
1manly_b_f_j_statistics_for_environmental_science_and_managem
.pdf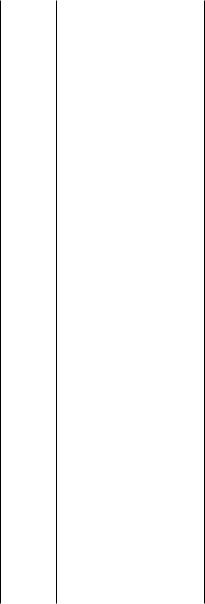
86 Statistics for Environmental Science and Management, Second Edition
Table 3.11 |
SomeCommonlyFittedGeneralizedLinearModels |
|
Inverse |
Gaussian |
Y |
|
Gamma |
Distributionsfor |
|
Binomial |
|
|
Poisson |
|
|
Normal |
Model Equation
Model Name
default allowed doubtful allowed allowed
ε+
i X i β∑
regressionLinear =Y
allowed default doubtful allowed allowed
ε+
) i X i β∑ exp(=
Y
Log-linear
not possible default doubtful doubtful
doubtful
ε+
)} i X i β∑ exp(+
)/{1 i X i β∑ exp(=
Y /n
regressionLogistic
allowed allowed doubtful default allowed
ε+
) i X i β∑ 1/(=
Y
Reciprocal
doubtful not possible allowed doubtful doubtful
ε+
) i X i β∑ ϕ(
/Y =n
Probit
doubtful not possible allowed doubtful doubtful
ε+
)} i X i β∑ exp{(exp(–
1=
Y /n
exponentialDouble
allowed allowed doubtful allowed allowed
ε+
)2 i X i β∑ (=
Y
Square
a and b constants allowed allowed doubtful allowed allowed
+b ε,
+1/a ) i X i β∑ (=
Y
Exponential
allowed allowed doubtful allowed default
ε+ }1⁄2
i X i β∑ 1/{=
Y
Square inverseroot
Models for Data |
87 |
The individual estimates in a generalized linear model can also be tested to see whether they are significantly different from zero. This just involves comparing the estimate divided by its estimated standard error,
z = b/SÊ(b)
with critical values for the standard normal distribution. Thus if the absolute value of z exceeds 1.96, then the estimate is significantly different from zero at about the 5% level.
One complication that can occur with some generalized linear models is overdispersion, with the differences between the observed and fitted values of the dependent variable being much larger than what is expected from the assumed error distribution. There are ways of handling this should it arise. For more information on this and other aspects of these models, see McCullagh and Nelder (1989) or Dobson (2001).
Example 3.3: Dolphin Bycatch in Trawl Fisheries
The accidental capture of marine mammals and birds in commercial fishing operations is of considerable concern in many fisheries around the world. This example concerns one such situation, which is of catches of the common dolphin (Delphinus delphis) and the bottlenose dolphin (Tursiops truncatus) in the Taranaki Bight trawl fishery for jack mackerel (Trachurus declivis, T. novaezelandiae, and T. murphyi) off the west coast of New Zealand.
The New Zealand Ministry of Fisheries puts official observers on a sample of fishing vessels to monitor dolphin bycatch, and Table 3.12 shows a summary of the data collected by these observers for the six fishing seasons 1989/90 to 1994/95, as originally published by Baird (1996, Table 3). The table shows the number of observed trawls and the number of dolphins accidentally killed, categorized by up to eight conditions for each fishing year. These are the fishing area (the northern or southern Taranaki Bight), the gear type (bottom or midwater), and the time (day or night). Excluding five cases where there were no observed trawls, this gives 43 observations on the bycatch under different conditions, in different years. Some results from fitting a generalized linear model to these data are also shown in the last two columns of the table.
The data in Table 3.12 will be used here to examine how the amount of bycatch varies with the factors recorded (year, area, gear type, and time of day). Because the dependent variable (the number of dolphins killed) is a count, it is reasonable to try fitting the data using a log-linear model with Poisson errors. A simple model of that type for the ith count is
Yi = Ti exp[α(fi) + β1Xi1 + β2Xi2 + β3Xi3] + εi |
(3.36) |
where Ti is the number of tows involved; α(fi) depends on the fishing year fi when the observation was collected in such a way that α(fi) = α(1) for observations in 1989/90, α(fi) = α(2) for observations in 1990/91, and so on up to α(fi) = α(6) for observations in 1994/95; Xi1 is 0 for North Taranaki and 1 for South Taranaki; Xi2 is 0 for bottom trawls and 1 for

88 Statistics for Environmental Science and Management, Second Edition
Table 3.12
Bycatch of Dolphins in the Taranaki Bight Trawl Fishery for Jack Mackerel on Tows Officially Observed
|
|
Gear |
|
|
Dolphins Killed |
|
|
|
|
|
|
|
|
|
|
Season |
Area |
Type |
Time |
Tows |
Observed |
Fitted |
Ratea |
1989/90 |
North |
Bottom |
Day |
48 |
0 |
0.0 |
0.1 |
|
North |
Bottom |
Night |
6 |
0 |
0.0 |
0.6 |
|
North |
Midwater |
Night |
1 |
0 |
0.0 |
3.9 |
|
South |
Bottom |
Day |
139 |
0 |
0.6 |
0.4 |
|
South |
Midwater |
Day |
6 |
0 |
0.2 |
2.8 |
|
South |
Bottom |
Night |
6 |
0 |
0.2 |
3.6 |
|
South |
Midwater |
Night |
90 |
23 |
21.9 |
24.4 |
1990/91 |
North |
Bottom |
Day |
2 |
0 |
0.0 |
0.0 |
|
South |
Bottom |
Day |
47 |
0 |
0.0 |
0.0 |
|
South |
Midwater |
Day |
110 |
0 |
0.0 |
0.0 |
|
South |
Bottom |
Night |
12 |
0 |
0.0 |
0.0 |
|
South |
Midwater |
Night |
73 |
0 |
0.0 |
0.0 |
1991/92 |
North |
Bottom |
Day |
101 |
0 |
0.4 |
0.4 |
|
North |
Midwater |
Day |
4 |
0 |
0.1 |
2.8 |
|
North |
Bottom |
Night |
36 |
2 |
1.3 |
3.6 |
|
North |
Midwater |
Night |
3 |
5 |
0.7 |
24.3 |
|
South |
Bottom |
Day |
74 |
1 |
1.9 |
2.5 |
|
South |
Midwater |
Day |
3 |
0 |
0.5 |
17.1 |
|
South |
Bottom |
Night |
7 |
5 |
1.5 |
22.1 |
|
South |
Midwater |
Night |
15 |
16 |
22.6 |
150.4 |
1992/93 |
North |
Bottom |
Day |
135 |
0 |
0.1 |
0.1 |
|
North |
Midwater |
Day |
3 |
0 |
0.0 |
0.5 |
|
North |
Bottom |
Night |
22 |
0 |
0.1 |
0.6 |
|
North |
Midwater |
Night |
16 |
0 |
0.7 |
4.2 |
|
South |
Bottom |
Day |
112 |
0 |
0.5 |
0.4 |
|
South |
Bottom |
Night |
6 |
0 |
0.2 |
3.9 |
|
South |
Midwater |
Night |
28 |
9 |
7.4 |
26.3 |
1993/94 |
North |
Bottom |
Day |
78 |
0 |
0.0 |
0.0 |
|
North |
Midwater |
Day |
19 |
0 |
0.0 |
0.2 |
|
North |
Bottom |
Night |
13 |
0 |
0.0 |
0.2 |
|
North |
Midwater |
Night |
28 |
0 |
0.4 |
1.6 |
|
South |
Bottom |
Day |
155 |
0 |
0.2 |
0.2 |
|
South |
Midwater |
Day |
20 |
0 |
0.2 |
1.1 |
|
South |
Bottom |
Night |
14 |
0 |
0.2 |
1.4 |
|
South |
Midwater |
Night |
71 |
8 |
6.8 |
9.6 |
1994/95 |
North |
Bottom |
Day |
17 |
0 |
0.0 |
0.1 |
|
North |
Midwater |
Day |
80 |
0 |
0.3 |
0.4 |
|
North |
Bottom |
Night |
9 |
0 |
0.0 |
0.5 |
|
North |
Midwater |
Night |
74 |
0 |
2.5 |
3.4 |

Models for Data |
89 |
Table 3.12 (continued)
Bycatch of Dolphins in the Taranaki Bight Trawl Fishery for Jack Mackerel on Tows Officially Observed
|
|
Gear |
|
|
Dolphins Killed |
|
|
|
|
|
|
|
|
|
|
Season |
Area |
Type |
Time |
Tows |
Observed |
Fitted |
Ratea |
|
South |
Bottom |
Day |
41 |
0 |
0.1 |
0.4 |
|
South |
Midwater |
Day |
73 |
6 |
1.8 |
2.4 |
|
South |
Bottom |
Night |
13 |
0 |
0.4 |
3.1 |
|
South |
Midwater |
Night |
74 |
15 |
15.8 |
21.3 |
a Dolphins expected to be captured per 100 tows according to the fitted model.
midwater trawls; and Xi3 is 0 for day and 1 for night. The fishing year is then being treated as a factor at six levels while the three X variables indicate the absence and presence of different particular conditions. The number of trawls is included as a multiplying factor in equation (3.36) because, other things being equal, the amount of bycatch is expected to be proportional to the number of trawls made. Such a multiplying factor is called an offset in the model.
The model was fitted using GenStat (Lawes Agricultural Trust 2007) to produce the estimates that are shown in Table 3.13. The estimates for the effects of different years are not easy to interpret because their estimated standard errors are quite large. Nevertheless, there are significant differences between years, as will be seen from the analysis of deviance to be considered shortly. The main thing to notice in this respect is the absence of any recorded bycatch in 1990/91.
The coefficient of X1, the area effect, is 1.822, with standard error 0.411. This estimate is very highly significantly different from zero
Table 3.13
Estimates from Fitting a Log-Linear Model to the Dolphin Bycatch Data
Parameter |
Estimate |
Standard Error |
|
|
|
α(1), year effect 1989/90 |
–7.328 |
0.590 |
α(2), year effect 1990/91 |
–17.520 |
21.380 |
α(3), year effect 1991/92 |
–5.509 |
0.537 |
α(4), year effect 1992/93 |
–7.254 |
0.612 |
α(5), year effect 1993/94 |
–8.260 |
0.636 |
α(6), year effect 1994/95 |
–7.463 |
0.551 |
Area effect (south vs. north) |
1.822 |
0.411 |
Gear effect (midwater vs. bottom) |
1.918 |
0.443 |
Time effect (night vs. day) |
2.177 |
0.451 |
|
|
|

90 Statistics for Environmental Science and Management, Second Edition
Table 3.14
Analysis of Deviance for the Log-Linear
Model Fitted to the Dolphin Bycatch Data
|
|
|
Change |
|
Effect |
Deviance |
df Deviance |
df |
|
|
|
|
|
|
No effects |
334.33a |
42 |
|
|
|
|
|
58.48b |
5 |
+ Year |
275.85a |
37 |
|
|
|
|
|
60.71b |
1 |
+ Area |
215.14a |
36 |
|
|
|
|
|
139.16b |
1 |
+ Gear type |
75.98a |
35 |
|
|
|
|
|
33.91b |
1 |
+ Time |
42.07 |
34 |
|
|
aSignificantly large at the 0.1% level, indicating that the model gives a poor fit to the data.
bSignificantly large at the 0.1% level, indicating that bycatch is very strongly related to the effect added to the model.
(z = 1.822/0.411 = 4.44, p = 0.000 compared with the standard normal distribution). The positive coefficient indicates that bycatch was higher in South Taranaki than in North Taranaki. Other things being equal, the estimated rate in the south is exp(1.822) = 6.18 times higher than the estimated rate in the north.
The estimated coefficient of X2, the gear-type effect, is 1.918 with standard error 0.443. This is very highly significantly different from zero (z = 4.33, p = 0.000). The estimated coefficient implies that the bycatch rate is exp(1.918) = 6.81 times higher for midwater trawls than it is for bottom trawls.
The estimated coefficient for X3, the time of fishing, is 2.177 with standard error 0.451. This is another highly significant result (z = 4.82, p = 0.000), implying that, other things being equal, the bycatch rate at night is exp(2.177) = 8.82 higher than it is during the day.
Table 3.14 shows the analysis-of-deviance table obtained by adding effects into the model one at a time. All effects are highly significant in terms of the reduction in the deviance that is obtained by adding them into the model, and the final model gives a good fit to the data (chisquared = 42.07 with 34 df, p = 0.161).
Finally, the last two columns of Table 3.12 show the expected counts of dolphin deaths to compare with the observed counts, and the expected number of deaths per 100 tows. The expected number of deaths per 100 tows is usually fairly low, but has the very large value of 150.4 for midwater tows, at night, in South Taranaki, in 1991/92. In summary, it seems clear that bycatch rates seem to have varied greatly with all of the factors considered in this example.

Models for Data |
91 |
3.7 Chapter Summary
•Statistical models describe observations on variables in terms of parameters of distributions and the nature of the random variation involved.
•The properties of discrete random variables are briefly described, including definitions of the mean and the variance. The hyper geometric, binomial, and Poisson distributions are described.
•The properties of continuous random variables are briefly described, including definitions of a probability density function, the mean, and the variance. The exponential, normal, and lognormal distributions are described.
•The theory of linear regression is summarized as a way of relating the values of a variable Y to the corresponding values of some other
variables X1, X2, …, Xp. The coefficient of multiple determination, R2, is defined. Tests for the significance of relationships and the use of analysis of variance with regression are described.
•The use of multiple regression is illustrated using an example where chlorophyll-a concentrations are predicted from phosphorus and nitrogen concentrations in lakes.
•The difference between factors and variables is described. The models for one-, two-, and three-factor analysis of variance are defined, together with their associated analysis-of-variance tables.
•A two-factor example on the survival time of fish is used to illustrate analysis of variance, where the two factors are the type of fish and the treatment before the fish were kept in a mixture of toxic metals.
•The structure of data for a repeated-measures analysis of variance is defined.
•The use of multiple testing methods for comparing means after analysis of variance is briefly discussed, as is the fact that these methods are not approved of by all statisticians. The use of contrasts is also discussed briefly.
•The structure of generalized linear models is defined. Tests for goodness of fit, and for the existence of effects based on the comparison of deviances with critical values of the chi-squared distribution, are described, as are tests for the significance of the coefficients of individual X variables.
•The use of a generalized linear model is illustrated by an example where the number of dolphins accidentally killed during commercial fishing operations is related to the year of fishing, the type of fishing gear used, and the time of day of fishing. A log-linear model, with the number of dolphins killed assumed to have a Poisson distribution, is found to give a good fit to the data.

92 Statistics for Environmental Science and Management, Second Edition
Exercises
Exercise 3.1
At the start of the chapter the hypergeometric, binomial, Poisson, normal, and lognormal distributions are described, with examples of where each might be used. Give one further environmental example where each distribution would likely be appropriate for data.
Exercise 3.2
The data in Table 3.15 data come from a study by Green (1973). These data show four soil variables (X1 to X4) and one vegetation variable (Y), where the variables are X1 = % soil with constant lime enrichment, X2 = % meadow soil with calcium groundwater, X3 = % soil with coral bedrock under conditions of constant lime enrichment, X4 = % alluvial and organic soils adjacent to rivers and saline organic soil at the coast, and Y = % deciduous seasonal broadleaf forest. The sample units for this study were 2.5 × 2.5-km plots in the Corozal District of Belize in Central America. Use multiple regression to relate Y to the X variables. Plot Y against each of the X variables and determine whether the relationships, if any, appear to be linear. If they are linear, then a regression of the form
Y = β0 + β1X1 + β2X2 + β3X3 + β4X4 + e
can be fitted. Otherwise, modify this equation as appropriate. For example, you might decide that the relationship with one of the variables does not seem to be linear, so you might decide to relate Y to both X and X2. Fit the equation that you have selected using any statistical package that is available to you, or in a spreadsheet. Produce residual plots to test assumptions. Remove any X variables from the equation if they do not appear to be related to Y. Clearly state your conclusions from this analysis about the extent that the amount of deciduous seasonal broadleaf forest can be predicted by the soil characteristics.
Exercise 3.3
Table 1.1 shows data from Norwegian lakes on SO4 concentrations (mg/L) as well as other variables. Carry out a two-factor analysis of variance on the SO4 data and report your conclusions concerning (a) whether there was any change from year to year and (b) whether the assumptions of the analysis of variance model are reasonable. For (b), residual plots should be examined. You can, for example, plot the residuals against latitudes and longitudes. An important question concerns whether the residuals from the fitted model show correlation in time or space. If they do, then a simple two-factor analysis of variance is not appropriate for the data. Note that this is a two-factor analysis of variance with some missing data for some lakes in some years. This can be handled by some statistical packages under what may be referred to as a general linear model
Models for Data |
93 |
Table 3.15
Soil and Vegetation Data for 2.5 × 2.5-km Plots in the Corozal District of Belize in Central America
Case |
X1 |
X2 |
X3 |
X4 |
Y |
Case |
X1 |
X2 |
X3 |
X4 |
Y |
|
|
|
|
|
|
|
|
|
|
|
|
1 |
40 |
30 |
0 |
30 |
0 |
77 |
30 |
0 |
0 |
50 |
0 |
2 |
20 |
0 |
0 |
10 |
10 |
78 |
50 |
10 |
0 |
30 |
5 |
3 |
5 |
0 |
0 |
50 |
20 |
79 |
100 |
0 |
0 |
0 |
60 |
4 |
30 |
0 |
0 |
30 |
0 |
80 |
50 |
0 |
0 |
50 |
20 |
5 |
40 |
20 |
0 |
20 |
0 |
81 |
10 |
0 |
0 |
90 |
0 |
6 |
60 |
0 |
0 |
5 |
0 |
82 |
30 |
30 |
0 |
20 |
0 |
7 |
90 |
0 |
0 |
10 |
0 |
83 |
20 |
20 |
0 |
20 |
0 |
8 |
100 |
0 |
0 |
0 |
20 |
84 |
90 |
0 |
0 |
0 |
50 |
9 |
0 |
0 |
0 |
10 |
40 |
85 |
30 |
0 |
0 |
0 |
30 |
10 |
15 |
0 |
0 |
20 |
25 |
86 |
20 |
30 |
0 |
50 |
20 |
11 |
20 |
0 |
0 |
10 |
5 |
87 |
50 |
30 |
0 |
10 |
50 |
12 |
0 |
0 |
0 |
50 |
5 |
88 |
80 |
0 |
0 |
0 |
70 |
13 |
10 |
0 |
0 |
30 |
30 |
89 |
80 |
0 |
0 |
0 |
50 |
14 |
40 |
0 |
0 |
20 |
50 |
90 |
60 |
10 |
0 |
25 |
80 |
15 |
10 |
0 |
0 |
40 |
80 |
91 |
50 |
0 |
0 |
0 |
75 |
16 |
60 |
0 |
0 |
0 |
100 |
92 |
70 |
0 |
0 |
0 |
75 |
17 |
45 |
0 |
0 |
0 |
5 |
93 |
100 |
0 |
0 |
0 |
85 |
18 |
100 |
0 |
0 |
0 |
100 |
94 |
60 |
30 |
0 |
0 |
40 |
19 |
20 |
0 |
0 |
0 |
20 |
95 |
80 |
20 |
0 |
0 |
50 |
20 |
0 |
0 |
0 |
60 |
0 |
96 |
100 |
0 |
0 |
0 |
100 |
21 |
0 |
0 |
0 |
80 |
0 |
97 |
100 |
0 |
0 |
0 |
95 |
22 |
0 |
0 |
0 |
50 |
0 |
98 |
0 |
0 |
0 |
60 |
0 |
23 |
30 |
10 |
0 |
60 |
0 |
99 |
30 |
20 |
0 |
30 |
0 |
24 |
0 |
0 |
0 |
50 |
0 |
100 |
15 |
0 |
0 |
35 |
20 |
25 |
50 |
20 |
0 |
30 |
0 |
101 |
40 |
0 |
0 |
45 |
70 |
26 |
5 |
15 |
0 |
80 |
0 |
102 |
30 |
0 |
0 |
45 |
20 |
27 |
60 |
40 |
0 |
0 |
10 |
103 |
60 |
10 |
0 |
30 |
10 |
28 |
60 |
40 |
0 |
0 |
50 |
104 |
40 |
20 |
0 |
40 |
0 |
29 |
94 |
5 |
0 |
0 |
90 |
105 |
100 |
0 |
0 |
0 |
70 |
30 |
80 |
0 |
0 |
20 |
0 |
106 |
100 |
0 |
0 |
0 |
40 |
31 |
50 |
50 |
0 |
0 |
25 |
107 |
80 |
10 |
0 |
10 |
40 |
32 |
10 |
40 |
50 |
0 |
75 |
108 |
90 |
0 |
0 |
10 |
10 |
33 |
12 |
12 |
75 |
0 |
10 |
109 |
100 |
0 |
0 |
0 |
20 |
34 |
50 |
50 |
0 |
0 |
15 |
110 |
30 |
50 |
0 |
20 |
10 |
35 |
50 |
40 |
10 |
0 |
80 |
111 |
60 |
40 |
0 |
0 |
50 |
36 |
0 |
0 |
100 |
0 |
100 |
112 |
100 |
0 |
0 |
0 |
80 |
37 |
0 |
0 |
100 |
0 |
100 |
113 |
60 |
0 |
0 |
40 |
60 |
38 |
70 |
30 |
0 |
0 |
50 |
114 |
50 |
50 |
0 |
0 |
0 |
39 |
40 |
40 |
20 |
0 |
50 |
115 |
60 |
30 |
0 |
10 |
25 |
40 |
0 |
0 |
100 |
0 |
100 |
116 |
40 |
0 |
0 |
60 |
30 |
94 Statistics for Environmental Science and Management, Second Edition
Table 3.15 (continued)
Soil and Vegetation Data for 2.5 × 2.5-km Plots in the Corozal District of Belize in Central America
Case |
X1 |
X2 |
X3 |
X4 |
Y |
Case |
X1 |
X2 |
X3 |
X4 |
Y |
|
|
|
|
|
|
|
|
|
|
|
|
41 |
25 |
25 |
50 |
0 |
100 |
117 |
30 |
0 |
0 |
70 |
0 |
42 |
40 |
40 |
0 |
20 |
80 |
118 |
50 |
20 |
0 |
30 |
0 |
43 |
90 |
0 |
0 |
10 |
100 |
119 |
50 |
50 |
0 |
0 |
25 |
44 |
100 |
0 |
0 |
0 |
100 |
120 |
90 |
10 |
0 |
0 |
50 |
45 |
100 |
0 |
0 |
0 |
90 |
121 |
100 |
0 |
0 |
0 |
60 |
46 |
10 |
0 |
0 |
90 |
100 |
122 |
50 |
0 |
0 |
50 |
70 |
47 |
80 |
0 |
0 |
20 |
100 |
123 |
10 |
10 |
0 |
80 |
0 |
48 |
60 |
0 |
0 |
30 |
80 |
124 |
50 |
50 |
0 |
0 |
30 |
49 |
40 |
0 |
0 |
0 |
0 |
125 |
75 |
0 |
0 |
25 |
80 |
50 |
50 |
0 |
0 |
50 |
100 |
126 |
40 |
0 |
0 |
60 |
0 |
51 |
50 |
0 |
0 |
0 |
40 |
127 |
90 |
10 |
0 |
10 |
75 |
52 |
30 |
30 |
0 |
20 |
30 |
128 |
45 |
45 |
0 |
55 |
30 |
53 |
20 |
20 |
0 |
40 |
0 |
129 |
20 |
35 |
0 |
80 |
10 |
54 |
20 |
80 |
0 |
0 |
0 |
130 |
80 |
0 |
0 |
20 |
70 |
55 |
0 |
10 |
0 |
60 |
0 |
131 |
100 |
0 |
0 |
0 |
90 |
56 |
0 |
50 |
0 |
30 |
0 |
132 |
75 |
0 |
0 |
25 |
50 |
57 |
50 |
50 |
0 |
0 |
30 |
133 |
60 |
5 |
0 |
40 |
50 |
58 |
0 |
0 |
0 |
60 |
0 |
134 |
40 |
0 |
0 |
60 |
60 |
59 |
20 |
20 |
0 |
60 |
0 |
135 |
60 |
0 |
0 |
40 |
70 |
60 |
90 |
10 |
0 |
0 |
70 |
136 |
90 |
10 |
0 |
10 |
75 |
61 |
100 |
0 |
0 |
0 |
100 |
137 |
50 |
0 |
5 |
0 |
30 |
62 |
15 |
15 |
0 |
30 |
0 |
138 |
70 |
0 |
30 |
0 |
70 |
63 |
100 |
0 |
0 |
0 |
25 |
139 |
60 |
0 |
40 |
0 |
100 |
64 |
95 |
0 |
0 |
5 |
90 |
140 |
50 |
0 |
0 |
0 |
50 |
65 |
95 |
0 |
0 |
5 |
90 |
141 |
30 |
0 |
50 |
0 |
60 |
66 |
60 |
40 |
0 |
0 |
50 |
142 |
5 |
0 |
95 |
0 |
80 |
67 |
30 |
60 |
10 |
10 |
50 |
143 |
10 |
0 |
90 |
0 |
70 |
68 |
50 |
0 |
50 |
50 |
100 |
144 |
50 |
0 |
0 |
0 |
15 |
69 |
60 |
30 |
0 |
10 |
60 |
145 |
20 |
0 |
80 |
0 |
50 |
70 |
90 |
8 |
0 |
2 |
80 |
146 |
0 |
0 |
100 |
0 |
90 |
71 |
30 |
30 |
30 |
40 |
60 |
147 |
0 |
0 |
100 |
0 |
75 |
72 |
33 |
33 |
33 |
33 |
75 |
148 |
90 |
0 |
10 |
0 |
60 |
73 |
20 |
10 |
0 |
40 |
0 |
149 |
0 |
0 |
100 |
0 |
80 |
74 |
50 |
0 |
0 |
50 |
40 |
150 |
0 |
0 |
100 |
0 |
60 |
75 |
75 |
12 |
0 |
12 |
50 |
151 |
0 |
40 |
60 |
40 |
50 |
76 |
75 |
0 |
0 |
25 |
40 |
|
|
|
|
|
|
|
|
|
|
|
|
|
|
|
|
|
|
Source: |
Green (1973). |
|
|
|
|
|
|
|
|
|
Models for Data |
95 |
option, or an option for unbalanced data. However, many packages assume that observations on all factor combinations are present, and these will not do the required analysis. If you do not have software available that will handle the missing values, then just do the analysis for those lakes without missing values.
Exercise 3.4
Table 3.16 shows the results of a fisheries observer program in New Zealand for the surface long-line fisheries. For different Fisheries Management Areas (FMAs), target fisheries species, and years, the table provides the number of fishing days observed on surface long-line vessels and the total number of marine mammals caught in the line. Carry out an analysis along the lines of Example 3.3, using a log-linear model. The analysis can be carried out using any computer package that has a log-linear model facility. Note the following points: (a) an offset is needed in the model to allow for the fact that, other things being equal, the bycatch is expected to be proportional to the observation time; (b) the FMAs, target species, and years should be treated as levels of factors; and (c) given the limited data, only try fitting main effects of these factors (i.e., no interactions between the factors). The offset should probably be entered as the natural logarithm of days of fishing. After completing your analysis, summarize your conclusions about the effects of the three factors on the probability of bycatch occurring.