
Encyclopedia of SociologyVol._3
.pdf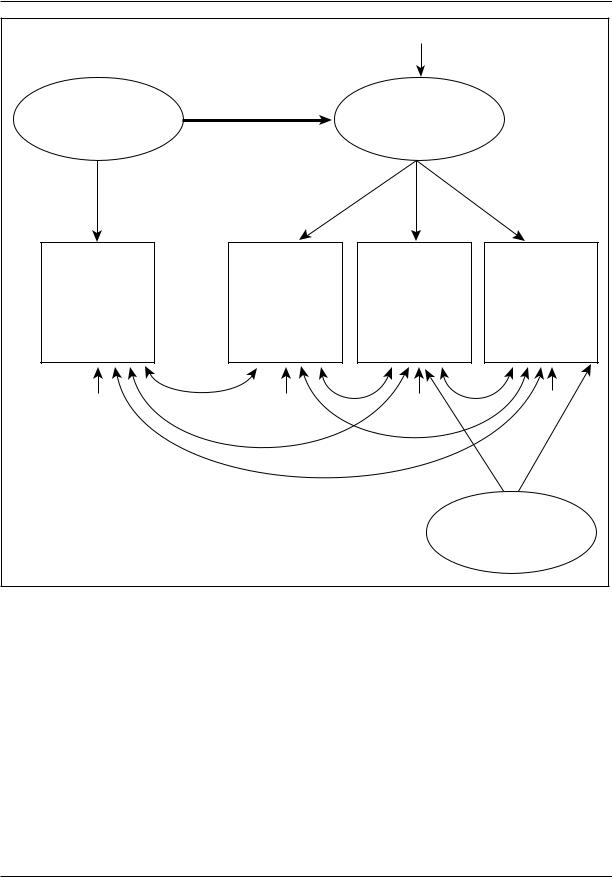
MULTIPLE INDICATOR MODELS
|
|
D |
|
|
I |
X = .4 |
LS |
|
|
Actual |
Actual |
|
|
|
|
|
|
||
Income |
True |
Life Satisfaction |
|
|
|
Effect |
|
|
|
a = 1 . 0 |
b = .5 |
c = .7 |
d=.7 |
|
|
|
|
|
|
I1 |
LS1 |
LS2 |
|
LS3 |
Measured |
Measured |
Measured |
|
Measured |
Income |
Life Satisfaction |
Life Satisfaction |
Life Satisfaction |
|
Response to |
Response to |
Response to |
|
Response to |
“How much money |
“I am satisfied |
“In most ways |
|
“The conditions |
with my life.” |
|
|||
do you earn yearly?” |
my life is close |
|
of my life |
|
|
|
|||
|
|
to my ideal.” |
|
are excellent.” |
r 1 = . 2 0 |
r 4 = . 3 5 |
r 6 = . 8 5 |
||
E1 |
E2 |
E3 |
|
E4 |
|
r 2 = . 2 8 |
r 5 = . 3 5 |
|
|
|
r 3 = . 2 8 |
|
|
|
|
|
|
e = . 6 |
f = . 6 |
|
|
|
|
O |
|
|
|
|
Actual |
|
|
|
Optimism |
Figure 3. Multiple-Indicator Model for Estimating the Effect of Income on Life Satisfaction with Nonrandom Measurement Error for Optimism*.
NOTE: *To obtain an overidentified model, paths k and l are ‘‘constrained’’ to have equal path coefficients.
In the absence of good fit—as a consequence of not allowing paths e and f to be included in the model to be tested (i.e., not specifying the ‘‘correct’’ model)—the SEM program might continue to ‘‘iterate’’ by substituting new estimates for c and the other causal paths in Figure 3—b, d, and x— which initially have unknown values. No matter what values are estimated for each of these causal paths, however, the program will not be able to eliminate a discrepancy between the implied and
the observed correlations for at least some of the pairs of indicators—a discrepancy that, as noted previously, the program will attempt to minimize based on the criterion of finding path estimates that result in the lowest average discrepancy summed across all possible comparisons of implied versus observed correlations in the model.
Indeed, a misspecification in one part of a model (in this instance, failure to model nonrandom
1918
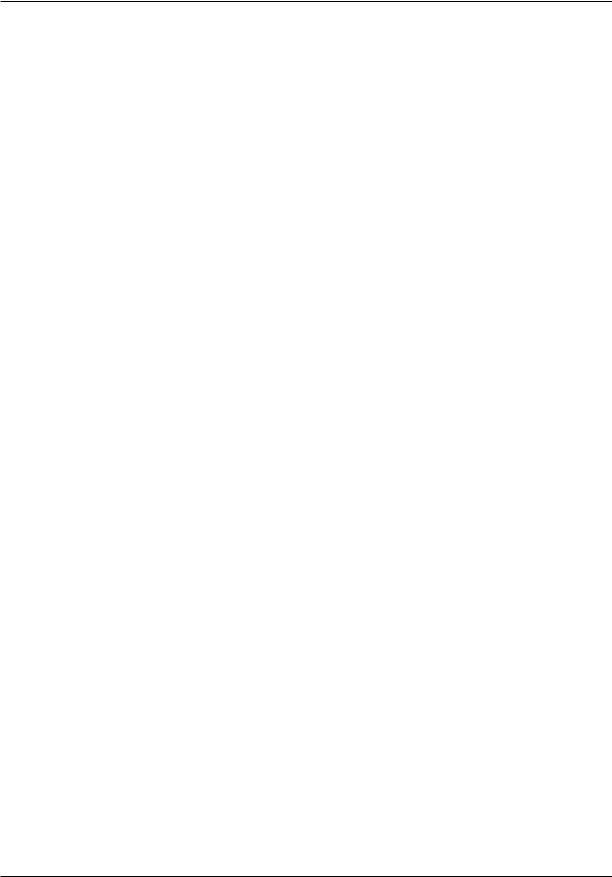
MULTIPLE INDICATOR MODELS
measurement error in LS1 and LS2 indicators) generally ‘‘reverberates’’ throughout the causal model, as the SEM program attempts to make adjustments in all path coefficients to minimize the average discrepancy. In other words, estimates for each path coefficient are most often at least slightly biased by misspecified models (the greater the misspecification, the greater the bias). Most importantly, the failure to model (and thus correct for) nonrandom measurement error will typically result in the SEM program’s estimating a value for the true effect of one construct on another—in the present example, the true effect (path x) of income on life satisfaction—that will be biased (either overor underestimated). For example, when we use a SEM program (EQS) to calculate estimates for the hypothesized causal paths in Figure 3 (excluding the paths for the contamination of optimism), we obtain a final solution with the following estimates: b= .38, c= .92, d= .92, and x =
.31. Thus, in the present example, the failure to model nonrandom measurement error has led to an underestimate (x= .31) of income’s true effect (x = .40) on life satisfaction. Interestingly, by far the largest discrepancy in fit occurs between I1 and LS1 (the implied correlation is .08 less than the observed correlation), not between LS2 and LS3(where there is 0 discrepancy)—demonstrating that misfit in one part of the model can produce misfit (reverberate) elsewhere in the model.
The hypothetical model depicted in Figure 3 is necessarily artificial (to provide simple illustrations of SEM principles). In real-life situations, it is unlikely (though still possible) that sources of contamination, when present, would impact only some of the multiple indicators for a given construct (e.g., only two of the three measures of life satisfaction). Furthermore, researchers would be on shaky ground if they were to actually claim that the correlated measurement error modeled in the present example stemmed from an identifiable source—such as optimism. (Indeed, to avoid specifically labeling the source of the inflated correlation between LS1 and LS2, researchers would most likely model a curved, double-headed arrow between E3 and E4 in Figure 3, and have the SEM program simply estimate the appropriate val- ue—.36—for this new path representing correlated measurement error.) To make a legitimate claim that a construct like optimism contaminates a construct such as life satisfaction, researchers
would also need to provide traditional measures of the suspected source of contamination (e.g., include established multiple indicators for optimism).
As a final example of the need to use overidentified multiple indicator models in order to detect misspecified models, consider a modification of Figure 3 in which we have access to the single indicator for income (I1) but have only two indicators for life satisfaction available to us—LS2 and LS3. Assume, further, that we are unaware that optimism contaminates the two life satisfaction indicators. Note that this new model is just identified. That is, there are three observed correlations (r2, r3, and r6) and three hypothesized causal paths to estimate (x, c, and d). Accordingly, this model will perfectly fit the data, despite the fact that we have not modeled (i.e., misspecified) the correlated measurement error (.36) that we’ve built into the .85 observed correlation (r6) between LS2 and
LS3.
More specifically, in order to reproduce the
.85 correlation, the SEM program will increase the estimate of reliability for the LS2 and LS3 life satisfaction indicators by increasing the c and d paths from their original (true) value of .7 each to new (biased) values of about .92 each. To reproduce the observed correlation of .28 for both I1/ LS2 and I1/LS3 (r2 and r3), the program can simply decrease the x path (i.e., the estimate of the true effect of income on life satisfaction) from its original (true) value of .40 to a new (biased) value of about .30—that is, for both path equations a*x*c and a*x*d: 1.0*.3*.92 . .28.
In sum, our just-identified model perfectly fits the data despite the fact our model has failed to include (i.e., has misspecified) the correlated measurement error created by optimism contaminating the two life satisfaction indicators. More importantly, the failure to model the nonrandom measurement error in these two indicators has led us to a biased underestimate of the true effect of income and life satisfaction: Income explains .32 = 9% of the variance in life satisfaction for the current (biased) model, but explains .42 = 16% variance in life satisfaction for the earlier (correctly specified) model.
As noted earlier, the debilitating effects of nonrandom measurement error are extremely diverse in their potential forms and correspondingly
1919
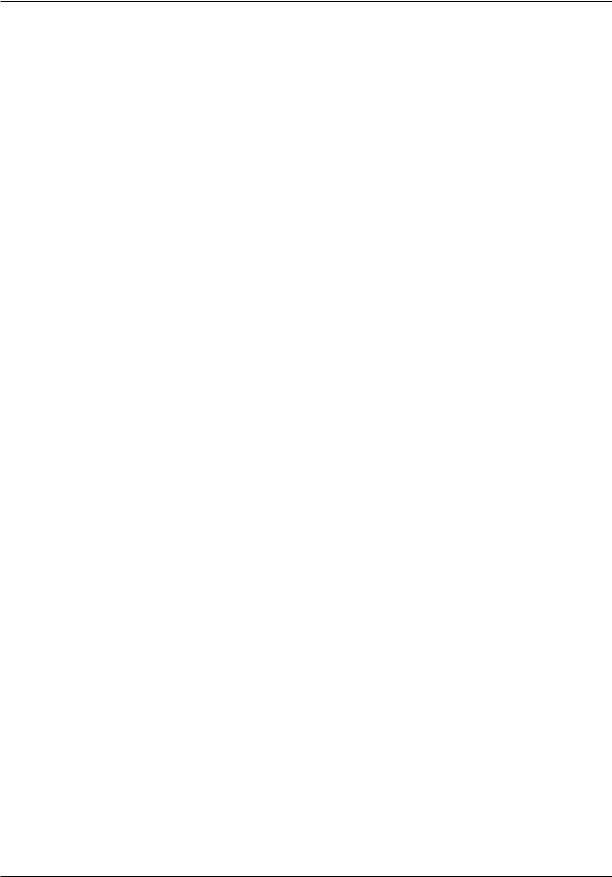
MULTIPLE INDICATOR MODELS
complex in their potential bias in underor overestimating causal links among latent constructs. The present discussion only touches on the issues and possible multiple indicator models for detecting and correcting this type of measurement error. The reader is encouraged to consult other sources that provide additional details (starting with Kline 1998).
STRENGTHS AND WEAKNESSES OF MULTIPLE-INDICATOR MODELS USING SEM
As we have seen, by incorporating multiple indicators of constructs, structural equation modeling procedures allow more rigorous tests of causal models than possible when using single indicators. With sufficient multiple indicators to provide an overidentified model, SEM procedures are particularly powerful in detecting and correcting for the bias created by less than perfectly reliable and valid measures of latent constructs of interest. In other words, by building a more accurate measurement model—that is, providing a more correct specification of the causal linkages between latent constructs and their indicators—SEM researchers can obtain more accurate estimates of the causal linkages in the structural model—that is, the effect of one latent variable on another.
A correct specification of the measurement model is, then, a means to the ultimate goal of obtaining accurate path estimates (also described as ‘‘parameter estimates’’) for the structural model (e.g., obtaining the true effect of income on life satisfaction by correcting for measurement error). Some research, however, makes the measurement model the central focus (e.g., whether the three indicators of life satisfaction correlate with each other in a manner consistent with their measuring a single latent construct). In this situation, the SEM procedure is known as ‘‘confirmatory factor analysis’’ (CFA). The CFA uses the typical SEM principle of positing a measurement model in which latent constructs (now described as ‘‘factors’’) impact multiple indicators (the path coefficient now described as a ‘‘factor loading’’). Unlike a ‘‘fullblown’’ SEM analysis, however, the CFA does not specify causal links among the latent constructs (factors), that is, does not specify a structural model (e.g., there is no interest in whether income affects life satisfaction). Instead, the CFA treats
potential relationships among any two factors as simply a ‘‘correlation’’ (typically represented in diagrams with a curved, double-headed arrow between the factors); that is, the causal ordering among latent constructs is not specified.
Researchers have developed especially powerful CFA procedures for establishing the validity of measures through significant enhancements to traditional construct validity techniques based on multitrait/multimethods (MTMM), second-order factor structures, and testing the invariance of measurement models across diverse subsamples (again, see Kline 1998 for an introduction). CFA has also been at the vanguard in developing ‘‘nonlinear’’ factor analysis to help reduce the presence of ‘‘spurious’’ or ‘‘superfluous’’ latent constructs (referred to as ‘‘methods’’ or ‘‘difficulty’’ factors) that can occur as a consequence of using multiple indicators that are highly skewed (i.e., non-normally distributed) (Muthen 1993, 1998). These nonlinear factor analysis procedures also provide a bridge to recent advances in psychometric techniques based on item-response theory (cf. Reise et al. 1993; Waller et al. 1996).
CFA provides access to such powerful psychometric procedures as a consequence of its enormous flexibility in specifying the structure of a measurement model. But this strength is also its weakness. CFA requires that the researcher specify all aspects of the measurement model a priori. Accordingly, one must have considerable knowledge (based on logic, theory, or prior empirical evidence) of what the underlying structure of the measurement model is likely to be. In the absence of such knowledge, measurement models are likely to be badly misspecified. Although SEM has available particularly sophisticated techniques for detecting misspecification, simulation studies indicate that these techniques do not do well when the hypothesized model is at some distance from the true model (cf. Kline 1998).
Under these circumstances of less certainty about the underlying measurement model, researchers often use ‘‘exploratory factor analysis’’ (EFA). Unlike CFA, EFA requires no a priori specification of the measurement model (though it still works best if researchers have some idea of at least the likely number of factors that underlie the set of indicators submitted to the program). Individual
1920
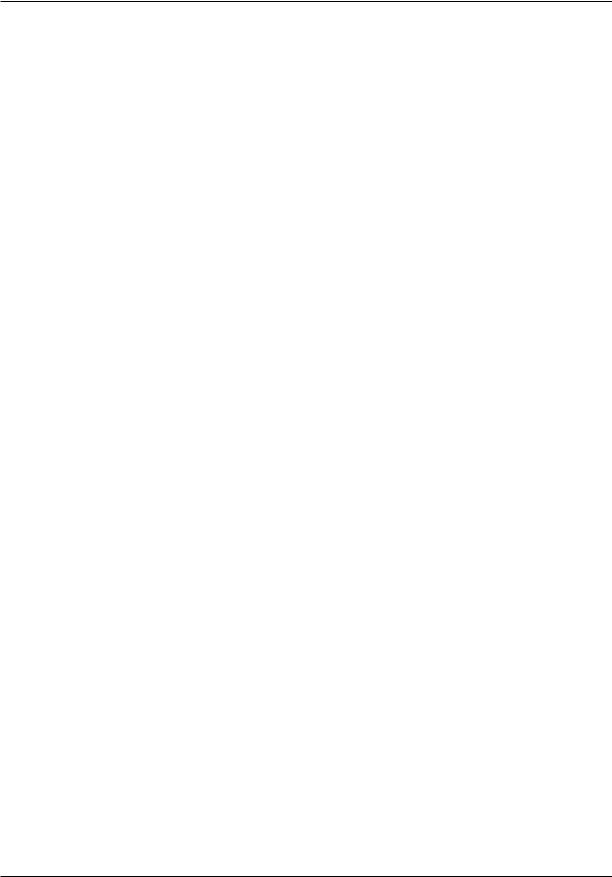
MULTIPLE INDICATOR MODELS
indicators are free to ‘‘load on’’ any factor. Which indicators load on which factor is, essentially, a consequence of the EFA using the empirical correlations among the multiple indicators to seek out ‘‘clusters’’ of items that have the highest correlations with each other, and designating a factor to represent the underlying latent construct that is creating (is the ‘‘common cause’’ of) the correlations among items. Of course, designating these factors is easier said then done, given that the EFA can continue to extract additional factors, contingent on how stringent the standards are for what constitutes indicators that are ‘‘more’’ versus ‘‘less’’ correlated with each other. (For example, does a set of items with correlations of, say, .5 with each other constitute the same or a different ‘‘cluster’’ relative to other indicators that correlate .4 with the first cluster and .5 with each other? Extracting more factors will tend to separate these clusters into different factors; extracting fewer factors is more likely to combine the clusters into one factor.) The issue of the number of factors to extract is, then, a major dilemma in using EFA, with a number of criteria available, including the preferred method, where possible, of specifying a priori the number of factors to extract (cf. Floydand Widaman 1995).
A potential weakness with EFA is that it works best with relatively ‘‘simple’’ measurement models. EFA falters in situations where the reasons for the ‘‘clustering’’ of indicators (i.e., interitem correlations) stem from complex sources (see Bollen 1989 for an example). As an aspect of this inability to handle complex measurement models, EFA cannot match CFA in the sophistication of its tests for validity of measures. On the other hand, because CFA is more sensitive to even slight misspecifications, it is often more difficult to obtain satisfactory model ‘‘fit’’ with CFA.
Exploratory and confirmatory factor analysis can complement each other (but see Floyd and Widaman 1995 for caveats). Researchers may initially develop their measurement models based on EFA, then follow that analysis with CFA (preferably on a second random subsample, in order to avoid modeling ‘‘sampling error’’). In this context, the EFA serves as a crude ‘‘first cut,’’ which it is hoped, results in a measurement model that is close enough to the ‘‘true model’’ that a subsequent CFA can further refine the initial model,
using the more sophisticated procedures available with the confirmatory procedure.
Even though researchers use EFA and CFA to focus exclusively on measurement models, these psychometric studies are really a preamble to subsequent work in which the measures previously developed are now used to test causal linkages among constructs in structural models. Most of these subsequent tests of causal linkages among constructs (e.g., the effect of income on life satisfaction) use data analytic procedures, such as ordinary least squares (OLS) regression or analysis of variance (ANOVA) that assume no underlying latent structure. Accordingly, these traditional analyses of structural models work with measured (observable) variables only, can accommodate only a single indicator per construct of interest and, therefore, must assume (in essence) that each single indicator is perfectly reliable and valid. In other words, in the absence of a multiple indicators measurement model for each construct, there is no way to make adjustments for less-than-perfect reliability or validity.
In an attempt to enhance the reliability of the single-indicator measures that traditional data analyses procedures require, researchers often combine into a single composite scale the set of multiple indicators (e.g., the three indicators of life satisfaction) that prior EFA and CFA research has established as measuring a given latent construct (factor). Although, as noted earlier, a single-indicator composite scale (e.g., summing a respondent’s score on each of the three life satisfaction indicators) is generally preferable to a noncomposite single indicator (at least when measuring more subjective and abstract phenomena), a composite scale is still less than perfectly reliable (often, much less). Accordingly, using such an enhanced (more reliable) measure will still result in biased parameter estimates in the structural model.
Validity also remains a concern. Even if prior psychometric work on measures has rigorously addressed validity (though this is often not the case), there is always a question of how valid the measure of a given construct is in the context of other constructs in the current structural model (e.g., what sort of ‘‘cross-contamination’’ of measures may be occurring among the constructs that might bias estimates of their causal linkages).
1921
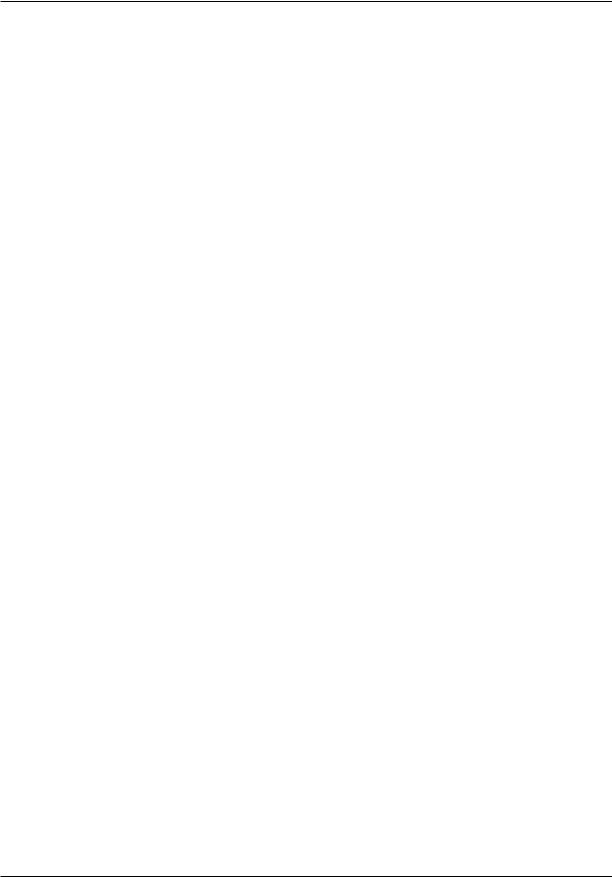
MULTIPLE INDICATOR MODELS
SEM remains a viable alternative to the more traditional and widely used data analysis procedures for assessing causal effects in structural models. Indeed, SEM is so flexible in specifying the structural, as well as measurement part of a causal model, that it can literally ‘‘mimic’’ most of the traditional, single indicator, data analysis procedures based on the general linear model (e.g., Analysis of Variance [ANOVA], Multivariate Analysis of Variance [MANOVA], Ordinary Least Squares [OLS] regression), as well as the newer developments in these statistical techniques (e.g., Hierarchical Linear Modeling [HLM]). SEM can even incorporate the single-indicator measurement models (i.e., based on observable variables only) that these other data analysis procedures use. The power to adjust for random and nonrandom measurement error (unreliability and invalidity) is realized, however, only if the SEM includes a multipleindicator (latent variable) measurement model.
A multiple-indicator measurement model also provides SEM procedures with a particularly powerful tool for modeling (and thus adjusting for) correlated measurement errors that inevitably occur in analyses of longitudinal data (cf. Bollen 1989). Likewise, multiple-indicator models allow SEM programs to combine latent growth curves and multiple subgroups of cohorts in a ‘‘cohortsequential’’ design through which researchers can examine potential cohort effects and developmental sequences over longer time frames than the period of data collection in the study. By also forming subgroups based on their pattern of attrition, (i.e., at what wave of data collection do respondents drop out of the study), SEM researchers can analyze the ‘‘extended’’ growth curves while adjusting for the potential bias from nonrandom missing data (Duncan and Duncan 1995)—a combination of important features that non-SEM methods cannot match. Furthermore, although SEM is rarely used with experimental research designs, multi- ple-indicator models provide exceptionally powerful methods of incorporating ‘‘manipulation checks’’ (i.e., whether the experimental treatment manipulates the independent variable that was intended) and various ‘‘experimental artifacts’’ (e.g., ‘‘demand characteristics’’) as alternative causal pathways to the outcome variable of interest (cf. Blalock 1985).
Although this article has focused on strengths of the SEM multiple indicator measurement model, the advantages of SEM extend beyond its ability to model random and nonrandom measurement error. Particularly noteworthy in this regard is SEM’s power to assess both contemporaneous and lagged reciprocal effects using nonexperimental longitudinal data. Likewise, SEM has an especially elegant way of incorporating maximum likelihood full-information imputation procedures for handling missing data (e.g., Arbuckle 1996; Schafer and Olsen 1998), as an alternative to conventional listwise, pairwise, and mean substitution options. Simulation studies show that the full-information procedures for missing data, relative to standard methods, reduce both the bias and inefficiency that can otherwise occur in estimating factor loadings in measurement models and causal paths in structural models.
So given all the apparent advantages of SEM, why might researchers hesitate to use this technique? As noted earlier in discussing limitations of confirmatory factor analysis, SEM requires that a field of study be mature enough in its development so that researchers can build the a priori causal models that SEM demands as input. SEM also requires relatively large sample sizes to work properly. Although recommendations vary, a minimum sample size of 100 to 200 would appear necessary in order to have a reasonable chance of getting solutions that ‘‘converge’’ or that provide parameter (path) estimates that are not ‘‘out of range’’ (e.g., a negative variance estimate) or otherwise implausible. Experts often also suggest that the ‘‘power’’ to obtain stable parameter estimates (i.e. the ability to detect path coefficients that differ from zero) requires the ratio of subjects-to- parameters-estimated be between 5:1 and 20:1 (the minimum ratio increasing as variables become less normally distributed). In other words, more complex models demand even larger sample sizes.
Apart from the issue of needed a larger N to accommodate more complex causal models, there also is some agreement among SEM experts that models should be kept simpler in order to have a reasonable chance to get the models to fit well (cf., Floyd and Widaman 1995). In other words, SEM works better where the total number of empirical indicators in a model is not extremely large (say 30 or less). Accordingly, if researchers wish to test
1922
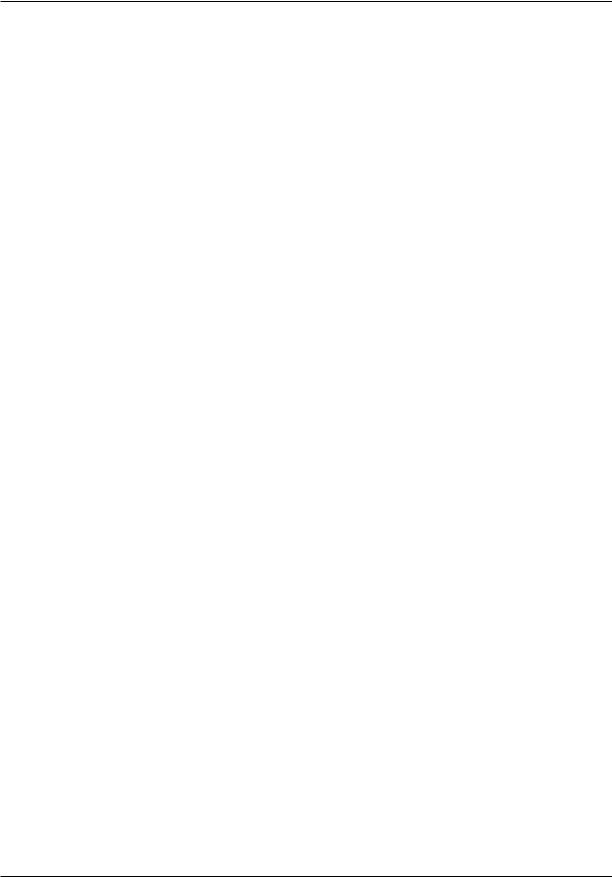
MULTIPLE INDICATOR MODELS
causal models with many predictors (latent constructs) or wish to model fewer constructs with many indicators per construct, SEM may not be the most viable option. Alternatively, one might consider ‘‘trimming’’ the proposed model of constructs and indicators through more ‘‘exploratory’’ SEM procedures of testing and respecifying models, possibly buttressed by preliminary model trimming runs with statistical techniques that can accommodate a larger number of predictors (e.g., OLS regression), and with the final model hopefully tested (confirmed) on a second random subsample. Combining pairs or larger ‘‘parcels’’ from a common set of multiple indicators is another option for reducing the number of total indicators, and has the added benefit of providing measures that have more normal distributions and fewer correlated errors from idiosyncratic ‘‘content overlap.’’ (One needs to be careful, however, of eliminating too many indicators, given that MacCallum et al. [1996] have shown that the power to detect good-fitting models is substantially reduced as degrees of freedom decline.)
An additional limitation on using SEM has been the complex set of procedures (based on matrix algebra) one has had to go through to input the model to be tested—accompanied by output that was also less than user-friendly. Fortunately, newer versions of several SEM programs can now use causal diagrams for both input and output of the structural and measurement models. Indeed, these simple diagramming options threaten to surpass the user-friendliness of the more popular mainstream software packages (e.g., Statistical Package for the Social Sciences [SPSS]) that implement the more traditional statistical procedures. This statement is not meant to be sanguine, however, about the knowledge and experience required, and the care one must exercise, in using SEM packages. In this regard, Kline (1998) has a particularly excellent summary of ‘‘how to fool yourself with SEM.’’ The reader is also encouraged to read Joreskog (1993) on how to use SEM to build more complex models from simpler models.
As the preceding discussion implies, using multiple-indicator models requires more thought and more complicated procedures than does using more common data analytic procedures. However, given the serious distortions that measurement errors can produce in estimating the true causal links among concepts, the extra effort in
using multiple-indicator models can pay large dividends.
REFERENCES
Arbuckle, J. L. 1996 ‘‘Full Information Estimation in the Presence of Incomplete Data. In G. Marcoulides and R. Schumacker eds., Advanced Structural Equation Modeling: Issues and Techniques. Mahwah, N. J.: Lawrence Erlbaum.
——— 1997 Amos User’s Guide Version 3.6. Chicago: SPSS.
Austin, J., and R. Calderon 1996 ‘‘Theoretical and Technical Contributions to Structural Equation Modeling: An Updated Annotated Bibliography.’’ Structural Equation Modeling 3:105–175.
———, and L. Wolfe 1991 ‘‘Annotated Bibliography of Structural Equation Modeling: Technical Work.’’ British Journal of Mathematical and Statistical Psychology
44:93–152.
Bentler, P.M. 1995. EQS: Structural Equations Program Manual. Encino, Calif.: Multivariate Software.
Blalock, H. M. 1979 Social Statistics, 2nd ed. New York: McGraw-Hill.
———, ed. 1985 Causal Model in Panel and Experimental Designs. New York: Aldine.
Bollen, K. A. 1989 Structural Equations with Latent Variables. New York: John Wiley.
Diener, E., R. A. Emmons, R. J. Larsen, and S. Griffin 1985 ‘‘The Satisfaction with Life Scale.’’ Journal of Personality Assessment 49:71–75.
Duncan, T. E., and S. C. Duncan 1995 ‘‘Modeling the Processes of Development via Latent Variable Growth Curve Methodology.’’ Structural Equation Modeling
2:187–213.
Floyd, F. J., and K. F. Widaman 1995 ‘‘Factor Analysis in the Development and Refinement of Clinical Assessment Instruments.’’ Psychological Assessment 7:286–299.
Haring, M. J., W. A. Stock, and M. A. Okun 1984 ‘‘A Research Synthesis of Gender and Social Class as Correlates of Subjective Well-Being.’’ Human Relations 37: 645–657.
Joreskog, K. G. 1993 ‘‘Testing Structural Equation Models.’’ In K. A. Bollen and J. S. Long, eds., Testing Structural Equation Models. Thousand Oaks, Calif.: Sage.
———, and D. Sorbom 1993 Lisrel 8: User’s Reference Guide. Chicago: Scientific Software.
Kim, J., and C. W. Mueller 1978 Introduction to Factor Analysis: What It Is and How to Do It. Beverly Hills, Calif.: Sage.
Kline, R. B. 1998 Principles and Practice of Structural Equation Modeling. New York: Guilford.
1923
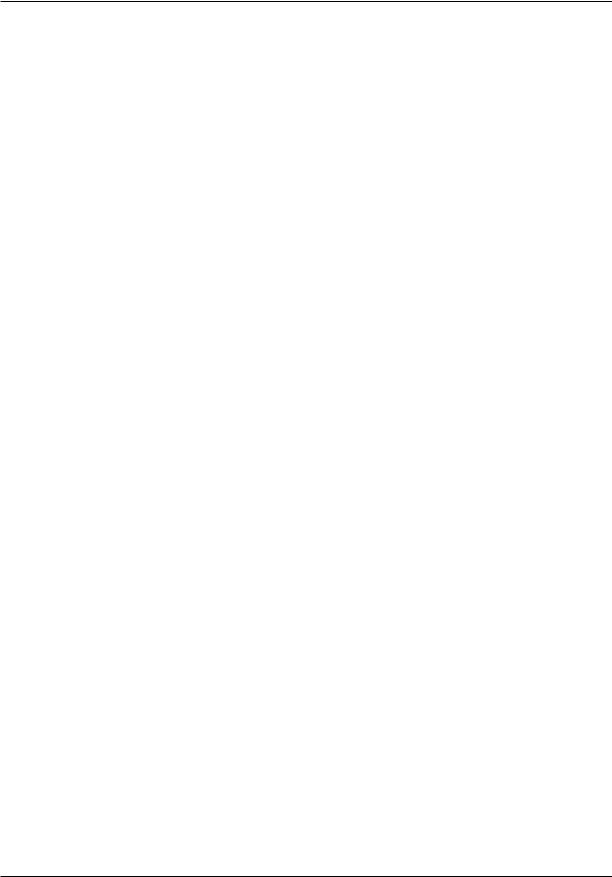
MUSIC
Larson, R. 1978 ‘‘Thirty Years of Research on the Subjective Well-Being of Older Americans. Journal of Gerontology 33:109–125.
Loehlin, J. C. 1998 Latent Variable Models: An Introduction to Factor, Path, and Structural Analysis, 3rd ed. Hillsdale, N.J.: Lawrence Erlbaum.
Marcoulides, G. A., and R. E. Schumacker, eds. 1996
Advanced Structural Equation Modeling: Issues and Techniques. Mahwah, N.J.: Lawrence Erlbaum.
Muthen, B. O. 1993 ‘‘Goodness of Fit with Categorical and Other Nonnormal Variables.’’ In K. A. Bollen and J. S. Long, eds., Testing Structural Equation Models. Thousand Oaks, Calif.: Sage.
——— 1998 Mplus User’s Guide: The Comprehensive Modeling Program for Applied Researchers. Los Angeles, Calif.: Muthen and Muthen.
Nunnally, J. C., and I. H. Bernstein 1994 Psychometric Theory, 3rd ed. New York: McGraw-Hill.
Randall, R. E., and G. A. Marcoulides (eds.) 1998 Interaction and Nonlinear Effects in Structural Equation Modeling. Mahwah, N. J.: Lawrence Erlbaum.
Reise, S. P., K. F. Widaman, and R. H. Pugh 1993 ‘‘Confirmatory Factor Analysis and Item Response Theory: Two Approaches for Exploring Measurement Invariance.’’ Psychological Bulletin 114:552–566.
Schafer, J. L., and M. K. Olsen 1998 ‘‘Multiple Imputation for Multivariate Missing-Data Problems: A Data Analyst’s Perspective.’’ Unpublished manuscript, Pennsylvania State University. http://www.stat.psu.edu/ ˜jls/index.html/#res.
Sullivan, J. L., and S. Feldman 1979 Multiple Indicators: An Introduction. Beverly Hills, Calif.: Sage.
Waller, N. G., A. Tellegen, R. P. McDonald, and D. T. Lykken 1996 ‘‘Exploring Nonlinear Models in Personality Assessment: Development and Preliminary Validation of a Negative Emotionality Scale.’’ Journal of Personality 64:545–576.
KYLE KERCHER
MUSIC
From the earliest days of the discipline, music has been a focus of sociological inquiry. Max Weber, for example, used the development of the system of musical notation as a prime illustration of what he saw as the increasing rationalization of European society since the Middle Ages (Max Weber 1958). Like Weber, many since have used music
and music making as a strategic research site for answering sociologically important questions. Nevertheless, music has not become the focus of a distinctive fundamental approach in sociology comparable to topics like ‘‘socialization,’’ ‘‘organization,’’ ‘‘deviance,’’ and ‘‘culture.’’ That is why the subject of this entry is the ‘‘sociology of music’’ and not ‘‘musical sociology’’ and why it takes such a long bibliography to suggest the range of work in the field.
While no musical sociology has developed, over the decades numerous aspects of music making or appreciation have been the substantive research site for addressing central questions in sociology. Broadly, these can be grouped together as six ongoing research concerns, and together they can be said to constitute the scattered but rich sociology of music. These six focuses will be considered in turn.
MACROSOCIOLOGY
Many sociologists have been concerned with the relationship between society and culture. Talcott Parsons, among others, believed that there was a close fit between the two, so that it would be possible to compare societies and changes taking place in society by studying elements of their cultures including music. This is what led Max Weber (1958) to focus on music as an example of the rationalization of Western society and led another early sociologist, Georg Simmel ([1882] 1968), to see music as a mirror of socio-psychologi- cal processes. The most ambitious cross-national attempt to directly link the structure of society with musical expression has been that of Lomax (1968).
Contemporary scholars see the links between social structure and music not as natural but as deliberately constructed, and they focus on the circumstances of that process. For example, Cerulo (1999) has found an association between the circumstances of a nation’s founding and its national anthem. Whisnant (1983) examined the politics of culture involved in constructing the idea of Appalachian folk culture, and Cloonan (1997) examines how ‘‘Englishness’’ is constructed in contemporary British pop music.
Another line of contemporary studies exploring the society-music link focuses on the globalization
1924
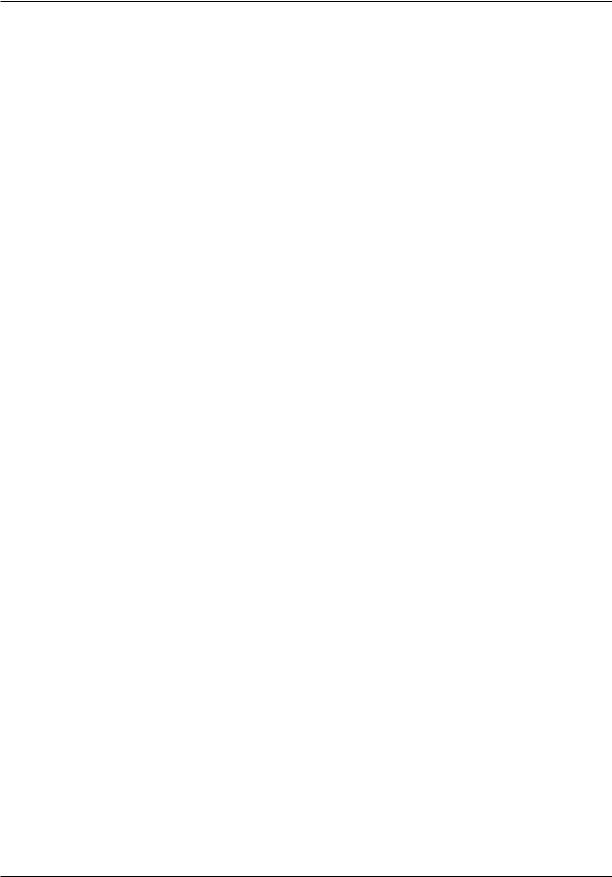
MUSIC
of music culture. The specific focuses and methods of these researchers vary widely, but together they show the resilience of local forms of musical expression in the context of the globalization of the media. See, for example, Wallis and Malm (1984), Nettl (1985, 1998), Hebdige (1987), Guilbault (1993), and Manuel (1993).
THE SOCIAL CONSTRUCTION OF
AESTHETICS
In line with the constructivist orientation just mentioned, a number of scholars have examined the processes by which aesthetic standards are set and changed. The seminal works in this line are by Meyer (1956) and Becker (1982). Hennion (1993) studies the evolution of standards in the Paris music world. Monson (1996) carefully examines the conventions of jazz improvisation. Ellison (1995) shows the close interplay between country music artists and their fans in defining the country music experience, and Frith (1996) examines many of the same issues in the world of rock music.
Alternative kinds of aesthetic standards have been contrasted. The most widely compared are ‘‘fine art,’’ ‘‘folk,’’ and ‘‘popular’’ aesthetics (Gans 1974). Using the example of jazz, Peterson (1972) shows that music can evolve from folk to pop and then to fine art. Frith (1996) perceptively shows that all three standards are used simultaneously in the rock world and elsewhere.
THE STUDY OF MUSIC WORLDS
In his early studies of interaction among dancehall musicians, Becker pioneered the study of the conventions that musicians use in making their kind of music together (summarized in Becker 1982). This line of activity has been continued in musical scenes varying from a group of coldly calculating recording session musicians (Peterson and White 1979; Faulkner 1983) to an impassioned gay community (DeChaine 1997). See also Zolberg (1980), Etzkorn (1982), Gilmore (1987), MacLeod (1993), Rose (1994), Monson (1996), Devereaux (1997), and Nettl (1998).
A related and often overlapping line of studies focuses on the socialization of performers. See, for example, Bennett (1980), Kingsbury (1988), Freeman (1996), DeChaine (1997), and Levine and
Levine (1996). The socialization of audiences has also been the focus of considerable attention. The focus of inquiry has included popular music fans (Hebdige 1979, Frith 1981, 1996), the Viennese supporters of Ludwig van Beethoven (DeNora 1995), country music fans (Ellison 1995), and hiphop fans (Rose 1995).
THE INSTITUTIONALIZATION OF
MUSIC FIELDS
While the art-world perspective focuses on the interaction of individuals and groups in making and appreciating music, research on institutionalization has to do with the creation of the organizations and the infrastructure that support distinctive music fields. DiMaggio (1982), for example, shows the entrepreneurial processes by which the classical music field was established in the United States. Menger (1983) and Hennion (1993) show the structure of the contemporary art music fields of France. Peterson (1990) and Ennis (1992) trace the reconfiguration of the pop music field into rock in the 1940s and 1950s, and Peterson (1997) traces the institutionalization of the country music field and its articulation with the larger field of commercial music in the mid-1950s.
Based on the production of culture perspective, some researchers have shown how elements of the production process, including law, technology, industry structure, industrial organization, careers, and market structures, profoundly shape the sort of music that is produced. The interplay between these processes is traced by Peterson (1990) to show why rock music emerged in the mid-1950s. For other examples of work in the production of culture perspective, see Keil (1966), Ryan (1985), Lopes (1992), Jones (1992), Frith (1993), Manuel (1993), Freeman (1996), and Levine and Levine (1996).
A number of studies have traced the development of specific music organizations and organization fields. Ahlquist (1997) shows the structure of the early-nineteenth-century entrepreneurial opera world, and Martorella (1982) shows the remarkably different field of opera that emerged following the American Civil War. Arian (1971) and Hart (1975) focus on the classical music orchestra. Peterson and White (1979) and Faulkner (1983) discuss the organization of the apparently
1925
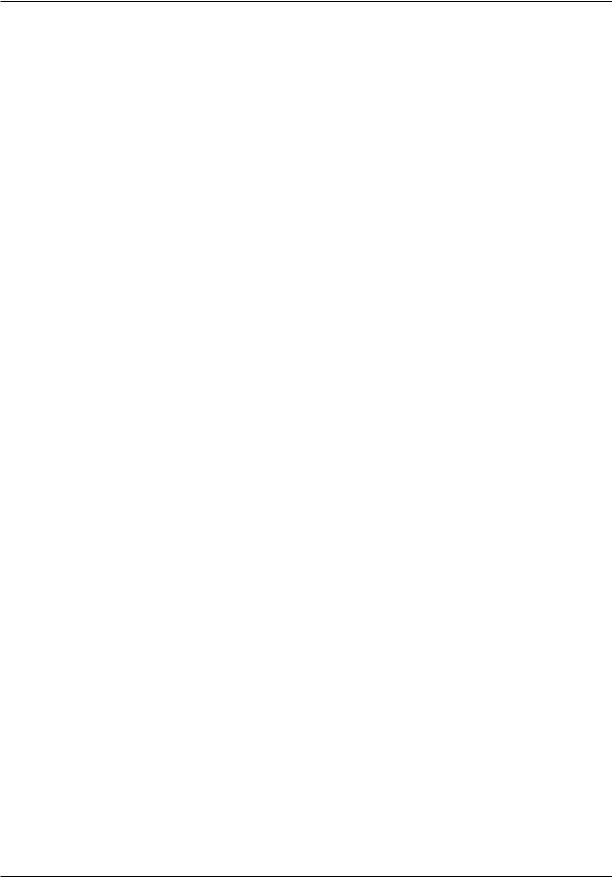
MUSIC
purely competitive world of recording studio session musicians. Gilmore (1987) focuses on three distinct contemporary classical music fields in New York City. MacLeod traces the field of club-date musicians in the same city. Guilbault (1993) shows the shaping of World Music in the West Indies. Negus (1999) compares the very different methods the major music industry corporations use in exploiting country music, rap, and reggae.
One specific line of studies focuses on the ‘‘concentration-diversity’’ hypothesis. Peterson and Berger (1975) show that periods of competition in the popular music field lead to a great diversity in the music that becomes popular, while concentration of the industry in a few firms leads to homogeneity in popular music. Using later data, Burnett (1990) challenges this finding, and Lopes (1992) shows how large multinational firms are able to control the industry while at the same time producing a wide array of musical styles.
MUSIC FOR MAKING DISTINCTIONS
All known societies employ music as a means of expressing identity and marking boundaries between groups (Lomax 1968), and a number of authors have shown the place of music in social class and status displays. DeNora (1995) shows the role that Beethoven’s music played in the Vienna of 1800 by marking off the rising business aristocracy from the older court-based aristocracy that championed the music of Haydn and Mozart. DiMaggio (1982) shows how the rising commercial elite of nineteenth-century Boston used support for classical music as a status marker, and recent survey research studies show how music preferences have been used in making social class distinctions. See, for example, Bryson (1996) and Peterson and Kern (1996).
Numerous studies have explored issues of racism in the meanings ascribed to music and its creators. Reidiger (1991), for example, shows how immigrant Irish, Germans, Jews, Swedes, and the like developed the ‘‘black-faced’’ minstrel show to satirize African-Americans and show their own affinity with the dominant English-speaking class as part of what they came to call the ‘‘white race.’’ Leonard (1962) and Peterson (1972) show the evolving attitudes of whites toward jazz and Afri- can-American culture. The most extensive line of work on race focuses on how jazz was created and
interpreted by African-American intellectuals as a form of resistance to the dominant white culture. See especially Jones (1963), Kofsky (1970), Vincent (1995), Devereax (1997), and Panish (1997). The racial shaping of blues, gospel, and rap have been explored, respectively, by Keil (1966), Heilbut (1997), and Rose (1994).
Music is gendered in many ways. Men are more likely to be producers, women consumers; women are often demeaned in the lyrics of rock, rap, and heavy metal, but they are characterized as strong in country music lyrics. Yet, compared to other topics, there has not been much research on gender in music and music making. Four different books suggest the range of topics that could be explored: McClary (1991) on the gendering of opera in which strong female leads do not succeed; Buffwack and Oermann (1993) on the decades of struggle of female country music entertainers for equality with men; Lewis (1990) on the gendered nature of rock videos; and Nehring (1997) on gender issues in pop music.
At least since the ‘‘jazz age’’ of the 1920s, music has been one of the primary ways in which generations are defined and define themselves. In the 1990s, for example, one slogan of the young was ‘‘If it’s ‘too loud,’ you’re too old.’’ Hebdige (1979), Frith (1981), Laing (1985), Liew (1993), Lahusen 1993, Epstein (1994), Weinstein (1994), Shevory (1995), and DeChaine (1997), among many others, show how specific contemporary youth groups use music to state distinctions not only between themselves and their parents but between themselves and other youth groups as well.
THE EFFECTS OF MUSIC
As just noted, new musical forms are identified with each new generation, and adults have tended to equate the music of young people with youthful deviance. Jazz, swing, rock, punk, disco, heavy metal, and rap, in turn, have been seen as the causes of juvenile delinquency, drug taking, and overt sexuality. See, for example, Leonard (1962), Hebdige (1977), and Laing (1985). Jazz was under attack for most of the first half of the twentieth century, and more recently heavy metal music has come in for the most sustained attention (Raschke 1990; Walser 1993; Weinstein 1994; Binder 1993; and Arnett 1996).
1926
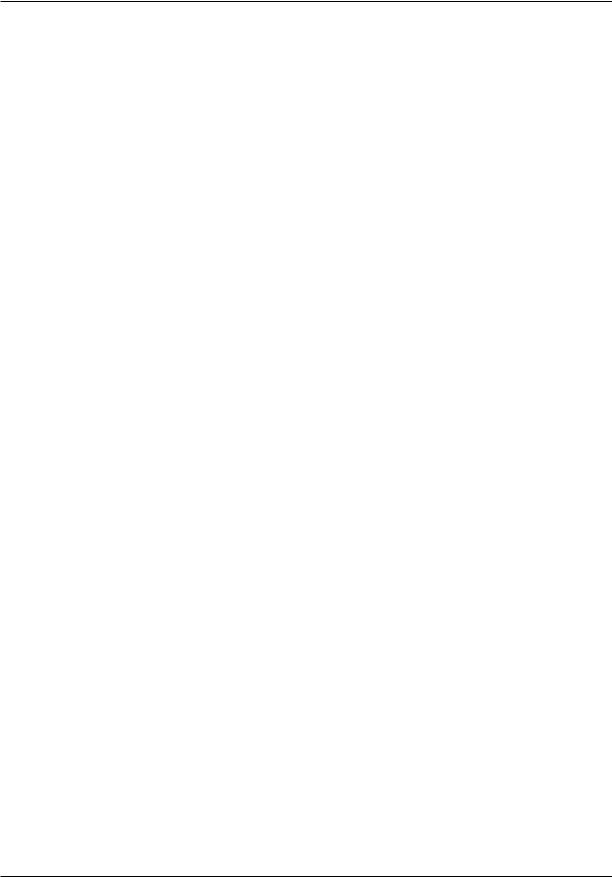
MUSIC
One of the most persistent research topics involving music has been the content analysis of song lyrics, on the often unwarranted assumption that one can tell the meaning a song has for its fans by simply interrogating lyrics (Frith 1996). Content analyses can be divided roughly into two types, holistic and systematic.
Systematic content analyses identify a universe of songs, draw a systematic sample from the universe, and analyze the lyrics in terms of a standard set of categories, typically using statistics to represent their results. Examples of systematic content analysis include Horton (1957), Carey (1969), Rogers (1989), and Ryan et al.(1996). Most such content analyses have focused on popular music, but one of the most remarkable is John H. Muller’s study of the changing repertoire of classical music over the first half of the twentieth century (1951)—a study that clearly deserves updating. Kate Muller (1973) makes a start. Holistic content analyses typically involve selecting songs that fit the research interest of their author and making judgments of the meaning of a song, or groups of songs, as a whole (Lewis 1990; McLurin 1992; Lahusen 1993; Ritzel 1998).
Finally the ‘‘effects’’ focus in social movements research returns the sociological study of music full circle to more macro-sociological concerns. Music has been an integral part of most social movements, as has been shown by a number of studies. For example, Denisoff and Peterson (1972) provide a collection of essays that describe the place of music in movements ranging from the International Workers of the World, Nazi youth groups, and folk protest music to avant-garde jazz, country music, rock, and religious fundamentalism. Denisoff (1971) has made an analysis of the use of music in the political protest movement of the 1960s and 1970s, and Neil Rosenberg (1993) has assembled a set of essays on the politicization of folk music in that same era. Treece (1997) describes the role of bossa nova in Brazil’s popular protest movement, and Eyerman and Jamison (1998) examine the place of music in more recent social movements.
Something approaching one-half the works cited here are by scholars who research music topics relevant to sociology, but who are not themselves sociologists. This openness and diversity is
important to the continuing vitality of the sociology of music. It is to be hoped that, in forthcoming decades, self-conscious efforts to bring more order to the scatter will facilitate the systematic articulation of research questions, stimulate research on more diverse music worlds, and encourage more cumulation of research findings. These efforts may also show the ways in which music can become a fundamental perspective in sociology similar to socialization, organization, deviance, culture, and the like.
REFERENCES
Ahlquist, Karen 1997 Democracy in the Opera: Music, Theater, and Culture in New York City, 1915–69. Urbana: University of Illinois Press.
Arian, Edward 1971 Bach, Beethoven and Bureaucracy. University: University of Alabama Press.
Arnett, Jeffrey 1996 Metal Heads: Heavy Metal Music and Adolescent Alienation. Boulder, Colo: Westview.
Atali, Jacques 1985 Noise: The Political Economy of Sound. Minneapolis: University of Minnesota Press.
Becker, Howard S. 1982 Art Worlds. Berkeley: University
of California Press.
Bennett, Stit 1980 Becoming a Rock Musician. Amherst: University of Massachusetts Press.
Binder, Amy 1993 ‘‘Media Depictions of Harm in Heavy Metal and Rap Music.’’ American Sociological Review
58:753–767.
Bryson, Bethany 1996 ‘‘’Anything but Heavy Metal’: Symbolic Exclusion and Musical Dislikes.’’ American Sociological Review 61:884–899.
Bufwack, Mary A., and Robert K. Oermann 1993 Finding Her Voice: The Saga of Women in Country Music. New York: Crown.
Burnett, Robert 1990 Concentration and Diversity in the International Phonogram Industry. Gothenburg, Sweden: University of Gothenburg Press.
Carey, James T. 1969 ‘‘Changing Courtship Patterns in Popular Song.’’ American Journal of Sociology 74:720–731.
Cerulo, Karen 1999 Identity Designs: The Sights and Sounds of a Nation. New Brunswick, N.H.: University Press of New England.
Cloonan, Martin 1997 ‘‘State of the Nation: ‘Englishness,’ Pop and Politics in the Mid-1990s.’’ Popular Music and Society 21(2):47–70.
DeChaine, D. Robert 1997 ‘‘Mapping Subversion: Queercore Music’s Playful Discourse of Resistance.’’
Popular Music and Society 21(1):7–38.
1927