
Mathematics for Life Sciences and Medicine - Takeuchi Iwasa and Sato
.pdf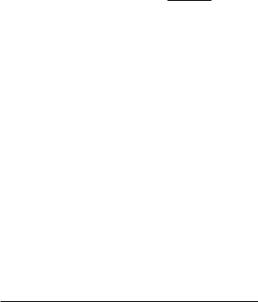
62 Yasuhiro Takeuchi and Wanbiao Ma
Theorem 13 (Ma and Takeuchi 2004). If (11) holds, then there exist a positive constant sequence h = hn(n = 0, 1, 2, . . .) such that (10) has a Hopf bifurcation from the positive equilibrium E+ at h = hn(n = 0, 1, 2, . . .).
Theorem 14 (Ma and Takeuchi 2004). The positive equilibrium E+ of (10) is locally asymptotically stable for 0 ≤ h < h0, and is unstable for h > h0.
It is clear that Theorems 10 and 12 give a completed analysis on the global asymptotic properties of the solutions (10) for b > µ1 and b ≥ µ3(µ2 + γ)/(µ3 + γ). For the case of µ1 = b < µ3(µ2 + γ)/(µ3 + γ), Theorem 11 shows that the disease ultimately tends to extinction. However, to give a more explicit estimation to the constant c in Theorem 11 is also an interesting problem. Theorem 14 gives a detailed analysis on the locally asymptotic properties of the positive equilibrium E+ of (10). However, analysis of global asymptotic properties (such as permanence and global asymptotic stability) of the positive equilibrium E+ of (10) remains to be still an important problem to be studied.
3.4 SIR epidemic models with time delays
and non-constant birth rate b(1 − β1N(t) )
1+N(t)
Recently, Song and Ma (2006) proposed the following delayed SIR epidemic model with density dependent birth rate:
S˙ (t) = −βS(t)I(t − h) − µ1S(t) + b 1 − β1 |
N (t) |
, |
|
1 + N (t) |
|
||
I˙(t) = βS(t)I(t − h) − µ2I(t) − γI(t) , |
|
(12) |
|
˙ |
|
|
|
R(t) = γI(t) − µ3R(t) , |
|
|
|
where S(t), I(t), R(t), N (t), µ1, µ2, µ3, b, γ and h have the same |
biological |
meanings as in model (10). The constant β1(0 ≤ β1 < 1) reflects the relation between the birth rate and the population density. For β1 = 0, the model (12) is reduced to a special case of the model (9).
It is easily to have that (12) always has a disease free equilibrium (i. e. boundary equilibrium) E0 = (S0, 0, 0), where
|
|
[b(1 |
|
β |
) |
|
µ |
]2 |
+ 4µ |
b |
|
||
S0 = |
[b(1 − β1) − µ1] + (2µ1 |
− |
1 |
|
− |
1 |
|
1 |
|
. |
|||
|
|
|
|
|
|
||||||||
Furthermore, if |
µ2 + γ |
|
|
|
|
|
|
|
|||||
|
S0 > S ≡ |
|
|
|
|
|
(13) |
||||||
|
|
|
|
, |
|
|
|
|
|||||
|
|
β |
|
|
|
|
|

3 Delayed SIR Epidemic Models |
63 |
then (12) also has an endemic equilibrium (i. e. interior equilibrium) E+ = |
(S , I , R ), where |
|
|
|
|
|
|
|
|
|
|
|
|
|
|
|
γI |
, |
||
I = |
− |
P + |
( |
P 2 − 4βS W Q |
, R = |
||||
2βS W |
µ3 |
||||||||
|
|
γ |
|
|
|||||
W = 1 + |
> 0 , |
|
|
|
|||||
µ3 |
|
|
|
||||||
|
|
|
|
|
|
|
|
P = [µ1S − b(1 − β1)]W + βS (1 + S ) ,
Q= [µ1S − b(1 − β1)](1 + S ) − bβ1 < 0 .
3.4.1Local asymptotic stability analysis
For local asymptotic stability of the disease free equilibrium E0 and the endemic equilibrium E+ of (12), we have the following results:
Theorem 15 (Song and Ma 2006). If S0 < S , then the disease free equilibrium E0 of (12) is locally asymptotically stable for any time delay h. If S0 > S , then E0 is unstable for any time delay h.
Theorem 16 (Song and Ma 2006). If S0 > S , then the endemic equilibrium E+ of (12) is locally asymptotically stable for any time delay h.
3.4.2 Global asymptotic stability of E0
For global asymptotic stability of the disease free equilibrium E0 of (12), we have the following result:
Theorem 17 (Song and Ma 2006). If S0 < S , the disease free equilibrium E0 of (12) is globally asymptotically stable for any time delay h. If S0 = S , E0 is globally attractive for any time delay h.
Theorem 17 shows that, while the endemic equilibrium E+ of (12) is not feasible (i. e. S0 ≤ S ), the disease free equilibrium E0 of (12) is also globally asymptotically attractive for any time delay h. Hence, from the biological point of view, Theorems 16 and 17 suggest the following conjecture, which includes Problem 2 as a special case, may be true:
Problem 3. If S0 > S , then the endemic equilibrium E+ of (12) is also globally asymptotically stable for any time delay h, i. e., in biology, the inequality S0 > S is the threshold for an epidemic disease to occur.
3.4.3 Permanence of (12)
It seems not to be so easy to give a positive answer to Problem 3 or even to Problem 2, but we have the following result which gives a partial answer to Problem 3.
64 Yasuhiro Takeuchi and Wanbiao Ma
Theorem 18 (Song, Ma and Takeuchi 2005). For any time delay h, the inequality S0 > S is necessary and su cient for permanence of (12).
Acknowledgement. The authors thank Japan Student Services Organization (JASSO) and Department of Foreign A airs of University of Science and Technology Beijing (USTB) for their financial support.
References
1.Anderson, R. M. and R. M. May (1979), Population biology of infectious diseases: Part I, Nature, 280, 361–367.
2. Beretta, E., V. Capasso and F. Rinaldi (1988), Global stability results for a generalized Lotka-Volterra system with distributed delays: Applications to predator-prey and epidemic systems, J. Math. Biol., 26, 661–668.
3.Beretta, E. and Y. Takeuchi (1995), Global stability of an SIR epidemic model with time delays, J. Math. Biol., 33, 250–260.
4.Beretta, E. and Y. Takeuchi (1997), Convergence results in SIR epidemic models with varying population size, Nonl. Anal., 28, 1909–1921.
5.Beretta, E., T. Hara, W. Ma and Y. Takeuchi (2001), Global asymptotic stability of an SIR epidemic model with distributed time delay, Nonl. Anal., 47, 4107–4115.
6.Butler, G., H. I. Freedman and P. Waltman (1986), Uniformly persistent systems, Proc. Amer. Math. Soc., 96, 425–430.
7.Chen, L., X. Song and Z. Lu (2002), Mathematical Models and Methods in Ecology, Sichuan Science Press, Chengdu, China.
8.Cooke, K. L. (1979), Stability analysis for a vector disease model, Rocky Mount. J. Math., 9, 31–42.
9.Cooke, K. L. and P. Van Den Driessche (1996), Analysis of an SEIRS epidemic model with two delays, J. Math. Biol., 35, 240–260.
10.Cooke, K. L., P. Van Den Driessche and X. Zou (1999), Interaction of maturation delay and nonlinear birth in population and epidemic models, J. Math. Biol., 39, 332–352.
11.Cushing, J. M. (1977), Integrodi erential Equations and Delays Models in Population Dynamics, Lecture Notes in Biomathematics, Vol.20, Springer-Verlag, New York.
12.Di Liddo, A. (1986), An SIR vector disease model with delay, Math. Model, 7, 793–802.
13.Freedman, H. I. and S. Ruan (1995), Uniform persistence in functional di erential equations, J. Di erential Equations, 115, 173–192.
14.Hale, J. K. (1977), Theory of Functional Di erential Equations, SpringerVerlag, New York.
15.Hale, J. K. and P. Waltman (1989), Persistence in infinitedimensional systems,
SIAM J. Math. Anal., 20, 388–395.
16.Hethcote, H. W. (1976), Qualitative analyses of communicable disease models,
Math. Biosci., 7, 335–356.
17.Hethcote, H. W. and P. Van Den Driessche (2000), Two SIS epidemiological models with delays, J. Math. Biol., 40, 2–26.
3 Delayed SIR Epidemic Models |
65 |
18.Kermack, W. O. and A. G. McKendrick (1927), A contribution to the mathematical theory of epidemics, Proc. Roy. Soc., A115, 700–721.
19.Kuang, Y. (1993), Delay Di erential Equations with Applications in Population Dynamics, Academic Press, New York.
20.Liu, W., S. A. Levin and Y. Iwasa (1986), Influence of nonlinear incidence rate upon the behavior of SIRS epidemiological models, J. Math. Biol., 23, 187–204.
21.Ma, W. and Y. Takeuchi (2004), Asymptotic properties of a delayed SIR epidemic model with density dependent birth rate, Discrete Cont. Dyn. Sys., 4, 671–678.
22.Ma, W., Y. Takeuchi, T. Hara and E. Beretta (2002), Permanence of an SIR epidemic model with distributed time delays, Tohoku J. Math., 54, 581–591.
23.Ma, W., M. Song and Y. Takeuchi (2004), Global stability of an SIR epidemic model with time delay, Appl. Math. Letters, 17, 1141–1145.
24.Ma, Z., Y. Zhou, W. Wang and Z. Jin (2004), Mathematical Models in Epidemiology Dynamics, Science Press, Beijing.
25.McDonald, N. (1978), Time Lags in Biological Models, Lecture Notes in Biomathematics, Vol.27, Springer-Verlag, New York.
26.Mena-Lorca, J. and H. W. Hethcote (1992), Dynamic models of infectious diseases as regulators of population sizes, J. Math. Biol., 30, 693–716.
27.Ruan, S. (2007), Spatial-temporal dynamics in nonlocal epidemiological models, Mathematics for Life Science and Medicine, Eds. Y. Takeuchi et al, SpringerVerlag, Berlin Heidelberg, 107–122.
28.Smith, H. L. (1983), Subharmonic bifurcation in an SIR epidemic model,
J. Math. Biol., 17, 163–177.
29.Song, M. and W. Ma (2006), Asymptotic properties of a revised SIR epidemic model with density dependent birth rate and time delay, Dyn. Conti. Discrete Impul. Sys., 13, 199–208.
30.Song, W. Ma and Y. Takeuchi (2005), Permanence of a delayed SIR epidemic model with density dependent birth rate, J. Compt. Appl. Math., (to appear).
31.Takeuchi, Y. and W. Ma (1999), Stability analysis on a delayed SIR epidemic model with density dependent birth process, Dyn. Conti. Discrete Impul. Sys., 5, 171–184.
32.Takeuchi, Y., W. Ma and E. Beretta (2000), Global asymptotic properties of a delayed SIR epidemic model with finite incubation time, Nonl. Anal., 42, 931–947.
33.Thieme, H. R. (2003), Mathematics in Population Biology, Princeton University Press.
34.Wang, W. (2002), Global behavior of an SEIRS epidemic model with time delay, Appl. Math. Letters, 15, 423–428.

4
Epidemic Models with Population Dispersal
Wendi Wang
Summary. The purpose of this chapter is to outline recent advances of mathematical models in the studies of epidemic diseases in heterogeneous geography. Section 4.1 presents a model with the immigration of infectives from outside the population. Section 4.2 introduces multi patches into epidemic models but assume that the population size in each patch is constant in time. One objective is to discuss conditions under which patches become synchronised. The second objective is to consider the invasion of malaria into a population distributed in distinct patches. In Sect. 4.3, a demographic structure is incorporated into the epidemic models with multi patches. The basic reproduction number of the model is established. Section 4.4 changes the mass action incidence to a standard incidence. Section 4.5 further considers the residence of individuals, which make mathematical models more accurate.
4.1 Introduction
Many mathematical models have been proposed to understand the mechanism of disease transmission. One way for this purpose is to improve classical models to see the e ect of various biological factors on propagation of a disease. First, we can consider nonlinear incidences caused by behavior changes or nonlinear treatment rates (see, for example, Liu et al.(1986, 1987), Ruan and Wang (2003), Wang and Ruan (2004b)). Secondly, we can introduce time delays from the latent period, infection period and recovery period (Cooke and van den Driessche (1996), Feng and Thieme (2000a, 2002b), Beretta et al. (2000, 2002), Wang and Ma (2002a, 2002b)), or consider an age e ect (Gyllenberg and Webb (1990), Inaba (1990)). We can also consider disease transmissions in multiple populations. For example, Xiao and Chen (2001) studied disease transmissions in a predator-prey system, Han et al. (2003) studied disease transmissions in a system of competing species. Most of these papers assume homogeneous space distribution both for populations and disease transmissions.
68 Wendi Wang
Space structure plays an important role in the spread of a disease. Some epidemic diseases have occurred in some regions frequently and were transmitted to other regions due to population dispersal. For example, SARS was first reported in Guangdong Province of China in November of 2002. The emerging disease spread very quickly to some other regions in Mainland China as well as Hong Kong, Singapore, Vietnam, Canada, etc. In March of 2003, the World Health Organization, for the first time in its history, issued a globally warning about the disease. In late June of 2003, the disease was under control globally, but it had spread to 32 countries and regions causing about 800 deaths and more than 8000 infections (see, for example, Wang and Ruan (2004a)). Hence, it is important to use mathematical models to understand the e ect of population dispersal on the spread of a disease. Basically, there are two ways for this purpose. First, we can introduce space variables and use reaction di usion equations (see Murray (1989) and Britton (2003) and the references cited therein). One of the major limitations of di usion models is the assumption of the movements of individuals among direct neighborhoods. In nature many organisms can move or can be transferred over large distances. For example, birds can fly to remote habitats, and human populations can move into other countries in a short time due to modern transportation tools. Further, many populations live in the form of communities, for example, human population live in cities. Thus, it is reasonable to adopt, as an alternative, patch models, in epidemiology. Here, one patch may represent a city or or a biological habitat.
4.2 Epidemic models with immigration of infectives
Communicable diseases may be introduced into a population by the arrival of infectives from outside the population. For example, travellers may return home from a foreign trip with an infection acquired abroad, or individuals who are HIV positive may enter into a prison. For these reasons, Brauer and van den Driessche (2001) proposed an epidemic model with immigration of infectives. They consider an SIS model. Let S(t) be the number of members of a population who are susceptible to an infection at time t, I(t) the number of members who are infective at time t. It is assumed that there is a constant flow A of new members into the population in unit time, of which a fraction p (0 ≤ p ≤ 1) is infective. The model is
S = (1 − p)A − βSI − dS + γI ,
I = pA + βSI |
|
(1) |
− |
(d + γ + α)I , |
|
|
|
where β is the disease transmission coe cient, d is a natural death rate, α is the disease-induced death rate and γ is the recovery rate.
Advantages of (1) are that only one patch is involved and infectives from outside are also included. These make the mathematical analysis much easier.
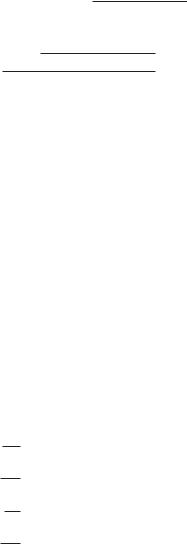
4 Epidemic Models with Population Dispersal
Writing N = S + I, we can transform (1) into
I = pA + βI(N − I) − (d + γ + α)I ,
N = A − dN − αI .
69
(2)
If β = 0, which means that only infectives are those who have entered the population from outside, it is easy to see that every solution approaches the endemic equilibrium (I0, N0) where
I0 = |
pA |
, N0 = |
A |
|
d + γ + α(1 − p) |
. |
d + γ + α |
|
|
||||
|
|
d d + γ + α |
If β > 0, for p > 0, (2) has a unique endemic equilibrium (I , N ) where
(
I = σ + σ2 + 4βA dp(d + α) ,
2β(d + α)
N = (A − αI )/d ,
σ = βA − d(d + γ + α) .
By the Bendixson-Dulac criterion, the endemic equilibrium is globally stable. Then it is easy to see that the number of infectives can be reduced by reducing p or A (i. e., the number of infectives entering the population) or by increasing γ (the recovery rate constant).
4.3 Constant population sizes in each patch
Multi-patch models with epidemic diseases were studied by Lloyd and May (1996, 2004) and Rodríguez and Torres-Sorando (2001). They consider n patches and assume that the population size of each patch remains constant even though individuals move among patches. This implies that the number of births exactly balances the number of deaths in every patch and that individuals do not move permanently from one patch to another.
4.3.1 An SEIS model
In the simplest case of one patch, an SEIR model is:
dS = µN − µS − λS , dt
dE = λS − (µ + σ)E , dt
dI = σE − (µ + γ)I , (3) dt
dR = γI − µR , dt
λ = βI ,
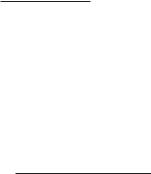
70 Wendi Wang
where S, E, I and R represent the numbers of susceptible, exposed (but not yet infectious), infectious, and recovered individuals respectively, µ is the birth rate and death rate, 1/σ is the average latent period of the disease, 1/γ is the average infectious period, λ is the infection force.
For n patches, it is assumed in paper (Lloyd and May 1986) that the dynamics of disease transmission in patch i is governed by
|
dSi |
|
= µiNi − µiSi − λiSi , |
|
|
|
dt |
|
|
dEi |
|
= λiSi − (µi + σi)Ei , |
||
|
|
dt |
|
|
|
|
dIi |
(4) |
|
|
|
|
|
= σiEi − (µi + γi)Ii , |
|
|
dt |
|
|
|
|
|
|
n |
λi = |
βij Ij , |
|
i=1 |
where Si, Ei, Ii and Ri represent the numbers of susceptible, exposed (but not yet infectious), infectious, and recovered individuals in patch i, respectively; the parameters with subscript i admit similar meanings as those without subscript i; the equation of Ri is dropped because Ri is independent of other variables, Further, Ni is a positive constant. Let Φ be a matrix whose entries equal βij . Φ describes the disease transmission between and within patches. The formulation of the model assumes that there is an epidemiological cross-coupling between patches, but that individuals do not migrate between patches. This might be thought of as arising from a situation in which individuals make short lived visits from their home patch to other patches.
Consider a matrix T = (Tij ) where
βij Niσ
Tij = (µ + σ)(µ + γ)) .
Set
s(T ) = max{Reλ : λ is an eigenvalue of T } .
By Lajmanovich & Yorke (1976), if s(T ) < 1, (4) has a stable disease free equilibrium; if s(T ) > 1, (4) has a unique endemic equilibrium (S , E , I ).
In order to find how the system approaches the endemic equilibrium, we
set λ = n βij I . Let A(Λ) be a matrix whose entry Aij (Λ) is defined by
i i=1 j
Aij = (Λ + µ + λ )δij − σ(Λ + µ) Si βij ,
(Λ + µ + γ)(Λ + µ + σ)
where δii = 1 and δij = 0 if i = j. Then the characteristic equation of (4) at the endemic equilibrium is det A = 0. Its roots determine the stability of the
4 Epidemic Models with Population Dispersal |
71 |
endemic equilibrium. In order to examine the stability analytically, we need to have analytical expressions for Si , Ei , Ii . For this purpose, paper Lloyd and May (1986) considers a simplified case where each patch is of the same population size (Ni = N ). Further, the contact rate is the same within each patch, and another (usually di erent and smaller) rate between each pair of distinct patches, which are given by
βij =
β , ifi = j ,
β , otherwise
with 0 ≤ ≤ 1. Then, for a special case where (4) is reduced to an SIR model (letting σ → ∞), the Φ matrix has eigenvalues given by
Γ = β(1 − ) |
|
||||
repeated n − 1 times, and |
|
|
|
|
|
Γ = β(n + 1 − ) . |
|
||||
A characteristic root Λ satisfies the following equation |
|
||||
(Λ + µR0) |
Λ + µ + γ |
R0 = Γ , |
(5) |
||
|
|
||||
(Λ + µ)N |
|||||
where |
|
|
|
|
|
|
|
N |
n |
|
|
R0 = |
|
|
βij . |
|
|
|
|
|
|
||
|
µ + γ |
|
|
j=1
Since Λ can be solved from (5), paper (Lloyd and May 1986) proved that the endemic equilibrium is stable and the patches often oscillate in phase for all but the weakest between patch coupling.
4.3.2 A malaria model
Now, we suppose that the habitat is partitioned into k patches. Ni and Xi(t) are the numbers of humans and infected humans, respectively, at time t in patch i(i = 1, . . . , k), Mi and Yi(t) represent the number of mosquitoes and infected mosquitoes, respectively, at time t in patch i(i = 1, . . . , k). Let us assume that humans can travel between patches but mosquitoes can not. Further, we assume that a fraction vij of the time devoted by humans to reside in patch i per unit time, is devoted to visit patch j(j = i, j = 1, . . . , k). After the visit, these humans return to their home patches. Under the above
72 Wendi Wang
assumptions, paper (Rodríguez and Torres-Sorando 2001) proposed the following model:
dXi |
= b(Ni − Xi(t))Yi(t) − gXi(t) + b(Ni − Xi(t)) |
vij Yj (t) , |
|
|
|
dt |
|
||
|
dYi |
|
j=i |
(6) |
|
= b(Mi − Yi(t))Xi(t) − mYi(t) + b(Mi − Yi(t)) |
vjiXj (t) , |
||
|
dt |
|
||
|
|
|
j=i |
|
i = 1, . . . , k ,
where b is the disease transmission coe cient due to contacts from susceptible humans and infectious mosquitoes, or susceptible mosquitoes and infectious humans, g is the recovery rate of infected human individuals, m is the recovery rate of infected mosquitoes.
Tow patterns of spatial array were considered in Rodríguez and TorresSorando (2001). The first is a unidimensional one, in which the k patches are arranged as cells in a row. This array can simulate the spatial distribution of patches along a coast. The second array is a bidimensional one, in which the k patches are arranged in a rectangle whose four sides are of the smae length or number of cells. This bidimensional array can simulate a general spatial distribution on a land surface.
1 |
|
2 |
|
3 |
4 |
|
5 |
|||
|
|
|
|
|
|
|
|
|
|
|
|
|
Fig. 4.1. Unidimensional array with k = 5 |
||||||||
|
|
|
|
|
|
|
|
|
|
|
|
|
1 |
|
2 |
|
|
3 |
|
|
|
|
|
|
|
|
|
|
|
|
|
|
|
|
4 |
|
5 |
|
|
6 |
|
|
|
|
|
|
|
|
|
|
|
|
|
|
|
|
7 |
|
8 |
|
|
9 |
|
|
|
|
|
|
|
|
|
|
|
|
|
|
Fig. 4.2. Bidimensional array with k = 9