
- •Abstract
- •Keywords
- •Contents
- •Introduction
- •Background on Array Processing
- •2.1 INTRODUCTION
- •2.1.1 Propagation Delays in Uniform Linear Arrays
- •2.1.2 Narrowband Approximation
- •2.1.3 Matrix Equation for Array Data
- •2.1.4 Eigenstructure of the Spatial Covariance Matrix
- •2.2 ANTENNA BEAMFORMING BASICS
- •2.2.1 The Conventional Beamformer
- •2.2.2 The Minimum Variance Distortionless Response Beamformer
- •3.1 CLASSICAL METHODS FOR DIRECTION OF ARRIVAL ESTIMATION
- •3.1.1 Delay-and-Sum Method
- •3.1.2 Capon’s Minimum Variance Distortionless Response Method
- •3.2 SUBSPACE METHODS FOR DOA ESTIMATION
- •3.2.1 Multiple Signal Classification Algorithm
- •3.2.2 Orthogonal Vector Methods
- •3.2.3 The Root MUSIC Algorithm
- •3.2.4 The Minimum Norm Method
- •3.2.5 Estimation of Signal Parameters via Rotational Invariance Techniques
- •3.2.6 Linear Prediction
- •3.2.7 The Unitary ESPRIT for Linear Arrays
- •3.2.8 QR ESPRIT
- •3.2.9 Beamspace DOA Estimation
- •3.2.10 The DFT Beamspace ESPRIT
- •3.2.11 The Multiple Invariance ESPRIT
- •3.2.12 Unitary ESPRIT for Planar Arrays
- •3.2.13 Maximum Likelihood Methods
- •3.2.13.1 The Alternating Projection Algorithm for ML DOA Estimation
- •4.1 ADAPTIVE SIMULATION EXAMPLE
- •Appendix
- •Signal Generator
- •The MUSIC Algorithm
- •The ESPRIT Algorithm
- •MVDR Method and the Classical Beamformer
- •Code to Simulate the MUSIC, the ESPRIT, the MVDR, the Min-Norm, and the Classical DOA Algorithms
- •References
- •Additional References
- •List of Symbols
- •List of Acronyms
- •Author Biography
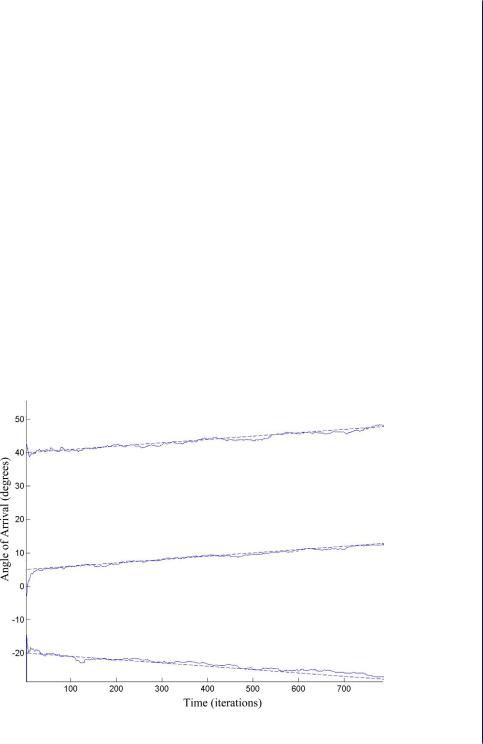
Adaptive Direction of Arrival Estimation 53
Next, the estimate of σ 2(n) is updated from the new eigendecomposition of Rxx(n) given in (4.9), where the diagonal elements of S(n) are σ 1(n) ,σ 2(n) , …, σr + 1(n) and have been placed in descending order. The N – r noise eigenvalues are σr2 + 1(n), ασ 2(n – 1), ασ 2(n – 1), …, ασ 2(n – 1), where ασ 2(n - 1) is listed N – r – 1 times. Taking an average of these values gives
σ 2 |
1 |
N − r − |
1 |
(n − 1) . |
|
(n) = ——σr2+1 |
(n) + α ———— σ 2 |
(4.12) |
|||
|
N − r |
N − r |
|
|
|
Karasalo’s method is an SVD updating algorithm and is often used as a reference method for comparing other subspace tracking algorithms. Although it is a good reference method, Karasalo’s algorithm is not often used in certain practical applications because the computation of the (r + 1) × (r + 2) SVD at each iteration is itself an iterative process. Other similar algorithms exist that replace the (r + 1) × (r + 2) SVD with a more efficient adaptive method [27, 28].
4.1ADAPTIVE SIMULATION EXAMPLE
In this simulation, there are three signal sources moving in space with time. Their DOAs are changing by 0.01° per iteration. The subspace tracking algorithm in Table 4.1 is used to track the signal subspace. The DOAs are recomputed after each iteration using the estimate of the signal subspace, Qs, and the ESPRIT algorithm given in (3.31)–(3.33a). The dotted line in Figure 4.1 represents the true DOA and the solid line represents the estimate of the DOA by the adaptive algorithm.
Figure 4.1: Adaptive DOA simulation using Karasalo’s subspace tracker and the ESPRIT algorithm.