
Учебники / Computer-Aided Otorhinolaryngology-Head and Neck Surgery Citardi 2002
.pdfComputer-Aided Otologic and Neurotologic Surgery |
309 |
17.5CONCLUSIONS
Our experience with CAS in the temporal bone and its surroundings has been encouraging. Modification of otologic instruments and simplified attachment of the reference arc to the head with a single screw makes the technology more adapted to the demands of otologic and neurotologic microsurgery. With attachment of the reference arc directly to the skull, calibration and registration remains stable throughout long cases.
The chief advantage of CAS in temporal bone surgery is the rapid and accurate orientation of dissection. Ultimately, time in the operating room is reduced and complications can be avoided. CAS is not, however, a substitute for experience, knowledge of anatomy, or surgical judgment.
REFERENCES
1.Sargent EW, Bucholz RD. Middle cranial fossa surgery with image-guided instrumentation. Otolaryngol Head Neck Surg 1997; 117:131–134.
2.House WF. Surgical exposure of the internal auditory canal and its contents through the middle cranial fossa. Laryngoscope 1961; 71:1363–85.
3.House WF. Transtemporal bone microsurgical removal of acoustic neuroma. Arch Otolaryngol 1964; 80:597–756.
4.Glasscock M. Middle fossa approach to the temporal bone. Arch Otolaryngol 1969; 90:41–57.
5.Fisch U. Transtemporal surgery of the internal auditory canal. Adv. Otorhinolaryngol 1970; 17:202–239.
6.Kartush JM, Kemink JL, Graham MD. The arcuate eminence: topographic orientation in middle cranial fossa surgery. Ann Otol Rhinol Laryngol 1985; 94:25–28.
7.Pialoux P, Freyss G. Narch P, deSaint-Macary M, Davaine F. Contributions a l’anatomie stereotaxique du conduit auditif interne et de la premiere portion du facial. Ann Otolaryngol Chir Cerviofac 1972; 89:141–142.
8.Brackmann DE, Giddings NA. Drainage procedures for petrous apex lesions. In: Brackmann DE, C Shelton, Arriaga MA, eds. Otologic Surgery. Philadelphia: W.B. Saunders Company, 1994:566–578.
9.Fong BP, Brackmann DE, Telischi FF. The long-term follow-up of drainage procedures for petrous apex cholesterol granulomas. Arch Otolaryngol Head Neck Surg 1995; 121:426–430.

18
Computer-Aided Tumor Modeling
and Visualization
Gregory J. Wiet, M.D.
The Ohio State University College of Medicine and Public Health and Columbus Children’s Hospital, Columbus, Ohio
Don Stredney
Ohio Supercomputer Center, Columbus, Ohio
Petra Schmalbrock, Ph.D.
The Ohio State University College of Medicine and Public Health, Columbus, Ohio
18.1INTRODUCTION
Our goal in this chapter is to provide an update on some of the new and exciting technologies for head and neck tumor visualization. Before this discussion one may ask, ‘‘Why is tumor visualization important?’’ From a surgical perspective, we believe that visualization provides two key elements to understanding the tumor and hence its management: tumor margin and tumor burden. These two elements dictate whether surgical management is possible and, if possible, the approach that should be undertaken. Margin is the local anatomy that is directly affected by the tumor and that may lie within the surgical trajectory. Burden describes the amount of tissue and structures that are directly affected by the abnormal tissue and that may end up being sacrificed to effect a cure. With the
311
312 |
Wiet et al. |
advent of more minimally invasive techniques, complete and accurate visualization becomes paramount. Our goal is to develop an interactive visualization environment that provides a better understanding of tumor margin and burden. Over the past 10 years, our focus has been on developing interactive tools for intuitive access to tumor information for head and neck surgeons in the Department of Otolaryngology at The Ohio State University [1–7]. Through innovative software and current and future hardware platforms, we are achieving a more complete and realistic visualization of head and neck tumors. In essence, the result will be an environment much like a cadaver lab, but with the ability to see deep structures without removing covering structures and to manipulate structures without destroying critical relationships. The work will occur in real time and with patient-specific data. We have now extended this technology from the desktop to the Internet for remote collaboration. A complete QuickTime movie of the system can be found at www.osc.edu/Biomed under Past Projects, Interactive Medical Data on Demand, A High-Performance Image-Based Warehouse Across Heterogeneous Environments.
We present our methods for data acquisition and processing, as well as interactive techniques to facilitate tumor visualization for diagnosis, preoperative assessment, and treatment planning, including extirpation. We further discuss implications for synchronous and asynchronous remote collaborations and extensibility to presurgical planning. We conclude with discussions regarding the value of interactive visualizations to exploring and managing treatment options.
18.2DATA ACQUISITION
As with any computer application, the accuracy of the visualization depends directly upon the quality of data in the system. Current data acquisition techniques do not provide enough resolution that is comparable to what we see in the real world. Despite this limitation, our ability to acquire large amounts of highresolution patient image data is rapidly increasing. Data sets comprising images 512 512 N in resolution are common [8]. Recent technology has introduced the next generation of imaging technology capable of delivering resolutions of 1024 1024 N with unprecedented clarity. Improvements in imaging have increased the use of temporal data sets that demonstrate cognitive function, cardiac function, joint movements, and aspects of development. Although very promising, all these data present an additional problem: storage. Research data and patient records will soon exceed gigabytes in storage needs. At The Ohio State University Medical Center, an estimated 7 terabytes of image data are obtained annually. Currently, shelf life of online image data is limited to 17 days.
Larger and larger data sets not only present storage problems, but they are essentially useless unless they can be rendered and visualized in a timely fashion. Real-time visualizations allow the user to derive structure from motion and gain

Computer-Aided Tumor Modeling and Visualization |
313 |
insight into the configuration of the tumor. Interactive visualizations provide a suite of tools to allow for more intuitive exploration of the information. Furthermore, we see interactive tumor visualizations as ways to manage the visualization of multimodal studies (computed tomography, magnetic resonance, nuclear medicine), facilitate minimally invasive management of tumors, and serve as a communication tool between health care providers. If these ever-increasing data sets are to be visualized in real time, heavy demands will be placed upon both the software and hardware used to process these data. Current technologies can provide interactive visualizations but not without such processes as decimation (removal of data to create a manageable size) or supersampling (introduction of false data to ‘‘fill in’’ defects in the original data). Care must be taken not to remove key data or introduce false data that might alter a diagnosis or treatment plan.
We must address several additional issues in regard to the concepts of tumor margin and burden. Some tumors present defined ‘‘capsules’’ that assist in margin definition, whereas others present poor margin definition. In infiltrative type tumors, margins must be arbitrarily determined using transfer functions that ultimately include healthy tissue that will be sacrificed during extirpation (Figure 18.1). More precise establishment of tumor margin is facilitated by using paramagnetic contrast agents (see below). Comparative studies (with and without contrast) can clarify ambiguous areas. These methods exploit certain characteristics of the tumor such as vascularity, fat content, water content, and density. New methodology will aid in defining tumor margin [9]. Radiolabeled tumor antibodies to epithelial surface antigens and radiolabeled receptor ligands are two examples. The ability to image biochemical processes, such as positron-emission tomography (PET) scanning, has now been used to characterize tumor lesions, differentiate recurrent disease from treatment effects, stage tumors, evaluate the extent of disease, and monitor therapy [10,11]. We use both computed tomography (CT) and magnetic resonance (MR) in our data acquisition, with an increas-
FIGURE 18.1 Image of infiltrative rat lymphoma model.
314 Wiet et al.
ing dependence on high-resolution MR because of its higher spatial resolution of soft tissue. Because one of the coauthors (PS) is continually developing new scanning protocols to increase spatial resolution, we will discuss these techniques and show several images of these new modes of data acquisition.
The inner ear and cerebralpontine angle are useful regions to image and demonstrate the capability of these techniques. Our institution acquires highresolution MR axial images with a 1.5 T MR system (General Electric, Milwaukee, WI) using the standard quadrature head coil. A segmented, interleaved, mo- tion-compensated acquisition in steady state (SIMCAST) sequence provides very high resolution of the region of interest. The SIMCAST sequence is a prototype three-dimensional (3D) gradient echo pulse sequence (8). To avoid potential problems with gross patient motion, we acquire four data sets with different RF phases in an interleaved fashion. For our clinical studies, SIMCAST images are acquired with TR/TE/flip angle of 17 ms/3.5 ms/40 degrees, 22 16 cm FOV, 512 256 matrix size, and 32 slices of 1.2 mm thickness or, more recently, with 0.8 mm slice thickness (interpolated to 0.4 mm). In all cases the SIMCAST images are acquired before contrast injection. Contrast-enhanced T1-weighted images are acquired after the injection of 10cc Gd-DTPA contrast agent with a 3D spoiled gradient echo sequence [12], using TR/TE/flip angle of 30 ms/4.2 ms/ 30 degrees, 1 excitation, 20 20 cm FOV, 512 288 matrix size, and 64 slices of 1.5 mm thickness. Both sequences have similar scan times of 7 minutes.
All lesions are clearly depicted with both sequences [13], and tumor volumes can be accurately measured for remarkably small tumors with volumes as low as 0.05 cc (Figure 18.2). Cochlea, vestibule, and semicircular canals are well depicted with SIMCAST, whereas these structures are seen poorly on the T1- weighted images. The complete course of the 7th and 8th nerves in the CPA and IAC is seen with SIMCAST in normal ears (Figures 18.2 and 18.3).
In patients with small tumors, portions of the vestibulocochlear or facial nerve branches can be seen on axial and reformatted SIMCAST images (Figures 18.2 and 18.4). In some cases, it is possible to observe the nerves displaced by the tumor (Figure 18.4), whereas nerve depiction is usually poor on T1-weighted images. Lesions were always clearly differentiated from the surrounding high signal CSF, but for some large lesions extending toward the brain stem the tumor/ brain boundary is not distinguishable merely by the tissue signal contrast. However, in most cases the tumor margin may be determined from the shapes of the anatomical structures or intervening vessels. Tumor/brain distinction is better on contrasted, enhanced T1-weighted images; however, because of the low CSF signal on T1-weighted images, fluid/bone boundaries are poorly defined, and thus the extent of tumor from the fluid spaces into the bony regions may not be as clearly distinguishable.
In our studies, IAC tumor signal was heterogeneous with both the T1- weighted and SIMCAST sequences in a significant numer of cases. This fact
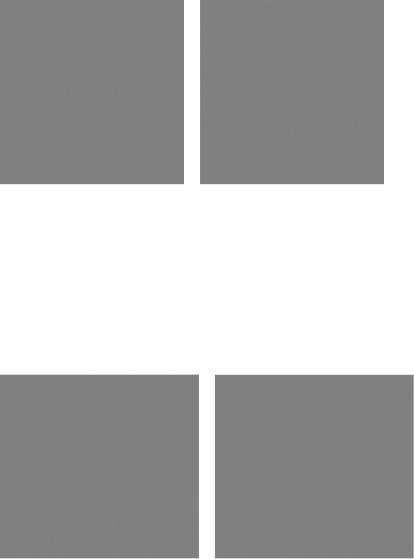
Computer-Aided Tumor Modeling and Visualization |
315 |
(A) |
(B) |
FIGURE 18.2 Incidental finding of a small 0.05 cc IAC mass in an 87-year-old male with vertigo and sudden hearing loss. The mass is clearly identified with SIMCAST (A, open arrow) and with the contrast enhanced study (B, open arrow). Inner ear anatomical structures including the cochlea (c), vestibule (v), and semicircular canal (sc) are clearly seen on the SIMCAST image. These structures are not or only partially seen on the T1 images. Small vascular structures (arrows) appear dark on SIMCAST and bright on T1 images. The SIMCAST images also depict the cochlear (cn) and inferior vestibular nerves (ivn), which are isointense with the tumor. Again only a portion of the cochlear nerve is seen on the T1 images.
(A) |
(B) |
FIGURE 18.3 High-resolution MRI (A) and CT (B) images of a normal subject. Both MRI and CT show the vestibule (v), cochlear (c), and lateral and posterior semicircular canals (lsc, psc), i.e., CT shows the bony outlines and the MRI shows the fluid filled spaces. The CT image also differentiates bone (bright) and air space (dark) in the middle ear and mastoid. However, no further differentiation of fluid and neural structures is achieved with CT. Only MRI can delineate nerves in the internal auditory canal (iac), vascular structures in the cerebellopontine angle (cpa) and the fluid-filled fourth ventricle.
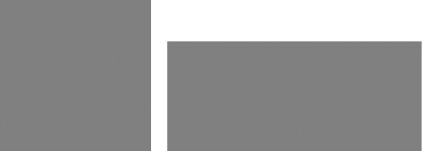
316 |
Wiet et al. |
(A) |
(B) |
FIGURE 18.4 Original axial (A) and sagittal reformatted (B) SIMCAST images of a 10-year-old patient with a small tumor (0.07 cc, open arrow) emanating from the superior vestibular nerve (as per surgery report). On the axial image, the cochlear nerve can be followed from the brain stem into the internal auditory canal (arrows), where it is pushed anteriorly by the tumor. The inferior branch of the vestibular nerve is seen continuous with the tumor. On the sagittal reformatted image, the facial (fn), cochlear (cn), and superior and inferior nerves (svn, ivn) are clearly distinguishable. The superior vestibular nerve is enlarged and surrounded by low signal tumor. In this patient, hearing preservation was achieved in surgery.
indicates that some of the tumors were probably necrotic, hemorrhagic, or partially cystic. On SIMCAST images cystic tumor regions had higher signal intensity than the brain, helping to distinguish the brain/tumor margin; however, these tumor regions were more difficult to separate from the CSF. Tumors that were partially cystic or necrotic did not enhance homogeneously. This factor led to difficulties in identifying margins between tumor and CSF on the contrastenhanced images with confidence (Figure 18.5), and such tumor portions were better defined by the SIMCAST studies.
Until such time as the SIMCAST technique can be reliably applied to other areas, we acquire an isotropic volume, employing a short 3DSPGR sequence; that is, we use a T1-weighted three-dimensional spoiled gradient echo using a TR/TE/FlipAngle of 33 ms/5 ms/45 degrees. The field of view (FOV) of 25 cm provides a voxel resolution of 256 128 128 and a slice thickness of 2 mm. Fourier interpolation of the data generates isotropic data sets for image processing. This protocol requires 9 minutes of scan time and also allows acquisitions with and without Gd-DTPA, the paramagnetic contrast agent commonly used in MR imaging for imaging tumors.
After the surgeons have initially read MR images to determine tumor location, CT studies are performed when appropriate. CT scans are performed on late-generation scanners, encompassing the specific tumor area to limit radiation
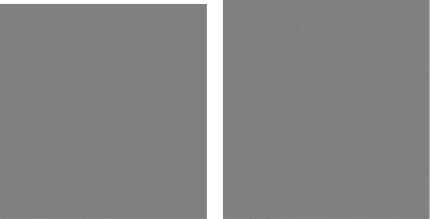
Computer-Aided Tumor Modeling and Visualization |
317 |
(A) |
(B) |
FIGURE 18.5 SIMACAST (A) and contrast enhanced T1-weighted (B) images of a heterogeneous 0.35 cc internal auditory canal mass in a 75-year-old female. This tumor is well delineated on the SIMCAST image (arrows). However, it does not enhance homogeneously, and, therefore, the tumor margin is not clearly seen on the T1 image and the tumor size is underestimated.
exposure to the patient with a 2-second scan time at 200 mA and 120 kilovolt peak. The matrix size is 512 512, and, whenever possible, gantry tilt is restricted to zero for axial scans. Continuous 1.5 mm sections are obtained using a 1.5 mm slice thickness and 1.5 mm table increments [14].
18.3DATA PROCESSING
18.3.1 Segmentation
Once transferred to OSC, image data are segmented using various transfer functions, including threshold and slice densities. We have employed neural network– based software (LEGION) [15] to automatically segment structures within the skullbase (Figure 18.6). This software allows one to segment structures within the volume rather than image by image and provides excellent first-pass results. Limited ‘‘clean-up’’ by the user is required in areas where the normal anatomy has been surgically or pathologically altered. The local software, Editmask, provides a robust GUI interface for loading multiple color sections into memory. The user is free to delineate regions of interest (ROIs) in image space, using either a polygon or paint mode. Color image masks are produced with ROIs differentiated with various colors (Figure 18.7). These masks ‘‘tag’’ volume ele-
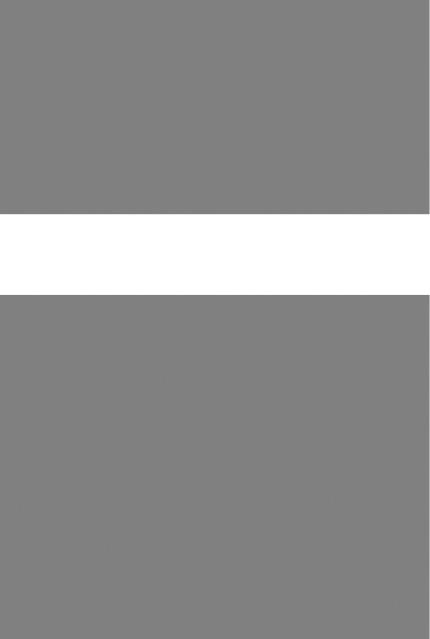
318 |
Wiet et al. |
FIGURE 18.6 Left, original axial CT. Right, structural segmentation after first pass with LEGION.
FIGURE 18.7 Graphical user interface for Editmask segmentation application.
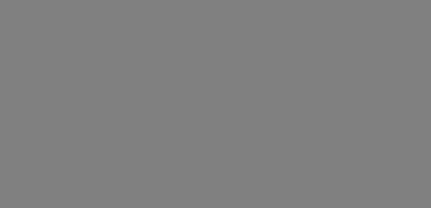
Computer-Aided Tumor Modeling and Visualization |
319 |
ments with structural or functional information, i.e., information that may be useful but is independent of structure.
18.3.2 Multimodal Data Merging
The use of multimodal images allows checks and validations that are critical in determining the extent of the tumor. We have developed techniques to merge these data acquired from different modalities by allowing direct visual manipulation of one volumetric data set with another. One data set is static and serves as a background, allowing the user to position, scale, and rotate another data set. We have employed this technique to merge the data sets acquired at various resolutions (Figure 18.8). This technique obviates the need for fiduciaries.
The following images were acquired from a patient with a sphenoid tumor (Figure 18.9). Binary masks were made for the skull, tumor, vessels, brain, and surrounding structures (Figure 18.10). An image of these components combined can be seen in Figure 18.11.
18.3.3 Rendering: Surface Versus Volume
Two basic approaches to rendering structures are isosurfaces, or a polygonal representation that approximates the surface, or volumetric, which also approximates the structure, but by discrete volume elements called voxels. Volume repre-
FIGURE 18.8 Image of image file merging environment. The CT volume image file is static, and the MRI image file is being merged to create one multimodal volume image file.