
Modern Banking
.pdf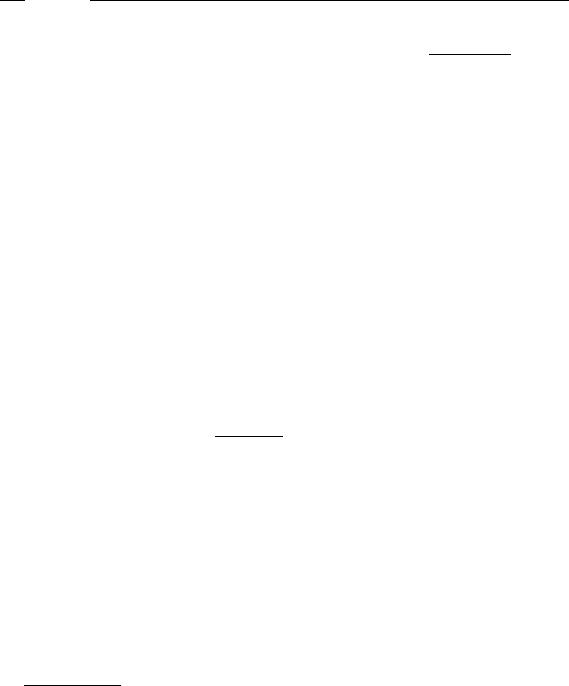
[ 148 ]
M O D E R N B A N K I N G
parametric distribution, usually a multivariate normal distribution. It is a partial valuation model because it can only account for linear dependencies (deltas) and ignores non-linear factors, for example, bond convexities or option gammas. This is why it is sometimes called the correlation or ‘‘delta-var’’ variation.
If this frequency distribution is chosen, then VaR is estimated using an equation which specifies portfolio risk as a linear combination of parameters, such as volatility or correlation. It provides an accurate VaR measure if the underlying portfolio is largely linear (e.g. traditional assets and linear derivatives), but is less accurate if non-linear derivatives are present.
Banks that use variance – covariance analysis normally make some allowances for nonlinearities. The Basel Amendment requires that non-linearities arising from option positions be taken into account.
In approaches (a) and (b), a data window must be specified, that is, how far back the historical distribution will go. The Basel Committee requires at least a year’s worth of data. Generally, the longer the data run, the better, but often data do not exist except for a few countries, and it is more likely the distribution will change over the sample period. In approach (b), there is the question of which variances–covariances of the risk factor returns are computed.
(c) Monte Carlo35 approach. Another full valuation approach, involving multiple simulations using random numbers to generate a distribution of returns. Distributional assumptions on the risk factors (e.g. commodity prices, interest rates, equity prices or currency rates) are imposed – these can be normal or other distributions. If a parametric approach is taken, the parameters of the distributions are estimated, then thousands of simulations are run, which produce different outcomes depending on the distributions used. The non-parametric approach uses bootstrapping, where the random realisations of the risk factor returns are obtained through iterations of the historical returns. In either approach, pricing methodology is used to calculate the value of a portfolio.
Unlike (a) and (b), the number of portfolio return realisations is much greater in number, from which the VaR estimates are derived. The Monte Carlo approach is usually rejected because it involves a large number of computations, which present practical problems if traders are computing VaR once, or several times a day. Computation costs are high, too.
3.5.4. VaR, Portfolios and Market Risk
It is possible to show simple applications of VaR for individual trading positions involving two currencies or equities. However, banks compute VaR for large portfolios of equities, bonds, currencies and commodities. Management will want an aggregate number showing the potential value at risk for the bank’s entire trading position. This aggregate VaR is not just a simple sum of the individual positions because they can be positively or negatively correlated with each other, which will raise or reduce the overall VaR. The components of
35 Generally, a Monte Carlo approach models different cash flows of a particular deal, and subjects them to thousands of simulations involving different scenarios to generate the risk parameters. For example, if a bank agrees a loan for a particular venture, detailed cash flow models are subjected to thousands of simulations to assess how changes in the economy will affect the probability of default, exposure at default and loss given default.
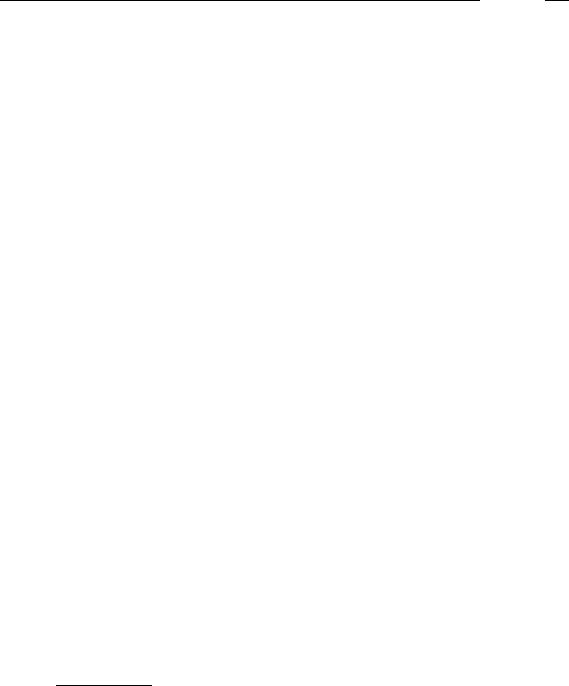
[ 149 ]
M A N A G E M E N T O F R I S K S I N B A N K I N G
any portfolio are sensitive to certain fundamental risks, the so-called ‘‘Greeks’’. These are as follows.
Delta or Absolute Price Risk: the risk that the price of the underlying asset will change (e.g. the stock or commodity price, exchange rate or interest rate). The delta risk is the effect of a change in the value of an underlying instrument on the value of the portfolio.
Gamma or Convexity Risk: the rate of change in the delta itself, or the change in the delta for a one point move in the underlying price. It allows for situations where there is a non-linear relationship between the price of the underlying instrument and the value of the portfolio.
Vega or Volatility Risk:36 this risk applies when an option is involved, or a product has characteristics similar to an option. It is the sensitivity of the option price for a given change in the value of volatility. An increase in volatility of the underlying asset makes the option more valuable. Therefore, if the market’s view of the volatility of the underlying instrument changes, so too will the value of the option.
Rho or Discount Risk: this risk applies primarily to derivatives, or any product which is valued using a discount rate, i.e. the value is determined by discounting expected future cash flows at a risk-free rate. If the risk-free rate changes, so too does the value of the derivative.
Theta or Time Decay Risk: the time value of the option. A change in the value of a portfolio because of the passage of time. For example, in an option theta rises with the length of time to the strike price.
To arrive at a VaR, the components of the portfolio are disaggregated according to the above risk factors (if they apply), netted out, then aggregated together.
Suppose a bank computes daily earnings at risk for its foreign exchange, bond and equity positions. Then it will end up with an interest DEAR, a foreign exchange DEAR and an equity DEAR. These will be summarised on a spread sheet, and if the bank operates in more than one country, their respective DEARs are reported too. Assume the bank is headquartered in Canada but also operates in the USA and the UK. Then a simplified version of the spread sheet will look like in Table 3.7. The interest rate column is highly simplified, for ease of exposition. Normally the interest rate risk would appear for a number of time buckets, with a column for each bucket. ‘‘Portfolio effects’’ is another name for benefits arising from diversification, which will depend on the degree to which various markets and assets are correlated with each other. There are two to account for. The first is the diversification effect arising from having a portfolio of currency, bonds and equity in one country. The other allows for the effects of holding bonds, foreign exchange and equity in more than one country. The portfolio/diversification effects will be calculated in a separate matrix and depend on numerous intercorrelations. In the table, it has been assumed that the diversification effects allow a total of $30 million to be reduced from the summed DEAR, giving a total DEAR of $45 million.
To show how VaR is reported by banks, the figures from Merrill Lynch’s Annual Report are provided. Merrill’s differentiates between trading and non-trading VaR, as can be seen from
36 As was noted in Heffernan (1996), vega is NOT a Greek letter, and a plea was made for a replacement. Unfortunately, as was feared, vega is now accepted as a Greek letter! Even prestigious researchers of the Bank of England have to include vega in ‘‘the Greeks’’, from which this excellent description of ‘‘the Greeks’’ is drawn. See Gray and Place (1999).

[ 150 ]
M O D E R N B A N K I N G
Table 3.7 A Hypothetical Daily Earnings at Risk for a Canadian bank (CDN$m)
Country |
Interest rate |
Forex Risk |
Equity Risk |
Total |
|
Risk DEAR |
DEAR |
DEAR |
|
Canada |
20 |
|
10 |
30 |
USA |
5 |
10 |
10 |
25 |
UK |
5 |
5 |
10 |
20 |
Total |
20 |
5 |
30 |
75 |
Gross Portfolio Effect |
|
|
|
−30 |
Total DEAR |
|
|
|
45 |
DEAR: Daily Earnings at Risk
Table 3.8 Merrill Lynch: value at risk ($m)
|
2001 |
2000 |
Daily/quarterly |
|
|
|
Average 2001 |
Trading VaR of which: |
256 |
215 |
194 |
Interest rate & credit spread |
113 |
81 |
64 |
Equity |
94 |
77 |
61 |
Commodity |
2 |
9 |
3 |
Currency |
3 |
14 |
11 |
Volatility |
44 |
34 |
35 |
Diversification benefit |
(144) |
(116) |
(92) |
Firm-Wide Trading VAR |
112 |
90 |
102 |
Non-Trading VaR of which: |
165 |
140 |
155 |
Interest rate & credit spread |
77 |
67 |
76 |
Currency |
20 |
23 |
19 |
Equity |
57 |
47 |
51 |
Volatility |
11 |
3 |
9 |
Diversification benefit |
(59) |
(44) |
(45) |
Firm-Wide Non-Trading VaR |
106 |
96 |
110 |
Overall VaR is based on a 99% confidence interval and 2-week holding period.VaR for non-trading instruments excludes US banks.
Daily average figures for traded VaR; quarterly average figures for non-traded VaR.
Diversification benefit: the difference between aggregate (firm-wide) VaR and the VaR summed from the four risk categories. The difference arises because the four market risk categories are not perfectly correlated. For example, the simulations of losses at a 99% confidence interval show the losses from each category will occur on different days. There are similar benefits within each category.
( ): negative.
Source: Merrill Lynch (2002), Annual Report, pp. 34–35.
Table 3.8. The daily trading VaR for 2001 is $256 million, which says, with an assumed volatility of $44 million, that average trading losses could exceed $256 million in 1 out of every 100 trading days. Had the confidence interval been 95%, average trading losses could exceed $256 million in 5 out of every 100 trading days. Again, the actual size of the losses on these 5 days is unknown, and could be much higher than $256 million. Table 3.8
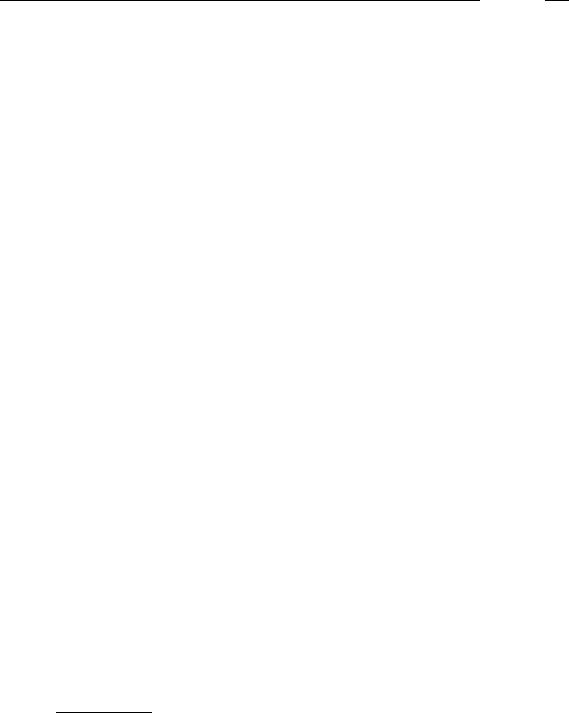
[ 151 ]
M A N A G E M E N T O F R I S K S I N B A N K I N G
shows that both trading and non-trading VaR at Merrill Lynch rose between 2000 and 2001 because (according to the Annual Report, 2002, p. 35) of increases in interest, equity and credit spread risk VaR. The figure would have been higher but for a partial offset due to the diversification effect. Commodity trading VaR also increased more than fourfold between the two years.
3.5.5. Problems with the VaR Approach
Danielsonn (2000, 2002) has been one of the most vociferous critics of value at risk, to be discussed below. Other authors37 have voiced similar concerns.
The first problem with VaR is that it does not give the precise amount that will be lost. For example, if a bank reports VaR ≥ $1 million at the 99th percentile, it means that losses in excess of VaR would be expected to occur 1% of the time. However, it gives no indication as to how much VaR will be exceeded – it could be $2.5 million, $450 million or $1 billion – there is no upper bound on what can be lost. Statistically, rather than giving the entire tail, it is giving an arbitrary point in the tail.
Second, the simpler VaR models depend on the assumption that financial returns are normally distributed and uncorrelated. Empirical studies have shown that these assumptions may not hold, contributing to an inaccurate VaR measure of market risk.
Anecdotal evidence and remarks from traders suggest it is also possible to manipulate VaR by up to a factor of five. A trader might be told to lower VaR because it is too high. By lowering VaR the bank can increase the amount of risk, and expected profit.
VaR does not give a probability of bank failure, only losses that arise from a bank’s exposure to market risk. On the other hand, it was never meant to. It is only a measure of the bank’s exposure, reflecting the increased trading activities of many banks.
If all traders are employing roughly the same model, then the measure designed to contain market risk creates liquidity risk. This point was illustrated by Dunbar (2000), commenting on the 1998 Russian crisis. Market risk had been modelled using VaR, based on a period of relatively stable data, because for the previous five years (with the exception of the Asian crisis, which was largely confined to the Far East) volatility on the relevant markets had been low. Financial institutions, conforming to regulations, employed roughly the same market risk models. The default by Russia on its external loans caused the prices of some assets to become quite volatile, which breached the risk limits set by VaR-type models. There was a flight from volatile to stable assets, which exaggerated the downward price spiral, resulting in reduced liquidity. Hence if all banks employ a similar VaR, it can actually escalate the crisis.
The above example also illustrates that statistical relationships applied to VaR which hold during a period of relative stability often break down and cannot be used during a crisis. While there may be little in the way of correlation between asset prices in periods of stability, in a crisis, all asset values tend to move together. This means any portfolio/diversification effects will disappear.
37 See Taleb (1996, 1997), an experienced trader with an MBA and a PhD, is also highly critical of the use of VaR. For an alternative view, see Jorion (1997).
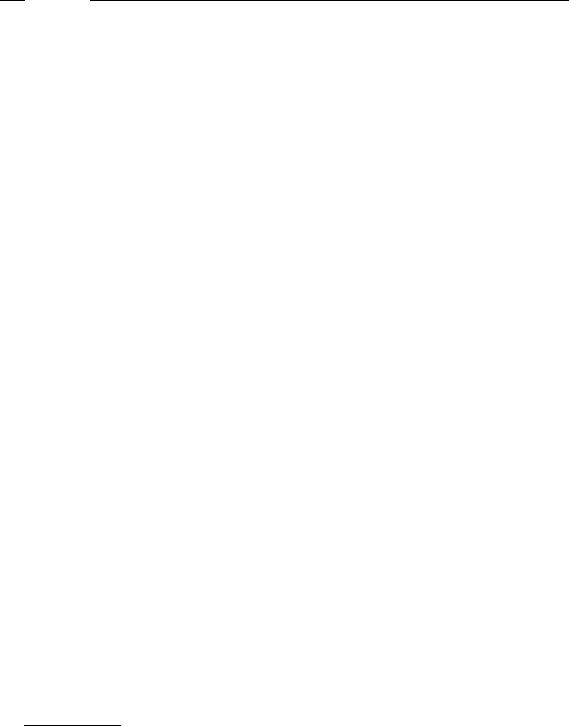
[ 152 ]
M O D E R N B A N K I N G
Variations in the model assumptions with respect to the holding period, confidence interval and data window will cause different risk estimates (Beder, 1995). Likewise, Danielsonn (2000) demonstrates the VaR models lack robustness, that is, the VaR forecasts across different assets are unreliable. To illustrate, Danielsonn employs a violation ratio. Violation is defined as the case where the realised loss is greater than the VaR forecast. The violation ratio is the ratio of realised number of VaR violations to the expected number of violations. If the V-ratio >1, the model is under-forecasting the risk; if V-ratio <1, it is over-forecasting. Put another way, over-forecasting means the model is thick tailed relative to the data; under-forecasting means the model is relatively thin tailed. Danielsonn reports disappointing results using this test. Different estimation methods produce different violation ratios, but all vary between, for example, 0.38 and 2.18 (using variations of Riskmetrics ).
For the above reasons, it is necessary to test the actual outcomes with the VaR predictions of losses. However, such tests also have a problem (Kupiec, 1995) because if the period over which the performance of the VaR model is relatively short,38 the tests lack statistical power. It is difficult to evaluate the accuracy of the model on the basis of a year of data. The choice of a 99% confidence interval allows for a loss to occur very 2.5 days in a year. Danielsonn (2000) argues such an allowance is irrelevant in a period of systemic crises, or even for the probability of a bank going bankrupt. If VaR violations occur more than 2.5 times per year under a 99% confidence interval, it does not usually indicate the bank is in any difficulty, and in light of this point, when are VaR breaches relevant? In defence of VaR, it was never meant to indicate that a bank was in difficulty. It is a benchmark number for banks to use to track their market risk exposure.
Both the parametric and non-parametric frequency distributions produce measures which rely on historical data, an implicit assumption is that they are a good predictor of future returns. But historical simulation is sensitive to the sampling period (Danielsonn and de Vries, 1997). For example, in an equity portfolio, the VaR outcomes will be quite different if the October 1987 crash is included than if it is excluded. Or, looking at US share price data from mid-1983 to mid-2000 would suggest sizeable index price falls were the exception, and if they happened, quickly reversed themselves. Agents armed with this information would think the future was like the past (a popular assumption in many models of VaR) and would have found the subsequent share price declines completely mystifying, and outside anything remotely predictable.
However, the non-parametric or historical simulation approaches are superior to the variance – covariance approach (advocated by Riskmetrics ) for two reasons. First, financial market returns do not always follow a normal distribution – large movements in the market (fat tails) occur more often than indicated by the normal distribution. Second, historical simulation allows for non-linearities between the position and risk factor returns, which are important when the VaR being computed includes derivatives, especially options.
There are other criticisms of the use of VaR which relate to the actual 1996 Basel Amendment and ‘‘Basel 2’’ agreement, but these will be discussed in Chapter 4. However,
38 For example, the 250 days specified by the Basel Amendment – see Chapter 4.
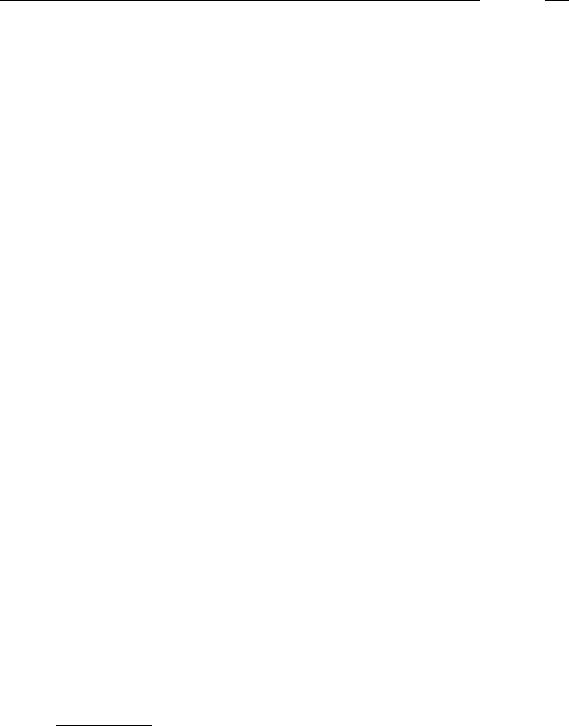
[ 153 ]
M A N A G E M E N T O F R I S K S I N B A N K I N G
to conclude this section, some quotes from Jorion (defending VaR) and Taleb (rejecting its use) are helpful.
First, comments in favour of VaR by Philippe Jorion:39
‘‘First, the purpose of VaR is not to describe the worst possible outcomes. It is simply to provide an estimate of the range of possible gains and losses. Many derivatives disasters have occurred because senior management did not inquire about the first-order magnitude of the bets being taken. Take the case of Orange County, for instance. There was no regulation that required the portfolio manager, Bob Citron, to report the risk of the $7.5 billion investment pool. As a result, Citron was able to make a big bet on interest rates that came to a head in December 1994, when the county declared bankruptcy and the portfolio was liquidated at a loss of $1.64 billion. Had a VaR requirement been imposed on Citron, he would have been forced to tell investors in the pool: Listen, I am implementing a triple-legged repo strategy that has brought you great returns so far. However, I have to tell you that the risk of the portfolio is such that, over the coming year, we could lose at least $1.1 billion in one case out of 20.’’ (Jorion, 1997, p. 1)
‘‘VaR has other benefits as well. By now, all U.S. commercial banks monitor the VaR of their trading portfolios on a daily basis. Suppose a portfolio VaR suddenly increases by 50 percent. This could happen for a variety of reasons – market volatility could have increased overnight, a trader could be taking inordinate risks, or a number of desks could be positioned on the same side of a looming news announcement. More prosaically, a position could have been entered erroneously. Any of these factors should be cause for further investigation, which can be performed by reverse-engineering the final VAR number. Without it, there is no way an institution can get an estimate of its overall risk profile.’’ (Jorion, 1997, p. 1)
‘‘Still, VaR must be complemented by stress-testing. This involves looking at the effect of extreme scenarios on the portfolio. This is particularly useful in situations of ‘dormant’ risks, such as fixed exchange rates, which are subject to devaluations. Stress-testing is much more subjective than VAR because it poorly accounts for correlations and depends heavily on the choice of scenarios. Nevertheless, I would advocate the use of both methods.’’ (Jorion, 1997, p. 2)
‘‘A second misconception raised in the discussion is that VaR involves a covariance matrix only and does not work with asymmetric payoffs. This is not necessarily the case. A symmetric, normal approximation may be appropriate for large portfolios, in which independent sources of risk, by the law of large numbers, tend to create normal distributions. But the delta-normal implementation is clearly not appropriate for portfolios with heavy option components, or exposed to few sources of risk, such as traders’ desks. Other implementations of VaR do allow asymmetric payoffs. VAR is an essential component of sound risk management systems. VaR gives an estimate of potential losses given market risks. In the end, the greatest benefit of VAR lies in the imposition of a structured methodology for critically thinking about risk. Institutions that go through the process of computing their VAR are forced to confront their
39 Comments from Jorion and Taleb (1997).

[ 154 ]
M O D E R N B A N K I N G
exposure to financial risks and to set up a proper risk management function. Thus the process of getting to VAR may be as important as the number itself. These desirable features explain the widespread view that the ‘quest for a benchmark may be over’.’’ (Jorion, 1997, p. 2)
Nassim Taleb opposes the use of VaR:40
‘‘. . . the professional risk managers I heard recommend a ‘guarded’ use of VaR on the grounds that it generally works or ‘it works on average’ do not share my definition of risk management. The risk management objective function is survival not profits and losses. One trader, according to Chicago legend, made $8 million in eight years and lost $80 million in eight minutes. According to the same standards, he would be ‘in general’ and ‘on average’ a good trader.’’ (Taleb, 1997, p. 3)
‘‘VaR has made us replace about 2500 years of market experience with a co-variance matrix that is still in its infancy.’’ (Taleb, 1997, p. 1)
‘‘[VaR can measure] the risks of common events, perhaps. Those that do not matter, but not the risks of rare events.’’ (Taleb, 1997, p. 2)
‘‘VaR players are all dynamic hedgers and need to revise their portfolios at different levels. As such they can make very uncorrelated markets become correlated. In 1993 hedge funds were long in seemingly independent markets. The first margin call in the bonds led them to liquidate their positions in the Italian, French, and German bond markets. Markets therefore became correlated.’’ (Taleb, 1997, pp. 2 – 3)
3.5.6. Stress Testing and Scenario Analysis to Complement VaR
Given the limitations of VaR, most banks apply scenario analysis and stress testing to complement estimates of market risk produced by VaR. Banks begin by identifying plausible unfavourable scenarios which cause extreme changes to the value of one or more of the four risk factors, i.e. interest, equity, currency or commodity prices. These might include an event which causes most financial agents to act in a similar manner, prompting severe illiquidity, as illustrated by the LTCM case, discussed earlier. Or the unexpected collapse of Enron and WorldCom (two American financial conglomerates in 2002), creating widespread fears about the quality and accuracy of company financial statements which, in turn, contributed to unexpected, dramatic declines in a key or several stock market prices. Other scenarios might be ill-founded rumours which prompt unexpected cash margin calls or changes in collateral obligations.
The stress test, based on a scenario, computes how much a bank’s portfolio could lose. Note the difference from VaR, which estimates the maximum amount that a portfolio, security or business unit (of the bank) could lose over a specified time period. In VaR, the third and fourth moments are assumed to be zero. Some forms of stress testing go beyond the
40 Comments from Taleb (1997) and Taleb and Jorion (1997).
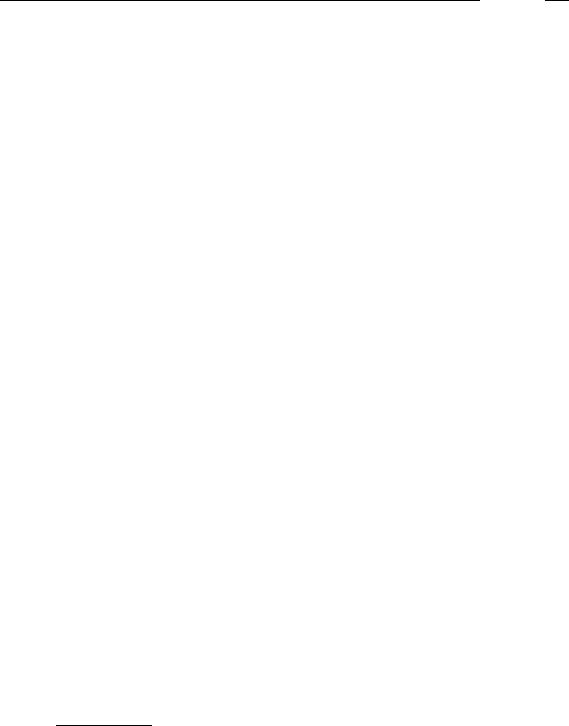
[ 155 ]
M A N A G E M E N T O F R I S K S I N B A N K I N G
second moment of the distribution (volatility) and consider the third (skewness) and fourth moment (kurtosis) of the distribution. Skewness will include the worst case outcomes. Kurtosis describes the relative thinness or fatness of the tails of a distribution compared to a normal distribution. For example, in a credit portfolio, if the loss distribution is leptokurtic (i.e. has fat tails), meaning extreme events are more likely than in a normal distribution (e.g. one large credit default results in massive losses). Thin (platykurtosis) tails suggests the opposite.
The size of the change in key risk factors will have to be computed. One group41 recommended changes in volatility such as currency changes of plus or minus 6%, or a 10% change in an equity index. The bank must also choose the frequency with which the stress tests should be conducted.
As a practical example, suppose there are two scenarios: (1) a 40% decline in UK and world equity prices or (2) a 15% decline in residential and commercial property prices. If these are the scenarios, the next step is to decide what stress tests should be conducted. Banks could be asked to identify the potential impact of (1) and (2) on market risk, credit risk and interest rate risk. In addition (or alternatively), building societies could be asked to compute the impact of (1) and (2) on the retail deposit rate, the mortgage rate and the income of building societies. The complexity of the stress tests that must be performed is immediately apparent. Complicated models are required if the banks or building societies are going to produce realistic answers to the questions.
A final task is to decide how to use the results. This presents a very difficult problem for the bank because, by definition, a bank cannot forecast ‘‘surprises’’ or unexpected events. Nor can it judge how frequently they will occur, and therefore, whether or not a special reserve should be created. Furthermore, if such a reserve is kept, how much should the bank be setting aside?
3.6. Management of Credit Risk
3.6.1. Background
Market risk has received an inordinate amount of attention in recent years but managing credit risk is the ‘‘bread and butter’’ of most commercial banks. Every commercial bank, by definition, has a loan portfolio. Increases in credit risk will raise the marginal cost of debt and equity, which in turn increases the cost of funds for the bank. Techniques for credit risk management are well known because the banking sector has had a long history of experience in this area. Nonetheless, loan quality problems are an important cause of bank failure, as will be seen in Chapter 6. For this reason, all bankers, not just those in a credit risk department, should be aware of the key factors affecting the quality of a loan portfolio, and the methods for managing it.
3.6.2. Credit Risk Decisions: Retail versus Corporate
If a bank is looking to minimise its aggregate credit risk, then good risk management of retail and corporate lending is essential. The approaches taken for retail and corporate loans
41 Derivative Policy Group (1995).

[ 156 ]
M O D E R N B A N K I N G
differ considerably, mainly because a corporation is able to produce a variety of financial ratios, which are not available when the suitability of an individual or a small firm for a loan is being assessed. Most bankers concede that lack of information makes retail lending more difficult than corporate lending. On the other hand, loans to corporates which turn out to be bad can be very serious for the bank because of the large sums involved. Countless cases abound: Maxwell and a number of London-based banks; Schroder and Deutsche Bank; and the collapse of Enron and WorldCom. The number of incidents where retail loan defaults have had serious consequences for a bank is very much lower, and usually occurs if a bank is over-exposed in one area such as mortgages, and property prices collapse at the same time as interest rates rise.
The principles used to model credit risk, and the methods used to minimise credit risk for the retail and corporate sectors, are discussed below.
3.6.3. Minimising Credit Risk
There are five key ways a bank can minimise credit risk: through accurate loan pricing, credit rationing, use of collateral, loan diversification and more recently through asset securitisation and/or the use of credit derivatives. The weights applied to each of the methods will vary, depending on whether the loan is commercial or retail.
(1) Pricing the loan: any bank will wish to ensure the ‘‘price’’ of a loan (loan rate) exceeds a risk adjusted rate, and includes any loan administration costs, that is:
RL = i + ip + fees |
(3.7) |
where:
RL: interest rate charged on the loan
i: market interest rate, such as LIBOR or an equivalent term42
ip: risk premium, negatively related to the probability of the loan being repaid (ip = 0 if repayment is certain)
In the above equation, ip and i are positively related, for one of two reasons. In the case of a variable rate loan, if the market rate rises, so will the interest rate charged on the loan, and the borrower will find it more difficult to repay the loan, so the probability of default increases. Or, at very high market rates, the loan rate will rise, attracting riskier borrowers (due to adverse selection), so the chances of the loan being repaid fall.
Other factors also influence the loan rate. If there is any collateral or security backing the loan, the rate charged should be lower than in the absence of security. In addition, there are non-price features: the bank may charge a high fee for arranging the loan but the interest rate will be lower. Or, some central banks impose reserve ratios, which means a percentage of a bank’s deposits is held at the central bank, often earning no interest. This is effectively a tax on deposits, which banks will try to make up by imposing higher loan rates.
42 The loan rate set will also depend on the term of the loan. Normally (but not always) the longer the term the higher the loan rate. This will be in the risk premium and will depend on the term structure of interest rates, which is often but not necessarily upward sloping.

[ 157 ]
M A N A G E M E N T O F R I S K S I N B A N K I N G
Thus, the loan rate should include a ‘‘market’’ rate, risk premium and administration costs. The riskier the borrower, the higher the premium. Should the risk profile of the loan be altered, the rate should change accordingly, though if increased, it should be borne in mind that the potential for adverse selection or adverse incentives is greater. The guidelines may also be difficult to implement in highly competitive markets.
(2)Credit Limits: another method for controlling credit risk. Given the potential for adverse selection, most banks do not rely solely on loan rates when taking a lending decision. Instead, the availability of a certain type of loan may be restricted to a selected class of borrowers, especially in retail markets. Branch managers are given well-defined credit constraints (and checklists – see below), and borrowers usually discover they may not borrow above some ceiling. In retail markets, banks normally quote one loan rate (or a very narrow range of rates) and then restrict the amount individuals or small firms can borrow according to criteria such as wealth or collateral. However, in the United States, legislation prevents banks from discriminating against certain retail customers. The Community Re-investment Act (1977) requires banks to provide evidence to the regulator that loan decisions do not discriminate against the local community. Under the Home Mortgage Disclosure Act (1975) regulators must be satisfied mortgage decisions by banks do not discriminate on the grounds of race, income, age or income status.
By contrast, in the wholesale markets, credit limits are of secondary importance; loan rates (and the risk premium) normally vary from business to business because banks have more information on the value of a firm, such as independent auditor reports on a company’s financial performance.
(3)Collateral or Security: Banks also use collateral to reduce credit risk exposure. However, if the price of the collateral (for example, houses, stock market prices) becomes more volatile, then for an unchanged loan rate, banks have to demand more collateral to offset the increased probability of loss on the credit. Another problem that can arise is if the price of collateral is negatively correlated with the ability of the borrower to repay, that is, as the probability of default among a borrower class increases, the price of the collateral declines. For example, in the 1980s, Texan banks made a large number of loans to firms in the booming oil industry, and the collateral was often the oil well(s) or Texan real estate. When oil prices collapsed, the value of the collateral also collapsed. In the late 1980s, over a quarter of US banks that failed were located in Texas.
In Britain, building societies and banks tend to enter into flexible rate mortgage agreements with homeowners, using the property as collateral. In the early 1990s, interest rates began to rise to counter inflation. The housing boom came to an abrupt end, and house prices fell rapidly. Householders who had borrowed up to 100% mortgages found themselves holding negative equity: the value of the house was less than the cost of the total outstanding mortgage. Many households were unable to make the mortgage repayments as recession set in and the unemployment rate rose. The banks/building societies realised they would end up having to dispose of real estate at very low prices. So many accommodated distressed borrowers, by allowing interest repayment holidays and other measures which meant increased arrears. Though costly, losses would have been far higher if houses were repossessed and sold. These examples illustrate an important point. Collateral tends to be a more effective means of managing risk for short-term (e.g. overnight loans) because the