for Determining Contextuality in Human Information Processing
- •3 Using Probabilistic Programs to Simulate Bell Scenario Experiments
- •References
- •1 Familiarity and Recollection, Verbatim and Gist
- •2 True Memory, False Memory, over Distributed Memory
- •3 The Hamiltonian Based QEM Model
- •4 Data and Prediction
- •5 Discussion
- •References
- •Decision-Making
- •1 Introduction
- •1.2 Two Stage Gambling Game
- •2 Quantum Probabilities and Waves
- •2.1 Intensity Waves
- •2.2 The Law of Balance and Probability Waves
- •2.3 Probability Waves
- •3 Law of Maximal Uncertainty
- •3.1 Principle of Entropy
- •3.2 Mirror Principle
- •4 Conclusion
- •References
- •1 Introduction
- •4 Quantum-Like Bayesian Networks
- •7.1 Results and Discussion
- •8 Conclusion
- •References
- •Cybernetics and AI
- •1 Introduction
- •2 Modeling of the Vehicle
- •2.1 Introduction to Braitenberg Vehicles
- •2.2 Quantum Approach for BV Decision Making
- •3 Topics in Eigenlogic
- •3.1 The Eigenlogic Operators
- •3.2 Incorporation of Fuzzy Logic
- •4 BV Quantum Robot Simulation Results
- •4.1 Simulation Environment
- •5 Quantum Wheel of Emotions
- •6 Discussion and Conclusion
- •7 Credits and Acknowledgements
- •References
- •1 Introduction
- •2.1 What Is Intelligence?
- •2.2 Human Intelligence and Quantum Cognition
- •2.3 In Search of the General Principles of Intelligence
- •3 Towards a Moral Test
- •4 Compositional Quantum Cognition
- •4.1 Categorical Compositional Model of Meaning
- •4.2 Proof of Concept: Compositional Quantum Cognition
- •5 Implementation of a Moral Test
- •5.2 Step II: A Toy Example, Moral Dilemmas and Context Effects
- •5.4 Step IV. Application for AI
- •6 Discussion and Conclusion
- •Appendix A: Example of a Moral Dilemma
- •References
- •Probability and Beyond
- •1 Introduction
- •2 The Theory of Density Hypercubes
- •2.1 Construction of the Theory
- •2.2 Component Symmetries
- •2.3 Normalisation and Causality
- •3 Decoherence and Hyper-decoherence
- •3.1 Decoherence to Classical Theory
- •4 Higher Order Interference
- •5 Conclusions
- •A Proofs
- •References
- •Information Retrieval
- •1 Introduction
- •2 Related Work
- •3 Quantum Entanglement and Bell Inequality
- •5 Experiment Settings
- •5.1 Dataset
- •5.3 Experimental Procedure
- •6 Results and Discussion
- •7 Conclusion
- •A Appendix
- •References
- •Investigating Bell Inequalities for Multidimensional Relevance Judgments in Information Retrieval
- •1 Introduction
- •2 Quantifying Relevance Dimensions
- •3 Deriving a Bell Inequality for Documents
- •3.1 CHSH Inequality
- •3.2 CHSH Inequality for Documents Using the Trace Method
- •4 Experiment and Results
- •5 Conclusion and Future Work
- •A Appendix
- •References
- •Short Paper
- •An Update on Updating
- •References
- •Author Index
- •The Sure Thing principle, the Disjunction Effect and the Law of Total Probability
- •Material and methods
- •Experimental results.
- •Experiment 1
- •Experiment 2
- •More versus less risk averse participants
- •Theoretical analysis
- •Shared features of the theoretical models
- •The Markov model
- •The quantum-like model
- •Logistic model
- •Theoretical model performance
- •Model comparison for risk attitude partitioning.
- •Discussion
- •Authors contributions
- •Ethical clearance
- •Funding
- •Acknowledgements
- •References
- •Markov versus quantum dynamic models of belief change during evidence monitoring
- •Results
- •Model comparisons.
- •Discussion
- •Methods
- •Participants.
- •Task.
- •Procedure.
- •Mathematical Models.
- •Acknowledgements
- •New Developments for Value-based Decisions
- •Context Effects in Preferential Choice
- •Comparison of Model Mechanisms
- •Qualitative Empirical Comparisons
- •Quantitative Empirical Comparisons
- •Neural Mechanisms of Value Accumulation
- •Neuroimaging Studies of Context Effects and Attribute-Wise Decision Processes
- •Concluding Remarks
- •Acknowledgments
- •References
- •Comparison of Markov versus quantum dynamical models of human decision making
- •CONFLICT OF INTEREST
- •Endnotes
- •FURTHER READING
- •REFERENCES

|
suai.ru/our-contacts |
quantum machine learning |
|
|
|
|
|
|
|
|
|
|
|
|
|
A recent study choice examined the relationship between lateral inhibition and the engagement of prefrontal and parietal areas in intertemporal choices [76]. The authors adapted mechanisms such as lateral inhibition and leakage [16,38] to test how self-control processes emerge when choosing between a smaller–sooner versus a later–larger reward. Hierarchical Bayesian analysis of competing models found that the best account of the decision process was a dynamic, oscillatory feature-selection process [13,49] combined with active suppression of tempting, but inferior, choice options through lateral inhibition [15,16]. More refined single-trial analyses revealed that distinct subregions within the prefrontal cortex (i.e., the dmPFC and the left dlPFC) were associated with inhibition of the tempting but inferior sooner–smaller reward options, consistent with extant theories of cognitive control [77,78].
Future Neuroscientific Research on Value-Based Decisions
With a very few exceptions [76], the neurobiological plausibility of component processes in value-based decisions, such as attentional switching or lateral inhibition, is yet to be tested in a systematic and rigorous fashion. We propose that much additional effort will need to be taken to develop a new agenda of neuroscientific research on multi-attribute value-based decisions. This agenda should be guided by four principles. We have outlined three of these principles above: we need (i) increased reliance on neuroscientific methods such as EEG and MEG that allow capturing the rapidly evolving component processes of multi-attribute decision making, (ii) intensified crosstalk with animal research on evidence accumulation, and (iii) principled use of experimental designs and stimuli that quantify the processing of well-defined attributes (e.g., intertemporal choice options that are characterized by the attributes amount and delay of reward). In addition, we propose that decision neuroscientists need to employ state-of-the-art tools to bridge the gap between cognitive modeling and neural recordings [79–83]. In particular, novel methods have been developed that allow joint modeling of neural and behavioral data such that neural data can directly constrain cognitive models [55,84–86]. These approaches offer a hitherto missing opportunity to dissociate between competing cognitive models and their presumed component processes (which often make highly similar behavioral predictions) on the basis of neurobiological plausibility.
Concluding Remarks
Empirical research on value-based decisions, based on multi-attribute and multi-alternative choices, has produced a collection of puzzling choice context effects that have challenged traditional static theories for over 30 years (Figure 1B and Box 1). The challenging set of empirical regularities was finally successfully addressed by extending classic sequential sampling models of evidence-based decisions into value-based decisions with new mechanisms (Box 3) such as stochastic integration of attribute comparisons, attention weighting depending on attribute values, lateral inhibition, and nonlinear evaluation of comparisons. Although these additional mechanisms enabled computational models to capture important context effects behaviorally, many researchers began the difficult quest of justifying the additional complexity brought on by their inclusion. In the present review we have highlighted several key advantages produced by sequential sampling models of value-based decisions, including temporal evolution of preference, decision times, eye movements, and connections to neural data. Perhaps equally interesting is the discovery of context effects in evidence-based decision paradigms (Box 2), suggesting the possibility of a framework for unifying evidenceand value-based decision making. However, to construct such a framework, we believe that modern cognitive scientists will need to synthesize evidence across both value-based and evidence-based domains to identify when advanced mechanisms affect cognitive dynamics, while looking to the many technological advances for better appreciating the temporal aspects of the computations of the mind (see[176T$DIF] Outstanding Questions for future issues).
Outstanding Questions
Do the new findings of context effects in perception and inference tasks require switching from simpler models previously used in evidence-based tasks to adopting some of the more advanced mechanisms developed for value-based decisions?
Is it possible to form a single sequential sampling model that can be applied to both value-based and inferencebased tasks, or are these domains of application so different that different models are needed for each one?
The advanced mechanisms used in value-based sequential sampling models introduce a very high level of complexity in these models. How can we develop rigorously empirical tests for these complex models?
Similarly, how can we avoid that these advanced mechanisms make models too complex and impractical for application to field research in marketing and consumer behavior?
What methodological advances will be necessary to investigate complex decision dynamics? Both precise spatial and temporal information about neural computations are essential. Currently, methods for combining high spatial and temporal modalities (e.g., EEG and fMRI) are complicated to implement. How will development in sig- nal-processing techniques change cognitive theories of decision making?
260 Trends in Cognitive Sciences, March 2019, Vol. 23, No. 3
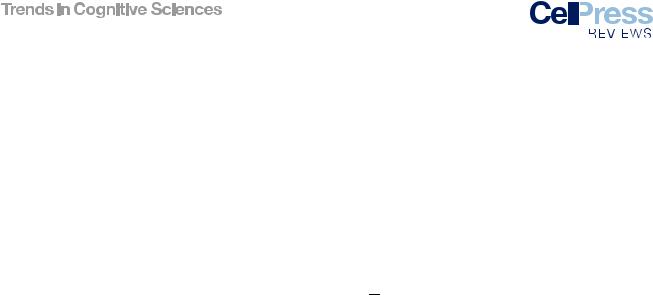
suai.ru/our-contacts |
quantum machine learning |
|
|
|
|
|
|
|
|
|
|
|
|
|
|
Finally, some initial progress has been made recently in uncovering the neural substrates underlying the advanced mechanisms used by sequential sampling models of value-based decision, for instance by linking lateral inhibition of choice options to the cognitive control system of the brain [76]. However, most of the advanced mechanisms that have been put forward in cognitive research on value-based decisions making are yet to be linked to brain data. More work and effort is needed in this regard, and we have delineated an agenda for future research. The ultimate goal is use neural data to constrain cognitive models [83] to identify a neurobiologically plausible account of value-based decision making and to avoid further inflation of proposals of sequential sampling models that are able to explain context effects.
Acknowledgments
J.R.B. was supported by the Air Force Office of Scientific Research (FA9550-15-1-0343), S.G, and J.R. were supported by
the Swiss National Science Foundation (100014-172761 and 100014-153616, respectively)]FID$T71[.
References
1.Ratcliff, R. et al. (2016) Diffusion decision model: current history and issues. Trends Cogn. Sci. 20, 260–281
2.Forstmann, B.U. et al. (2016) Sequential sampling models in cognitive neuroscience: advantages, applications, and extensions. Annu. Rev. Psychol. 67, 641–666
3.Hanks, T.D. and Summerfield, C. (2017) Perceptual decision making in rodents, monkeys, and humans. Neuron 93, 15–31
4.Link, S.W. (1975) The relative judgment theory of two choice response time. J. Math. Psychol. 12, 114–135
5.Vickers, D. (1979) Decision Processes in Perception, Academic Press
6.Laming, D.R. (1968) Information Theory of Choice Reaction Time, Wiley
7.Ratcliff, R. (1978) A theory of memory retrieval. Psychol. Rev. 85, 59–108
8.Nosofsky, R.M. and Palmeri, T.J. (1997) An exemplar-based random walk model of speeded classification. Psychol. Rev. 104, 266
9.Ashby, F.G. (2000) A stochastic version of general recognition theory. J. Math. Psychol. 44, 310–329
10.Latimer, K. et al. (2015) Single-trial spike trains in parietal cortex reveal discrete steps during decision-making. Science 349, 184–187
11.Shadlen, M.N. and Newsome, W.T. (2001) Neural basis of a perceptual decision in the parietal cortex (area LIP) of the rhesus monkey. J. Neurophysiol. 86, 1916–1936
12.Schall, J.D. (2003) Neural correlates of decision processes: neural and mental chronometry. Curr. Opin. Neurobiol. 12, 182–186
13.Busemeyer, J. and Townsend, J. (1993) Decision field theory: a dynamic-cognitive approach to decision making in an uncertain environment. Psychol. Rev. 100, 432–432
14.Roe, R. et al. (2001) Multialternative decision field theory: a dynamic connectionist model of decision making. Psychol. Rev. 108, 370–392
15.Usher, M. and McClelland, J.L. (2001) The time course of perceptual choice: the leaky, competing accumulator model.
Psychol. Rev. 108, 550–592
16.Usher, M. and McClelland, J.L. (2004) Loss aversion and inhibition in dynamical models of multialternative choice. Psychol. Rev. 111, 757–769
17.Tsetsos, K. et al. (2010) Preference reversal in multiattribute choice. Psychol. Rev. 117, 1275
18.Krajbich, I. et al. (2010) Visual fixations and the computation and comparison of value in simple choice. Nat. Neurosci. 13, 1292
19.Krajbich, I. and Rangel, A. (2011) Multialternative drift-diffusion model predicts therelationship between visual fixationsandchoice in value-based choice. Proc. Natl. Acad. Sci. 108, 13852–13857
20.Tsetsos, K. et al. (2012) Salience driven value integration explains decision biases and preference reversal. Proc. Natl. Acad. Sci. 109, 9659–9664
21.Bhatia, S. (2013) Associations and the accumulation of preference. Psychol. Rev. 120, 522–543
22.Trueblood, J.S. et al. (2014) The multiattribute linear ballistic accumulator model of context effects in multialternative choice.
Psychol. Rev. 121, 179–205
23.Noguchi, T. and Stewart, N. (2018) Multialternative decision by sampling: a model of decision making constrained by process data. Psychol. Rev. 125, 512–544
24.Rieskamp, J. et al. (2006) Extending the bounds of rationality: evidence and theories of preferential choice. J. Econ. Lit. 44, 631–661
25.Luce, R.D. (1977) The choice axiom after twenty years. J. Math. Psychol. 15, 215–233
26.Train, K.E. (2009) Discrete Choice Methods with Simulation,
Cambridge University Press
27.Tversky, A. (1972) Elimination by aspects: a theory of choice.
Psychol. Rev. 79, 281
28.Cataldo, A.M. and Cohen, A.L. (2018) Reversing the similarity effect: the effect of presentation format. Cognition 175, 141–156
29.Dhar, R. et al. (2004) Toward extending the compromise effect to complex buying contexts. J. Mark. Res. 41, 258–261
30.Farmer, G.D. et al. (2017) The effect of expected value on attraction effect preference reversals. J. Behav. Decis. Mak. 30, 785–793
31.Heath, T.B. and Chatterjee, S. (1995) Asymmetric decoy effects on lower-quality versus higher-quality brands: meta-analytic and experimental evidence. J. Consum. Res. 22, 268–284
32.Huber, J. et al. (1982) Adding asymmetrically dominated alternatives: violations of regularity and the similarity hypothesis. J. Consum. Res. 9, 90–98
33.Huber, J. et al. (2014) Let’s be honest about the attraction effect.
J. Mark. Res. 51, 520–525
34.Wedell, D.H. (1991) Distinguishing among models of contextually induced preference reversals. J. Exp. Psychol. Lear. Mem. Cogn. 17, 767–778
35.Simonson, I. (1989) Choice based on reasons: the case of attraction and compromise effects. J. Consum. Res. 16, 158–174
36.Simonson, I. and Tversky, A. (1992) Choice in context: tradeoff contrast and extremeness aversion. J. Mark. Res. 29, 281–295
37.Tversky, A. and Kahneman, D. (1991) Loss aversion in riskless choice: a reference-dependent model. Q. J. Econ. 106, 1039– 1061
38.Roe, R. et al. (2001) Multi-alternative decision field theory: a dynamic connectionist model of decision making. Psychol. Rev. 108, 370–392
39.Dhar, R. and Nowlis, S.M. (1999) The effect of time pressure on consumer choice deferral. J. Consum. Res. 25, 369–384
Trends in Cognitive Sciences, March 2019, Vol. 23, No. 3 261

|
suai.ru/our-contacts |
quantum machine learning |
|
|
|
|
|
|
|
|
|
|
|
|
|
40.Pettibone, J.C. (2012) Testing the effect of time pressure on asymmetric dominance and compromise decoys in choice.
Judgm. Decis. Mak. 7, 513–523
41.Gluth, S. et al. (2018) Value-based attentional capture affects multi-alternative decision making. eLife 7, e39659
42.Tversky, A. and Simonson, I. (1993) Context-dependent preferences. Manag. Sci. 39, 1179–1189
43.Bhatia, S. (2017) Comparing theories of reference-dependent choice. J. Exp. Psychol. Learn. Mem. Cogn. 43, 1490–1507
44.Howes, A. et al. (2016) Why contextual preference reversals maximize expected value. Psychol. Rev. 123, 368–391
45.Soltani, A. et al. (2012) A range-normalization model of contextdependent choice: a new model and evidence. PLoS Comput. Biol. 8, e1002607
46.Ronayne, D. and Brown, G.D. (2017) Multi-attribute decision by sampling: an account of the attraction, compromise and similarity effects. J. Math. Psychol. 81, 11–27
47.Rigoli, F. et al. (2017) A unifying Bayesian account of contextual effects in value-based choice. PLoS Comput. Biol. 13, e1005769
48.Turner, B.M. et al. (2018) Competing theories of multialternative, multiattribute preferential choice. Psychol. Rev. 125, 329–362
49.Dai, J. and Busemeyer, J.R. (2014) A probabilistic, dynamic, and attribute-wise model of intertemporal choice. J. Exp. Psychol. Gen. 143, 1489–1514
50.Diederich, A. (2003) Mdft account of decision making under time pressure. Psychon. Bull. Rev. 10, 157–166
51.Diederich, A. (2003) Decision making under conflict: decision time as a measure of conflict strength. Psychon. Bull. Rev. 10, 167–176
52.Krajbich, I. et al. (2012) The attentional drift-diffusion model extends to simple purchasing decisions. Front. Psychol. 3, 193
53.Molloy, M.F. et al. (2018) What is in a response time? On the importance of response time measures in constraining models of context effects. Decision Published online July 16, 2018. http://dx.doi.org/10.1037/dec0000097
54.Mullett, T.L. and Stewart, N. (2016) Implications of visual attention phenomena for models of preferential choice. Decision 3, 231–253
55.Turner, B.M. et al. (2016) Why more is better: a method for simultaneously modeling EEG, fMRI, and behavior. Neuroimage 128, 96–115
56.Basten, U. et al. (2010) How the brain integrates costs and benefits during decision making. Proc. Natl. Acad. Sci. 107, 21767–21772
57.Gluth, S. et al. (2012) Deciding when to decide: time-variant sequential sampling models explain the emergence of valuebased decisions in the human brain. J. Neurosci. 32, 10686– 10698
58.Hare, T.A. et al. (2011) Transformation of stimulus value signals into motor commands during simple choice. Proc. Natl. Acad. Sci. 108, 18120–18125
59.Hunt, L.T. et al. (2012) Mechanisms underlying cortical activity during value-guided choice. Nat. Neurosci. 15, 470–476
60.Clithero, J.A. and Rangel, A. (2014) Informatic parcellation of the network involved in the computation of subjective value. Soc. Cogn. Affect. Neurosci. 9, 1289–1302
61.Levy, D.J. and Glimcher, P.W. (2012) The root of all value: a neural common currency for choice. Curr. Opin. Neurobiol. 22, 1027–1038
62.Strait, C.E. et al. (2014) Reward value comparison via mutual inhibition in ventromedial prefrontal cortex. Neuron 82, 1357– 1366
63.Gluth, S. et al. (2015) Effective connectivity between hippocampus and ventromedial prefrontal cortex controls preferential choices from memory. Neuron 86, 1078–1090
64.Pisauro, M.A. et al. (2017) Neural correlates of evidence accumulation during value-based decisions revealed via simultaneous EEG-fMRI. Nat. Commun. 8, 15808
65.Chau, B.K.H. et al. (2014) A neural mechanism underlying failure of optimal choice with multiple alternatives. Nat. Neurosci. 17, 463–470
66.Polanía, R. et al. (2014) Neural oscillations and synchronization differentially support evidence accumulation in perceptual and value-based decision making. Neuron 82, 709–720
67.Brunton, B.W. et al. (2013) Rats and humans can optimally accumulate evidence for decision-making. Science 340, 95–98
68.Hanks, T.D. et al. (2015) Distinct relationships of parietal and prefrontal cortices to evidence accumulation. Nature 520, 220– 223
69.Chung, H.-K. et al. (2017) Why do irrelevant alternatives matter? An fMRI-TMS study of context-dependent preferences. J. Neurosci. 37, 11647–11661
70.Hedgcock, W. and Rao, A.R. (2009) Trade-off aversion as an explanation for the attraction effect: a functional magnetic resonance imaging study. J. Mark. Res. 46, 1–13
71.Hu, J. and Yu, R. (2014) The neural correlates of the decoy effect in decisions. Front. Behav. Neurosci. 8, 271
72.Mohr, P.N.C. et al. (2017) Attraction effect in risky choice can be explained by subjective distance between choice alternatives.
Sci. Rep. 7, 8942
73.Gluth, S. et al. (2017) The attraction effect modulates reward prediction errors and intertemporal choices. J. Neurosci. 37, 371–382
74.Menon, V. and Uddin, L.Q. (2010) Saliency, switching, attention and control: a network model of insula function. Brain Struct. Funct. 214, 655–667
75.Hunt, L.T. et al. (2014) Hierarchical competitions subserving multi-attribute choice. Nat. Neurosci. 17, 1613
76.Turner, B.M. et al. (2018) On the neural and mechanistic bases of self-control. Cereb. Cortex 1–19
77.Botvinick, M.M. et al. (2001) Conflict monitoring and cognitive control. Psychol. Rev. 108, 624–652
78.Botvinick, M.M. et al. (2004) Conflict monitoring and anterior cingulate cortex: an update. Trends Cogn. Sci. 8, 539–546
79.Gluth, S. and Rieskamp, J. (2017) Variability in behavior that cognitive models do not explain can be linked to neuroimaging data. J. Math. Psychol. 76, 104–116
80.Turner, B.M. et al. (2017) Approaches to analysis in modelbased cognitive neuroscience. J. Math. Psychol. 76, 65–79
81.Purcell, B.A. et al. (2010) Neurally constrained modeling of perceptual decision making. Psychol. Rev. 117, 1113
82.Anderson, J.R. et al. (2008) Using fMRI to test models of complex cognition. Cogn. Sci. 32, 1323–1348
83.Turner, B.M. et al. (2013) A Bayesian framework for simultaneously modeling neural and behavioral data. Neuroimage 72, 193–206
84.Turner, B.M. et al. (2017) Approaches to analysis in modelbased cognitive neuroscience. J. Math. Psychol. 76, 65–79
85.van Ravenzwaaij, D. et al. (2017) A confirmatory approach for integrating neural and behavioral data into a single model. J. Math. Psychol. 76, 131–141
86.Turner, B.M. et al. (2015) Informing cognitive abstractions through neuroimaging: the neural drift diffusion model. Psychol. Rev. 122, 312
87.Berkowitsch, N.A. et al. (2014) Rigorously testing multialternative decision field theory against random utility models. J. Exp. Psychol. Gen. 143, 1331–1348
88.Hancock, T.O. et al. (2018) Decision field theory: improvements to current methodology and comparisons with standard choice modelling techniques. Transp. Res. B Methodol. 107, 18–40
89.Hotaling, J.M. and Rieskamp, J. (2018) A quantitative test of computational models of multialternative context effects. Decision Published online July 12, 2018. http://dx.doi.org/10.1037/ dec0000096
90.Liew, S.X. et al. (2016) The appropriacy of averaging in the study of context effects. Psychon. Bull. Rev. 23, 1639–1646
262 Trends in Cognitive Sciences, March 2019, Vol. 23, No. 3

suai.ru/our-contacts |
quantum machine learning |
|
|
|
|
|
|
|
|
|
|
|
|
|
|
91.Hutchinson, J.W. et al. (2000) Unobserved heterogeneity as an alternative explanation for reversal effects in behavioral research.
J. Consum. Res. 27, 324–344
92.Trueblood, J.S. et al. (2015) The fragile nature of contextual preference reversals: reply to Tsetsos, Chater, and Usher (2015). Psychol. Rev. 122, 848–853
93.Trueblood, J.S. et al. (2013) Not just for consumers: context effects are fundamental to decision making. Psychol. Sci. 24, 901–908
94.Dutilh, G. and Rieskamp, J. (2016) Comparing perceptual and preferential decision making. Psychon. Bull. Rev. 23, 723–737
95.Hotaling, J.M. et al. (2010) Theoretical developments in decision field theory: comment on Tsetsos, Usher, and Chater (2010).
Psychol. Rev. 117, 1294–1298
96.Turner, B.M. et al. (2013) A method for efficiently sampling from distributions with correlated dimensions. Psychol. Methods 18, 368–384
97.Morey, R.D. et al. (2016) The philosophy of Bayes factors and the quantification of statistical evidence. J. Math. Psychol. 72, 6–18
98.Turner, B.M. and Van Zandt, T. (2012) A tutorial on approximate Bayesian computation. J. Math. Psychol. 56, 69–85
99.Turner, B.M. and Sederberg, P.B. (2012) Approximate Bayesian computation with differential evolution. J. Math. Psychol. 56, 375–385
100.Turner, B.M. and Sederberg, P.B. (2014) A generalized, likeli- hood-free method for parameter estimation. Psychon. Bull. Rev. 21, 227–250
101.Turner, B.M. et al. (2016) Bayesian analysis of simulation-based models. J. Math. Psychol. 72, 191–199
102.Turner, B.M. et al. (2013) Bayesian analysis of memory models.
Psychon. Bull. Rev. 120, 667–678
103.Turner, B.M. and Van Zandt, T. (2014) Hierarchical approximate Bayesian computation. Psychometrika 79, 185–209
Trends in Cognitive Sciences, March 2019, Vol. 23, No. 3 263