
Encyclopedia of SociologyVol._3
.pdf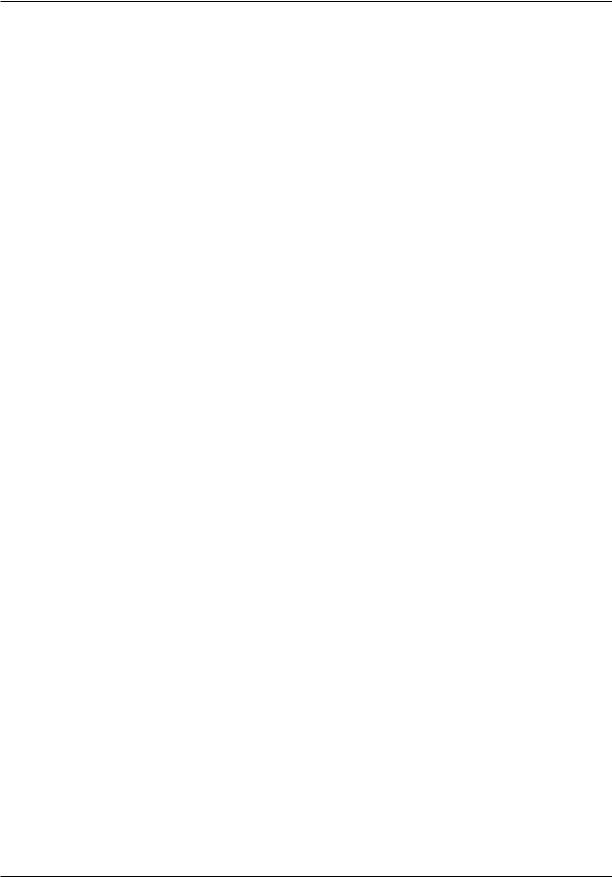
MEASUREMENT
having an underlying theoretical correlation coefficient for each independent variable to the criterion variable of .8, have been used to create a score. The variables defined by these cutting points correspond to a notion of variables with response categories, say, such as ‘‘agree,’’ ‘‘probably agree,’’ ‘‘probably disagree,’’ and ‘‘disagree,’’ and as it happens the responses theoretically are well distributed, roughly 16 percent, 34 percent, 34 percent, and 16 percent.
B.Three groups or categories are created by using two dividing points in the theoretical distribution, -1 and +1, corresponding to a notion of variables with response categories, say, such as ‘‘agree,’’ ‘‘don’t know or neutral,’’ and ‘‘disagree,’’ and these are again theoretically well distributed, but in this case the center category is large (about 68 percent ofresponses). The scores for these samples are identified as SumB.8 for the case of the underlying theoretical distribution of the independent variables having a correlation coefficient with the criterion variable of .8.
C.Two groups or categories are created by using one dividing point at the mean of the theoretical distribution, and this corresponds to variables with response categories such as ‘‘agree’’ and ‘‘disagree,’’ or ‘‘yes’’ and ‘‘no.’’ Division of a variable
at the midpoint of the distribution is usually considered statistically to be the most efficient for dichotomous response categories.
DL. Two groups or categories may be created that are not symmetrical, unlike the case of C above. Here we create two sets of samples, one identified by Sum DL with the cutting point at -1 standard deviation, or the left side of the distribution as it is usually represented. Presumably, when variables are not well distributed, more information is lost in the data collection if the skew is due to the way the question is formulated.
DR. Two groups or categories are created with the skew on the right side of
the distribution at +1 standard deviation. SumDR scores are created in parallel to the SumDL scores.
Finally, the rows of SumX are those where scores are computed and no conversion of the variables has been carried out, so the independent variables are drawn from the theoretically formulated unit normal distributions at the level of correlation coefficient between the independent variables and the criterion variable.
In summary, for each sample the predictor score is an additive score based on four variables, each related to the criterion variable in a theoretical distribution at a given level, with the examples in the exercise the levels chosen as product moment correlation coefficients of .8, .6, .4, and .2.
The rationale for the choice of four variables in each score is drawn arbitrarily from the theoretical distribution of reliability coefficients, which, for variables of equal reliability, is a curve of diminishing return with each increment of the number of variables. At four variables a score is usually considered to be at an efficient point, balancing such things as time and effort needed to collect data, independence of variable definitions, and the amount of improvement of reliability expected. Using more variables in the ‘‘real’’ world usually involves using less ‘‘good’’ items progressively from the point of view of content, as well as the diminishing efficiency even if they were equally as good as the first four.
With regard to the criterion variable, the dependent variable to be predicted, we have generated three as follows: First, the variable is drawn from a theoretical unit normal distribution without modification, and this involves correlation coefficients reported in column XO. Second, the dependent variable is drawn from the theoretical distribution dichotomized at the mean of 0, creating a symmetric dichotomous variable (two categories), and this involves correlation coefficients reported in column XOA. Third, the variable is drawn from the theoretical distribution dichotomized at -1 standard deviation, creating an asymmetrical dichotomous variable and this involves correlation coefficients reported in column XOB.
As noted above, we are dealing with generated data from theoretical distributions, so all that is done is subject to sampling error, which permits in a limited way for variation of results to be shown. This is useful in illustrating the variation that occurs simply by random processes in the study of variables. For the exercise there were 648 samples
1798
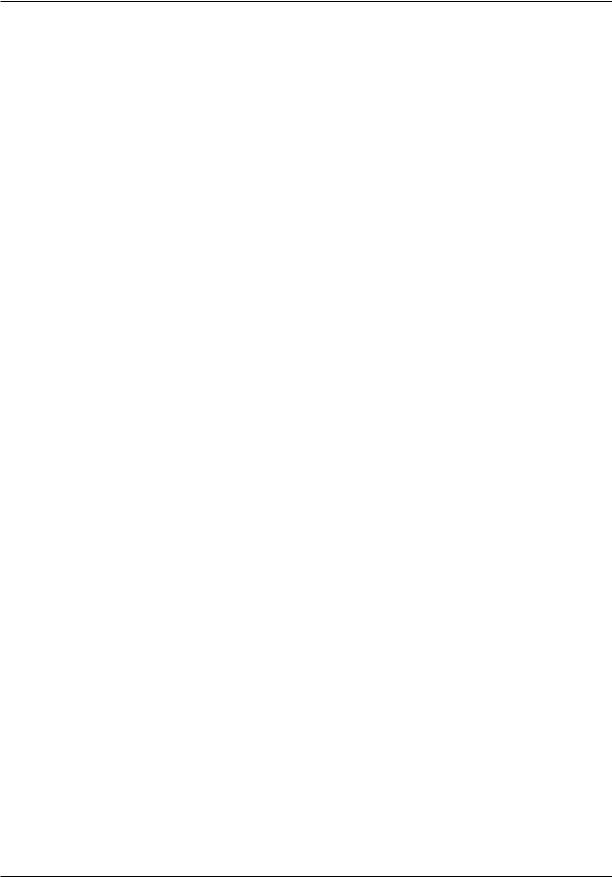
MEASUREMENT
of 150 cases each. The choice of 150 cases was selected for several reasons, convenience in the data set generated being one, but also because 150 cases was, as a rule of thumb, a reasonable number of cases proposed for doing research at an earlier time in the discipline when a Guttman scale was anticipated, and too much ‘‘shrinkage’’ in the subsequent use of the Guttman scale was to be avoided. Shrinkage referred to the experience of finding out that the scale in a subsequent use did not have the same characteristics as in the first (generating) use. The samples are grouped in the six procedures, as noted above, of the full normal data and of five created sets of categories, each with nine repetitions (i.e., samples) carried out to permit examination of distribution of results for each theoretical level of correlation coefficient and for each of the three criterion variable definitions.
A sense of the stability of the relationships involved is provided by using many samples, and the values that are in columns XO, XOA, and XOB are the median product moment correlation coefficients between a Sum score and a criterion variable. In the table there are two other sets of columns. XOR, XOAR, and XOBR are the actual range of values for the product moment correlation coefficients between a Sum score and the criterion variable for nine independently drawn samples of 150 cases each. The additional (last three columns) at the right of the table are the squared values of XO, XOA, and XOB, and these values represent the amount of variance that is accounted for by the correlation coefficient.
The table is somewhat complex because it includes a great deal of information. Here we will only review a number of relatively obvious points, but with emphasis. First, note the relationship between SumX.8 and XO, which is .94. Recall that the theoretical correlation coefficient between each independent predictor variable and the criterion variable was defined as .8 in the exercise. Thus, we illustrate rather dramatically the importance of building scores rather than depending on a single predictor variable. The actual improvement in prediction is from a theoretical value of 64 percent of the variance being accounted for by a single predictor variable to the median value of 88 percent of the variance in the median sample of the exercise, an extremely impressive improvement! Examining the correlation coefficient between SumX.6, SumX.4, and SumX.2, it is seen that the
advantage of using scores rather than single items is actually even more dramatic when the relationship between the criterion and the predictor variables is weaker.
Now, examine the relationships between all the Sum variables and XO. It is noted that having the SumA.8 as a well distributed categorical variable lowers the correlation to XO somewhat (.92) compared to the full normal SumX.8 (.94), and, indeed, with SumB.8 and SumC.8 the correlation coefficients are still lower. This illustrates that the more information that is available in the categorical data, the more variance can be predicted for a criterion variable. Note further, that for SumDL.8 and SumDR.8, which are based on dichotomous but skewed variables, the correlation coefficients are still lower. Note that the results for the weaker variables SumX.6 to SumDR.6, for SumX.4 to SumDR.4, and for SumX.2 to SumDR.2 are in general roughly parallel to the results noted, but some irregularity is noted when the relationship between the criterion and the predictor variables is weaker.
The results we have been examining are the median values of nine samples in each case. It is appropriate to look at column XOR and to note that there is quite a bit of variation in the correlation coefficients based on the nine samples of size 150 cases each.
One additional finding needs to be pointed out and emphasized. Compare the correlation coefficients of SumDT.8 and XOB, and of SumDR.8 and XOB. In the former case, the criterion variable and predictor variables are both asymmetric but matched (at the same cutting point), and the median correlation coefficient value is .72. In the latter case they are asymmetric but unmatched, and the median correlation coefficient is .26. This indicates the need to know how cutting points (difficulty levels) of variables can affect the values of correlation coefficients that are encountered.
The reader is urged to examine this table in detail, and it may be useful to keep the table as a guideline for interpreting results. How? If the researcher finds as a result a correlation coefficient of a given size, where does it fit on this table, taking into consideration the characteristics of the criterion variable and the scores based on the predictor variables? Doing this type of examination should help the researcher understand the
1799
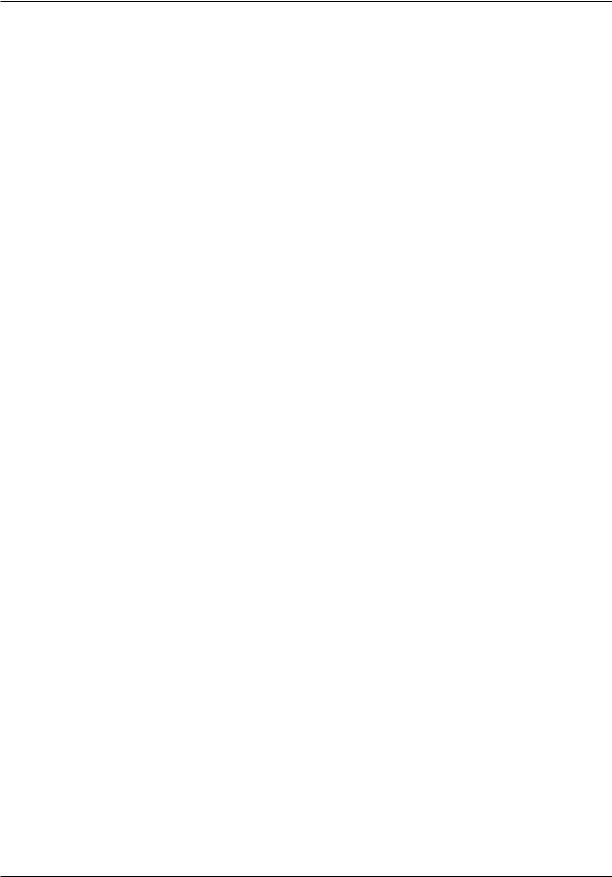
MEASUREMENT
meaning of a finding. However, this is but one approach to the issues of measurement. The articles in this encyclopedia that are vital for researchers to consider are those on Reliability, Validity, and Quasi-Experimental Research Design. Additionally, measurement issues are discussed in a variety of types of texts, ranging from specialized texts to books on research methods to general statistics books, such as the following: Agresti and Finaly 1997; Babbie 1995; De Vellis 1991; Knoke and Bohrnstedt 1994; Lewis-Beck 1994; Neuman, 1997; and Traub 1994.
OTHER MEASURES
The consideration of measurement thus far has concentrated on interval measurement, with some emphasis on how it can be degraded. There are many other issues that are appropriately considered, including the fact that the concepts advanced by Stevens do not include all types of measures. As the discussion proceeds to some of these, attention should also be given to the notion of nominal scales. Nominal scales can be constructed in many ways, and only a few will be noted here. By way of example, it is possible to create a classification of something being present for objects that is then given a label A. Sometimes a second category is not defined, and then the second category is the default, the thing not being present. Or two categories can be defined, such as male and female. Note that the latter example can also be defined as male and not male, or as female and not female. More complex classifications are illustrated by geographical regions, such as north, west, east, and south, which are arbitrary and follow the pattern of compass directions. Such classifications, to be more meaningful, are quickly refined to reflect more homogeneity in the categories, and sets of categories develop such as Northeast, Middle Atlantic, South, North Central, Southwest, Mountain, Northwest, and Pacific, presumably with the intention of being inclusive (exhaustive) of the total area. These are complex categories that differ with regard to many variables, and so they are not easily ordered.
However, each such set of categories for a nominal scale can be reduced to dichotomies, such as South versus ‘‘not South’’; these variables are commonly called ‘‘dummy variables.’’ This
permits analysis of the dummy variable as though it represented a well-distributed variable. In this case, for example, one could think of the arbitrary underlying variable as being ‘‘southernness,’’ or whatever underlies the conceptualization of the ‘‘South’’ as being different from the rest of the regions. Similarly, returning to the male versus female variable, the researcher has to consider interpretatively what the variable is supposed to represent. Is it distinctly supposed to be a measure of the two biological categories, or is it supposed to represent the social and cultural distinction that underlies them?
Many, if not most, of the variables that are of interest to social and behavioral science are drawn from the common language, and when these are used analytically, many problems or ambiguities become evident. For example, the use of counts is common in demography, and many of the measures that are familiar are accepted with ease. However, as common a concept as city size is not without problems. A city is a legal definition, and so what is a city in one case may be quite different from a city in another case. For example, some cities are only central locations surrounded by many satellite urban centers and suburbs that are also defined as cities, while other cities may be made up of a major central location and many other satellite urban centers and suburbs. To clarify such circumstances, the demographers may develop other concepts, like Standard Metropolitan Areas (SMAs), but this does not solve the problem completely; some SMAs may be isolated, and others may be contiguous. And when is a city a city? Is the definition one that begins with the smallest city with a population of 2,500 (not even a geographical characteristic), or 10,000 population, or 25,000 population? Is city size really a concept that is to be measured by population numbers or area, or by some concept of degree of urban centralization? Is New York City really one city or several? Or is New York City only part of one city that includes the urban complexes around it? The point that is critical is that definitions have to be fixed in arbitrary ways when concepts are drawn practically from the common language, and social concepts are not necessarily parsimoniously defined by some ideal rules of formulating scientific theory. Pragmatic considerations frequently intervene in how data are collected and what data become available for use.
1800
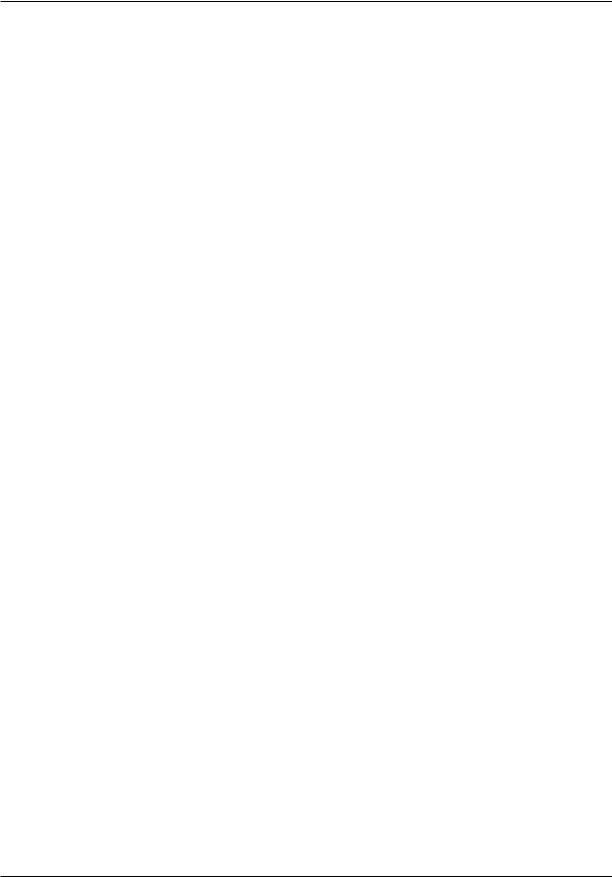
MEASUREMENT
An additional point is appropriate here: that in demography and in other substantive areas, important measures include counts of discrete entities, and these types of measures do not easily fit the Stevens classification of levels of measurement. A discussion of several technical proposals for more exhaustive classifications of types of measures is considered by Duncan (1984).
CONSTRUCTING MEASURES
There are obviously many ways that measures can be constructed. Some have been formalized and diffused, such as Louis Guttman’s cumulative scale analysis, so popular that it has come to be known universally as Guttman scaling, a methodological contribution that was associated with a sociologist and had an appeal for many. An early comprehensive coverage of Guttman scaling can be found in Riley and colleagues (1954). The essence of Guttman scaling is that if a series of dichotomous items is assumed to be drawn from the same universe of content, and if they differ in difficulty, then they can be ordered so that they define scale types. For example, if one examines height, questions could be the following: (1) Are you at least five feet tall?
(2) Are you at least five and a half feet tall? (3) Are you at least six feet tall? (4) Are you at least six and a half feet tall? Responses to these would logically fall into the following types: a + to indicate yes and a - to indicate a no:
1 |
2 |
3 |
4 |
|
|
|
|
+ |
+ |
+ |
+ |
+ |
+ |
+ |
_ |
+ |
+ |
_ |
_ |
+ |
_ |
_ |
_ |
_ |
_ |
_ |
_ |
The types represent ‘‘perfect’’ types, that is, responses made without a logical error. The assumption is that within types, people are equivalent. In the actual application of the procedure, some problems are evident, possibly the most obvious being that there are errors because in applying the procedure in studies, content is not as well specified as being in a ‘‘universe’’ as is the example of height; thus there are errors, and
therefore error types. The error types were considered of two kinds: unambiguous, such as - + + +, which in the example above would simply be illogical, a mistake, and could logically be classed as + + +
+ with ‘‘minimum error.’’ The second kind is ambiguous, such as + + - +, which with one (minimum) error could be placed in either type + + - - or type + + + +.
Experience with Guttman scaling revealed a number of problems. First, few scales that appeared ‘‘good’’ could be constructed with more than four or five items because the amount of error with more items would be large. Second, the error would tend to be concentrated in the ambiguous error type. Third, scales constructed on a particular study, especially with common sample sizes of about one hundred cases, would not be as ‘‘good’’ in other studies. There was ‘‘shrinkage,’’ or more error, particularly for the more extreme items. The issue of what to do with the placement of ambiguous items was suggested by an alternative analysis (Borgatta and Hays 1952): that the type + + - + was not best placed by minimum error, but should be included with the type + + + - between the two minimum error locations. The reason for this may be grasped intuitively by noting that when two items are close to each other in proportion of positive responses, they are effectively interchangeable, and they are involved in the creation of the ambiguous error type. The common error is for respondents who are at the threshold of decision as to whether to answer positively or negatively, and they are most likely to make errors that create the ambiguous error types.
These observations about Guttman scaling lead to some obvious conclusions. First, the scaling model is actually contrary to common experience, as people are not classed in ordered types in general but presumably are infinitely differentiated even within a type. Second, the model is not productive of highly discriminating classes. Third, and this is possibly the pragmatic reason for doubting the utility of Guttman scaling, if the most appropriate place for locating nonscale or error types of the common ambiguous type is not by minimum error but between the two minimum error types, this is effectively the same as adding the number of positive responses, essentially reducing the procedure to a simple additive score. The remaining virtue of Guttman scaling in the logical placement of unambiguous errors must be
1801
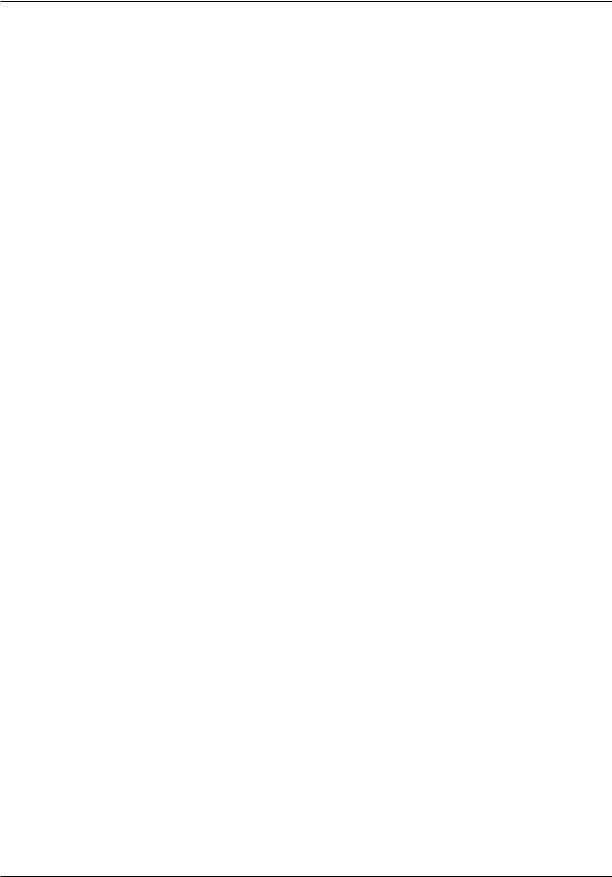
MEASUREMENT
balanced against other limitations, such as the requirement that items must be dichotomous, when much more information can be gotten with more detailed categories of response, usually with trivial additional cost in data collection time.
In contrast with Guttman scaling, simple addition of items into sum scores, carried out with an understanding of what is required for good measurement, is probably the most defensible and useful tool. For example, if something is to be measured, and there appear to be a number of relatively independent questions that can be used to ascertain the content, then those questions should be used to develop reliable measures. Reliability is measured in many ways, but consistency is the meaning usually intended, particularly internal consistency of the component items, that is, high intercorrelation among the items in a measure. Items can ask for dichotomous answers, but people can make more refined discriminations than simple yeses and nos, so use of multiple (ordered) categories of response increases the efficiency of items.
The question of whether the language as used has sufficient consistency to make refined quantitative discriminations does not appear to have been studied extensively, so a small data collection was carried out to provide the following example. People were asked to evaluate a set of categories with the question ‘‘How often does this happen?’’ The instructions stated: ‘‘Put a vertical intersection where you think each category fits on the continuum, and then place the number under it. Categories 1 and 11 are fixed at the extremes for this example. If two categories have the same place, put the numbers one on top of the other. If the categories are out of order, put them in the order you think correct.’’ A continuum was then provided with sixty-six spaces and the external positions of the first and the last indicated as the positions of (1) always and (11) never. The respondents were asked to locate the following remaining nine categories: (2) almost always (3) very often;
(4) often; (5) somewhat often; (6) sometimes; (7) seldom; (8) very seldom; (9) hardly ever; and (10) almost never. It is not surprising that average responses on the continuum are well distributed, with percent locations respectively as 9, 15, 27, 36, 48, 65, 75, 86, and 93; the largest standard deviation for placement location is about 11 percent. Exercises with alternative quantitatively oriented
questions and use of a series of six categories from ‘‘definitely agree’’ to ‘‘definitely disagree’’ provide similar evidence of consistency of meaning. In research, fewer than the eleven categories illustrated here usually used, making the task of discrimination easier and faster for respondents. The point of emphasis is that questions can be designed to efficiently provide more information than simple dichotomous answers and thus facilitate construction of reliable scores.
MEASUREMENT IN THE REAL WORLD
Many variations exist on how to collect information in order to build effective measurement instruments. Similarly, there are alternatives on how to build the measuring instruments. Often practical considerations must be taken into account, such as the amount of time available for interviews, restraints placed on what kinds of content can be requested, lack of privacy when collecting the information, and other circumstances.
With the progressive technology of computers and word processors, the reduced dependence of researchers on assistants, clerks, and secretaries has greatly facilitated research data handling and analysis. Some changes, like Computer Assisted Telephone Interviewing (CATI) may be seen as assisting data collection, but in general the data collection aspects of research are still those that require most careful attention and supervision. The design of research, however, still is often an ad hoc procedure with regard to the definition of variables. Variables are often created under the primitive assumption that all one needs to do is say, ‘‘The way I am going to measure XXX is by responses to the following question.’’ This is a procedure of dubious worth, since building knowledge about the measurement characteristics of the variables to be used should be in advance of the research, and is essential to the interpretation of findings.
A comment that is commonly encountered is that attempting to be ‘‘scientific’’ and developing a strict design for research with well-developed measures forecloses the possibility of getting a broad picture of what is being observed. The argument is then advanced that attempting to observe and accumulate data in systematic research is not as revealing as observing more informally (qualitatively) and ‘‘getting a feel’’ for what is going on.
1802

MEASUREMENT
Further, when systematic research is carried out, so goes the argument, only limited variables can be assessed instead of ‘‘getting the complete picture.’’ This, of course, is the ultimate self-delusion and can be answered directly. If positive findings for the theory do not result from more rigorous, welldesigned research, then the speculative generalizations of more casual observation are never going to be any more than that, and giving them worth may be equivalent to creating fictions to substitute for reality. The fact that attempted systematic empirical research has not produced useful findings does not mean that more intuitive or qualitative approaches are more appropriate. What it means is that the theory may not be appropriate, or the design of the research may be less than adequate.
Further, this does not mean that there is anything wrong with informal or qualitative research. What it does mean is that there is a priority order in the accumulation of knowledge that says that the informal and qualitative stages may be appropriate to produce theory, which is defined as speculation, about what is being observed, and this may then be tested in more rigorous research. This is the common order of things in the accumulation of scientific knowledge.
If a sociological theory has developed, then it must be stated with a clear specification of the variables involved. One cannot produce a volume on the concept of anomie, for example, and then use the word ‘‘anomie’’ to mean twenty different things. The concept on which one focuses must be stated with a clear specification of one meaning, and there are two elements that go into such a definition. The first is to indicate how the concept is to be measured. The second is more commonly neglected, and that is to specify how the concept is differentiated from other concepts, particularly those that are closely related to it in meaning.
The development of well-measured variables in sociology and the social sciences is essential to the advancement of knowledge. Knowledge about how good measurement can be carried out has advanced, particularly in the post–World War II period, but it has not diffused and become sufficiently commonplace in the social science disciplines.
It is difficult to comprehend how substituting no measurement or poor measurement for the best measurement that sociologists can devise can
produce better or more accurate knowledge. Examples of the untenable position have possibly decreased over time, but they still occur. Note for example: ‘‘Focus on quantitative methods rewards reliable (i.e., repeatable) methods. Reliability is a valuable asset, but it is only one facet of the value of the study. In most studies, reliability is purchased at the price of lessened attention to theory, validity, relevance. etc.’’ (Scheff 1991). Quite the contrary, concern with measurement and quantification is concern with theory, validity, and relevance!
Finally, it is worth emphasizing two rules of thumb for sociologists concerned with research, whether they are at the point of designing research or interpreting the findings of a research that has been reported. First, check on how the variables are specified and ask whether they are measured well. This requires that specific questions be answered: Are the variables reliable? How does one know they are reliable? Second, are the variables valid? That is, do they measure what they are supposed to measure? How does one know they do? If these questions are not answered satisfactorily, then one is dealing with research and knowledge of dubious value.
(SEE ALSO: Levels of Analysis: Nonparametric Statistics; Reliability, Validity; Quasi-Experimental Research Design)
REFERENCES
Agresti, Alan, and Barbara Finaly 1997 Statistical Methods for Social Sciences. Englewood Cliffs, N. J.: Prentice Hall.
Babbie, Earl 1995 The Practice of Social Research. Belmont, Calif.: Wadsworth.
Borgatta, Edgar F. 1968 ‘‘My Student, the Purist: A Lament.’’ Sociological Quarterly 9:29–34.
———, and David G. Hays 1952 ‘‘Some Limitations on the Arbitrary Classifications of Non-Scale Response Patterns in a Guttman Scale.’’ Public Opinion Quarterly 16:273–291.
De Vellis, Robert F. 1991 Scale Development. Newbury Park, Calif.: Sage.
Duncan, Otis Dudley 1984 Notes of Social Measurement. New York: Russell Sage Foundation.
Herzog, Thomas 1997 Research Methods and Data Analysis in the Social Sciences. Englewood Cliffs, N.J.: Prentice Hall.
1803
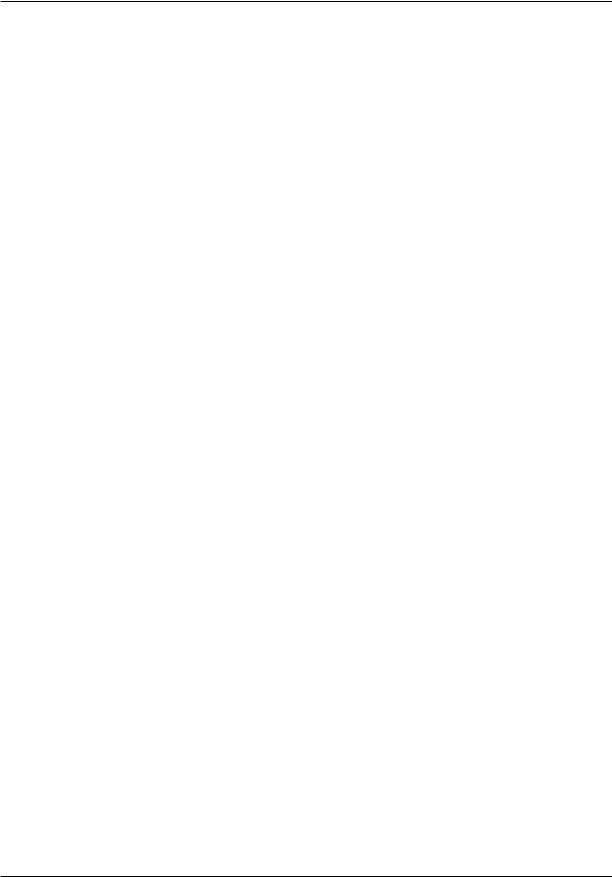
MEASURES OF ASSOCIATION
Kendall, Maurice G. 1948 Rank Correlation Methods.
London: Griffin.
Knoke, David, and George W. Bohrnstedt 1994. Statistics for Social Data Analysis. Itasca, Ill.: Peacock.
Lewis-Beck, Michael, ed. 1994 Basic Measurement. Beverly Hills, Calif.: Sage.
Neuman, Lawrence W. 1997 Social Research Methods:
Qualitative and Quantitative Approaches. Boston: Allyn
and Bacon.
Nunnally, Jum C. 1978 Psychometric Theory. New York:
McGraw-Hill.
Riley, Matilda White, John W. Riley, Jr., and Jackson Toby 1954 Sociological Studies in Scale Analysis. New Brunswick, N.J.: Rutgers University Press.
Scheff, Thomas J. 1991 ‘‘Is There a Bias in ASR Article Selection.’’ Footnotes 19(2, February):5.
Stevens, S. S., ed. 1966 Handbook of Experimental Psychology. New York: John Wiley.
Traub, Ross E. 1994 Reliability for the Social Sciences. Beverly Hills, Calif.: Sage.
EDGAR F. BORGATTA
YOSHINORI KAMO
MEASUREMENT
INSTRUMENTS
See Factor Analysis; Measurement; Quasi-Experi- mental Research Designs; Reliability; Survey Research; Validity.
MEASURES OF ASSOCIATION
Long before there were statisticians, folk knowledge was commonly based on statistical associations. When an association was recognized between stomach distress and eating a certain type of berry, that berry was labeled as poisonous and avoided. For millennia, farmers the world over have observed an association between drought and a diminished crop yield. The association between pregnancy and sexual intercourse apparently was not immediately obvious, not simply because of the lag between the two events, but also because the association is far from perfect—that is, pregnancy does not always follow intercourse. Folk knowledge has also been laced with superstitions, commonly based on erroneously believed statistical associations. For example, people have
believed that there is an association between breaking a mirror and a long stretch of bad luck, and in many cultures people have believed that there is an association between certain ritual incantations and benevolent intervention by the gods.
Scholarly discussions sometimes focus on whether a given association is actually true or erroneously believed to be true. Is there an association between gender and mathematical ability? Between harsh punishment and a low incidence of crime? Between the size of an organization and the tendency of its employees to experience alienation? In contemporary discussions, questions and conclusions may be expressed in terms of ‘‘risk factors.’’ For example, one might seek to find the risk factors associated with dropping out of school, with teen suicide, or with lung cancer. ‘‘Risk factors’’ are features that are associated with these outcomes but that can be discerned prior to the outcome itself. Although a reference to ‘‘risk’’ suggests that the outcome is undesirable, researchers may, of course, explore factors that are associated with positive as well as negative outcomes. For example, one could examine factors associated with appearance in Who’s Who, with the success of a treatment regimen, or with a positive balance of international trade.
Referring to a ‘‘risk factor’’ entails no claim that the associated factor has an effect on the outcome, whereas a statistical association is sometimes erroneously interpreted as indicating that one variable has an effect on the other. To be sure, an interest in the association between two variables may derive from some hypothesis about an effect. Thus, if it is assumed that retirement has the effect of reducing the likelihood of voting, the implication is that retirement and non-voting should be statistically associated. But the reverse does not hold; that is, the fact that two variables are statistically associated does not, by itself, imply that one of those variables has an effect on the other. For example, if it is true that low attachment to parents encourages involvement in delinquency, it should be true that low attachment and delinquency are statistically associated. But a statistical association between low attachment and delinquency involvement might arise for other reasons as well. If both variables are influenced by a common cause, or if both are manifestations of the same underlying tendency, those variables will be statistically associated with each other, even if there is no effect of
1804
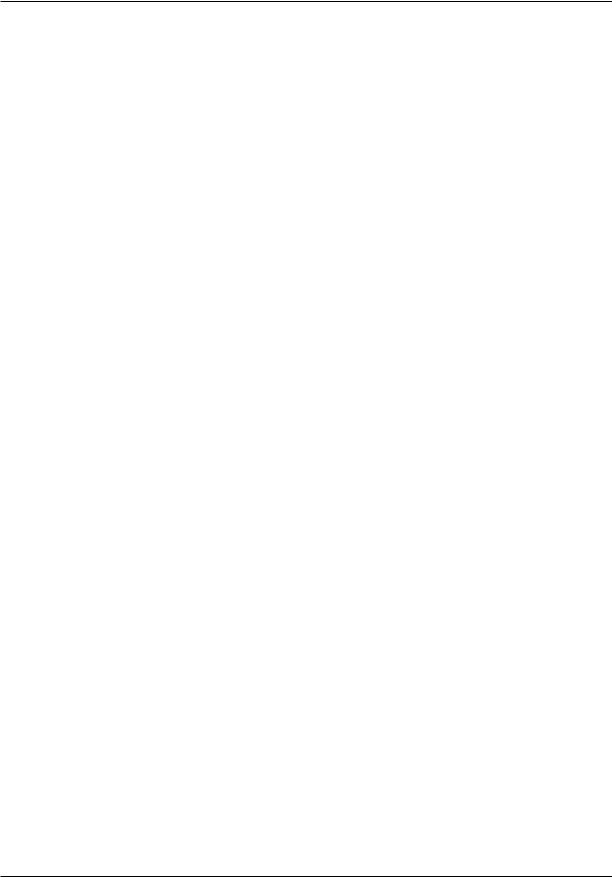
MEASURES OF ASSOCIATION
one on the other. Finding a statistical association between two variables, even a strong association, does not, in itself, tell the reason for that association. It may result from an effect of one variable on another, or from the influence of a common cause on both variables, or because both variables reflect the same underlying tendency. Furthermore, if the association is transitory, it may appear simply because of an accident or coincidence. Discovering the reason for a statistical association always entails inquiry beyond simply demonstrating that the association is there.
WHY MEASURE ASSOCIATION?
The focus here is on measures of association for categorical variables, with brief attention to measures appropriate for ordered categories. Quantitative variables, exemplified by age and income, describe each case by an amount; that is, they locate each case along a scale that varies from low to high. In contrast, categorical variables, exemplified by gender and religious denomination, entail describing each case by a category; that is, they indicate which of a set of categories best describes the case in question. Such categories need not be ordered. For example, there is no inherent ordering for the categories that represent region. But if the categories (e.g., low, medium, and high income) are ordered, it may be desirable to incorporate order into the analysis. Some measures of association have been designed specifically for ordered categories.
The degree of association between categorical variables may be of interest for a variety of reasons. First, if a weak association is found, we may suspect that it is just a sampling fluke—a peculiarity of the sample in hand that may not be found when other samples are examined. The strength of association provides only a crude indication of whether an association is likely to be found in other samples, and techniques of statistical inference developed specifically for that purpose are preferred, provided the relevant assumptions are met.
Second, a measure of the degree of association may be a useful descriptive device. For example, if the statistical association between region and college attendance is strong, that suggests differential access to higher education by region. Furthermore, historical changes in the degree of
association may suggest trends of sociological significance, and a difference in the degree of association across populations may suggest socially important differences between communities or societies. For example, if the occupations of fathers and sons are more closely associated in Italy than in the United States, that suggests higher generational social mobility in the latter than in the former. Considerable caution should be exercised in comparing measures of association for different times or different populations, because such measures may be influenced by a change in the marginal frequencies as well as by a change in the linkage between the variables in question. (See Reynolds 1977)
Third, if a statistical association between two variables arises because of the effect of one variable on the other, the degree of association indicates the relative strength of this one influence as compared to the many other variables that also have such an effect. Unsophisticated observers may assume that a strong association indicates a causal linkage, while a weak association suggests some kind of noncausal linkage. But that would be naïve. The strength of association does not indicate the reason for the association. But if an association appears because of a causal link between two variables, the strength of that association provides a rough but useful clue to the relative importance of that particular cause relative to the totality of other causes. For example, income probably influences the tendency to vote Democratic or Republican in the United States, but income is not the only variable affecting the political party favored with a vote. Among other things, voting for one party rather than another is undoubtedly influenced by general political philosophy, by recent legislative actions attributed to the parties, by specific local representatives of the parties, and by the party preferences of friends and neighbors. Such multiple influences on an outcome are typical, and the degree of association between the outcome and just one of the factors that influence it will reflect the relative ‘‘weight’’ of that one factor in comparison to the total effect of many.
Fourth, if a statistical association between two variables arises because both are influenced by a common cause, or because both are manifestations of the same underlying tendency, the degree of association will indicate the relative strength of the common cause or the common tendency, in
1805
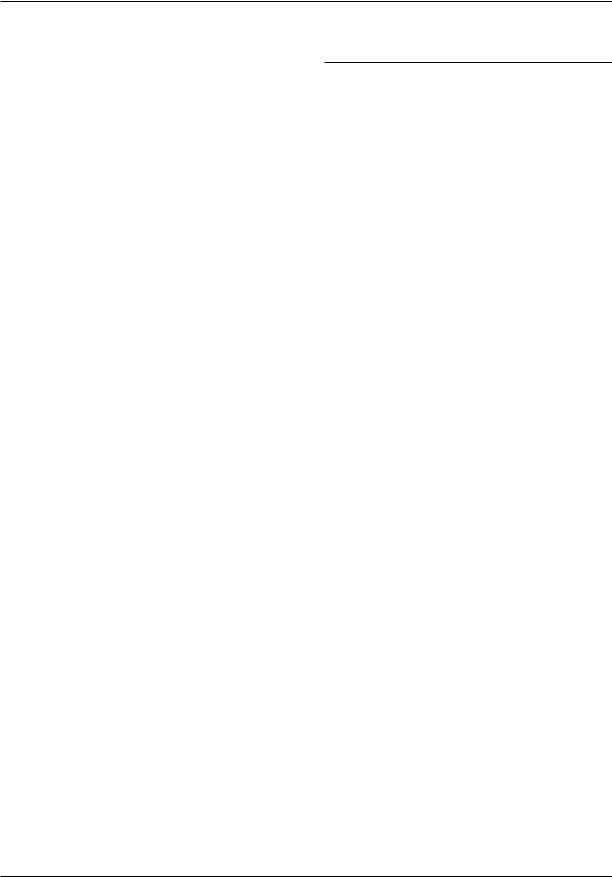
MEASURES OF ASSOCIATION
comparison to the many other factors that influence each of the two variables. Assume, for example, that participation in a rebellious youth subculture influences adolescents to use both alcohol and marijuana. If the resulting association between the two types of substance use is high, this suggests that the common influence of the rebellious youth subculture (and perhaps other common causes) is a relatively strong factor in both. On the other hand, if this association is weak, it suggests that while the rebellious youth subculture may be a common cause, each type of substance use is also heavily influenced by other factors that are not common to both types.
Fifth, the degree of association indicates the utility of associated factors (‘‘risk factors’’) as predictors, and hence the utility of such factors in focusing social action. Assume, for example, that living in a one-parent home is statistically associated with dropping out of high school. If the association between these two variables is weak, knowing which students live in a one-parent home would not be very useful in locating cases on which prevention efforts should be concentrated for maximum effectiveness. On the other hand, if this association is strong, that predictor would be especially helpful in locating cases for special attention and assistance.
In summary, we may be interested in the degree of statistical association between variables because a weak association suggests the possibility that the association is a fluke that will not be replicated, because changes in the degree of association may help discern and describe important social trends or differences between populations, because the degree of association may help determine the relative importance of one variable that influences an outcome in comparison to all other influences, because the degree of association may reflect the degree to which two variables have a common cause, and because the degree of association will indicate the utility of associated factors in predicting an outcome of interest.
MEASURING THE DEGREE OF
ASSOCIATION
The degree of statistical association between two variables is most readily assessed if, for a suitable set of cases, the relevant information is tallied in a cross-classification table. Table 1 displays such a
College Attendence by Race in a Sample of 20-Year-Olds in Centerville, 1998
(Hypothetical Data)
ATTENDING |
|
|
ASIAN- |
|
COLLEGE? |
WHITE |
BLACK |
AMERICAN |
TOTAL |
|
|
|
|
|
Yes |
400 |
60 |
80 |
540 |
No |
300 |
140 |
20 |
460 |
Total |
700 |
200 |
100 |
1,000 |
Table 1
table. For a contrived sample of young adults, this table shows the number who are and who are not attending college in each of three racial groupings. Hence the two variables represented in this table are (1) race (white, black, Asian-American) and (2) attending college (or not) at a given time. The frequencies in the cells indicate how the cases are jointly distributed over the categories of these two variables. The totals for each row and column (the ‘‘marginal frequencies’’ or simply the ‘‘marginals’’) indicate how the cases are distributed over these two variables separately.
If young adults in all racial groupings were equally likely to be attending college, then there would be no association between these two variables. Indeed, the simplest of all measures of association is just a percentage difference. For example, blacks in this set of cases were unlikely to be attending college (i.e., 30 percent were enrolled), while Asian-Americans were very likely to be attending (80 percent). Hence we may say that the percentage attending college in the three racial groupings represented in this table ranges from 30 percent to 80 percent, a difference of 50 percentage points. In this table, with three columns, more than one percentage difference could be cited, and the one alluded to above is simply the most extreme of the three comparisons that could be made between racial groupings. Generally speaking, a percentage difference provides a good description of the degree of association only in a table with exactly two rows and two columns. Even so, citing a percentage difference is a common way of describing the degree of statistical association.
Leaving aside the difference between percentages, most measures of association follow one of two master formulas, and a third way of assessing
1806
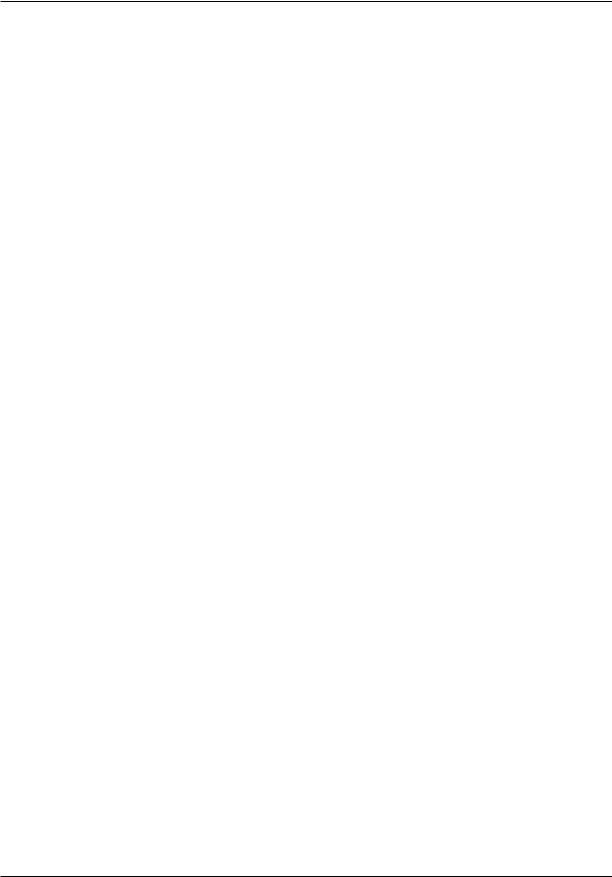
MEASURES OF ASSOCIATION
association provides the basis for analyzing several variables simultaneously. The oldest of these master formulas is based on the amount of departure from statistical independence, normed so that the measure will range from 0 (when the two variables are statistically independent and hence not associated at all) to 1.0 or something approaching 1.0 (when the cross-classification table exhibits the maximum possible departure from statistical independence). The several measures based on this master formula differ from each other primarily in the way the departure from statistical independence is normed to yield a range from 0 to 1.
The second master formula is based on the improvement in predictive accuracy that can be achieved by a ‘‘prediction rule’’ that uses one variable to predict the other, as compared to the predictive accuracy achieved from knowledge of the marginal distribution alone. The several measures based on this master formula differ from each other in the nature of the ‘‘prediction rule’’ and also in what is predicted (e.g., the category of each case, or which of a pair of cases will be higher). When such a measure is 0 there is no improvement in predictive accuracy when one variable is predicted from another. As the improvement in predictive accuracy increases, these measures of association will increase in absolute value up to a maximum of 1, which indicates prediction with no errors at all.
A third important way of assessing association, used primarily when multiple variables are analyzed, is based on the difference in odds. In Table 1, the odds that an Asian-American is attending college are ‘‘4 to 1’’; that is, 80 are in college and 20 are not. If such odds were identical for each column, there would be no association, and the ratio of the odds in one column to the odds in another would be 1.0. If such ratios differ from 1.0 (in either direction), there is some association. An analysis of association based on odds ratios (and more specifically on the logarithm of odds ratios) is now commonly referred to as a loglinear analysis. This mode of analysis is not discussed in detail here.
Departure from Statistical Independence. The traditional definition of statistical independence is expressed in terms of the probability of events; that is, events A and B are statistically independent if, and only if:
P(A/B) = P(A) |
(1) |
‘‘P(A)’’ may be read as the probability that event A occurs. This probability is usually estimated empirically by looking at the proportion of all relevant events (A plus not-A) that are A. Referring to Table 1, if event A means attending college, then the probability of event A is estimated by the proportion of all relevant cases that are attending college. In Table 1, this is .54 (i.e., 540 were attending out of the table total of 1,000).
‘‘P(A|B)’’ may be read as the conditional probability that event A occurs, given that event B occurs, or, more briefly ‘‘the probability of A given B.’’ This conditional probability is usually estimated in a manner parallel to the estimation of P(A) described above, except that the relevant cases are limited to those in which event B occurs. Referring to Table 1 again, if event A means attending college and event B refers to being classified as Asian-American, then P(A|B) = .80 (i.e., 80 are attending college among the 100 who are AsianAmerican).
As indicated above, the traditional language of probability refers to ‘‘events,’’ whereas the traditional language of association refers to ‘‘variables.’’ But it should be evident that if ‘‘events’’ vary in being A or not-A, then we have a ‘‘variable.’’ The difference between the language used in referring to the probability of ‘‘events’’ and the language used in referring to a statistical association between ‘‘variables’’ need not be a source of confusion, since one language can be translated into the other.
If we take as given the ‘‘marginal frequencies’’ in a cross-classification table (i.e., the totals for each row and each column), then we can readily determine the probability of any event represented by a category in the table; that is, we can determine P(A). Since P(A|B) = P(A) if statistical independence holds, we can say what P(A|B) would be for any A and B in the table if statistical independence held. Otherwise stated, if the marginal frequencies remain fixed, we can say what frequency would be expected in each cell if statistical independence held. Referring again to Table 1 and assuming the marginal frequencies remain fixed as shown, if statistical independence held in the table, then 54 percent of those in each racial grouping would be attending college. This is because, if statistical
1807