
- •Table of Contents
- •EViews 5.1 Update Overview
- •Overview of EViews 5.1 New Features
- •Chapter 1. EViews 5.1 Enhanced Graph Customization
- •Basic Graph Characteristics
- •Adding and Editing Text
- •Updated Graph Command Summary
- •Chapter 2. EViews 5.1 Workfile Page Creation Tools
- •Creating a New Page Using Identifiers
- •Updated Workfile Page Command Summary
- •Chapter 3. EViews 5.1 Panel and Pool Testing
- •Omitted Variables Test
- •Redundant Variables Test
- •Fixed Effects Testing
- •Hausman Test for Correlated Random Effects
- •Updated Panel and Pool Command Summary
- •Chapter 4. EViews 5.1 EcoWin Database Support
- •Interactive Graphical Interface
- •Tips for Working with EcoWin Databases
- •Updated EcoWin Command Summary
- •Chapter 5. EViews 5.1 Miscellaneous Features
- •Enhanced Copy Command
- •Equation Forecast Coefficient Uncertainty
- •Additional GARCH Output
- •Global Default for Maximum Number of Errors
- •Chapter 6. EViews 5.1 Command Reference Update Summary
- •addtext
- •area
- •axis
- •copy
- •dbopen
- •draw
- •drawdefault
- •errbar
- •fixedtest
- •forecast
- •garch
- •hilo
- •legend
- •line
- •linkto
- •makegarch
- •makemap
- •pagecreate
- •options
- •ranhaus
- •scat
- •setelem
- •spike
- •template
- •testadd
- •testdrop
- •textdefault
- •xyline
- •xypair
- •Index
- •area 45
- •Axis
- •Bar graph 49
- •Conditional variance
- •Coordinates
- •Copy
- •Create
- •workfile page 84
- •Database
- •Drag(ging)
- •Error bar graph 63
- •EViews Enterprise Edition 31
- •Fixed effects
- •Font options
- •Forecast
- •Frequency conversion 51
- •GARCH
- •Graph
- •border 5
- •color settings 5
- •modifying 5
- •place text in 8, 42, 107
- •scatterplot graph 94
- •Legend
- •line 76
- •makegarch 83
- •Open
- •Page
- •Pie graph 91
- •Random effects
- •Test
- •Workfile
- •create page in 84
- •xypair 114
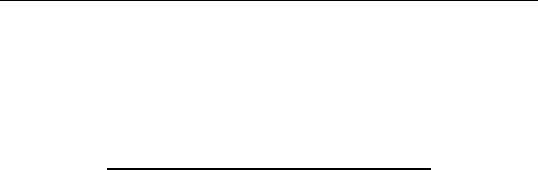
Fixed Effects Testing—53
Test Equation:
Dependent Variable: LSCRAP
Method: Panel EGLS (Cross-section random effects)
Date: 11/24/04 Time: 11:31
Sample: 1987 1989
Cross-sections included: 54
Total panel (balanced) observations: 162
Use pre-specified random component estimates
Swamy and Arora estimator of component variances
Variable |
Coefficient |
Std. Error |
t-Statistic |
Prob. |
|
|
|
|
|
|
|
|
|
|
C |
0.419327 |
0.073162 |
5.731525 |
0.0000 |
D88 |
-0.168993 |
0.095791 |
-1.764187 |
0.0796 |
D89 |
-0.442265 |
0.095791 |
-4.616981 |
0.0000 |
UNION |
0.534321 |
0.082957 |
6.440911 |
0.0000 |
|
|
|
|
|
|
|
|
|
|
|
Effects Specification |
|
|
|
|
|
|
S.D. |
Rho |
|
|
|
|
|
|
|
|
|
|
Cross-section random |
|
|
1.390029 |
0.8863 |
Idiosyncratic random |
|
|
0.497744 |
0.1137 |
|
|
|
|
|
|
|
|
|
|
The first thing to note is that the restricted specification removes the test variables GRANT and GRANT_1. Note further that the output indicates that we are using existing estimates of the random component variances (“Use pre-specified random component estimates”), and that the displayed results for the effects match those for the unrestricted specification.
Fixed Effects Testing
EViews 5.1 provides built-in tools for testing the joint significance of the fixed effects estimates in least squares specifications. To test the significance of your effects you must first estimate the unrestricted specification that includes the effects of interest. Next, select
View/Fixed/Random Effects Testing/Redundant Fixed Effects – Likelihood Ratio. EViews will estimate the appropriate restricted specifications, and will display the test output as well as the results for the restricted specifications.
Note that where the unrestricted specification is a two-way fixed effects estimator, EViews will test the joint significance of all of the effects as well as the joint significance of the cross-section effects and the period effects separately.
Let us consider Example 3.6.2 in Baltagi (2001), in which we estimate a two-way fixed effects model. The results for the unrestricted estimated gasoline demand equation are given by:
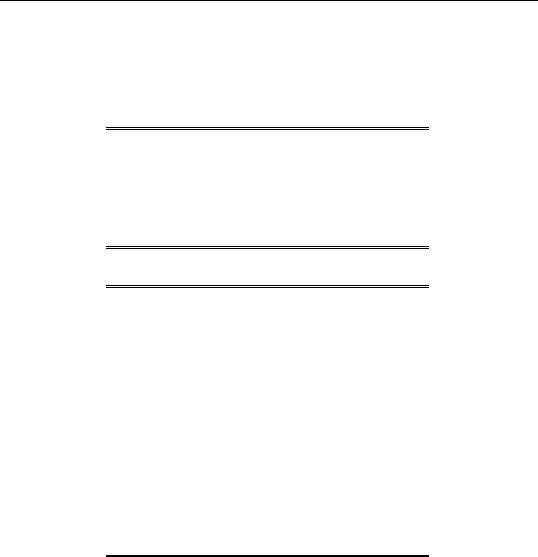
54—Chapter 3. EViews 5.1 Panel and Pool Testing
Dependent Variable: LGASPCAR
Method: Panel Least Squares
Date: 11/24/04 Time: 11:57
Sample: 1960 1978
Cross-sections included: 18
Total panel (balanced) observations: 342
Variable |
Coefficient |
Std. Error |
t-Statistic |
Prob. |
|
|
|
|
|
|
|
|
|
|
C |
-0.855103 |
0.385169 |
-2.220073 |
0.0272 |
LINCOMEP |
0.051369 |
0.091386 |
0.562103 |
0.5745 |
LRPMG |
-0.192850 |
0.042860 |
-4.499545 |
0.0000 |
LCARPCAP |
-0.593448 |
0.027669 |
-21.44787 |
0.0000 |
|
|
|
|
|
|
|
|
|
|
Effects Specification
Cross-section fixed (dummy variables)
Period fixed (dummy variables)
R-squared |
0.980564 |
Mean dependent var |
4.296242 |
Adjusted R-squared |
0.978126 |
S.D. dependent var |
0.548907 |
S.E. of regression |
0.081183 |
Akaike info criterion |
-2.077237 |
Sum squared resid |
1.996961 |
Schwarz criterion |
-1.639934 |
Log likelihood |
394.2075 |
F-statistic |
402.2697 |
Durbin-Watson stat |
0.348394 |
Prob(F-statistic) |
0.000000 |
|
|
|
|
|
|
|
|
Note that the specification has both cross-section and period fixed effects. When you select the fixed effect test from the equation menu, EViews estimates three restricted specifications: one with period fixed effects only, one with cross-section fixed effects only, and one with only a common intercept. The test results are displayed at the top of the results window:
Redundant Fixed Effects Tests
Equation: Untitled
Test cross-section and period fixed effects
Effects Test |
Statistic |
d.f. |
Prob. |
|
|
|
|
|
|
|
|
Cross-section F |
113.351303 |
(17,303) |
0.0000 |
Cross-section Chi-square |
682.635958 |
17 |
0.0000 |
Period F |
6.233849 |
(18,303) |
0.0000 |
Period Chi-square |
107.747064 |
18 |
0.0000 |
Cross-Section/Period F |
55.955615 |
(35,303) |
0.0000 |
Cross-Section/Period Chi-square |
687.429282 |
35 |
0.0000 |
|
|
|
|
|
|
|
|

Hausman Test for Correlated Random Effects—55
Notice that there are three sets of tests. The first set consists of two tests that evaluate the joint significance of the cross-section effects using sums-of-squares (F-test) and the likelihod function (Chi-square test). The corresponding restricted specification is one in which there are period effects only. The two statistic values (113.35 and 682.64) and the associated p-values strongly reject the null that the effects are redundant.
The remaining results evaluate the joint significance of the period effects, and of all of the effects, respectively. All of the results suggest that the corresponding effects are statistically significant.
Below the test statistic results, EViews displays the results for the test equations. In this example, there are three distinct restricted equations so EViews shows three sets of estimates.
Lastly, note that this test statistic is not currently available for instrumental variables and GMM specifications.
Hausman Test for Correlated Random Effects
A central assumption in random effects estimation is the assumption that the random effects are uncorrelated with the explanatory variables. One common method for testing this assumption is to employ a Hausman (1978) test to compare the fixed and random effects estimates of coefficients (for discussion see, for example Wooldridge (2002, p. 288), and Baltagi (2001, p. 65)).
To perform the Hausman test, you must first estimate a model with your random effects specification. Next, select View/Fixed/Random Effects Testing/Correlated Random Effects - Hausman Test. EViews will automatically estimate the corresponding fixed effects specifications, compute the test statistics, and display the results and auxiliary equations.
For example, Baltagi (2001) considers an example of Hausman testing (Example 1, p. 69), in which the results for a Swamy-Arora random effects estimator for the Grunfeld data are compared with those obtained from the corresponding fixed effects estimator. To perform this test in EViews 5.1, we first estimate the random effects estimator, obtaining the results:
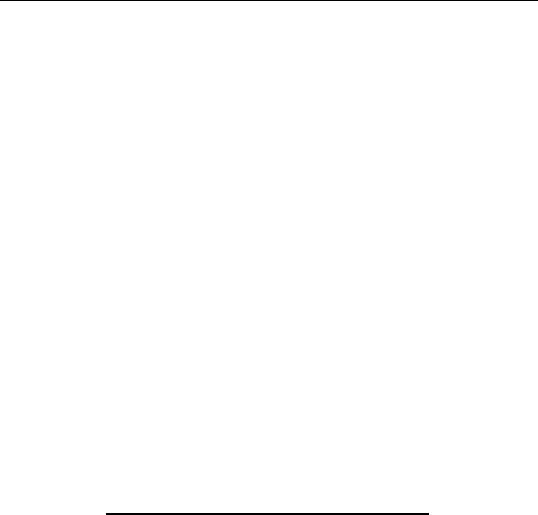
56—Chapter 3. EViews 5.1 Panel and Pool Testing
Dependent Variable: I |
|
|
|
|
Method: Panel EGLS (Cross-section random effects) |
|
|
||
Date: 11/24/04 |
Time: 12:45 |
|
|
|
Sample: 1935 1954 |
|
|
|
|
Cross-sections included: 10 |
|
|
|
|
Total panel (balanced) observations: 200 |
|
|
|
|
Swamy and Arora estimator of component variances |
|
|
||
|
|
|
|
|
|
|
|
|
|
Variable |
Coefficient |
Std. Error |
t-Statistic |
Prob. |
|
|
|
|
|
|
|
|
|
|
C |
-57.83441 |
28.88930 |
-2.001932 |
0.0467 |
F |
0.109781 |
0.010489 |
10.46615 |
0.0000 |
K |
0.308113 |
0.017175 |
17.93989 |
0.0000 |
|
|
|
|
|
|
|
|
|
|
|
Effects Specification |
|
|
|
|
|
|
S.D. |
Rho |
|
|
|
|
|
|
|
|
|
|
Cross-section random |
|
84.20095 |
0.7180 |
|
Idiosyncratic random |
|
52.76797 |
0.2820 |
|
|
|
|
|
|
|
|
|
|
|
Next we select the Hausman test from the equation menu by clicking on View/Fixed/Random Effects Testing/Hausman Test of Random vs. Fixed. EViews estimates the corresponding fixed effects estimator, evaluates the test, and displays the results in the equation window. If the original specification is a two-way random effects model, EViews will test the two sets of effects separately as well as jointly.
There are three parts to the output. The top portion describes the test statistic and provides a summary of the results. Here we have:
Hausman Specification Test (Random vs. Fixed Effects)
Equation: EQ263
Test for correlated cross-section random effects
|
Chi-Sq. |
|
|
Test Summary |
Statistic |
Chi-Sq. d.f. |
Prob. |
|
|
|
|
|
|
|
|
Cross-section random |
2.131366 |
2 |
0.3445 |
|
|
|
|
|
|
|
|
The statistic provide little evidence against the null hypothesis that there is no misspecification.
The next portion of output provides additional test detail, showing the coefficient estimates from both the random and fixed effects estimators, along with the variance of the difference and associated p-values for the hypothesis that there is no difference. Note that in some cases, the estimated variances can be negative so that the probabilities cannot be computed.