
- •Distribution Overview
- •Discrete Distributions
- •Continuous Distributions
- •Probability Theory
- •Random Variables
- •Transformations
- •Expectation
- •Variance
- •Inequalities
- •Distribution Relationships
- •Probability and Moment Generating Functions
- •Multivariate Distributions
- •Standard Bivariate Normal
- •Bivariate Normal
- •Multivariate Normal
- •Convergence
- •Statistical Inference
- •Point Estimation
- •Empirical distribution
- •Statistical Functionals
- •Parametric Inference
- •Method of Moments
- •Maximum Likelihood
- •Delta Method
- •Multiparameter Models
- •Multiparameter delta method
- •Parametric Bootstrap
- •Hypothesis Testing
- •Bayesian Inference
- •Credible Intervals
- •Function of parameters
- •Priors
- •Conjugate Priors
- •Bayesian Testing
- •Exponential Family
- •Sampling Methods
- •The Bootstrap
- •Rejection Sampling
- •Importance Sampling
- •Decision Theory
- •Risk
- •Admissibility
- •Bayes Rule
- •Minimax Rules
- •Linear Regression
- •Simple Linear Regression
- •Prediction
- •Multiple Regression
- •Model Selection
- •Non-parametric Function Estimation
- •Density Estimation
- •Histograms
- •Kernel Density Estimator (KDE)
- •Smoothing Using Orthogonal Functions
- •Stochastic Processes
- •Markov Chains
- •Poisson Processes
- •Time Series
- •Stationary Time Series
- •Estimation of Correlation
- •Detrending
- •ARIMA models
- •Causality and Invertibility
- •Spectral Analysis
- •Math
- •Gamma Function
- •Beta Function
- •Series
- •Combinatorics
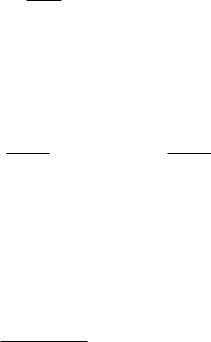
17.3Bayes Rule
Bayes rule (or Bayes estimator) |
|
|
|
|
||||||
r(f; ) = inf |
r(f; ) |
|
|
|
|
|
||||
|
b |
|
j |
e |
|
|
|
|
|
|
|
(x) = inf r(e |
|
|
|
|
|
R |
|
|
|
|
b |
x) |
8 |
|
) |
|
|
j |
x)f(x) dx |
|
|
|
x = r(f; ) = r( |
|
|||||||
|
b |
|
|
|
|
b |
b |
|
|
Theorems
Squared error loss: posterior mean
Absolute error loss: posterior median
Zero-one loss: posterior mode
17.4Minimax Rules
Maximum risk
|
sup R( ; ) |
|
|
R(b) = |
R(a) = sup R( ; a) |
||
|
b |
|
Minimax rule
sup R( ; b) = inf R(e) = inf sup R( ; e)
|
e |
e |
b= Bayes rule ^ 9c : R( ; b) = c
Least favorable prior
bf = Bayes rule ^ R( ; bf ) r(f; bf ) 8
18 Linear Regression
De nitions
Response variable Y
Covariate X (aka predictor variable or feature)
18.1Simple Linear Regression
Model |
|
E [ i j Xi] = 0; V [ i j Xi] = 2 |
|
Yi = 0 + 1Xi + i |
|||
Fitted line |
b |
|
|
|
|
|
|
Predicted ( tted) values |
r(x) = b0 + b1x |
||
Residuals |
Ybi = r(Xi) |
|
|
|
|
b |
+ 1Xi |
^i |
= Yi Yi = Yi 0 |
||
|
b |
b |
b |
Residual sums of squares (rss)
n
X
rss(b0; b1) = ^2i
i=1
Least square estimates |
|
|
|
|
|
|
|
|
|
|
|
|
|
|
|
|
|
|
|
|
|
T |
= (b0; b1) |
T |
|
min rss |
|
|
|
|
|
|
|||||||
|
|
b |
|
: |
b0;b1 |
|
|
|
|
|
|
|
|
||||||
|
|
|
|
|
|
|
|
|
|
|
|
|
|
|
|
|
|
|
|
0 = Yn |
1Xn |
|
|
|
|
|
|
|
= |
|
|
n |
|
|
|
|
|||
b1 |
bn |
|
|
|
|
|
|
|
|
|
|
|
|
|
|||||
P |
n |
|
|
|
|
Xn)2 |
|
P |
n |
i=1 Xi2 |
|
|
|
|
|||||
i=1 |
(Xi |
|
Yn) |
|
|
nX2 |
|||||||||||||
= |
|
i=1(Xi |
|
Xn)(Yi |
|
|
|
i=1 XiYi |
|
nXY |
|||||||||
|
P |
|
|
|
|
|
|
|
|
|
|
P |
|
|
|
|
|
||
b |
|
|
|
|
|
|
|
|
|
|
|
|
|
|
|
E h j Xni = |
0 |
|
|
|
|
|
|
|
|
|
|
|
|
|
|
|
|
|
|
|
||||||
|
|
1 |
|
|
|
|
|
|
|
|
|
|
|
|
|
|
|
|
|
|
|
|||||||
|
|
b |
|
|
|
|
2 |
|
n 1 |
|
|
n Xi2 |
|
|
|
|
|
|
||||||||||
|
|
j |
n |
|
|
|
|
|
Xn |
|
|
|||||||||||||||||
|
|
|
|
|
|
nsX2 |
|
|
|
|
|
Xn |
|
1 |
|
|
|
|
||||||||||
|
|
hb |
|
|
i |
|
|
|
|
|
|
|
|
P |
|
|
|
|
|
|
|
|
|
|
|
|||
|
|
|
|
|
|
|
|
|
|
|
|
n=1 Xi2 |
|
|
|
|
|
|
||||||||||
|
|
V X = |
|
|
|
|
|
|
|
|
|
|
i=1 |
|
|
|
|
|
|
|
||||||||
|
|
se( 0) = sXpnr |
P |
i n |
|
|
|
|
|
|
|
|||||||||||||||||
|
|
|
b |
b |
|
|
b |
|
|
|
|
|
|
|
|
|
|
|
|
|
|
|
|
|||||
|
|
|
|
|
|
|
|
|
|
|
|
|
|
|
|
|
|
|
|
|
|
|
|
|
||||
|
|
|
|
|
|
|
|
|
|
|
|
|
|
|
|
|
|
|
|
|
|
|
|
|||||
|
|
se( 1) = |
sXbp |
|
|
|
|
|
|
|
|
|
|
|
|
|
|
|
|
|
|
|
||||||
|
|
n |
|
|
|
|
|
|
|
|
|
|
|
|
|
|
|
|
|
|
||||||||
|
|
|
b |
bn |
|
|
|
|
|
|
|
|
|
|
|
|
|
|
|
1 |
|
|
|
n |
|
|
||
|
|
|
|
|
|
|
|
|
|
|
|
|
|
|
|
|
|
|
|
|
|
|
||||||
where s2 |
= n 1 |
|
|
(X |
X |
n |
)2 |
and 2 = |
|
|
|
|
|
^2 |
(unbiased estimate). |
|||||||||||||
i=1 |
n 2 i=1 |
|||||||||||||||||||||||||||
|
X |
|
|
|
i |
|
|
|
|
|
|
|
|
|
|
|
i |
|
||||||||||
Further |
properties: |
|
|
|
|
|
|
|
|
|
|
|
|
|
b |
|
|
|
P |
|
|
|||||||
|
|
|
P |
|
|
|
|
|
|
|
|
|
|
|
|
|
|
|
|
|
|
PP
Consistency: b0 ! 0 and b1 ! 1
Asymptotic normality:
|
|
|
|
0 0 |
D |
|
|
(0; 1) |
|
and |
|
|
1 1 |
|
D |
(0; 1) |
|
|
|
|||||||||||||||
|
|
|
|
|
|
|
|
|
|
|
|
|
||||||||||||||||||||||
|
|
|
|
bse( 0) |
! N |
|
|
bse( 1) |
! N |
|
|
|||||||||||||||||||||||
|
|
|
|
|
|
|
|
|
|
|
|
|
|
|
|
|
|
|
|
|||||||||||||||
|
|
|
|
|
b |
|
|
|
|
|
|
|
|
|
|
|
|
|
|
|
b |
0 |
|
|
1 |
|
|
|
|
|
|
|
||
|
Approximate 1 |
|
bcon dence intervals for band : |
|
|
|
|
|
|
|
||||||||||||||||||||||||
|
|
|
|
|
|
|
|
|
|
|
||||||||||||||||||||||||
|
|
|
|
|
|
|
b0 z =2se(b0) |
and |
b1 z =2se(b1) |
|
|
|
|
|
||||||||||||||||||||
|
|
|
|
|
|
0 |
|
|
1 |
|
|
|
b |
1 |
|
1 |
6 |
|
|
|
|
|
|
|
b |
j |
|
j |
|
|
=2 |
|
||
|
|
Wald test for |
|
H : |
|
= 0 vs. H : = 0: reject H0 if |
|
W |
|
> z where |
||||||||||||||||||||||||
R2 |
|
W = b1=se(b1). |
|
|
|
|
|
|
|
|
|
|
|
|
|
|
|
|
|
|
|
|
|
|
|
|
|
|
|
|
|
|||
|
|
b |
|
|
n |
|
|
|
|
|
|
|
|
|
|
|
|
|
|
n |
|
|
|
|
|
|
|
|
|
|
|
|
||
|
|
|
|
|
|
|
|
|
|
|
|
|
|
|
|
|
|
|
|
|
|
|
|
|
|
|
|
|
|
|
||||
|
|
R2 = |
|
|
i=1 |
(Yi |
Y )2 |
|
= 1 |
|
|
|
|
|
i=1 ^i2 |
|
= 1 |
|
|
rss |
|
|
||||||||||||
|
|
|
|
|
|
|
|
|
|
|
|
|
|
|
|
|
|
|
|
|
|
|
||||||||||||
|
|
Pi=1 |
b |
|
|
|
|
P |
i P |
|
|
|
|
tss |
|
|
||||||||||||||||||
|
|
|
|
Y )2 |
|
|
|
|
Y )2 |
|
18 |
|||||||||||||||||||||||
|
|
|
|
|
n |
|
(Yi |
|
|
n |
(Yi |
|
|
|
||||||||||||||||||||
|
|
|
P |
|
|
|
|
|
|
|
|
|
|
|
=1 |
|
|
|
|
|
|
|
|
|
|
|
|
|
|
|
||||
|
|
|
|
|
|
|
|
|
|
|
|
|
|
|
|
|
|
|
|
|
|
|
|
|
|
|
|
|
|
|
|
|
|
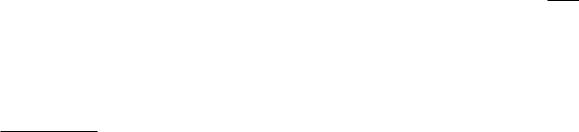
Likelihood
|
n |
n |
n |
|
|
|
|
|
|
iY |
Y |
Y |
|
|
|
|
|
L = |
f(Xi; Yi) = |
fX(Xi) fY jX(Yi j Xi) = L1 L2 |
|
|
||||
|
=1 |
i=1 |
i=1 |
|
|
|
|
|
|
n |
|
|
|
|
|
|
|
|
iY |
fX(Xi) |
|
|
|
|
|
|
L1 = |
|
|
|
|
|
|
||
L2 |
=1 |
fY jX(Yi j Xi) / n exp ( 2 2 |
|
Yi ( 0 1Xi) |
|
) |
||
= |
|
|
||||||
|
n |
|
1 |
|
|
|
2 |
|
|
i=1 |
|
|
|
i |
|
||
|
Y |
|
|
|
X |
|
|
|
Under the assumption of Normality, the least squares parameter estimators are also the MLEs, but the least squares variance estimator is not the MLE
2 = |
1 n |
^i2 |
||
n |
|
|||
|
|
|||
|
=1 |
|
||
b |
|
Xi |
|
|
|
|
|
18.2 Prediction
Observe X = x of the covariate and want to predict their outcome Y .
|
Y = 0 + 1x |
|
|
|
+ 2x Cov 0; 1 |
|||||
V Y = V 0 |
+ x2V 1 |
|||||||||
h |
b |
b b |
|
|
h i |
|
|
h |
i |
|
i |
h i |
|
|
|
|
|||||
Prediction interval |
b |
b |
|
|
b |
|
|
b |
b |
|
|
2 |
= 2 |
|
in=1(Xi X )2 |
+ 1 |
|
||||
|
n |
|
n |
|
|
|
2 |
|
|
|
|
|
|
i |
(Xi |
|
X) j |
|
|||
|
b |
P |
|
|
||||||
|
b |
P |
|
|
|
|
|
Yb z =2 bn
18.3Multiple Regression
|
|
|
Y = X + |
|
|
|
|
where |
0X...11 ... |
X...1k 1 = |
0 ...11 |
|
0 ...11 |
||
X = |
= |
||||||
|
BXn1 |
|
XnkC |
B kC |
|
B nC |
|
Likelihood |
@ |
A |
@ A |
|
@ A |
||
L( ; ) = (2 2) n=2 exp |
2 2 rss |
||||||
|
|
|
|
1 |
|
|
|
|
|
|
|
|
|
N |
|
|
|
|
|
|
|
|
|
rss = (y X )T (y X ) = kY X k2 = |
Xi |
|
|||||
(Yi xiT )2 |
|||||||
|
|
|
|
|
|
=1 |
|
If the (k k) matrix XT X is invertible, |
|
|
|
|
|
|
|
||||||||
|
|
|
|
|
|
= (XT X) 1XT Y |
|
|
|||||||
|
V |
|
Xnb |
= 2(XT X) 1 |
|
|
|
||||||||
|
|
h |
j |
|
|
i |
|
2 |
(X |
T |
X) |
1 |
|
||
|
|
b |
|
|
|
|
; |
|
|
|
|||||
Estimate regression function |
|
|
|
b |
N |
|
|
|
|
|
|
||||
|
|
|
|
|
|
|
|
k |
|
|
|
|
|
|
|
|
|
|
|
|
r(x) = |
jxj |
|
|
|
|
|||||
|
|
|
|
|
|
b |
=1 |
b |
|
|
|
|
|
||
Unbiased estimate for 2 |
|
|
|
|
Xj |
|
|
|
|
|
|||||
|
|
|
|
|
1 |
|
|
n |
|
|
|
|
|
|
|
|
2 |
= |
|
|
|
|
^i2 |
|
^ = X Y |
||||||
|
|
|
|
|
|
||||||||||
mle |
n |
|
k |
||||||||||||
b |
|
|
|
|
|
Xi |
|
|
|
|
b |
|
|
||
|
|
|
|
|
|
|
|
=1 |
|
|
|
|
|
|
|
|
|
= X |
2 = |
n k |
2 |
|
|
||||||||
|
|
b |
|
|
|
|
b |
|
|
n |
|
|
|
|
1 Con dence interval
bj z =2seb(bj)
18.4Model Selection
Consider predicting a new observation Y for covariates X and let S J denote a subset of the covariates in the model, where jSj = k and jJj = n. Issues
Under tting: too few covariates yields high bias
Over tting: too many covariates yields high variance
Procedure
1.Assign a score to each model
2.Search through all models to nd the one with the highest score
Hypothesis testing
H0 : j = 0 vs. H1 : j 6= 0 8j 2 J
Mean squared prediction error (mspe)
h i
mspe = E (Yb(S) Y )2
Prediction risk
nn
h i
XX
R(S) = |
mspe = |
i=1 E |
(Y |
(S) |
|
Y )2 |
|
i=1 |
i |
bi |
|
|
i |
19 |