
- •Contents
- •Preface
- •1.1 Smart Antenna Architecture
- •1.2 Overview of This Book
- •1.3 Notations
- •2.1 Single Transmit Antenna
- •2.1.1 Directivity and Gain
- •2.1.2 Radiation Pattern
- •2.1.3 Equivalent Resonant Circuits and Bandwidth
- •2.2 Single Receive Antenna
- •2.3 Antenna Array
- •2.4 Conclusion
- •Reference
- •3.1 Introduction
- •3.2 Data Model
- •3.2.1 Uniform Linear Array (ULA)
- •3.3 Centro-Symmetric Sensor Arrays
- •3.3.1 Uniform Linear Array
- •3.3.2 Uniform Rectangular Array (URA)
- •3.3.3 Covariance Matrices
- •3.4 Beamforming Techniques
- •3.4.1 Conventional Beamformer
- •3.4.2 Capon’s Beamformer
- •3.4.3 Linear Prediction
- •3.5 Maximum Likelihood Techniques
- •3.6 Subspace-Based Techniques
- •3.6.1 Concept of Subspaces
- •3.6.2 MUSIC
- •3.6.3 Minimum Norm
- •3.6.4 ESPRIT
- •3.7 Conclusion
- •References
- •4.1 Introduction
- •4.2 Preprocessing Schemes
- •4.2.2 Spatial Smoothing
- •4.3 Model Order Estimators
- •4.3.1 Classical Technique
- •4.3.2 Minimum Descriptive Length Criterion
- •4.3.3 Akaike Information Theoretic Criterion
- •4.4 Conclusion
- •References
- •5.1 Introduction
- •5.2 Basic Principle
- •5.2.1 Signal and Data Model
- •5.2.2 Signal Subspace Estimation
- •5.2.3 Estimation of the Subspace Rotating Operator
- •5.3 Standard ESPRIT
- •5.3.1 Signal Subspace Estimation
- •5.3.2 Solution of Invariance Equation
- •5.3.3 Spatial Frequency and DOA Estimation
- •5.4 Real-Valued Transformation
- •5.5 Unitary ESPRIT in Element Space
- •5.6 Beamspace Transformation
- •5.6.1 DFT Beamspace Invariance Structure
- •5.6.2 DFT Beamspace in a Reduced Dimension
- •5.7 Unitary ESPRIT in DFT Beamspace
- •5.8 Conclusion
- •References
- •6.1 Introduction
- •6.2 Performance Analysis
- •6.2.1 Standard ESPRIT
- •6.3 Comparative Analysis
- •6.4 Discussions
- •6.5 Conclusion
- •References
- •7.1 Summary
- •7.2 Advanced Topics on DOA Estimations
- •References
- •Appendix
- •A.1 Kronecker Product
- •A.2 Special Vectors and Matrix Notations
- •A.3 FLOPS
- •List of Abbreviations
- •About the Authors
- •Index
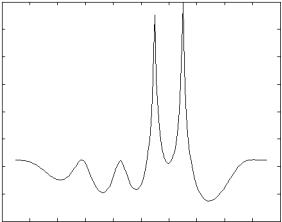
52 |
Introduction to Direction-of-Arrival Estimation |
of 10 dB was assumed. Figure 3.7 shows the result. In comparison with Figure 3.6, the linear prediction method performs better than Capon’s method, with defined peaks at 10° and 30°. However, the sidelobes are more prominent. In addition, the formula is more complex, requiring slightly more computing time and power. In our tests, the best resolution achieved was found to be 7°.
3.5 Maximum Likelihood Techniques
Maximum likelihood (ML) techniques were some of the first techniques investigated for DOA estimation [11]. Since ML techniques were computationally intensive, they are less popular than other techniques. However, in terms of performance, they are superior to other estimators, especially at low SNR conditions.
Given an received data sequence x(tn), it is desired to reconstruct the components of the data due only to the desired signals. The parameter values for which the reconstruction approximates the received data with maximal accuracy are taken to be the DOA and desired signal waveform estimates. The approach taken here is to subtract from x(tn) an estimate
A(θ)s(t n |
) of the signal components A(θ)s(tn). If the estimates θ and |
|
|
Magnitude (dB)
Linear projection
40
30
20
10
0
−10
−20
−30
−40 −100 −80 −60 −40 −20 0 20 40 60 80 100
Angle (degree)
Figure 3.7 DOA estimation with the linear prediction; the signal impinges at 10° and 30°.

Overview of Basic DOA Estimation Algorithms |
53 |
|
|
s(t n ) are sufficiently good, the residual x(t n ) − A(θ)s(t n ) will consist primarily of noise and interference with smallest energy. In other words, minimizing the energy in the residual x(t n ) − A(θ)s(t n ) by proper choice of θ and s(t n ) can result in accurate estimates of θi ≈ θ and s(t n ) ≈ s(t n ).
The method can be stated mathematically in a least-squares form as
min |
|
|
|
|
x(t n |
) − A(θ)s(t n ) |
|
2 |
|
|
|
|
|
(3.48) |
|||
|
|
|
|
|
|
|
|
|
θ , s (t n |
) |
|
|
|
|
|
|
|
|
|
|
|
|
|
N |
||
|
|
|
|
|
for which the best least squares fit between the received signals and a reconstruction of the signal components is sought. After mathematical manipulations, the solution for s(t n ) in terms of any θ can be found as
s(t n ) = (A |
H |
|
|
−1 |
H |
|
) = W |
H |
x(t n |
) (3.49) |
|
|
A |
|
|||||||
|
(θ)A(θ)) |
|
(θ)x(t n |
|
||||||
|
|
|
|
|
|
|
|
|
|
|
By substituting this signal estimate back into the residual and minimizing it over the DOA estimates, θ can be shown to be equivalent to maximizing a matrix trace
max trace{PA (θ)R xx }
θ
where PA (θ) is the matrix for the space spanned by the columns of A(θ),
PA (θ)= A(θ)(A |
H |
(θ)A(θ)) |
−1 |
H |
(θ) |
(3.51) |
||
A |
||||||||
|
|
|
|
|
|
|
If θ is the exact solution (direction of the arrival), the reconstructed data A(θ)s(n) is equal to the true signal components plus residential noise,
X(n ) − A(θ)S(n ) = X(n ) − PA (θ)X(n )
=(A(θ)S(n ) + N)− (A(θ)S(n ) + PA (θ)N) (3.52)
=(I − PA (θ))N